Top 20 Analytics Case Studies in 2024
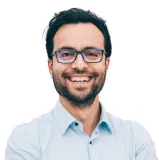
Although the potential of Big Data and business intelligence are recognized by organizations, Gartner analyst Nick Heudecker says that the failure rate of analytics projects is close to 85%. Uncovering the power of analytics improves business operations, reduces costs, enhances decision-making , and enables the launching of more personalized products.
In this article, our research covers:

How to measure analytics success?
What are some analytics case studies.
According to Gartner CDO Survey, the top 3 critical success factors of analytics projects are:
- Creation of a data-driven culture within the organization,
- Data integration and data skills training across the organization,
- And implementation of a data management and analytics strategy.
The success of the process of analytics depends on asking the right question. It requires an understanding of the appropriate data required for each goal to be achieved. We’ve listed 20 successful analytics applications/case studies from different industries.
During our research, we examined that partnering with an analytics consultant helps organizations boost their success if organizations’ tech team lacks certain data skills.
Enterprise | Industry of End User | Business Function | Type of Analytics | Description | Results | Analytics Vendor or Consultant |
---|---|---|---|---|---|---|
Fitbit | Health/ Fitness | Consumer Products | IoT Analytics | Better lifestyle choices for users. | Bernard Marr&Co. | |
Dominos | Food | Marketing | Marketing Analytics | Increased monthly revenue by 6%. | Google Analytics 360 and DBI | |
Brian Gravin Diamond | Luxury/ Jewelry | Sales | Sales Analytics | Improving their online sales by understanding user pre-purchase behaviour. | New line of designs in the website contributed to 6% boost in sales. | Google Analytics Enhanced Ecommerce |
* | Marketing Automation | Marketing | Marketing Analytics | Conversions improved by the rate of 10x | Google Analytics and Marketo | |
Build.com | Home Improvement Retail | Sales | Retail Analytics | Providing dynamic online pricing analysis and intelligence | Increased sales & profitability Better, faster pricing decisions | Numerator Pricing Intel and Numerator |
Ace Hardware | Hardware Retail | Sales | Pricing Analytics | Increased exact and ‘like’ matches by 200% across regional markets. | Numerator Pricing Intel and Numerator | |
SHOP.COM | Online Comparison in Retail | Supply Chain | Retail Analytics | increased supply chain and onboarding process efficiencies. | 57% growth in drop ship orders | SPS Commerce Analytics and SPS Commerce |
Bayer Crop Science | Agriculture | Operations | Edge Analytics/IoT Analytics | Faster decision making to help farmers optimize growing conditions | AWS IoT Analytics AWS Greengrass | |
Farmers Edge | Agriculture | Operations | Edge Analytics | Collecting data from edge in real-time | Better farm management decisions that maximize productivity and profitability. | Microsoft Azure IoT Edge |
Lufthansa | Transportation | Operations | Augmented Analytics/Self-service reporting | Increase in the company’s efficiency by 30% as data preparation and report generation time has reduced. | Tableau | |
Walmart | Retail | Operations | Graph Analytics | Increased revenue by improving customer experience | Neo4j | |
Cerved | Risk Analysis | Operations | Graph Analytics | Neo4j | ||
Nextplus | Communication | Sales/ Marketing | Application Analytics | With Flurry, they analyzed every action users perform in-app. | Boosted conversion rate 5% in one month | Flurry |
Telenor | Telco | Maintenance | Application Analytics | Improved customer experience | AppDynamics | |
Cepheid | Molecular diagnostics | Maintenance | Application Analytics | Eliminating the need for manual SAP monitoring. | AppDynamics | |
* | Telco | HR | Workforce Analytics | Finding out what technical talent finds most and least important. | Improved employee value proposition | Crunchr |
Hostelworld | Vacation | Customer experience | Marketing Analytics | 500% higher engagement across websites and social | Adobe Analytics | |
Phillips | Retail | Marketing | Marketing Analytics | Testing ‘Buy’ buttons increased clicks by 20%. | Adobe | |
* | Insurance | Security | Behavioral Analytics/Security Analytics | Identifying anomalous events such as privileged account logins from | Securonix | |
Under Armour | Retail | Operations | Retail Analytics | IBM Watson |
*Vendors have not shared the client name
For more on analytics
If your organization is willing to implement an analytics solution but doesn’t know where to start, here are some of the articles we’ve written before that can help you learn more:
- AI in analytics: How AI is shaping analytics
- Edge Analytics in 2022: What it is, Why it matters & Use Cases
- Application Analytics: Tracking KPIs that lead to success
Finally, if you believe that your business would benefit from adopting an analytics solution, we have data-driven lists of vendors on our analytics hub and analytics platforms
We will help you choose the best solution tailored to your needs:
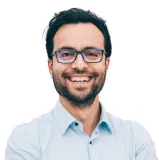
Next to Read
14 case studies of manufacturing analytics in 2024, iot analytics: benefits, challenges, use cases & vendors [2024].
Your email address will not be published. All fields are required.
Related research
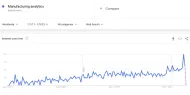
Top 10 Manufacturing Analytics Use Cases in 2024
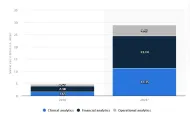
Top 10 Healthcare Analytics Use Cases & Challenges in 2024
Why Attend?
- Live Online
- 1,00,000+ people attended since 2009
- Rs 1999 FREE
- Certificate of Participation
- An exclusive Surprise

10 Best Google Analytics Case Studies
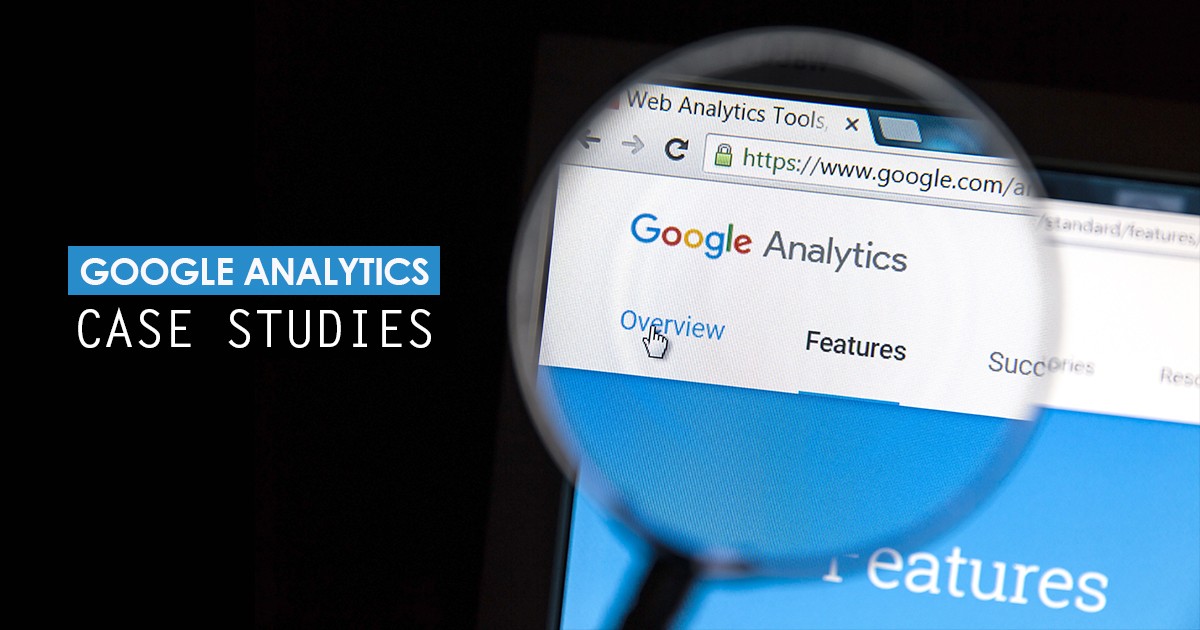
Best Google Analytics Case Studies
Google Analytics has various products under its umbrella such as Google Analytics, Google Analytics 360, Google Tag Manager, Google Big Query etc. These products have assisted many big brands to achieve their milestones with their new and innovative approach. The power of these platforms has been beautifully captured in these best Google Analytics case studies.
1. Revenue shoot-up of Dominos
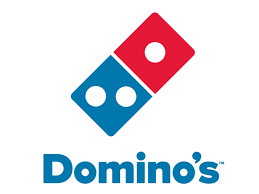
Ordering a pizza nowadays is a piece of cake, isn’t it?
Well, it is for end users. Let’s say, you are browsing your Facebook feed, you see Dominos has 1+1 offer for Wednesday. You are like whatever, I am not ordering today. Then you are watching a video on YouTube, Dominos shows its mouth-watering cheese burst pizza, you are tempted, but you still are saying no. Finally you meet your friend in the evening and decide to have dinner outside. Now the place on top of your mind is dominos and you hit the store. Well, Dominos got you!
There were multiple influencing stages in your purchase and there are millions of people out there who follow numerous stages. It is crucial for Dominos to connect and analyze users’ cross-channel & cross-device behaviour and also connect online and offline behaviour.
That’s what they did with Google analytics 360 product and partnership with DBI (Digital Business Intelligence) company. This Google Analytics case study has captured the essence of Dominos strategy.
Though Dominos has word class analytics solutions to measure their every marketing effort, these were in silos. Dominos knew there is a big ocean of opportunities available once they eradicate these silos and merge them. That’s what they did with Google Big Query. DBI developed a custom BigQuery Solution for Dominos to store and fetch massive data of Dominos. It also helped Dominos to connect their analytics and CRM data seamlessly to connect online & offline data.
The result says it all;
- Increased monthly revenue by 6%
- Reduced ad spending cost by 80% year on year
2. Donations flow in for Cancer.org
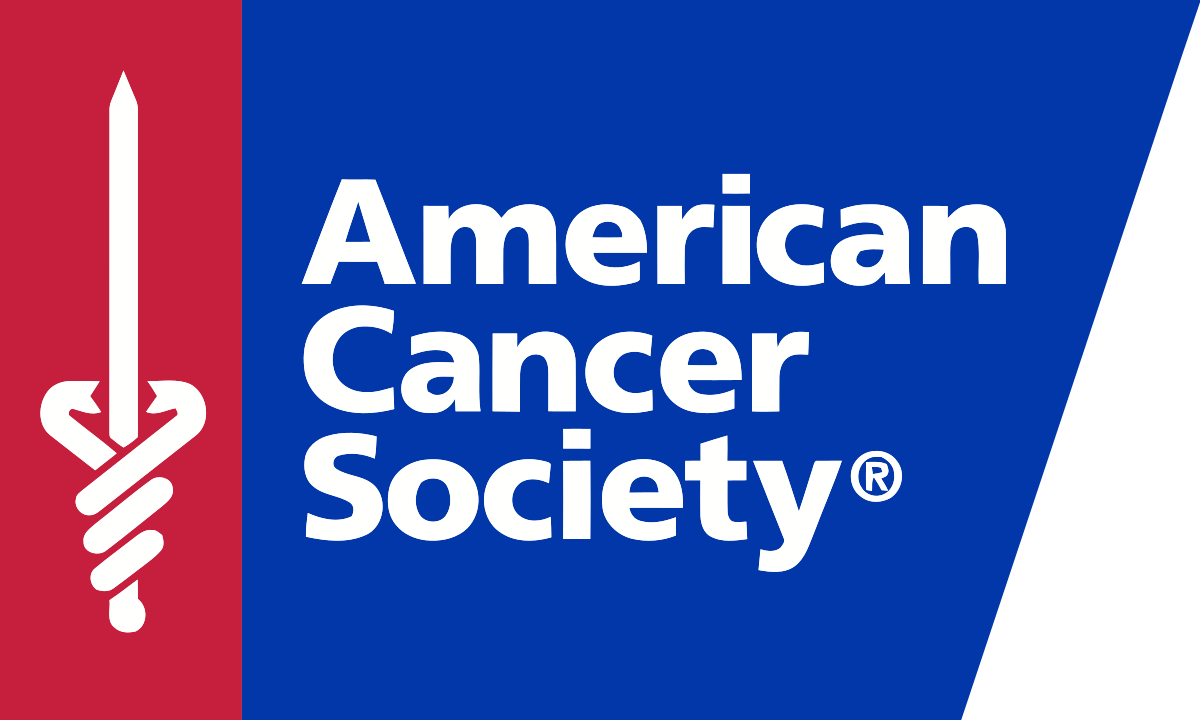
American cancer society has worked for 100 years to make the world to eradicate cancer. The company had realized the need to know how users consume their website and their purpose. This would help them to channelize their marketing efforts and reach their revenue goal.
The organization tied up with Search Discovery agency which is an authorized reseller of Google Analytics.
The first step; they created 3 types of users on their website; Information seekers, Event Participants and Donors. Next step was to understand each segments’ goals using Custom Dimensions of Google Analytics. Once the goals of each segment were known, they created a scoring system for each dimension using the custom metric to check whether they have met their goals. They also designed remarketing campaigns for these audiences and customized the content for them.
End Result: Revenue jumped by 5.4% year on year.
3. Brian Gavin Diamonds increased Customer Acquisition
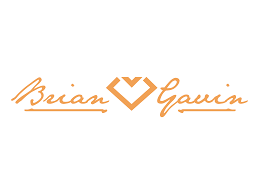
Brian Gravin Diamond are Texas-based Custom diamond jewelry makers. Most of their sales come from their e-commerce website. They had a goal to improve their online sales by understanding user pre-purchase behaviour.
Along with Google Analytics, they decided to implement Google’s Enhanced Ecommerce to achieve their goal. One of the best find out was that their new line of designs has cropped up an interest in their website visitors which contributed to 6% boost in sales. They decided to launch a new line of similar inventory in the fall.
Pertaining to their cart abandonment trend, they learned that they have lost around $500K. They identified there is no proper way to bring back card abandons. They build a guest checkout flow for these users to complete the purchase.
End Result: 60% increase in checkout to the payment page.
4. Revamped Social Media Strategy of Fairmont Hotels
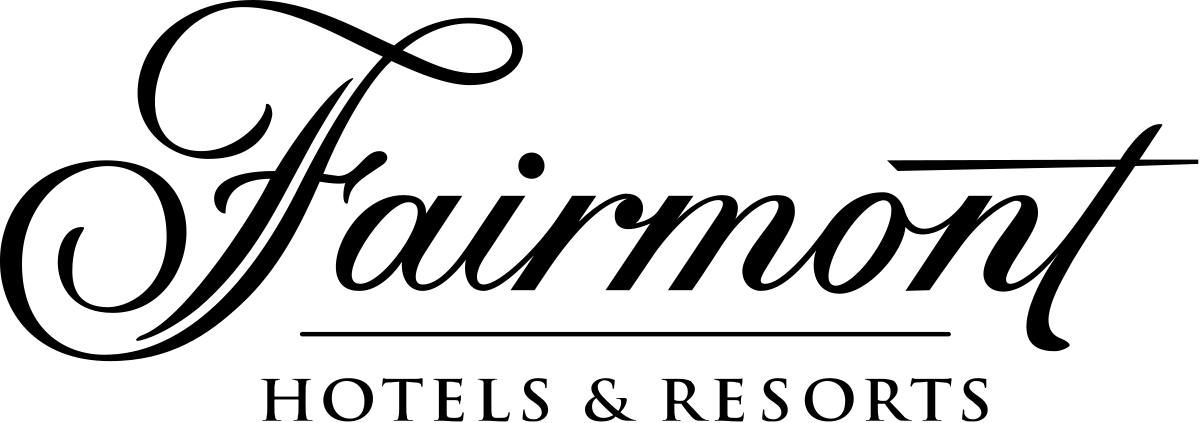
Fairmont is a luxury line of hotels having 60 distinctive hotels across the world. The company generates a lot buzz on social media channels especially twitter. Generating buzz is one thing and measuring the effectiveness of the buzz is other. This one of the top Google Analytics case studies explains Fairmont’s strategy.
The company decided to track the quality of traffic from Twitter. Normally traffic from third-party sites including social media is shown as a referral in analytics. So if anyone clicks a link of Fairmont on the Twitter platform, it is reflected in the referral traffic of Twitter in analytics, but if this link is copied and shared to others platforms like email, WhatsApp etc., the source would be shown as direct.
To make sure the aggregation of the source is accurate, the company decided to use URL builder, in which a link can be given parameters like source, medium, campaign name etc., which lets the company track the source of twitter traffic irrespective of where the link is clicked on.
Result: Better understanding of social media traffic.
5. 10X higher conversion rate for Marketo
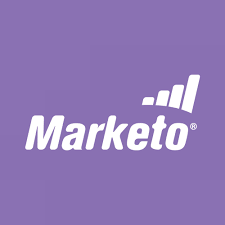
Marketo is a leading marketing automation company associated with numerous B2C & B2B companies. The company had a goal to improve their conversion rate with the aid of their Real-Time Personalization product and Google Analytics.
Marketo merged the data of their website visitors’ characteristics like industry vertical, the product they are interested in etc. by sending the data to Google analytics in the form of events and the demographic and behaviour data from Google Analytics. This led to the creation of detailed audience segments based on product interest and demographic data. They created awesome remarketing campaigns in ad words and served the audience with most relevant data.
Result: Conversions improved by the rate of 10x compared to traditional display marketing.
Accelerate Your Career or Business Growth! Learn & Leverage Digital Marketing Join Free Live Orientation Session Join Free Live Orientation Session
6. ROAS improved by 30% for Panasonic
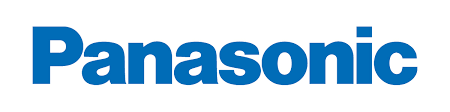
Renowned brands have realized the power of digital marketing and so is Panasonic. Panasonic decided to integrate all their marketing data of all the websites to a single platform which is Google Analytics 360 along with the agency NRI Netcom. With this one platform to view all their marketing data, they soon got two powerful insights;
- Most of the purchases of their products happen during life-changing events like marriage, moving to a new city etc.
- The most commonly purchased combination of products
They created audience lists based on the products the users showed interest into and put this data across ad words, double-click, Google display network to remarket with the relevant content. With the insight of the popular combination of purchases, they started remarketing the other relevant products for the user in a particular segment; eg; users were marketed with speakers who previously showed interest in TV.
End Result: Improved Return on Ad Spend (ROAS) by 30%.
7. 130% increase in conversion rate for Top Tarif
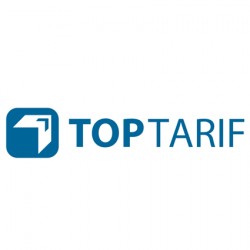
Top Tarif is a price comparison website of Germany. The objective of the company was to increase conversion by maintaining the same cost per conversion. They chose to fine-tune their remarketing approach by making the lists more granular.
They built remarketing lists based on the users’ previous web usage, depth of price comparison, keywords used to reach the website, the date of last visit etc. They focused on users who would more likely convert.
Result: 130% growth in conversions and 31.5% improvement in conversion rate.
8. Top Talents flow to “Teach For America”
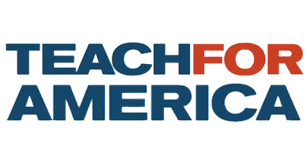
This is one of the best Google Analytics case studies.
Teach For America is an organization focused on providing quality education to underprivileged children of America. Their main resource is the young, educated, passionate and responsible citizens of the country. To accomplish this goal in the competitive market, they tied up with LunaMetric to leverage on the power of Google Analytics.
They created remarketing lists based on the initial information provided applicants in terms of their GDP, educational stream, career status etc. and imported the data to Google Analytics in the form of dimensions. They then advertised to these audiences on search platform when they research anything related to their stream, career etc.
Result: 57% increased conversion with audience targeting.
9. Remarketing yields 1300% ROI for Watchfinder

Watchfinder is a UK based retailer of premium pre-owned watches. Considering the fact that their average order value is more than 3,500 Euros, the company was aware of the fact that the purchase lead time would take weeks to months. Also, less than 1% of purchases happened on the first visit. There was a need for Watchfinder to convince users who showed interest in this meantime.
Watchfinder collaborated with Periscopix, a Google Analytics Premium Partner and created remarketing campaigns to do the magic. They created 20 remarketing lists based on the user location, stage in the purchase funnel and also the brand they showed interest in. They remarketed to these audiences and improved their results.
Result: 1300% ROI and 13% increase in average order value.
10. 200% transaction rates for Alfa Strakhovanie
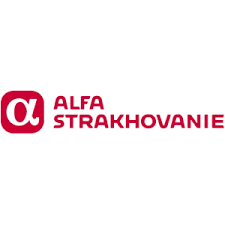
Alfa Strakhovanie is Russia’s largest insurance company in the travel and auto sector. Their goal was to know their most valuable customers and decide how much to spend on them. The catch with insurance companies is they will know the real value of user once the policy expires without any claims. Their objective was to revise their policy pricing based on the segment of the user, eg; a person of 18-24 age with speedy cars and a new driving licensee is riskier.
The company partnered with AGIMA analytics agency. They used Enhanced E-commerce solutions and custom metrics to analyze the segment data and drive the results.
Results: Transactions rates were doubled.
These are some of the brands which are boosting their business with the aid of data provided by Google Analytics and its products. These Google Analytics case studies give a ready reckoner for beginners. One can also derive many strategies by following the ideas used in these case studies.
Remarketing is the one unmatched feature in the world of Google Analytics. Most of the case studies mentioned here have capitalized on this feature. Use it wisely to deliver the best results.
Keeping a track of the latest happenings in Digital Marketing can be daunting, learning Digital Marketing all alone unbearably troublesome. Is that what you feel too? If yes, then enroll in our Certified Digital Marketing Master Course.
Table of Contents
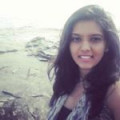
Leave a Comment Cancel Reply
Your email address will not be published. Required fields are marked *
Save my name, email, and website in this browser for the next time I comment.

- Covers all Digital Marketing Techniques
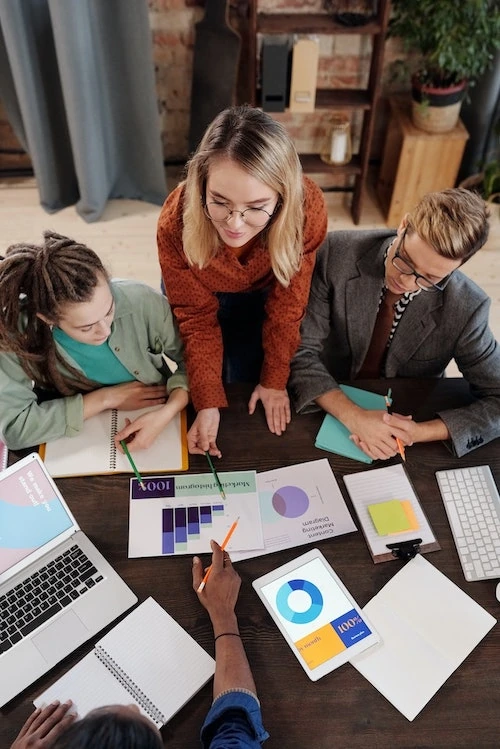
- Digital Media Mastery (with Paid Media Expertise)
Discuss With A Career Advisor
Not Sure, What to learn and how it will help you?
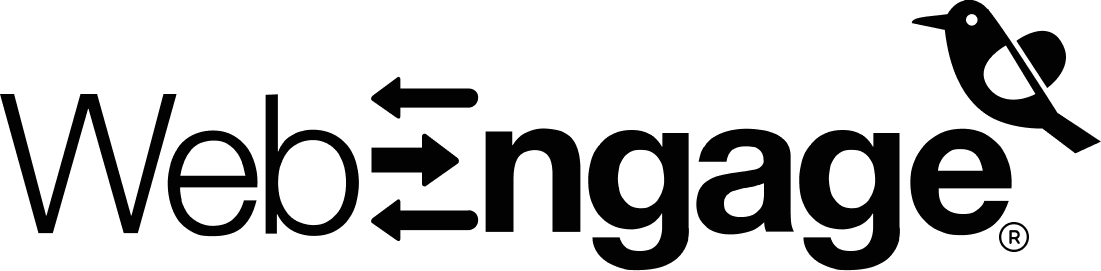
- Generative AI
- Engagement Score
- Predictive Segmentation
- Catalog & Recommendation
- Intelligent Campaign Orchestration
- Journey Designer
- Mobile Push Notification
- Email Marketing
- Web Push Notification
- WhatsApp Engagement
- Facebook Ads
- SMS Marketing
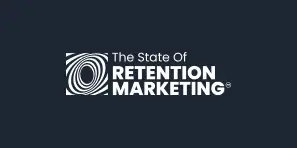
The State of Retention Marketing
The world’s first retention-first magazine to help brands grow businesses sustainably through riveting stories, use cases, and more.
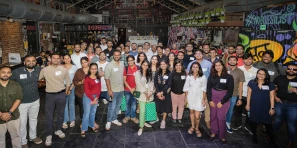
Retention DNA
Retention DNA is a collaborative hub where growth-minded individuals converge to ideate, collaborate, and solve collectively.
- Request a Demo Dashboard
- referral marketing
- Creator Economy
- State of Careers in Retention Marketing Report
- AI and ML Marketing Automation
- Predictive analytics
- WebEngage Startup Program
- Impact story
- Startup Program: Customer Spotlight
- Company Updates
- Customer Retention
- Omnichannel Marketing
- Marketing Automation
- Cross-Channel Engagement
- Product Updates
- Uncategorized
- Mobile Marketing
- Campaign Personalization
- User Segmentation
- Insights & Analytics
- Conversion Rate Optimization
- Customer Relationship Management (CRM)
- Company Announcements
- Customer Onboarding
- Customer Spotlight
- User Engagement
- Growth Marketing
- Retention Marketing
- Niche Marketing
- Customer Journey
- Customer Experience
- Moment Marketing
- Customer Relationship Management
- Feature Article
- Thought Leadership
- Press Release
- Customer Centricity
- Industry Specific
23 Mins Read
2,972 views, a complete guide to web analytics | with real-life examples.
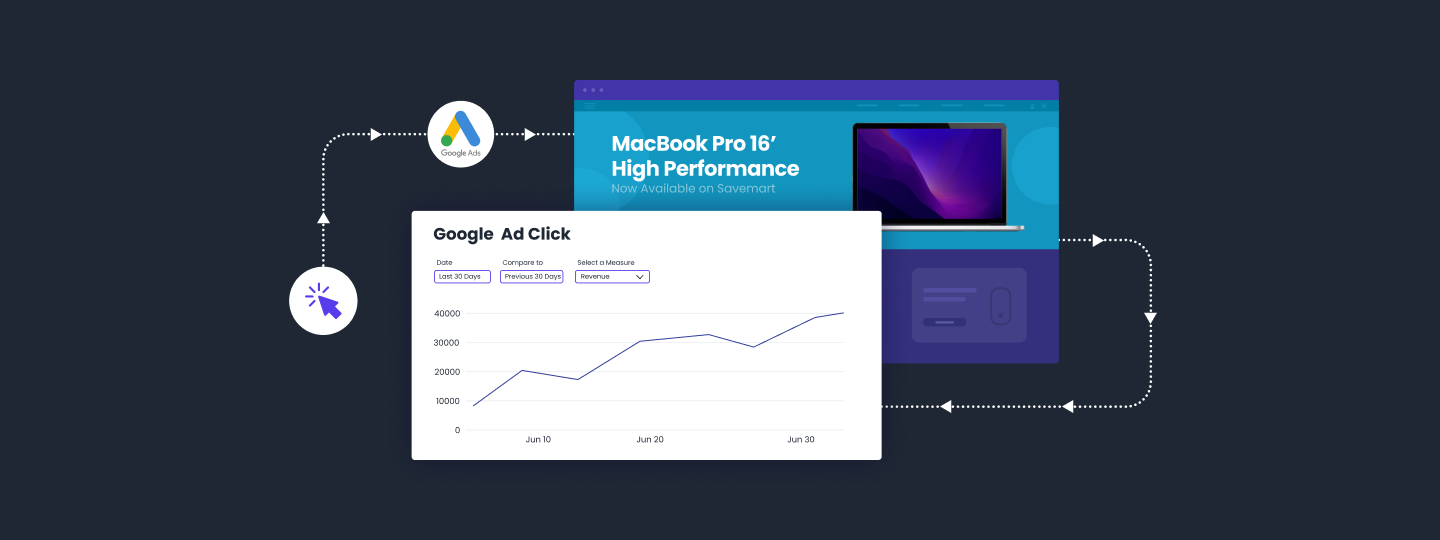
Introduction
If you’re looking to start or expand your online business, understanding web analytics can be a valuable tool.
You can learn a lot about your website’s visitors from web analytics, including how they spend time on your website and what their preferences are. By tracking metrics like page visits, unique visitors, bounce rate, and conversion rate, you can optimize your website design, content strategy, and marketing efforts.
In this guide, we’ll explore the key metrics and tools of web analytics and show you how to use them effectively. We’ll also explore real-world examples of businesses that used web analytics to solve business problems and drive growth.
Whether you’re a newbie business owner or looking to scale up your online business, this guide addresses all you need to know about web analytics. Let’s dive in.
What are web analytics, and why do they matter?
Web analytics involve collecting, examining, analyzing, and displaying information on website visitors and their behavior. This helps website owners understand how users interact with their website and what could be tweaked to improve user experience.
Let’s suppose you own a website that sells scented candles. By using web analytics, you can learn about things like:
- The number of visitors on your website
- The locations they’re coming from (country, state, or city)
- The pages they keep returning to
- The time they spend on each page
- The way they engage with your pages (for example, whether they put something in their shopping cart or leave your site when they face an image-heavy page that’s taking longer to load)
- Whether or not they end up making a purchase
This information could help you in many ways. For example:
- If a slow-loading page turns buyers away, you could redesign it to improve site speed.
- Creating a festival-specific collection showcasing candles related to the festival.
- Displaying pop-ups or notifications with offers on festival-themed scented candles based on your visitors’ behavior and interests. Suppose many people are leaving without buying anything right at the checkout stage. In that case, you might need to streamline the checkout process or add more payment options to encourage buyers.
Web analytics are crucial processes that help online business owners understand user behaviors on their websites. With the help of this information, website owners can improve their site designs, offer a better user experience, and grow their businesses.
Mastering the Web Analytics Process
Navigating web analytics can feel overwhelming, but let’s break it down into simple, manageable steps. 1. Define Your Goals Know what you want to achieve:
- More traffic?
- Higher conversion rates?
- Better engagement?
Clear goals help you focus on the right metrics. 2. Identify Key Metrics Pinpoint KPIs that align with your goals:
- Traffic: Page views, sessions, unique visitors.
- Engagement: Bounce rates, session duration, pages per session.
- Conversions: Conversion rates, goal completions, sales figures.
3. Implement Tracking Tools Set up tools like Google Analytics:
- Account setup: Follow the setup wizard.
- Install tracking code: Add to your website’s HTML to collect data.
4. Collect and Monitor Data Gather and observe data:
- Initial collection: Let your tool gather data for a baseline.
- Regular monitoring: Check analytics regularly for trends or issues. 5. Analyze Data Dive into the numbers: Identify trends: Look for patterns. Compare metrics: See how different metrics relate. 6. Generate Insights Turn data into actionable insights:
- User behavior: Why are users leaving?
- Content performance: What’s working well?
- 7. Take Action Implement changes based on insights:
- Optimize pages: Improve load times, and enhance UX.
- Adjust strategies: Focus on what’s working.
- 8. Review and Repeat Make it a continuous process:
- Monthly reviews: Regularly go over your data.
- Continuous improvement: Refine strategies based on new data.
Important metrics of web analytics
First, let’s understand some key metrics that web analytics help measure.
Page visits (sessions) and page views
Page visits track the traffic to a website within a given time frame. Page visits, also known as sessions, measure how many times a user visited your website, including all pageviews and interactions within a specific period. A session starts when visitors enter your website and ends when they leave or become inactive after a specified time. A high number of page visits from disinterested buyers might not be helpful. In contrast, fewer visits from high-intent buyers can indicate the page’s effectiveness in driving conversions.
Page views are the number of views a webpage gets (or the number of times it loads or reloads) over a given period.
Let’s see how these two are different.
Imagine a visitor coming to a website and viewing five different pages during their visit. This would count as one page visit (or session) but five page views.
Also, page visits occur when visitors land on a webpage from an external source (like a search engine). But page views occur when a single user loads or reloads a webpage, regardless of whether they came from an external source or were already on your website.
Unique visitors
Unique visitors are those who have visited a website during a given time, regardless of how many times they’ve visited. Page views track the total number of views which might include repeat visits by the same person. Unique visitors metric shows the actual number of visitors to the website.
Let’s say there is a website called “Something.com,” and we want to analyze its visitor data.
During the month of April 2023, Something.com had 10,000 unique visitors and 30,000 page views.
This means that there were 10,000 unique individuals who visited Something.com at least once during April 2023. It doesn’t matter if they visited the website multiple times, they are counted as a unique visitor only once.
On the other hand, the total number of page views is 30,000. This includes all the visits to the website, whether they are by unique visitors or repeat visits by the same person. So, if someone visited Something.com eleven times during April 2023, it would contribute eleven page views to the total count.
A session measures a user’s time on a website, starting when they first land on it and ending when they leave or become inactive for a specified period (usually 30 minutes). Users may view multiple pages during a session, take various actions, or engage with different website elements. The number of sessions can be a valuable metric for understanding how visitors interact with a website over time.
Bounce rate
Bounce rate gives the percentage of visitors who leave after viewing only one webpage and taking no further action.
Time on page
Time on page indicates how long a user spends on a specific website before moving to another page or leaving the site. You can measure it by deducting the time when the user first accessed the page from when they navigated to another page or closed the website.
Click-through rate (CTR)
CTR calculates the percentage of users who click on a call-to-action (CTA) link compared to the total number who view a page, email, or advertisement. This tells you how engaging or effective your marketing campaign is.
Conversion rate
Conversion rate calculated the percentage of users who take a specific action or complete a desired goal, such as purchasing something, downloading an eBook, or subscribing to an email newsletter. For example, if a website had 2,000 visitors and 500 made a purchase, the conversion rate would be 25%.
Exit rate denotes the percentage of visitors who leave a website or web page after viewing it. This differs from bounce rate, which considers only those who leave a website after viewing just one page. Exit rate considers all pages a user may have viewed before leaving.
Exit rate is a helpful metric for identifying potential problem areas on a website, such as pages that may be causing users to lose interest or become frustrated.
Remember, pages like the checkout page will naturally have high exit rates. So a high exit rate isn’t always problematic unless it’s happening on a landing page or a product page.
Traffic sources
Traffic sources are the channels through which users find and access your website, such as social media platforms, search engine result pages, and more.
For example, if you’re running an email marketing campaign, the CTA can direct users to a specific page on your website where you want them to take action, such as purchasing a product or downloading a white paper.
If your visitors are referral traffic, they might first land on an article or blog post on your site whose link was shared by another website.
Return on investment (ROI)
ROI is a financial measurement of the profitability of your website, which compares the cost of running your website or marketing campaigns to the revenue generated.
WebEngage’s Customer Data Platform (CDP) helps you track all of these key metrics across different sources, such as websites, mobile apps, and data warehouses, in one place. This way, you can obtain a complete and unified view of your customers. The insights you gain from this help you to build more personalized and targeted campaigns.
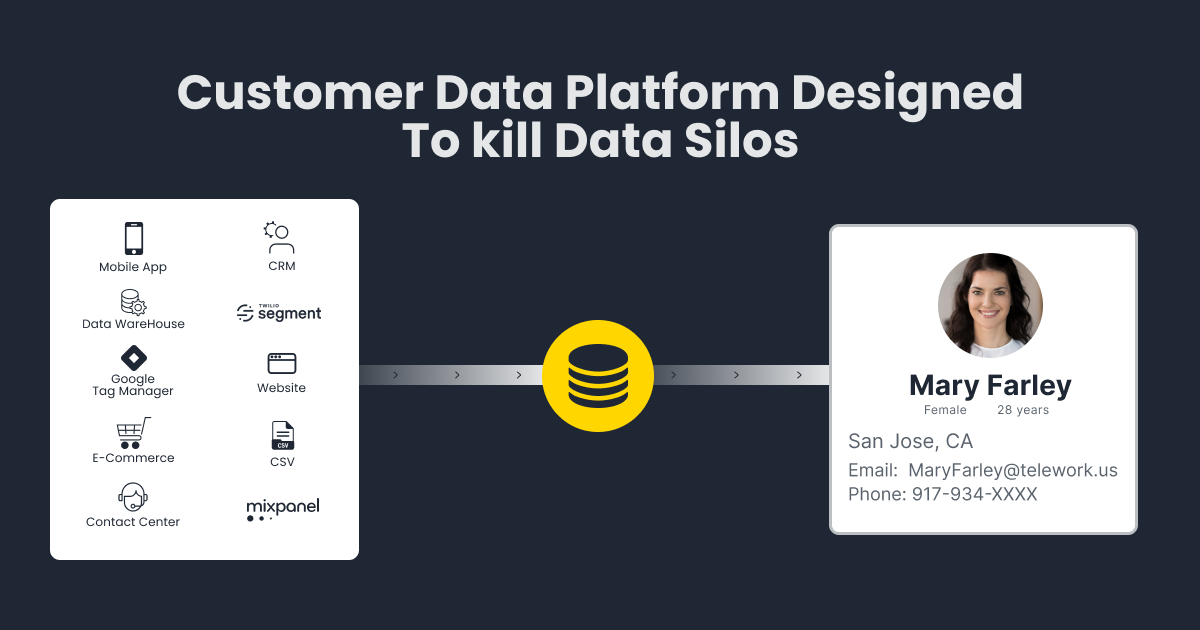
Common Issues with Web Analytics
1. inaccurate data, 2. data overload, 3. misinterpreting data, 4. tracking gaps.
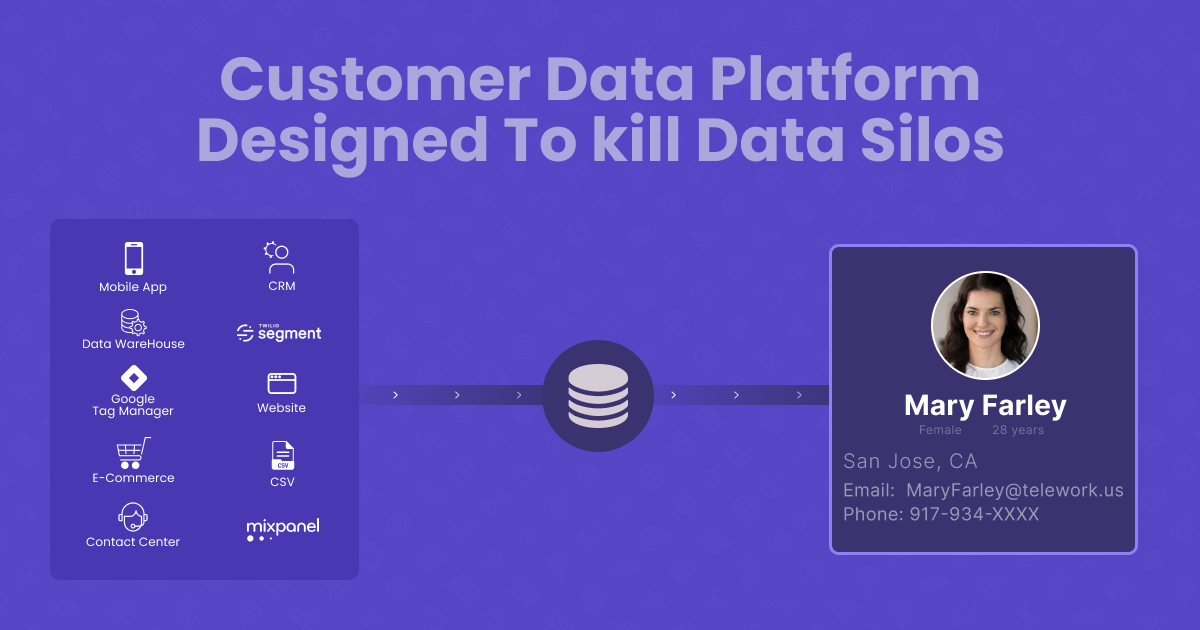
What kind of problems do web analytics help solve?
Here are some ways in which web analytics can boost your business growth. The accompanying impact stories show how they can be put into practice.
User behavior tracking
Web analytics help track your visitors and provide insights about their behavior. Suppose a user visits a website and clicks on a product page. Web analytics tools monitor how users interact with the product page, including metrics like the length of time they spend on the page, whether they added the product to their shopping cart, and if they ultimately made a purchase.
The software also tracks the user’s behavior on other pages, such as the homepage and checkout page.
The user’s behavior data is aggregated and analyzed to understand patterns like which products are popular, what pages have high bounce rates, and where users tend to drop off in the checkout process. This information can help you tailor your website for a better user experience and higher conversion rates. According to Forrester , companies that make data-driven website changes are three times more likely to improve customer experience (CX) than companies that don’t consider data analytics.
Let’s see this in action.
TravelTriangle , India’s biggest OTA marketplace, wanted to investigate why people visited their web pages or mobile app but left without booking a trip. With the help of the Lead Scoring model designed by WebEngage, TravelTriangle could assign scores to its visitors. A high lead score implied high intent users. Once the user segments were defined, TravelTriangle targeted the high-intent users via hyper-personalized communication and cross-channel engagements. As a result, the drop-offs on the travel destination landing pages were reduced by 10%, and the company’s remarketing cost declined by 30%.
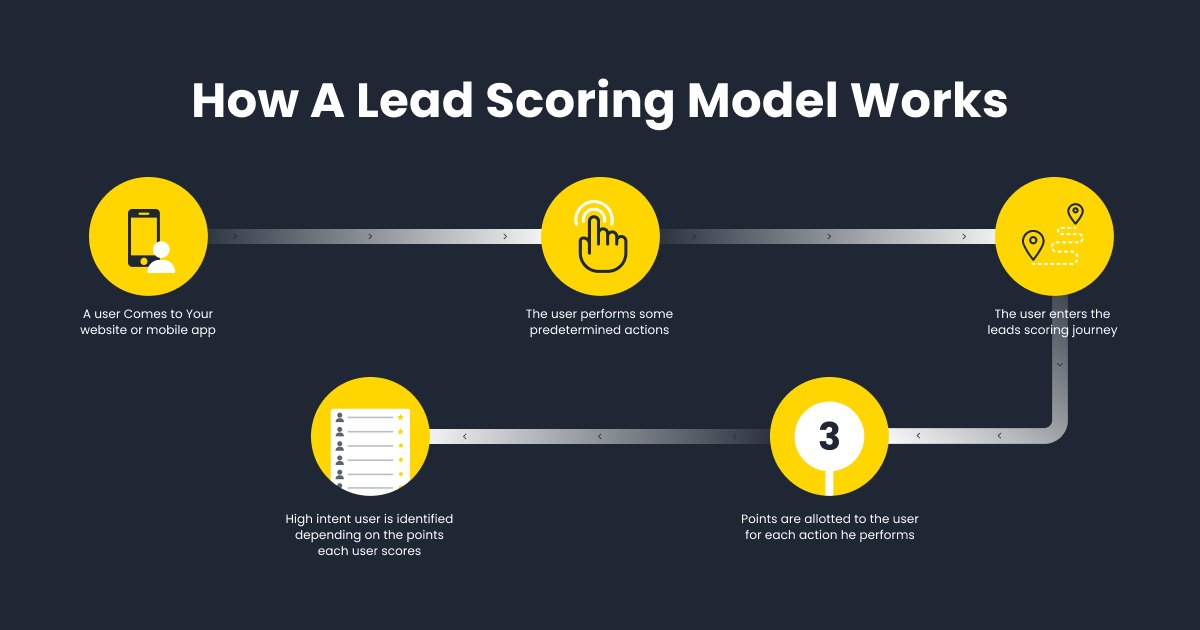
Website design and content strategy
You can use web analytics to identify areas for improvement in your website’s design and content. Using these insights, you can create a more user-friendly website that offers content tailored to your target audience’s preferences. This will help boost your engagement and conversions.
For example, a business might use web analytics to identify the traffic sources to its website and the most popular pages among its visitors. The company could optimize its website design and content strategy with this information to improve engagement and conversion rates.
Happilo , an Indian gourmet health food brand, faced trouble retaining its customers due to a lack of personalized and relevant website content.
Happilo deployed WebEngage’s in-line personalization tool, which helps create a customized website experience for each user based on their behavior and attributes. You don’t need any coding experience to use the in-line personalization tool.
As a result of implementing in-line personalization, Happilo could achieve a 15% growth in conversion rates through repeat purchases and a staggering 286% uplift in conversion by targeting cart abandoners.
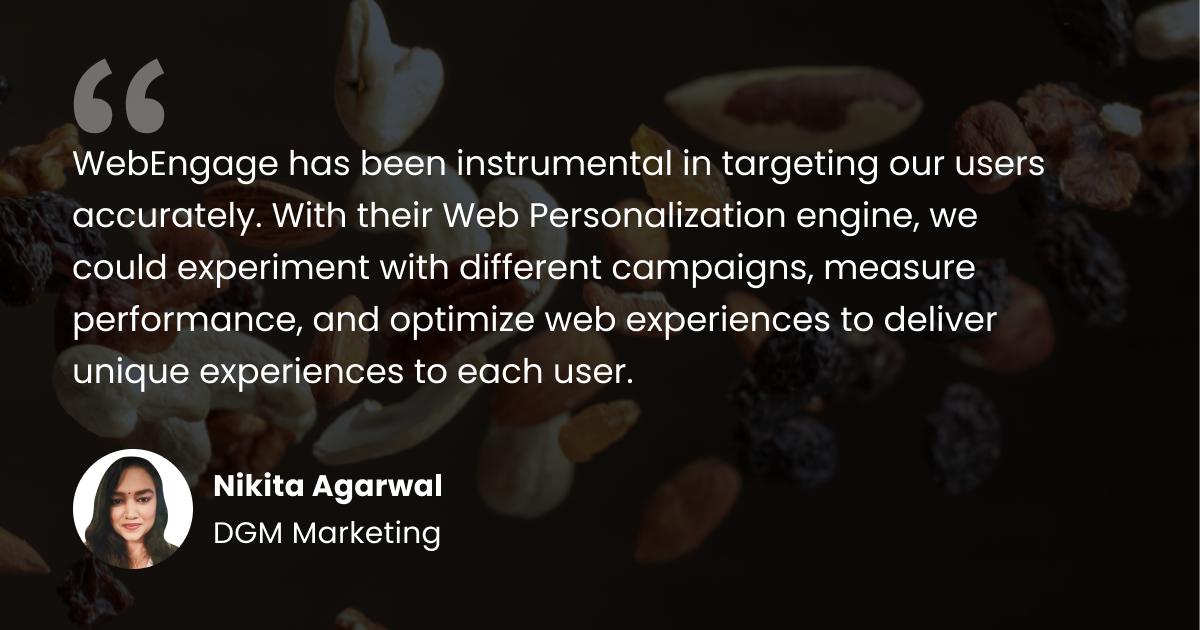
Marketing efforts
Businesses can use web analytics to track the performance of their digital marketing and advertising campaigns and choose the best channels for reaching their target audience. Let’s see how this is done.
Scripbox , India’s leading digital wealth manager, needed help optimizing its marketing efforts to enhance acquisitions and retention. With WebEngage’s Journey Designer and analytics platform, the company could segment its customer base based on domestic and NRI residents. Then they targeted the segments with more personalized communication using WebEngage’s dashboard features like ‘ Send Intelligently ‘ and ‘ A/B testing .’ WebEngage’s web analytics tools also empowered Scripbox to measure and rank its marketing campaigns’ efforts and optimize the most effective ones.
As a result, the company witnessed a 3X growth in user engagement , a 25-30% growth in email open rates, and frequency capping in marketing campaigns leading to a 25% decrease in unsubscribe rate.
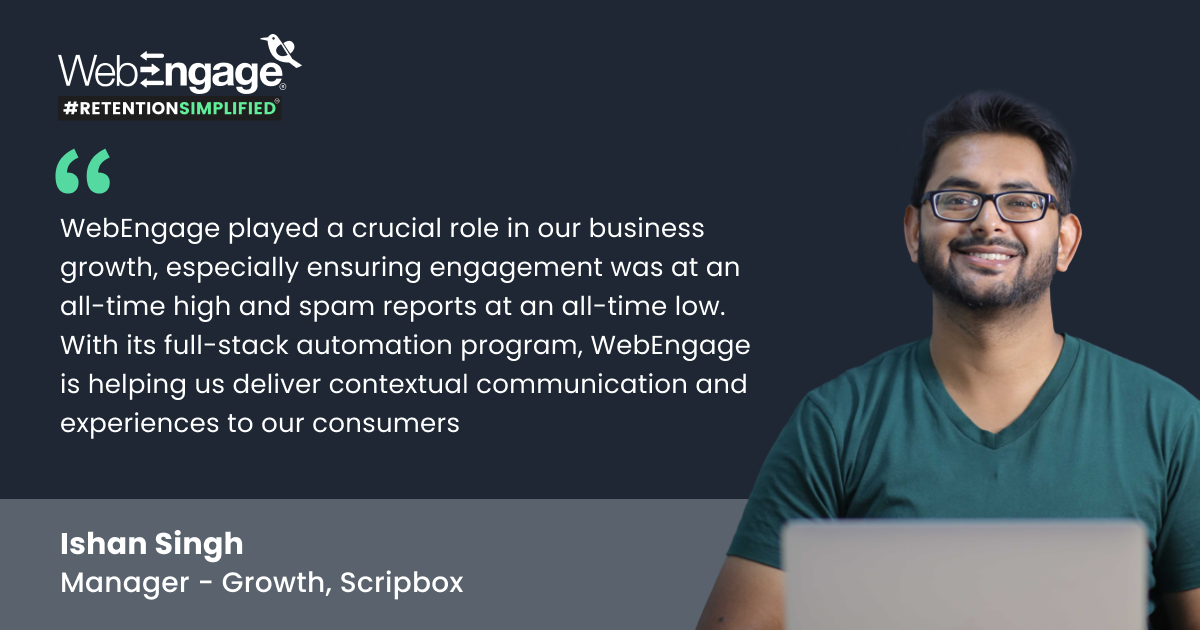
How to leverage Web Analytics to create high-impact strategies that drive business growth?
Define goals and objectives.
To use web analytics insights effectively, you must first define the goals you’re trying to achieve. For that, you first need to determine your overall business objectives. Are you trying to boost sales or improve engagement? Identifying your goals will help you focus your efforts and ensure that you’re tracking the right metrics.
Make the goals as precise as possible. So instead of setting a goal like increasing web traffic, set an objective like ‘increasing website traffic by 20% in the next four months.’ All your goals should be specific, measurable, achievable, relevant, and time-bound (SMART).
Track the right metrics
Once you’ve identified your objectives, it’s time to determine the Key Performance Indicators (KPIs) that help measure the progress toward your goals. Let’s assume you want to boost sales. In that case, your KPIs could be conversion rates, cart abandonment rates, and revenue per user.
Choose the right tools
Select the web analytics tools that will help you collect the data you need to measure and analyze your chosen metrics most efficiently. Analytics tools can help you track user behavior on your website, including which pages users visit, their time on a page, and their actions. Google Analytics is a popular tool with many features, but other options are also available.
WebEngage has some advanced and sophisticated analytics tools like Funnels , Paths , and Live Analytics that help you in cases like:
- Visualizing how customers are engaging with your brand
- Tailoring your sales funnel to suit customer behavior
- Getting real-time insights on your marketing campaigns
- Encouraging customers to return to your eCommerce site
- Understanding the reasons for customer drop-offs
Analyze the data
Once you’ve installed the right analytics tools and tracked your chosen metrics, you must analyze the data. Look for patterns, trends, and anomalies. Understand which areas of your website are engaging users (or causing them to leave). This knowledge can inform your content and design decisions. For example, Clovia , a leading full-stack lingerie brand in India, used WebEngage’s Funnels to analyze user behaviors and customer drop-offs on its websites and apps. A checkout funnel was created for high-intent users to observe their behavior patterns at different times.
Identify traffic sources
Understanding how users find your website is crucial for building effective marketing strategies. Analytics tools can reveal which channels (e.g., search engines, social media, email) drive traffic and which generate the most conversions.
User segmentation
Segmenting users based on demographics, behavior, or other factors can help you identify patterns and trends that might not be immediately visible when looking at aggregate data. This information can inform your targeting strategies and help you create more effective messaging and campaigns.
Use A/B testing
Analytics tools can help you test different variations of your website and marketing campaigns to identify the highest-performing versions. You can tweak your website and campaigns accordingly.
Make data-driven decisions
Based on your analysis, decide how to improve the performance of your website. Adjust your website design, content, or marketing strategies to achieve your goals.
In continuation to our last example, Clovia analyzed the data it collected. Using WebEngage’s Journey Designer, the company created a personalized multi-channel journey that encouraged customers to complete the checkout process. Using web analytics tools, Clovia determined the best time to reach out to cart abandoners. As a result, it experienced an impressive 85% growth in its overall revenue .
Continuously monitor and refine
Your job doesn’t end when you reach your goals. Keeping track of your website metrics and making tweaks to your strategy according to the insights received is an ongoing process. Over time, your business metrics will change. But one thing will remain true: you will always need to track your website’s performance and make necessary adjustments with the help of web analytics tools.
Something to keep in mind
There are certain instances, like dark social, where web analytics might not give you the most accurate insights.
Dark social refers to social sharing and online referrals that occur through private messaging platforms, email, or other non-public channels, making it difficult for marketers and analysts to track and measure. In other words, it is the sharing of content or links that take place outside of public social networks without identifying the source.
Imagine sharing a link with your friend via private messaging through WhatsApp or Facebook Messenger. In these cases, the referrer information is often lost. Then when your friend clicks on a shared link and visits a website, the source of the traffic appears as “direct” instead of being attributed to the specific sharing channel.
Web analytics tools can provide some insights and help shed light on dark social to a certain extent, but they are not designed to fully capture and track it.
To gain a more comprehensive understanding of dark social, you might need to employ alternative methods, such as surveys, user interviews, or social listening tools, to gather data and insights from users themselves about their sharing behaviors.
Web Analytics Best Practices
Final thoughts.
If you dream of growing your online business, there’s no doubt that web analytics are crucial. Without understanding your website traffic and user behavior, making informed decisions about optimizing your website design and marketing strategies is almost impossible. But don’t worry. You need not do everything by yourself.
WebEngage is here to help you harness the power of data-driven decision-making. Our advanced analytics features are designed to help you gain deep insights so you can make informed decisions that drive growth and boost conversions. With tools like funnels, paths, cohorts, and live analytics, you’ll have all the information you need to customize your website and marketing campaigns for maximum impact. Need more proof? Check out our Impact Stories . Our analytics’ capabilities have helped businesses like yours achieve remarkable results. We’re confident we can help you. Ready to take the leap? Head to our website to book a demo today .
How important is web analytics?
How do you analyze web analytics.
- Set Clear Goals: Define what you want to achieve (e.g., more traffic, higher conversion rates).
- Identify Key Metrics: Focus on metrics that align with your goals, such as page views, bounce rates, and conversion rates.
- Use Analytics Tools: Utilize tools like Google Analytics, SEMrush, and Hotjar to collect and analyze data.
- Monitor Data Regularly: Keep an eye on your data to spot trends and anomalies.
- Generate Insights: Interpret the data to understand user behavior and identify areas for improvement.
What is an example of web analytics?
What are the limitations of web analytics.
- 1. Incomplete Data: Not all user interactions are tracked, leading to gaps in data.
- 2. Data Quality Issues: Incorrect tracking code placement or bot traffic can result in inaccurate data.
- 3. Interpretation Challenges: Data can be misleading without proper context, leading to incorrect conclusions.
- 4. Privacy Concerns: Collecting user data must comply with privacy regulations like GDPR and CCPA, which can limit the amount of data you can gather.
- 5. Technical Skills Required: Effective analysis often requires a good understanding of the tools and data interpretation skills.
- What are the risks of web analytics? The risks of web analytics include:
- 1. Privacy Violations: Mismanaging user data can lead to privacy breaches and legal issues, especially with regulations like GDPR and CCPA.
- 2. Data Misinterpretation: Incorrectly interpreting data can lead to poor business decisions.
- 3. Over-Reliance on Data: Focusing too much on data can stifle creativity and intuition in decision-making processes.
- 4. Security Risks: Storing and managing large amounts of user data can make your systems a target for cyberattacks.
- Compliance Risks: Failing to comply with data protection laws can result in hefty fines and damage to your reputation.
- Created: 26th May, 2023
- Last Updated: 2nd September, 2024
- web analytics , Retention Simplified
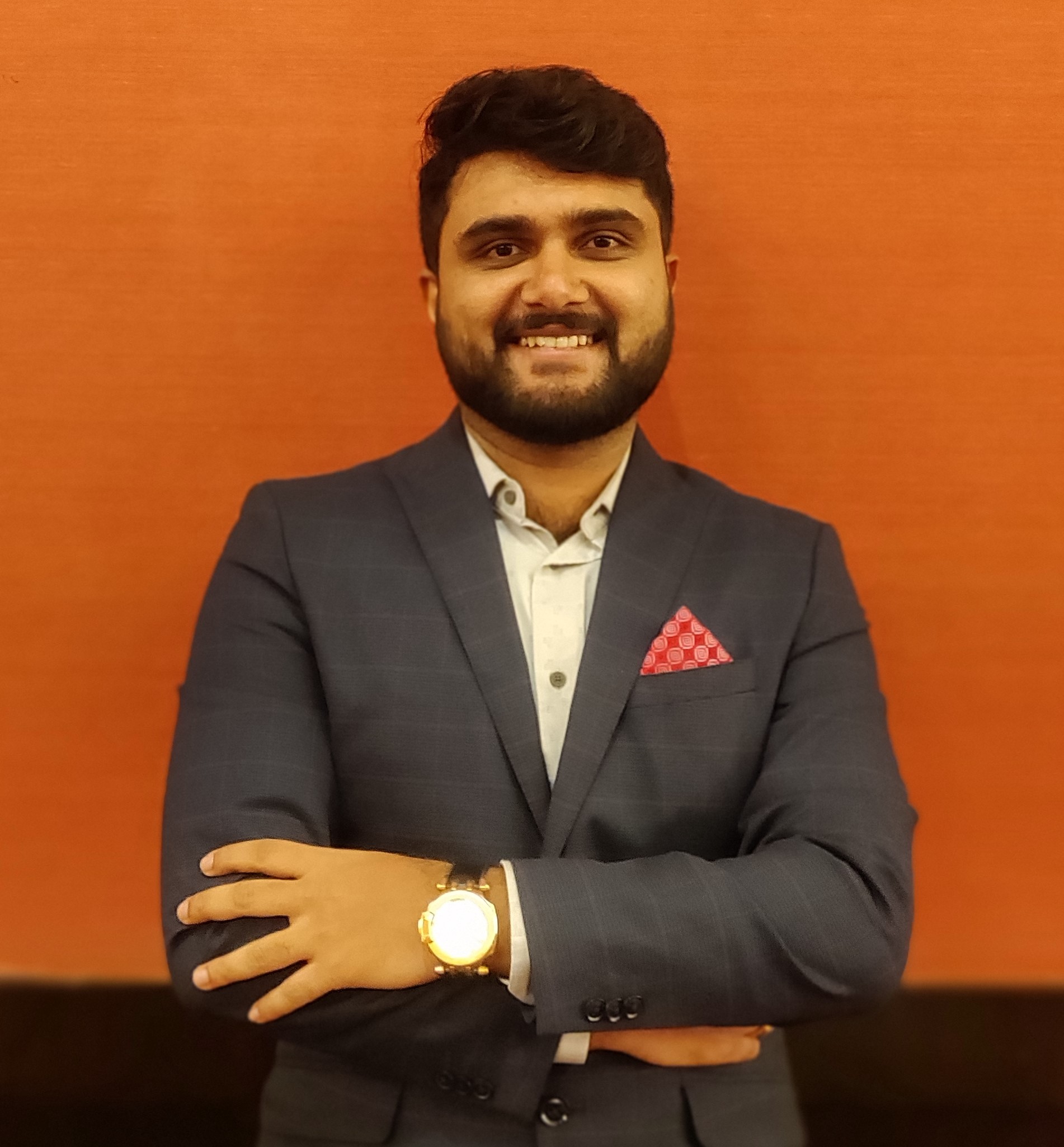
9 Mins Read
Reduce defaulters with automated omnichannel collection reminders.
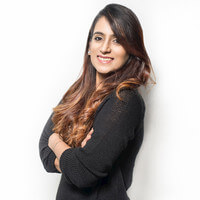
Mastering Moment Marketing: 7 Strategies for D2C Success
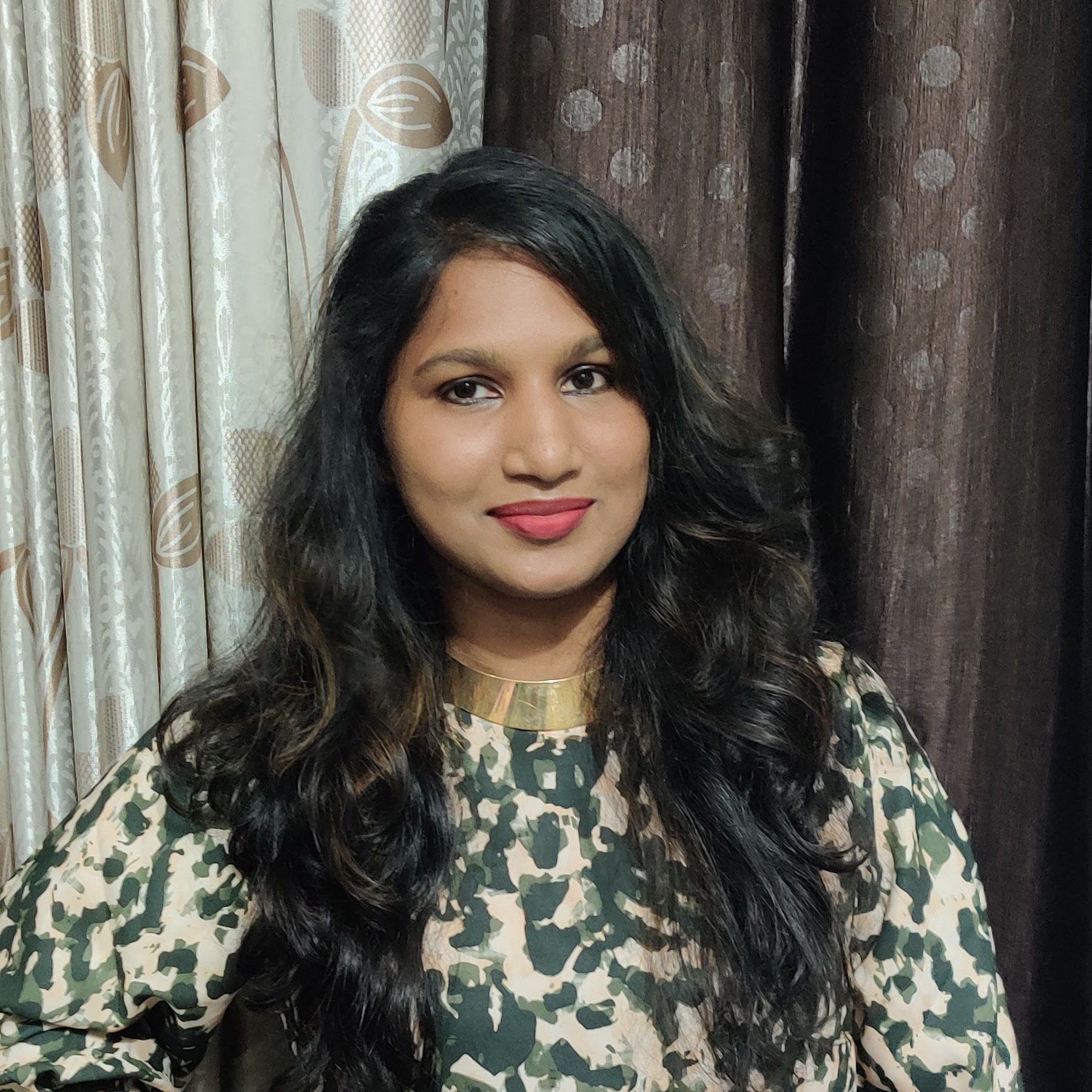
10 Campaign Mistakes You’re Making in a Hospitality Business: Marketing 101
Subscribe to our FREE weekly newsletter to be up-to-date with the latest trends and developments.
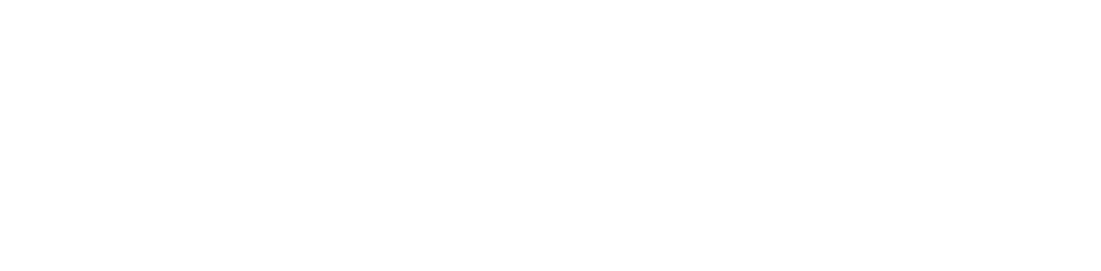
“I like many things about WebEngage, but what stands out is their agility and being customer centric .”

Nitin Sethi,
SVP, CDO – Consumer Businesses
800+ Global brands trust WebEngage

What to expect from the demo call?
- Deep dive into industry specific use-cases
- Learn how leading brands are using WebEngage to solve for user retention
- Identify the gaps in your current growth strategy, get your queries answered, etc.
Talk to our team
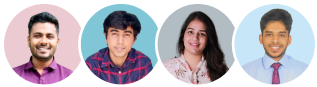
Supercharge Your Startup's Growth
Book a free consultation.
The Program helped us scale our business faster and bring down acquisition costs with time.

Ankit Agarwal
Founder, PHOOL
Become a Partner
Grab your copy.

10 Product Analytics Case Studies
Successful companies understand the value of utilizing product analytics to make informed decisions, optimize user experiences, and drive growth . From entertainment giants like Netflix to e-commerce platforms like Shopify , businesses across industries leverage product analytics to gain a competitive edge. In this blog post, we’ll explore 10 inspiring case studies showcasing the power of product analytics.
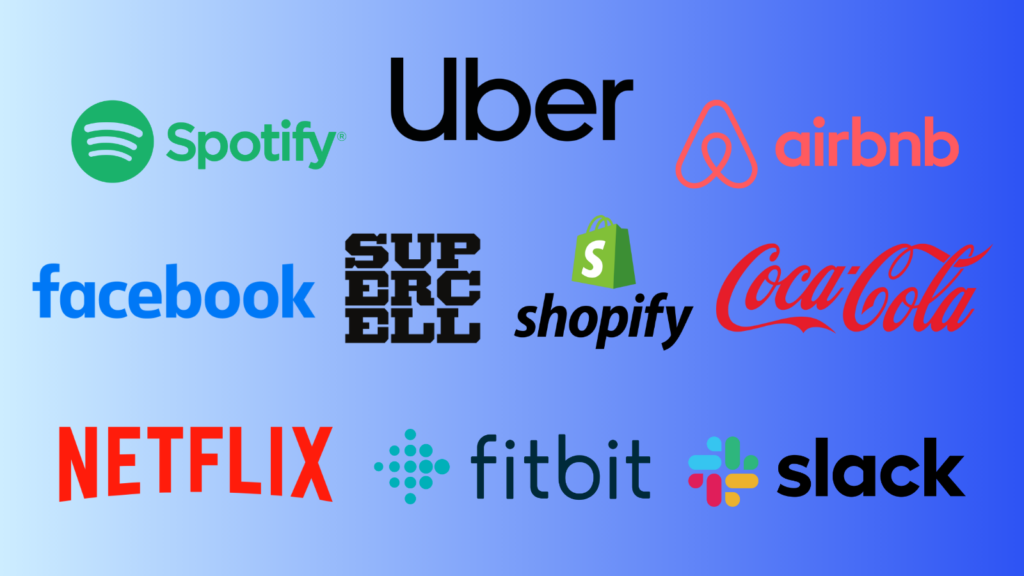
Real-world examples of how data-driven insights transformed businesses
1. Netflix ‘s Content Recommendation System: Personalized Engagement Delve into the realm of data-driven innovation as you uncover the inner workings of Netflix ‘s cutting-edge recommendation algorithm. Through meticulous analysis of user data, this algorithm breathes life into personalized entertainment, decoding individual preferences, viewing history, and interactions to craft a seamless streaming experience, resulting in a profound boost in user engagement and unwavering retention rates. This fusion of data and innovation is a testament to the power of harnessing user insights to revolutionize the entertainment industry, showcasing unparalleled content curation. Read the case here >>
2. Airbnb ‘s Dynamic Pricing Strategy : Revenue Optimization Experience the revolution of dynamic pricing, where data-driven insights and innovative hospitality transform travel. Airbnb uses real-time data to shape pricing, aligning with demand, local events , and seasons. This ensures hosts maximize earnings while keeping guests satisfied. Travelers find prices tailored to their preferences and budget, building transparency and trust. This fresh pricing approach balances host profitability and guest affordability, redefining hospitality through data-guided strategies. Read the case here >>
3. Spotify ‘s Music Personalization: Tailored Playlists Explore the world of personalized music through Spotify’s ingenious algorithm. By analyzing users’ listening behavior, Spotify crafts personalized playlists that uniquely resonate. These curated musical journeys transcend genres, leading to delightful discoveries and cherished rediscoveries. Through this innovative blend of data analysis and musical intuition, Spotify creates longer listening sessions and heightened user satisfaction, showcasing the transformative power of finely tuned data in crafting auditory experiences. Read the case here >>
4. Shopify ‘s Conversion Rate Optimization: Enhanced E-commerce Sales Dive into e-commerce optimization with Shopify’s advanced analytics. Every click, scroll, and interaction in this digital marketplace leaves insights. Shopify ‘s analytics tools uncover valuable data, enabling businesses to decode customer behavior, spot bottlenecks, and enhance the sales funnel . Armed with these insights, businesses adeptly tackle conversion rate challenges , refining user experiences for persuasion. As they fine-tune websites, adjusting the layout, navigation, product presentation, and checkout, a tangible improvement in sales and revenue emerges. This narrative showcases how data-driven choices reshape e-commerce, orchestrating growth one insight at a time. Read the case here >>
5. Uber ‘s Surge Pricing Algorithm: Efficient Demand Management Explore the world of dynamic pricing through Uber’s lens. Uber’s data-driven surge pricing in urban transportation is an optimization exemplar. The algorithm identifies demand spikes during peak hours, special events, or adverse weather. It then adjusts fares, balancing rider expectations and driver incentives to align supply with demand. This equilibrium ensures reliable rides for riders and encourages drivers into high-demand areas. This data symphony showcases efficiency, aligning rider and driver interests and boosting Uber ‘s peak-time revenue. Read the case here >>
6. Coca-Cola ‘s Freestyle Machines: Flavor Innovation Experience the realm of beverage innovation where Coca-Cola’s data-driven insights create a symphony of flavors and precise inventory. The Freestyle machines showcase how data fuels innovation and efficiency. By analyzing customer preferences, consumption patterns, and flavor combinations, Coca-Cola crafts new blends for evolving tastes. These inventive mixes tantalize taste buds and highlight data-creativity synergy. Beyond flavor, data guides inventory management. Freestyle machines’ real-time data grasp popular beverages by location, optimizing inventory to match demand. This fusion of data and beverage artistry quenches thirst and demonstrates how data sparks innovation, improves offerings, and refines operational excellence. Read the case here >>

7. Fitbit ‘s User Engagement Enhancement: Health Tech Insights Enter the health and fitness tech world, where Fitbit’s mastery of product analytics shines as a guide for evolving insights. In the dynamic wearable landscape, understanding user preferences shapes resonating experiences. With various sensors and data collection tools , Fitbit deciphers patterns like steps, heart rate, sleep, and workouts. This data portrays users’ fitness journeys, refining features based on goals and needs. By empowering users, Fitbit creates an engaged ecosystem. Data insights drive product innovation, enhancing the journey towards better health. Read the case here >>
8. Facebook ‘s News Feed Customization: Tailored Engagement Enter the realm of social media dynamics, where Facebook’s data mastery shines in tailoring content consumption. The News Feed is a virtual hub for sharing, interacting, and exploring in this digital arena. Using diverse data streams, from interactions to browsing habits, Facebook employs algorithms to curate personalized content symphonies. This approach lets users discover posts, stories, and updates that personally resonate, fostering community connections beyond demographics. As users dive into this sea of tailored content, engagement thrives, cementing the platform in their daily lives. This showcases the convergence of data and interaction, with Facebook’s insights orchestrating seamless digital journeys. Read the case here >>
9. Slack’ s Collaboration Revolution: Data-Driven Features Enter the world of workplace collaboration, where Slack’s data-driven innovation shines. Effective communication and collaboration are pivotal for modern productivity. Slack pioneers this realm, utilizing product analytics to understand user interactions, preferences, and challenges. This treasure trove guides Slack’s evolution, enabling seamless feature integration to meet users’ needs. With real-time data guiding them, Slack enhances messaging, integrates third-party tools, and refines the user experience . As teams work on the platform, every action shapes refined user journeys. The outcome is a harmonious work rhythm, embodying the idea that data-guided innovation creates user-centered excellence. Read the case here >>
10. Supercell ‘s Monetization Mastery: Community and Revenue Growth Step into the dynamic mobile gaming world, where Supercell shines as a data-driven gaming leader. In mobile gaming, engagement and monetization go hand in hand, and Supercell excels by using product analytics to create experiences that deeply resonate with players. Every interaction, from swipes to cleared levels, generates data that Supercell transforms into valuable insights. This understanding of player behavior is the foundation of their community engagement strategy. Supercell curates content updates aligned with player preferences, sparking excitement and leading to irresistible in-game purchases. This harmonious blend of data insights and game design propels community engagement while ensuring player satisfaction generates revenue. In the dynamic realm of mobile gaming, Supercell ‘s expertise in product analytics illustrates how carefully orchestrated data shapes digital experiences, fosters enduring player connections, and cultivates thriving gaming ecosystems. Read the case here >>
These case studies showcase the transformative impact of product analytics across various sectors. By harnessing the power of data, companies can better understand their customers, optimize processes, and ultimately achieve their business goals. Each case study link takes you to an in-depth analysis of how these companies implemented product analytics to drive success.
As technology evolves and data becomes more accessible, these examples provide a glimpse into the vast potential of product analytics. Stay tuned to the ever-evolving landscape of data-driven insights that continue to shape how businesses operate and deliver value to their customers.
Related Posts
- Video: Product Analytics Masterclass
- How Prescriptive Analytics is Transforming Product Analytics
- Apple AirPods: A Case Study in Product Analytics
Post navigation
Previous post.

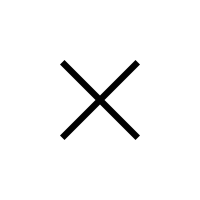
- Case Studies
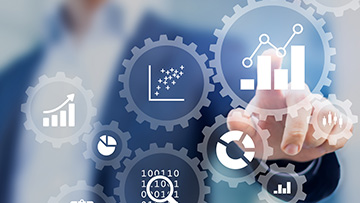
- June 6, 2019
High Volume ClickStream Analytics to Monetize Search Page Ads
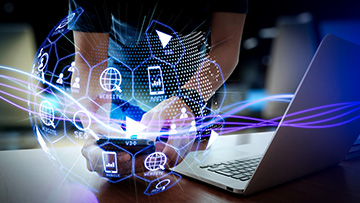
- February 23, 2021
Digital Roadmap for Website-led Customer Acquisition
(re)think data think latentview, technical expertise, domain expertise, advisory services.
- Financial Services
- Industrials
- Infographics
Whitepapers
- Leadership Team
- Corporate Social Responsibility
- Advisory Council
- Alumni Portal
Privacy Policy
- Partnerships
- Data Engineering
- Data Science
- Data Visualization
- Customer Analytics
- Marketing Analytics
- Supply Chain Analytics
- HR Analytics
- Financial Analytics
- Risk & Fraud Analytics
- Analytics Roadmap
- GenAI Readiness
- Global Innovation Hub
- ConnectedView
- InsightLens
- MigrateMate
- OneCustomerView
- Smart Innovation
- Investor Relations
Are you an agency specialized in UX, digital marketing, or growth? Join our Partner Program
Learn / Guides / Web analytics guide
Back to guides
6 steps to creating a customer-centric web analytics strategy
Analyzing website performance data can feel like you’re floating aimlessly in a sea of numbers, graphs, and percentages, without being able to anchor them to real-life scenarios. For example, you can track the abandonment rate of a particular page, but that doesn’t tell you why customers are exiting in the first place.
Web analytics data gives you the information you need to analyze what’s happening on your site—but understanding and utilizing those insights is another thing entirely. And without a web analytics strategy, you’ll be doing a lot of guesswork.
Last updated
Reading time.
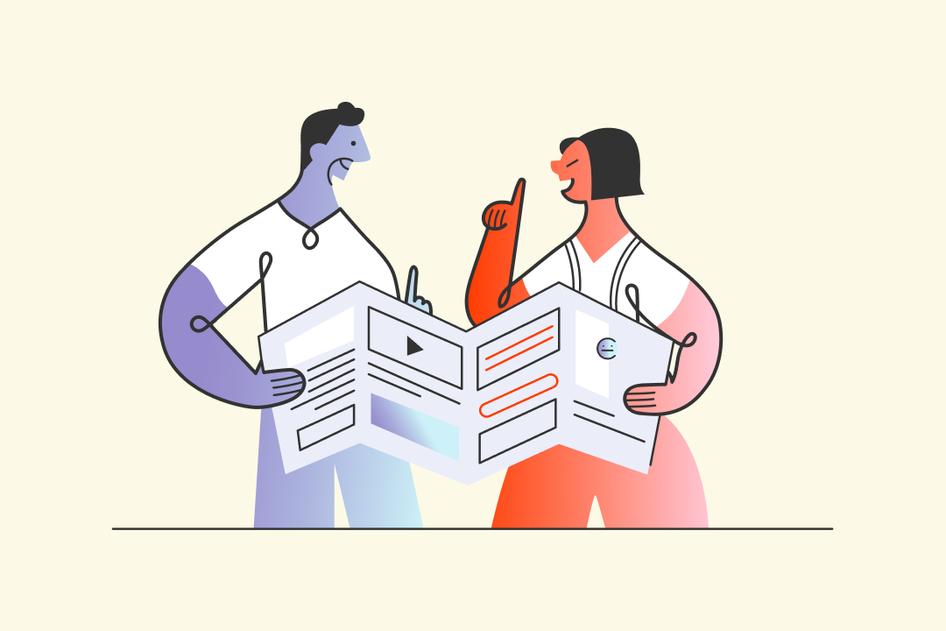
A well-crafted analytics strategy ensures you make website optimizations based on objective data that reflects your customers’ needs and provides the best user experience (UX) possible , while keeping your business goals and priorities on track.
This step-by-step guide helps you get down to the basics of creating an impactful, customer-centric web analytics strategy guided by data-driven decisions.
Gain a deeper understanding of your customers
Hotjar tells the customer's story—so you can go beyond the data with your web analytics strategy.
What is a web analytics strategy?
A web analytics strategy is the collecting, reporting, and analysis of website data. It’s essential for gaining insights into what drives your business forward and developing an understanding of the user experience—and how you can improve it.
In short, a web analytics strategy is a playbook for achieving your goals and meeting your business objectives.
Why do you need a web analytics strategy?
It’s simple: if you have a website for your business, you need a web analytics strategy.
Having a web analytics strategy in place before you dive into your data and customer insights ensures you make data-driven business decisions that keep your customer at the forefront of everything you do and will:
How to create an impactful web analytics strategy in 6 steps
We’ve brought it back to the basics and created this six-step guide to help you develop a strategy that puts your customer at the forefront, so you can keep making the right decisions for the right reasons.
Let’s dive in!
1. Collect website data and customer insights
Web analytics tools give you insights into your website and customer behavior so you can assess metrics like traffic, user interaction with your site, and visitor acquisition.
We’re spoiled for choice when it comes to web analytics tools : there are plenty of options with varying costs, collaborative capabilities, and user interfaces. Select the tools that collect information in the way you find most helpful.
For example, using traditional quantitative web analytics tools with qualitative behavior analytics tools—like pairing Hotjar with Google Analytics —results in a winning combination that provides insights from both perspectives, helping you answer the whys behind the whats of web analysis .
When you combine tools like Hotjar Heatmaps, Recordings, and Surveys with your Google Analytics dashboard, you add visual representation to your data and give your customers a voice, which lets you see (from your customers’ perspectives) exactly where you’re going right or wrong.
After reviewing Google Analytics data, installing Hotjar is one of the first steps we take in every client engagement. It’s the window we use to understand the customer journey.
By analyzing both quantitative and qualitative data , you’ll see the hard facts (the numbers, graphs, and percentages we mentioned earlier) and the behavioral context behind them that tells the story of a customer’s journey.
Typically, you’ll want to analyze at least the following metrics to get a clear sense of your user behavior:
Traffic to your site
Acquisition of your website visitors
Bounce rates and abandonment rates
Time spent on-site
Conversions
Keep reading to learn which KPIs we recommend for a customer-centric web analytics strategy .
The opportunity for inspiration is endless, so we’d caution against only looking at so-called ‘vanity metrics’ and remember your reason for implementing your web analytics strategy in the first place.
Celebrating the wins is important, but the point of analyzing data is not just to pat yourself on the back about the sales you’re making and ignore the rest. Look for opportunities and be motivated by the potential of your site to offer an amazing user experience.
Once you’re set up with an analytics platform that works for you, you’re ready to collect insights into your customers’ experience and use them to inform the next steps of your strategy.
2. Define your goals and objectives
Your goals and objectives set the tone for your web analytics strategy.
Think critically about what you want to get out of your website, and your business as a whole—in the short-term, long-term, and everything in between—and create actionable, understandable steps for everyone involved.
Objectives can be thought of as your reason for being: why your site exists, what makes your company unique, and what product or service you’re offering your customer.
Goals get more specific, and it’s here that you need to ask yourself what you’re trying to accomplish on your website. Are you looking to increase sales? Reach a growing audience of happy and loyal customers? Spread the word about your newsletter? All of the above?
The clearer you are at the start, the easier it is to assess your progress later on. Don’t overcomplicate things—it can be as simple and actionable as this:
"Our objective is to build awareness of our brand, and our goal is to increase the number of sign-ups to our weekly newsletter by 50% by the end of the next quarter."
And with that, you have a great foundation on which to base the rest of your strategy.
Pro tip: developing a web analytics strategy isn't just a box-ticking exercise.
Come up with new ways to continually build deeper connections with your customers and understand what drives them—or what turns them away.
Think of your customer as the lighthouse that guides you, and critically assess whether everything you’re doing is being done for the right reasons. For example, your business priority may be to increase sales, but rather than simply optimizing the last step in the checkout process, look to create a more seamless journey and better user experience to get prospects to checkout.
As your goals get more specific, it's important to understand where your strategy fits within the marketing funnel and how different your approach to each stage may need to be.
This brings us to the next step…
3. Understand where you fit within the marketing funnel
Your website goals will differ depending on which stage of the funnel your target customers are in, and your customers will be looking for different things depending on where they are in their journey.
For example, someone at the top of the funnel may not even know what they need yet—they’ve just learned about your business and are now trying to gauge who you are and what you do. This is the time to convey your branding and personality while laying the groundwork for trust.
At the bottom of the funnel, your customer is actively in the market and prepared to purchase; they just need a reason to choose you over a competitor.
Let’s look at how the marketing funnel is defined in simple terms.
Analyzing customers at each level of the funnel helps you apply your web analytics strategy to every stage of their journey. You’ll be able to respond to their specific needs instead of relying on a one-size-fits-all approach.
We recommend continually assessing your customers within the funnel so you can monitor whether you’re offering the best experience possible.
4. Determine your KPIs
Key performance indicators (KPIs) guide your web analytics strategy and provide inspiration on how to take action in specific areas through a deeper understanding of your customers’ needs.
KPIs like conversions, page views, acquisitions, and bounce rates reveal a lot about the health of your site and business, and give you visibility into where your customers are coming from and their behavior and experience on your website.
By mapping your KPIs back to your goals and objectives, you can track your results and measure your progress in a way that makes sense. For example, if one of your goals is to increase the number of customers in Germany, measuring KPIs such as acquisition, conversion rates, and the number of visitors in Germany would be one way to see whether you’re on track to hitting your target.
How to pick KPIs for different stages of the marketing funnel
We’ve already talked a little about the value of funnel analysis to better understand your customers’ motivations.
The same applies to your KPIs: setting KPIs within the context of the funnel will guarantee you’re looking at the right metrics for the right reasons. By defining KPIs representing customers at each stage of their journey, you can home in on essential insights into their on-site behavior.
Let’s look at how your KPIs might differ throughout the funnel:
By understanding the difference in KPIs—and the people they represent—at each level of the funnel, you’ll stay attuned to customer behavior and be better equipped to anticipate optimization opportunities that address exactly what they need.
A note on conversions
We’ve mentioned conversions a few times now—they’re often hailed as one of the most important measures of success for a business. A conversion happens when a website user takes any desired action—like landing on a specific page, clicking a certain link, or making a purchase—and conversions will look different depending on your business and target audience.
Conversion rate optimization , or CRO, is optimizing, experimenting, or making changes to your site to increase conversions.
And while conversions are important, a lot of work needs to come before this stage. It can be easy to get caught up in the numbers and ROI, but to reach the people you’re targeting, it’s essential to keep the user experience at the heart of your optimizations and use this as the driving force behind your strategy.
Something led them to your site—maybe curiosity driven by a marketing campaign, or they were looking for a solution to a problem—and something will either drive them away or convince them to take the next step.
Combining traditional web analytics tools like Google Analytics with behavior analytics platforms like Hotjar provides an extra level of insight into the user experience, so you can stay connected to your customers' needs even while working towards your CRO.
5. Segment your data for reporting
Segmenting the data you’ve gathered from a source like Google Analytics lets you isolate the specific information and metrics you’re most interested in, piece together the puzzle, zoom out, and build a cohesive picture of your customer's journey.
Your segmentation subsets can be as specific as you need them to be. They should support the goals and KPIs you’ve already set in place so you can assess your web analytics strategy's impact on your results and see your users’ behavior coming to life.
For example, if your goal is to increase purchases made by customers in a specific location, reviewing all customer data from every country isn’t going to give you the insights you need. Instead, segment your data to represent the subset of the customers you’re specifically targeting. Setting strict parameters for analyzing your data from the start saves you from a lot of headaches later on.
You can segment your data into subsets as specific as:
Customers who purchased only a specific product
The number of page views of a certain page
Customers in a certain location
All website traffic that occurred within a particular time frame
Mobile vs desktop users
Purchases made exceeding a certain value
Customers who added something to their cart but didn’t check out
Google Analytics allows for a huge variety of segmentation, so explore your dashboard there. You can also apply Hotjar’s filters to Heatmaps, Recordings, Feedback, and Funnels to enjoy a closer look at specific behavior patterns and site performance within your chosen subsets.
6. Analyze the results
It’s time to analyze the data you’ve collected!
The point of putting a web analytics strategy into place isn’t just to set it out to sail and hope for the best. It’s what you do with the results that really matters.
Remember the lighthouse we mentioned? With your customer as your focal point, setting frequent check-ins, clear targets, and committed deadlines to assess your website performance prevents you from wasting time on something that isn’t working, so you can react to issues as quickly as possible. Actively engaging with your data also ensures your goals stay manageable and actionable.
Let’s go back to an example we mentioned earlier: imagine your goal for Q1 is to increase newsletter sign-ups by 50%. Scheduling regular check-ins throughout the quarter lets you assess performance and ensure you’re on track to achieving your goal. If your progress toward your target is slower than expected, you can look at new optimization opportunities without waiting to see how they play out.
That’s not to say you need to conduct an extensive analysis of your data whenever you want to find out how your website is performing.
We’d suggest saving the deep dives for data collected over longer periods—such as monthly or quarterly—to get the most valuable insights. For more frequent (daily or weekly) performance check-ins, keep your analysis brief and high-level; this is enough to stay on top of your website performance without getting bogged down by micro-details.
Pro tip: Hotjar Heatmaps, Feedback, and Recordings are helpful tools for visualizing your data so it’s easier to share the performance results of your site.
Heatmaps show which parts of a web page capture visitors’ attention (or get ignored), giving you an at-a-glance impression of which parts of your site are being overlooked
Recordings collect every customer’s session on your website, so you can see firsthand how they experience and interact with each page
Feedback and Surveys let your visitors communicate what they think and feel about every element of your website in their own words
Creating engaging, visual, and well-structured performance reports for sharing your results makes otherwise dense information easier for the wider team and stakeholders to understand.
Ultimately, you want your data to be understandable and actionable. Prioritize helping your audience make sense of the data and connect with the individuals behind the numbers.
Where to take your web analytics strategy from here
The next step? It’s simple: keep going!
A customer-centric web analytics strategy is the key to deeper insights —not just into your customers, but also into your business. Keep reviewing your results, assessing the impact of your web analytics strategy, and above all, putting your customer at the front and center of your website optimizations.
By staying objective with the data and continually looking for opportunities to improve your user experience, you’ll be equipped to take your goals and objectives through to long-term success.
Make Hotjar part of your web analytics strategy
Take your website analytics strategy to the next level with behavior analytics and product experience insights from Hotjar.
Web analytics strategy FAQs
A web analytics strategy is the collecting, reporting, and analysis of website data to drive your business goals and better understand your customers and target audience.
What are the key steps of a web analytics strategy?
The steps of a data-driven web strategy are:
Collect website data and customer insights
Define your goals and objectives
Understand where you fit within the marketing funnel
Determine your KPIs
Segment your data for reporting
Analyze the results
What web analytics metrics should I measure?
The metrics you measure will depend on various factors of your website analytics strategy, such as your business objectives, target audience, or where your goals fit within the marketing funnel. However, some of the more common metrics we recommend reviewing are:
Bounce rate
Abandonment rate
Acquisitions
Web analytics guide
Previous chapter
Web analytics tools
Next chapter
Explore web analytics case studies. Find the path toward becoming data‑driven.
Our clients have become confident about making the right digital marketing decisions. their teams understand their business priorities and the path to reach their targets..
Agata continues to keep improving our data in ways we didn’t think were possible. We look forward to working with her from here on out.
James Clayson Director, Played Software
Hexagon is a global leader in sensor, software and metrology solutions. They turn manufacturing plants into smart factories, boosting productivity and product quality for their clients.
This well-established company launched a new ecommerce website to increase the sales of their aftermarket accessories. But without a measurement strategy in place, they were struggling to see their customers’ digital footprint and to accurately report on their webshop performance. Their team were lacking the tools and processes needed to optimise their online sales.
They realised that to grow their online shop further, they needed to connect the dots between customer journeys and sales. Their driving force when contacting Business Ahead was to work towards creating a digital insight function in-house while growing the revenue from the webshop.
The initial Google Analytics audit and measurement strategy defined the path towards becoming data driven for Hexagon. Our collaborative approach suited Hexagon as they were able to see our process and how each activity was bringing them closer to achieving their business goals.
We delivered:
- A measurement strategy with clear roadmap for fixing issues and gaining new insight
- A list of data-driven actions to boost shop performance and generate hundreds of thousands of new revenue within months
- A series of guidelines to keep data consistent and trustworthy
After that, we proceeded with Steady Growth Evolution. We continue to support Hexagon on their road towards getting more clarity and sales.
- Web analytics implementation and maintenance
- Report automation
- Workshops and training sessions
With our solutions, Hexagon has the support it needs to make better informed decisions. As we continue to work together, their team is empowered to embrace more and more of their web analytics.
Business Ahead delivered value beyond our expectations.
“When we got in touch with Business Ahead, we knew our web analytics data was inaccurate – but we didn’t know the extent of the issues and how to resolve them. Agata presented a clear plan of action that was detailed and thorough. Her unique way of communicating and structuring information made it easy to understand and follow – even the technical details.
By having realistic analytics, we are able to make better informed decisions and launch campaigns that are better targeted and measurable.
We were impressed with the insight report: it provided value beyond our expectations. In the past we conducted analysis in-house and with other partners. Agata’s report was more in-depth, better thought-out and gave clear instructions on what to do to gain new revenue, all backed by data. Implementing these recommendations was a no-brainer for us.
The user guides are really helpful for the entire team to get on-board. We have adopted them as our internal standard and best practice for working with Google Analytics data.
We are a very successful organisation but grounded in face-to-face selling and we want to become more cutting-edge. Business Ahead is helping us achieve that and I’m inspired by the progress we’ve already achieved.”
Arif Atkinson Head of Ecommerce
Agata is a whizz with data. She streamlined my reporting processes; I look forward to continuing to learn from Agata.
Steph Bridgeman Director, Experienced Media Analysts
Played Software
Played Software are the creators of an activity finder app. With their database of activities covering the whole of UK, their clients offer local communities an opportunity to find activities near them and book online. Their clients include Change 4 life and Lucozade.
Played Software wanted to provide extra value for their clients by giving them access to app usage data. Unfortunately, web analytics data was either unavailable or incohesive and they were struggling to present it in a meaningful way.
Single page web applications are notoriously problematic when it comes to tracking – and this activity finder app was no exception. We needed to develop a new measurement strategy and implementation from the ground up.
Based on the app’s functionality, we defined the interactions that needed to be measured and the structure for how each element should be tracked. We worked with the developers to adjust some of the code to make sure accurate tracking was possible. Finally, we configured web analytics to collect all the data effectively and we performed all the necessary testing to ensure the data was robust.
Once the data was in place, we also built a series of dashboards that could easily be replicated for additional clients as they came on board.
As a result, Played Software could offer each of their clients a custom-branded dashboard that showed them who visited their finder, which activities they browsed and which ones they booked. This was a key innovation for them and it allowed them to produce a new revenue stream based on the insight generated.
Agata took full ownership of the project, she clearly understood what needed to be done and how to achieve it.
“Analytics and efficiently recording accurate data is a key part of our business. It’s also an area we struggled to find someone who could manage it successfully for us.
Agata took full ownership of the project, she clearly understood what needed to be done and how to achieve it. She removed all the worry from this part of our business and continues to keep improving our data in ways we didn’t think were possible. We look forward to working with her from here on out.”
Agata did some amazing work fixing our existing tracking and analytics set up. She was great at communicating the issue, and very speedy in providing and implementing the solutions. I would highly recommend her to anyone.
James Williams Head of Digital Marketing, IMI Mobile
Assurant is the Fortune 500 brand behind insurance fulfilment solutions for top UK banks.
Assurant has traditionally relied on call centres to log claims for broken, lost or stolen mobile phones and other gadgets. They are in the process of improving their customer experience and are developing ways to save on costs associated with running call centres.
The company is actively developing a state-of-the-art web application that allows users to log a claim and get a decision in seconds. Data is at the centre of ensuring that the apps are optimised and that users can log claims consistently. Unfortunately, when they got in touch with Business Ahead, much of the data was not in place and not ready for the future developments planned.
Agata has been working with Assurant to develop their web application tracking. She has also introduced processes to ensure that all the necessary metrics are meaningful, accessible and robust enough to respond to agile development changes. After understanding what the key interactions were and what the future vision for the product was, we developed the tracking guidelines to systematise user journey tracking and to keep requirements consistent across multiple clients. We continue to work with the Assurant developers to implement new tracking and to educate them on how to produce updates that ensure tracking continuity. We’ve also implemented correct data collection and automated dashboards that help monitor the performance of the applications. Finally, we perform regular analysis to define actions that improve usability and to monitor performance after each release. The tracking and insight generation activities have saved Assurant and their clients millions in call centre costs over the past year.
Let’s go beyond simple onboarding. Let’s create incredible value, right from the start. Let’s chat.
Discover how good data transforms your business. Learn how you can achieve your KPIs with a data measurement roadmap and web analytics expertise.
Schedule a 30‑minute call with Business Ahead founder, Agata.
Weekly Web Analytics Strategies
Business Ahead Ltd is registered in England and Wales. R eg. no.: 11394787.
Privacy policy
Business Ahead work with International Growing Companies, who are at the place where they need their online activities to contribute meaningfully to their business KPIs.
We help our clients become confident about making the right digital marketing decisions. With our support, their teams understand their business priorities and the path to reach their targets.
Our team of analysts are versed in Google Analytics, Google Analytics 360, Google Tag Manager and Google Data Studio. We use these tools to link data with your other sources like CRM, advertising and social media to paint the complete picture of your customers’ journeys.
Based in Manchester, UK, we work with businesses all over the world. We follow our proven framework to help you become data-driven within your own unique set-up.
Start your data-driven journey by signing up for our Making Sense of Web Analytics (MSoWA) Newsletter.
Join the newsletter
Get weekly web analytics strategies for Product and Marketing Managers.
Latest posts
Experts on the top 5 digital analytics trends in 2023 – spotted at measuresummit, 10 qualities to look for when hiring a web analyst, what is google analytics governance everything you need to know, migrating to ga4: 15 recommendations for a smooth transition.
© 2024 All Rights Reserved
Simplifying Search Marketing
60% DECREASE in Pageviews – a Web Analytics Case Study
August 28, 2018 by Scott Benson Leave a Comment
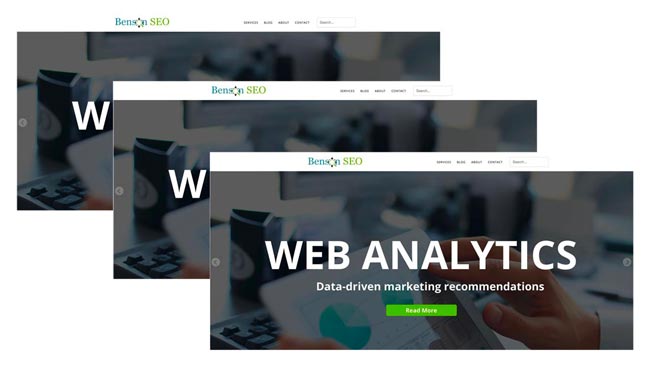
A few months back we had the privilege of working with one of the nation’s top veterans support nonprofits. We were brought in through a partner to take a look at their SEO, but in doing so, immediately noticed some problems with their web analytics – their Google Analytics implementation was not tracking their visitors and their actions correctly. Part of our SEO audit process is to also review a website’s analytics implementation. Our reasoning for insisting on auditing the analytics is simple; you can’t improve what you can’t measure .
This is a website that exists primarily to collect donations to support vets and to sign up new memberships. Measuring how people find the website and which marketing channels are driving those conversions is critical.
How is it that a DECREASE in Pageviews by 60% could be a good thing? Let’s dig in…
Background and Recommendations for Google Analytics and Tag Manager:
- Not all web analytics systems are plug-n-play, even Google Analytics, so watch your implementation, and if you install yourself (out-of-the-box), then hire someone to test it.
- You own your data. Yes, the agencies you work with do need unrestricted access, but you need to know who’s in there and what they’re doing.
- These tools now have change history reports. Learn to use them.
- Yes, you should be running Google Analytics, and all marketing tracking scripts through Google Tag Manager. Here’s a good visual framework to follow for implementation:
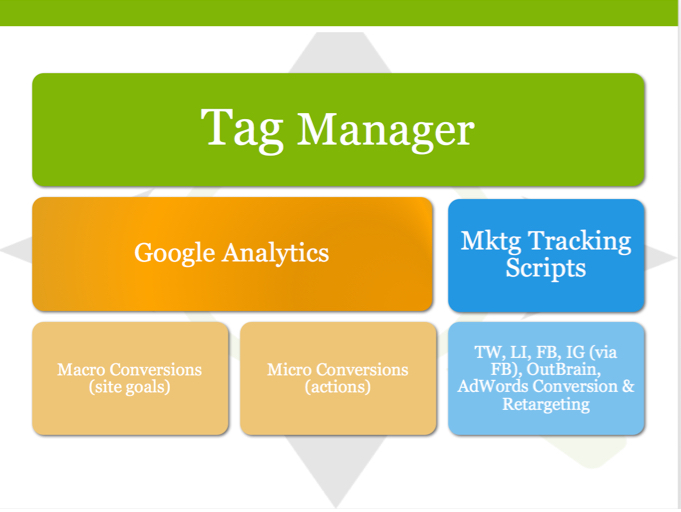
Problem: Bounce Rate Red Flag
One of the first things we noticed when viewing some marketing reporting the organization provided, and jumping into their analytics, was an unbelievably low bounce rate. Now, I should note, I probably care less about bounce rate than most, depending on the type of page I’m reviewing, and the intent of the page, so don’t run screaming for the hills about bounce rate just yet. The problem was really with just how low the bounce rate was that we were seeing. In 2017 and 2018 the site was averaging somewhere around 15% bounce across all page types. Congrats, that’s either the stickiest site I’ve ever seen, or it’s just dead wrong. Well, we knew it was wrong without even finding the source of the problem.
We had to go back to 2015 to find a more normal looking bounce rate, which happened to be around 60% and is quite good. Obviously, we were dealing with a problem that had been around a while and likely longer than a lot of our contacts at the organization.
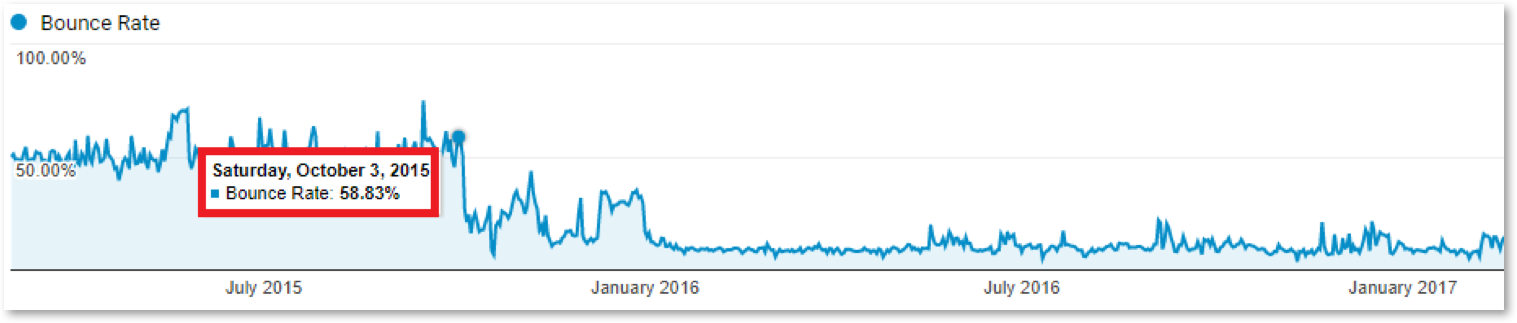
Problem: Triple Counting Pageviews
Once we saw the bounce rate issues, we had to find what was causing it to be so low. There’s a few known issues to check, and luckily it was one of the first tests we ran, and one of the easier to diagnose once you find it. By visiting their site and overriding the traffic source values with Google UTM parameters , we could see in the Real-Time reports in Google Analytics, that on nearly every entrance to the website — no matter the landing page – each of those “hits” were counting 3 times.
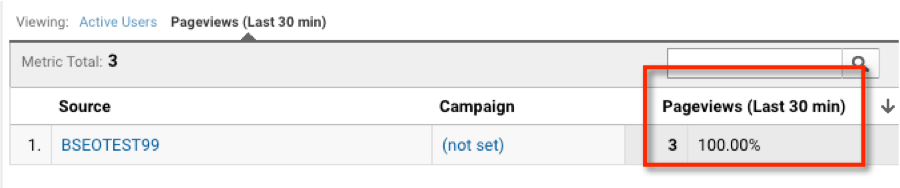
When Does Bounce Rate Matter?
I mentioned earlier my dislike for bounce rate as a metric for success. As an example, if you run a news-oriented site, or a blog, those types of pages historically have a very high bounce rate, and many people incorrectly assume that’s all bad. It’s not. Understanding how Google calculates bounce rate is critical in understanding when it is and isn’t important. If a customer of yours searches for a topic you’ve written about, and finds it on Google.com and clicks, then reads the entire blog post for 5 minutes, consumes the information they need and is fully satisfied by that experience, but they leave your site without clicking another link, then that visit is counted as a bounce by default. That also happens to be a very common user experience with blog posts, as the user finds the answer to the question they entered in Google .
In this case, the way the Google Analytics scripts were implemented was causing additional problems. At some point in those 3 pageviews, the user’s traffic source was being dropped, and some of the user visits were being attributed to “Direct to site”, as if they had typed in the domain name directly into the browser and visited directly.
The biggest issue here is in conversion attribution. The organization relies heavily on a robust email marketing program, and on social media traffic. As those marketing channels sent visitors to the site to donate or sign up their membership, and the users actually did take those actions, unknown numbers of those conversions were incorrectly attributed to Direct to site traffic. This results in the appearance of underperforming marketing campaigns, and an artificial attribution to a high brand performance. Direct site traffic is often assumed to mean the organization has a strong brand recognition, resulting in users simply typing the domain name into a browser.
Where should the CMO throw marketing dollars if the channels that cost money appear to be performing poorly?
Why is Google Analytics Tracking Multiple Pageviews?
Why was all this happening? The site was running two Google Tag Manager containers, and through those, running a combined 3 Google Analytics tracking scripts. Each GTM container was running their Universal Analytics scripts, which up until very recently was the most up-to-date tracking method, but additionally one of the scripts was also running the legacy GA.js Classic script. While Google claims you can run multiple Universal scripts, you can’t run the old and the new together, and likely can only run multiple if they’re actually different accounts, so each pageview is sent to both tracking accounts. I highly recommend the Tag Assistant Chrome plugin by Google , which is one of the tools we used to diagnose the problems.
In the end, the solution to this client’s analytics issues was simple. After some testing, we were able to work with the client to migrate the two GTM containers into one, shut down the Classic GA script, and pause the unnecessary second Universal Analytics script. We migrated all other marketing tracking scripts to the one GTM container, and worked on some of the rules for when those fire. After those fixes the bounce rate normalized to near 60%, which is actually quite good for this type of website, and those seemingly underperforming marketing channels started being attributed to revenue the way they should have been all along. The impact really opened some eyes internally, and we were really pleased with that result. Also as a result of the work to fix their tracking issues, the overall pageview numbers came way down. What was once a “soft” reporting success metric (whether correct or not), was now going to be ignored in favor of better conversion attribution.
Additionally we proposed a new structure for their Google Analytics reporting profiles. Their domain runs several sub-domains for their various marketing and eCommerce efforts. These were all reporting under the default view, and they didn’t have proper backup profiles. We first recommended utilizing a “Production”, “Testing”, and an “Unfiltered” view to make sure our data in the Production view is clean. The Unfiltered view is a data back-up and a best practice for Google Analytics. To properly measure all those sub-domains we recommended utilizing hostname and page path overrides in Google Analytics filters to see each individual site by itself, as well as roll-up reporting views that showed all the properties together, but cleanly showing the sub-domain name in the reports. Creating additional views as outlined below allows for different members of your organization to view only the data they need. For example, if I only work in HR and Recruiting, I might only be interested in what happens on a “jobs.client.org” web property. I don’t care what the marketing team is doing on the “www” site. You can create these departmental views, and have the roll-ups for a broader look at your entire organization.
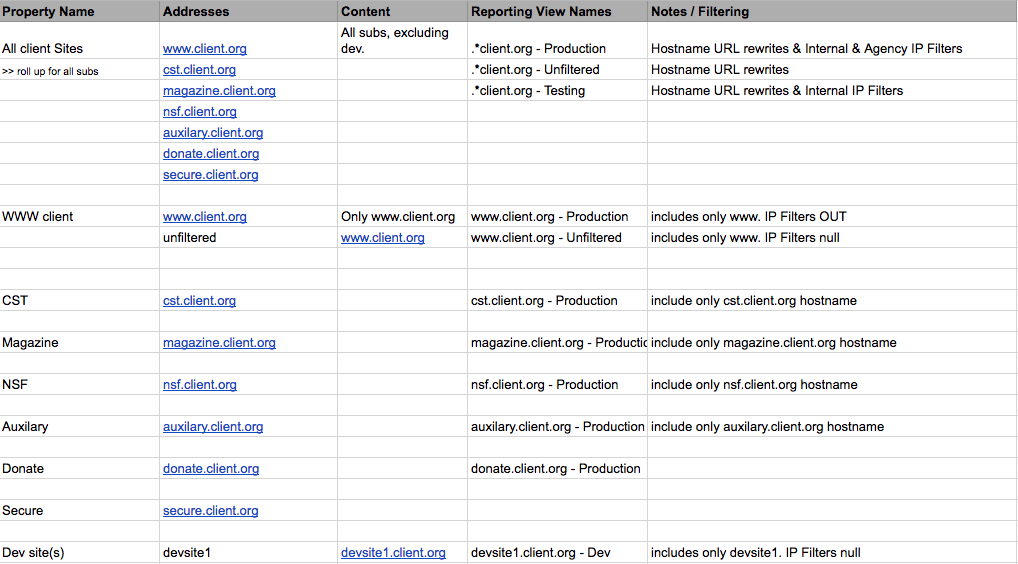
Google Analytics is a fantastic resource, it’s free (with paid versions), and it can be incredibly easy to set up a basic implementation. It is also very easy to get that implementation wrong. Be sure to know what your digital marketing agency, or web development company is doing when they set you up — clean data is imperative to good marketing.
If you’d like an audit of your analytics or SEO performance, contact the best SEO agency in Washington, DC.
Leave a Reply Cancel reply
Your email address will not be published. Required fields are marked *
Save my name, email, and website in this browser for the next time I comment.

Phone: 202-842-5031
Email: [email protected], address: 4702 woodberry street riverdale, md 20737.
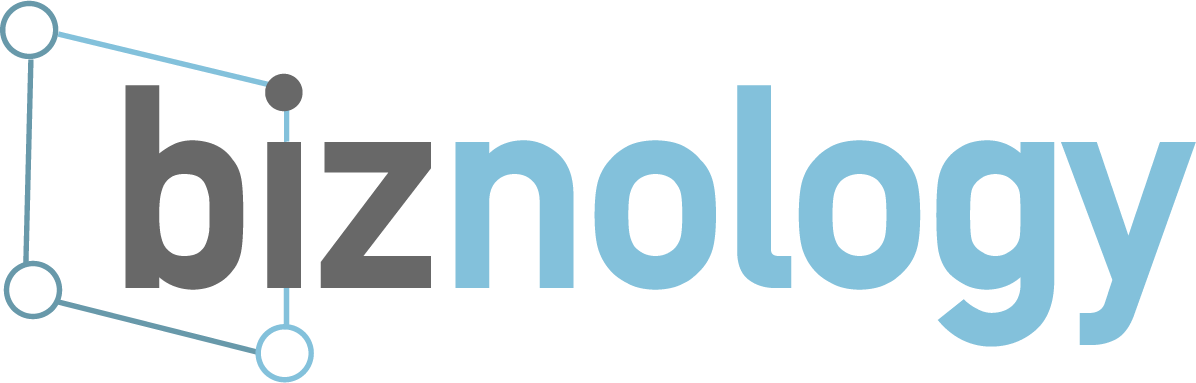
- The Digital Enterprise
- Change & Transformation
- C-Suite Corner
Trending Now
10 case studies show how web analytics prove roi.
- Share on Facebook
- Tweet on Twitter
- BT FINANCIAL GROUP : Is a leading provider of superannuation, investment, and insurance products. The BT website focuses on service and usability with an online application form as one of the key conversion points. Landing pages with different combinations of the design elements for testing are created to optimize the user experience and maximize conversions. With conversion testing, BT increases form completions by more than 60%.
- BUILDDIRECT : does business in more than 100 countries with an expanding portfolio of building materials. Though the company is growing rapidly, management is eager to improve the efficiency of its online spending. Through web analytics, BuildDirect finds home buyers who purchase a sample have a 60% likelihood of returning to the site within the next 30 days and placing a full order, BuildDirect uses GA’s A/B testing capabilities to perfect its marketing approach. With insights from web analytics, BuildDirect increases sales by 50%.
- HARVARD UNIVERSITY : To expand the digital reach of two established schools, Harvard Summer School and Harvard Extension School, Harvard ran a 12-month SEO and PPC campaign. They use web analytics as an audit to identify technical setbacks, content positioning, to create new landing pages for search traffic, and top-of-funnel paid search awareness campaigns. The result are: 1) 89% increase in visits from organic search, 2) 75% increase in registrations from organic search, 3) 30% increase in CTR with AdWords, and 4) 124% increase in ROAS with AdWords.
- KEEN FOOTWEAR : Is an outdoor shoe manufacturing company based in Portland, Oregon. The company needs a better way to measure, analyze, and understand metrics that mattered on their social network to provide meaningful insight. A framework involving reach, engagement, influence, sentiment, and effect is developed. Key Performance Indicators (KPIs) within each area are established. This is using the metrics: 1) Page Likes increase by 92%, 2) Post Reach increase by 342%, 3) Post Engagement increase by 137%, and 4) Active Users increase by 213%.
- MOTOREASY : Is a company that sells extended auto warranties. Motoreasy’s Web site is re-designed to give you a quote for an extended auto warranty on your car. This involves: 1) telling people what you want them to do (fill in the form) and 2) tell them the benefits of doing so (you’ll get a quote which could save you money). The telephone number is featured prominently at the top, making it easy for them to call if they found filling out the online form too tedious. These changes reduce the drop out rate from 65 percent to 29 percent overnight. This increases the completion rate of the sign up page from 31% to 69%.
- NIKE GOLF : Is the golf-specific retail branch of Nike. Although there is the benefit of the Nike brand, there is also the lack of a focused keyword strategy on the Nike Golf website. It is very difficult for search engines to crawl for content. Research helps make decisions like whether target keywords should be “golf apparel,” “golf clothing,” “golf clothes,” or “golf sportswear.” As a result of the research, Nike Golf sees a 169% in total increase in organic search traffic.
- ON THE BEACH: Offers value for money flights and hotels to the world’s most popular beach holiday destinations, providing consumers with a huge selection of travel products, including 50 million airplane seats and more than 30,000 hotels. On the Beach finds that their generic search is undervalued under last click reporting, a discovery that allows the company to build a custom attribution model and increase budget on generic campaigns. This helps drive a higher volume of site traffic, holiday sales, and market share in the travel sector, which in turn led to a 25% increase in ROI.
- PBS : Helps individual PBS producers and local PBS stations create and promote each section within PBS.org. PBS wants to develop a coordinated approach to analysis and reporting that would inform their future strategic decisions. Analysis of search engine trends leads to an increase in PBS traffic by 30%. Web analytics is set up to allow PBS to evaluate the way users consumed video. As a result, PBS increases both conversions and visits by 30%.
- PUMA : Has rich, dynamic web site; but, just as PUMA constantly improves its products, it also believes in making site changes that help visitors easily achieve their goals. While testing its web site header, it finds a variation that increases online orders by 7.1%. Puma more than doubles the amount of time visitors spend interacting with PUMA brand content, such as news, videos, and photos. It results in 47% more traffic.
- RYANAIR : Is Europe’s largest low fare airline. 99% of Ryanair’s bookings are made through its website making it the company’s single most important marketing tool. Web Analytics helps understand email and visitor behaviour. Ryanir is able to increase click-through rates by 200%, decrease bounce rate by 18%, increase visitor traffic by 16% to strategic pages, and double revenue generated from their email campaigns.
Related Posts
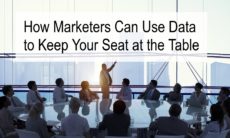
SearchChat Podcast: How Marketers Can Use Data to Keep Your Seat at the Table
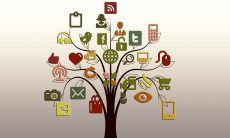
Personal brands for everyone
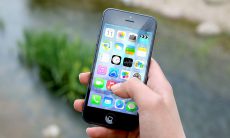
Mobile marketing must-haves in 2017
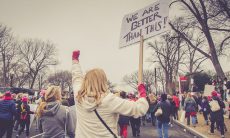
Should a brand take a stand on social issues?
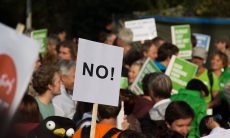
How the new tax bill exacerbates millennials’ frustration with “brand capitalism”
Outside-in marketing: a pragmatic approach to content marketing, join the discussion cancel reply.
Your email address will not be published. Required fields are marked *
POPULAR POSTS
Team flow institute releases recommendations on how to prepare for the successful integration of ai, envisioning the future of human work in the age of ai: the team flow institute 2024 forecast, how digital technologies are revolutionizing the commercial real estate industry, lessons learned from the mgm hack, team flow institute launches to create a collective vision for the fourth industrial revolution.

- Privacy Overview
- Strictly Necessary Cookies
This website uses cookies so that we can provide you with the best user experience possible. Cookie information is stored in your browser and performs functions such as recognising you when you return to our website and helping our team to understand which sections of the website you find most interesting and useful.
Strictly Necessary Cookie should be enabled at all times so that we can save your preferences for cookie settings.
If you disable this cookie, we will not be able to save your preferences. This means that every time you visit this website you will need to enable or disable cookies again.
Safalta Exam Preparation Online
Top 10 google analytics case studies in 2023.
- Whatsapp Channel

She has started her career as a Content Writer and writes on blogs related to career.
Free Demo Classes
Register here for Free Demo Classes
Waw! Just one step away to get free demo classes.

Source: Safalta.com

Download Now: Free digital marketing e-books [ Get your downloaded e-book now ]
- Better accurate data, greater optimizations, and higher ROI
- New apps, tools, and stores may be launched more quickly.
- Website speed has been increased by 8%.
- Tag dissemination was reduced from days to hours.
- Personas for each part were created in depth.
- Using online performances, we determined seven important audience categories.
- Improved features and informed content
- Decreased IT resource strain and expense
- Tag use has been simplified.
- Provided overall tag administration across all GoPro online media.
- Lesser time constraints for new campaign launches
How can I use Google to discover a case study?
What is google analytics' future, what are the five basic google analytics reports.
- Report on AdWords.
- Report on All Pages.
- Report on Traffic Acquisition.
- Audience Feedback.
- Report on E-commerce Tracking.
How many websites will be using Google Analytics in 2022?

Start Learning & Earning
- Digital Marketing
- Job Ready Courses
- Graphic Designing
- Advance Excel

Trending Courses

Master Certification in Digital Marketing Programme (Batch-16)
Now at just ₹ 64999 ₹ 125000 48% off

Professional Certification Programme in Digital Marketing (Batch-10)
Now at just ₹ 49999 ₹ 99999 50% off

Advanced Certification in Digital Marketing Online Programme (Batch-27)
Now at just ₹ 24999 ₹ 35999 31% off

Advance Graphic Designing Course (Batch-10) : 100 Hours of Learning
Now at just ₹ 16999 ₹ 35999 53% off

Flipkart Hot Selling Course in 2024
Now at just ₹ 10000 ₹ 30000 67% off

Advanced Certification in Digital Marketing Classroom Programme (Batch-3)
Now at just ₹ 29999 ₹ 99999 70% off

Basic Digital Marketing Course (Batch-24): 50 Hours Live+ Recorded Classes!
Now at just ₹ 1499 ₹ 9999 85% off

WhatsApp Business Marketing Course
Now at just ₹ 599 ₹ 1599 63% off

Advance Excel Course
Now at just ₹ 2499 ₹ 8000 69% off
अपनी वेबसाइट पर हम डाटा संग्रह टूल्स, जैसे की कुकीज के माध्यम से आपकी जानकारी एकत्र करते हैं ताकि आपको बेहतर अनुभव प्रदान कर सकें, वेबसाइट के ट्रैफिक का विश्लेषण कर सकें, कॉन्टेंट व्यक्तिगत तरीके से पेश कर सकें और हमारे पार्टनर्स, जैसे की Google, और सोशल मीडिया साइट्स, जैसे की Facebook, के साथ लक्षित विज्ञापन पेश करने के लिए उपयोग कर सकें। साथ ही, अगर आप साइन-अप करते हैं, तो हम आपका ईमेल पता, फोन नंबर और अन्य विवरण पूरी तरह सुरक्षित तरीके से स्टोर करते हैं। आप कुकीज नीति पृष्ठ से अपनी कुकीज हटा सकते है और रजिस्टर्ड यूजर अपने प्रोफाइल पेज से अपना व्यक्तिगत डाटा हटा या एक्सपोर्ट कर सकते हैं। हमारी Cookies Policy , Privacy Policy और Terms & Conditions के बारे में पढ़ें और अपनी सहमति देने के लिए Agree पर क्लिक करें।
Download App for Live stream
Live stream is currently not available on web. Kindly download our app.
Popular Searches
- Current Affairs
Most Popular Exams
- CBSE Term 2

You have awarded with Avid Reader-3 for reading 50 ebooks on safalta.com. Keep learning and earn coins and badges.
- Deutschland
- Asia, Australia & New Zealand
- Europe, Middle East & Africa
- United States & Canada
- Latinoamérica
Google Analytics Performance Marketing Case Studies
When you change the way data is collected and analyzed, you gain insights into your customers and their purchase behaviors. The brands in the section below, including Westwing, Travelocity and PBS, did just that with products such as Google Analytics Premium and Universal Analytics.
Share this page
Lenovo: a radically new view of results, accuweather measures holistic analytics with google analytics premium, watchfinder clocks 1,300% roi using precision remarketing with google analytics, westwing uses universal analytics to better understand customers' purchase path, rooms to go improves the shopper experience by integrating google analytics premium.
- Digital Marketing
- Facebook Marketing
- Instagram Marketing
- Ecommerce Marketing
- Content Marketing
- Data Science Certification
- Machine Learning
- Artificial Intelligence
- Data Analytics
- Graphic Design
- Adobe Illustrator
- Web Designing
- UX UI Design
- Interior Design
- Front End Development
- Back End Development Courses
- Business Analytics
- Entrepreneurship
- Supply Chain
- Financial Modeling
- Corporate Finance
- Project Finance
- Harvard University
- Stanford University
- Yale University
- Princeton University
- Duke University
- UC Berkeley
- Harvard University Executive Programs
- MIT Executive Programs
- Stanford University Executive Programs
- Oxford University Executive Programs
- Cambridge University Executive Programs
- Yale University Executive Programs
- Kellog Executive Programs
- CMU Executive Programs
- 45000+ Free Courses
- Free Certification Courses
- Free DigitalDefynd Certificate
- Free Harvard University Courses
- Free MIT Courses
- Free Excel Courses
- Free Google Courses
- Free Finance Courses
- Free Coding Courses
- Free Digital Marketing Courses
Top 10 Marketing Analytics Case Studies [2024]
The power of marketing analytics to transform business decisions is indisputable. Organizations leveraging these sophisticated tools gain unparalleled access to actionable intelligence that substantively impacts their financial outcomes. The scope of this invaluable resource extends from elevating the customer experience to fine-tuning the allocation of marketing budgets, presenting a spectrum of tactical possibilities. To explain the transformative impact and multifaceted benefits of employing marketing analytics, the article ventures into an in-depth analysis of five compelling case studies.
Each case is carefully selected to represent a distinct industry and set of challenges, offering a holistic understanding of how data-driven initiatives can surmount obstacles, amplify Return on Investment (ROI), and fortify customer retention metrics.
Case Study 1: How Amazon Boosted Sales by Personalizing Customer Experience
The situation: a tricky problem in early 2019.
Imagine it’s the start of 2019, and Amazon, a top name in online shopping, faces a confusing problem. Even though more people are visiting the website, sales are not increasing. It is a big deal, and everyone at Amazon wonders what’s happening.
The Problem: Complex Challenges
Figuring out the root problem was not easy. Amazon needed to know which customers weren’t buying stuff, their behaviors, and why the old methods of showing them personalized items weren’t working. It was a complicated issue that needed a smart and modern solution.
Related: Role of Data Analytics in B2B Marketing
The Solution: Using Advanced Tools
That’s when Amazon decided to use more advanced marketing tools. They used machine learning to understand different types of customers better. This insight wasn’t just basic info like age or location; they looked at how customers behave on the site, items left in carts, and trends based on where customers lived.
The Key Numbers: What They Tracked
To understand if the new plan was working, Amazon focused on a few key metrics:
1. Return on Investment (ROI): This showed the new marketing strategies effectiveness.
2. Customer Lifetime Value (CLV): This KPI helped Amazon understand how valuable customers were over the long term.
3. Customer Acquisition Cost (CAC): This measured how costly it was to get new customers.
4. Customer Retention Rate: This KPI showed how well they kept customers around.
5. Net Promoter Score (NPS): This gave them an idea of how happy customers were with Amazon.
The Results: Big Improvements
The new plan worked well, thanks to advanced marketing analytics tools. In just three months, Amazon increased its sales by 25%. Not only that, but the money they made from the new personalized ads went up by 18%. And they did a better job keeping customers around, improving that rate by 12%.
Lessons Learned: What We Can Take Away
So, what did we learn from Amazon’s success?
1. Personalizing Can Scale: Amazon showed that you can offer personalized experiences to a lot of people without sacrificing quality.
2. Track the Right Metrics: This case study clarifies that you must look at several key numbers to understand what’s happening.
3. Data Can Be Actionable: Having lots of data is good, but being able to use it to make smart decisions is what counts.
Related: Tips to Succeed with Marketing Analytics
Case Study 2: McDonald’s – Decoding Social Media Engagement Through Real-time Analytics
Setting the stage: a tantalizing opportunity beckons.
Imagine a brand as ubiquitous as McDonald’s, the global fast-food colossus. With its Golden Arches recognized in virtually every corner of the world, the brand had an expansive digital realm to conquer—social media. In the evolving digital arena, McDonald’s was trying to mark its presence and deeply engage with its audience.
The Maze of Complexity: A Web of Challenges
Steering the complicated world of social media isn’t for the faint-hearted, especially when catering to a customer base as diverse as McDonald’s. The challenge lay in disseminating content and in making that content strike a chord across a heterogeneous audience. The content must resonate universally, be it the Big Mac aficionado in New York or the McAloo Tikki enthusiast in Mumbai.
The Game Plan: A Data-driven Strategy
McDonald’s adopted a strategy that was nothing short of a data-driven symphony. Utilizing real-time analytics, the brand monitored a series of Key Performance Indicators (KPIs) to track the impact of its social media content:
1. Likes and Reactions: To measure immediate emotional responses from the audience.
2. Shares and Retweets: To gauge the virality potential of their content.
3. Impressions and Reach: To assess the scope and scale of engagement.
4. Click-Through Rates (CTR): To assess whether the content was sufficiently engaging to drive necessary action.
Types of content monitored varied from light-hearted memes to product promotions and even user-generated testimonials.
Related: Difference Between Marketing Analytics and Business Analytics
The Finale: Exceptional Outcomes and a Standing Ovation
The result? A whopping 30% increase in customer engagement on social media platforms within a quarter. But that’s not the end of the story. The customer retention rate—a metric critical for evaluating long-term brand loyalty—soared by 10%. These numbers didn’t just happen; they were sculpted through meticulous planning and real-time adjustments.
The Wisdom Gleaned: Eye-opening Insights and Key Takeaways
Several critical insights emerged from this exercise in digital finesse:
1. Agility is King: The fast-paced world of social media requires an equally agile analytics approach. Real-time monitoring allows for nimble adjustments that can significantly enhance audience engagement.
2. Diverse Audiences Require Tailored Approaches: The ‘one-size-fits-all’ approach is a fallacy in today’s digital age. Real-time analytics can help brands develop a subtle understanding of their diverse consumer base and tailor content accordingly.
3. Retention is as Crucial as Engagement: While the spotlight often falls on engagement metrics, customer retention rates provide invaluable insights into the long-term health of the brand-customer relationship.
4. Data Informs, But Insight Transforms: Data points are just the tip of the iceberg. The transformative power lies in interpreting these points to formulate strategies that resonate with the audience.
Related: VP of Marketing Interview Questions
Case Study 3: Zara—Harnessing Predictive Analytics for Seamless Inventory Management
The prelude: zara’s global dominance meets inventory complexities.
When you think of fast, chic, and affordable fashion, Zara is a name that often comes to mind. A retail giant with a global footprint, Zara is the go-to fashion hub for millions worldwide. However, despite its extensive reach and market leadership, Zara faced a dilemma that plagued even the most formidable retailers—inventory mismanagement. Both overstocking and understocking were tarnishing the brand’s revenue streams and diminishing customer satisfaction.
The Conundrum: A Dynamic Industry with Static Models
The fashion sector is a rapidly evolving giant, where the ups and downs of trends and consumer preferences create a landscape that is as dynamic as it is unpredictable. Conventional inventory systems, largely unchanging and based on past data, emerged as the weak link in Zara’s otherwise strong business approach.
The Tactical Shift: Machine Learning to the Rescue
Recognizing the inherent limitations of traditional approaches, Zara turned to predictive analytics as their technological savior. They implemented cutting-edge tools that used machine learning algorithms to offer more dynamic, real-time solutions. The tools were programmed to consider a multitude of variables:
1. Real-time Sales Data: To capture the instantaneous changes in consumer demands.
2. Seasonal Trends: To account for cyclical variations in sales.
3. Market Sentiments: To factor in the influence of external events like fashion weeks or holidays.
Related: MBA in Marketing Pros and Cons
The Metrics Under the Microscope
Zara’s analytics model put a spotlight on the following KPIs:
1. Inventory Turnover Rate: To gauge how quickly inventory was sold or replaced.
2. Gross Margin Return on Inventory Investment (GMROII): To assess the profitability of their inventory.
3. Stock-to-Sales Ratio: To balance the inventory levels with sales data.
4. Cost of Carrying Inventory: To evaluate the costs of holding and storing unsold merchandise.
The Aftermath: A Success Story Written in Numbers
The results were startlingly positive. Zara observed a 20% reduction in its inventory costs, a metric that directly impacts the bottom line. Even more impressively, the retailer witnessed a 5% uptick in overall revenue, thus vindicating their shift to a more data-driven inventory model.
The Gold Nuggets: Key Takeaways and Strategic Insights
1. Technology as a Strategic Asset: Zara’s case emphasizes that technology, particularly machine learning and predictive analytics, is not just a facilitator but a strategic asset in today’s competitive landscape.
2. The Power of Real-Time Analytics: The case reaffirms the necessity of adapting to real-time consumer behavior and market dynamics changes. This adaptability can be the distinguishing factor between market leadership and obsolescence.
3. Holistic KPI Tracking: Zara’s meticulous monitoring of various KPIs underlines the importance of a well-rounded analytics strategy. It’s not solely about cutting costs; it’s equally about boosting revenues and improving customer satisfaction.
4. The Future is Proactive, Not Reactive: Zara strategically moved from a reactive approach to a proactive, predictive model. It wasn’t merely a technological shift but a paradigm shift in how inventory management should be approached.
Related: Hobby Ideas for Marketing Leaders
Case Study 4: Microsoft—Decoding Public Sentiment for Robust Brand Management
Background: microsoft’s expansive reach and the perils of public opinion.
Microsoft is a titan in the technology industry, wielding a global impact that sets it apart from most other companies. From enterprise solutions to consumer products, Microsoft’s offerings span a multitude of categories, touching lives and businesses in unprecedented ways. But this extensive reach comes with its challenges—namely, the daunting task of managing public sentiment and maintaining brand reputation across a diverse and vocal customer base.
The Intricacies: Coping with a Data Deluge
The issue wasn’t just what people said about Microsoft but the sheer volume of those conversations. Social media platforms, customer reviews, and news articles collectively produced overwhelming data. Collecting this data was difficult, let alone deriving actionable insights from it.
The Playbook: Employing Sentiment Analysis for Real-time Insights
Microsoft addressed this issue head-on by embracing sentiment analysis tools. These tools, often leveraging Natural Language Processing (NLP) and machine learning, parsed through the voluminous data to categorize public sentiments into three buckets:
1. Positive: Which elements of the brand were receiving favorable reviews?
2. Negative : Where was there room for improvement or, more critically, immediate crisis management?
3. Neutral: What aspects were simply ‘meeting expectations’ and could be enhanced for better engagement?
Related: How to Become a Marketing Thought Leader?
Metrics that Mattered
Among the KPIs that Microsoft tracked were:
1. Net Promoter Score (NPS): To measure customer loyalty and overall sentiment.
2. Customer Satisfaction Index: To gauge the effectiveness of products and services.
3. Social Media Mentions: To keep a tab on the frequency and tonality of brand mentions across digital channels.
4. Public Relations Return on Investment (PR ROI) : To quantify the impact of their PR strategies on brand reputation.
Outcomes: A Leap in Brand Reputation and Diminished Negativity
The result was a 15% improvement in Microsoft’s Brand Reputation Score. Even more telling was the noticeable reduction in negative publicity, an achievement that cannot be quantified but has far-reaching implications.
Epilogue: Lessons Learned and Future Directions
Precision Over Ambiguity: Sentiment analysis provides precise metrics over ambiguous opinions, offering actionable insights for immediate brand management strategies.
1. Proactive Vs. Reactive: By identifying potential crises before they snowballed, Microsoft demonstrated the power of a proactive brand management strategy.
2. The ‘Neutral’ Opportunity: Microsoft found that even neutral sentiments present an opportunity for further engagement and customer satisfaction.
3. Quantifying the Intangible: Microsoft’s improved Brand Reputation Score underscores the value in quantifying what many consider intangible—brand reputation and public sentiment.
Related: Reasons Why Marketing Managers Get Fired
Case Study 5: Salesforce—Attribution Modeling Unlocks the Full Potential of Marketing Channels
Background: salesforce’s prowess meets marketing complexity.
Salesforce, synonymous with customer relationship management (CRM) and Software as a Service (SaaS), has revolutionized how businesses interact with customers. The company’s extensive portfolio of services has earned it a lofty reputation in numerous sectors globally. Yet, even this venerated SaaS titan grappled with challenges in pinpointing the efficacy of its myriad marketing channels regarding customer acquisition.
The Challenge: Decoding the Marketing Mix
Salesforce diversified its marketing investments across multiple channels—from search engine optimization (SEO) to pay-per-click (PPC) campaigns and email marketing. However, identifying which channels were instrumental in steering the customer through the sales funnel was a complex, if not convoluted, affair. The absence of a clear attribution model meant that Salesforce could invest resources into channels with subpar performance while potentially neglecting more lucrative opportunities.
The Solution: Attribution Modeling as the Rosetta Stone
To unravel this Gordian Knot, Salesforce employed attribution modeling—a sophisticated analytics technique designed to quantify the impact of each touchpoint on the customer journey. This model shed light on crucial metrics such as:
1. Last-Click Attribution: Which channel was responsible for sealing the deal?
2. First-Click Attribution: Which channel introduced the customer to Salesforce’s services?
3. Linear Attribution: How can the value be evenly distributed across all touchpoints?
4. Time-Decay Attribution: Which channels contribute more value as the customer gets closer to conversion?
The Dashboard of Key Performance Indicators (KPIs)
Among the KPIs that Salesforce monitored were:
1. Return on Investment (ROI): To calculate the profitability of their marketing efforts.
2. Customer Lifetime Value (CLV): To gauge the long-term value brought in by each acquired customer.
3. Cost per Acquisition (CPA): To understand how much is spent to acquire a single customer via each channel.
4. Channel Efficiency Ratio (CER): To evaluate the cost-effectiveness of each marketing channel.
Related: How to Become a Chief Marketing Officer?
Results: A Refined Marketing Strategy Paying Dividends
By adopting attribution modeling, Salesforce could make data-driven decisions to allocate their marketing budget judiciously. The outcome? A notable 10% surge in overall revenue and a 5% increase in ROI. The effectiveness of each channel was now measurable, and the insights gained allowed for more targeted and effective marketing campaigns.
Postscript: Reflective Takeaways and Industry Wisdom
1. Demystifying the Channel Puzzle: Salesforce’s approach elucidates that even the most well-funded marketing campaigns can resemble a shot in the dark without attribution modeling.
2. Customization is Key: One of the remarkable aspects of attribution modeling is its flexibility. Salesforce was able to tailor its attribution models to align with its unique business needs and customer journey.
3. Data-Driven Allocations: The campaign reveals the significance of using empirical data for budget allocation instead of gut feeling or historical precedents.
4. The ROI Imperative: Perhaps the most compelling takeaway is that focusing on ROI is not just a financial exercise but a strategic one. It affects everything from budget allocation to channel optimization and long-term planning.
Related: How Can CMO Use Marketing Analytics?
Case Study 6: Starbucks – Revolutionizing Customer Loyalty with Analytics-Driven Rewards
The backdrop: starbucks’ quest for enhanced customer loyalty.
Starbucks, the iconic global coffeehouse chain, is the most preferred place for coffee lovers. Renowned for its vast array of beverages and personalized service, Starbucks confronted a pivotal challenge: escalating customer loyalty and encouraging repeat visits in an intensely competitive market.
The Dilemma: Deciphering Consumer Desires in a Competitive Arena
In the dynamic landscape of the coffee industry, understanding and catering to evolving customer preferences is paramount. Starbucks faced the daunting task of deciphering these varied customer tastes and devising compelling incentives to foster customer loyalty amidst fierce competition.
The Strategic Overhaul: Leveraging Analytics in the Loyalty Program
Starbucks revamped its loyalty program by embracing a data-driven approach and deploying sophisticated analytics to harvest and interpret customer data. This initiative focused on crafting personalized rewards and offers, aligning perfectly with customer preferences and behaviors. The analytics framework delved into:
1. Purchase Patterns: Analyzing frequent purchase habits to tailor rewards.
2. Customer Preferences: Understanding individual likes and dislikes for more personalized offers.
3. Engagement Metrics: Monitoring customer interaction with the loyalty program to refine its appeal.
The Analytical Lens: Focused KPIs
Starbucks’ revamped loyalty program was scrutinized through these key performance indicators:
1. Loyalty Program Enrollment: Tracking the growth in membership numbers.
2. Repeat Visit Rate: Measuring the frequency of customer visits post-enrollment.
3. Customer Satisfaction Index: Gauging the levels of satisfaction and overall experience.
4. Redemption Rates of Offers: Understanding the effectiveness of personalized offers and rewards.
The Triumph: A Narrative of Success through Numbers
The implementation of analytics in the loyalty program bore significant fruit. Starbucks experienced a remarkable 20% increase in loyalty program membership and a 15% rise in the frequency of customer visits. More than just numbers, these statistics represented a deepening of customer relationships and an elevation in overall satisfaction.

The Crux of Wisdom: Essential Insights and Strategic Perspectives
1. Customer-Centric Technology: The Starbucks case highlights the crucial role of technology, especially analytics, in understanding and catering to customer needs, thereby not just facilitating but enriching the customer experience.
2. Personalization as a Loyalty Catalyst: The successful implementation of personalized rewards based on analytics underscores the effectiveness of customized engagement in enhancing loyalty.
3. Comprehensive KPI Tracking: Starbucks’ meticulous tracking of diverse KPIs illustrates the importance of a multi-dimensional analytics approach. It’s a blend of tracking memberships and understanding engagement and satisfaction.
4. Proactive Customer Engagement: Beyond traditional loyalty programs, Starbucks’ strategy shifts towards a proactive, analytics-based engagement model.
Related: Marketing Executive Interview Questions
Case Study 7: Uber – Revolutionizing Ride-Hailing with Predictive Analytics
Setting the scene: uber’s mission to refine ride-hailing.
Uber, a pioneer in the ride-hailing sector, consistently leads the way in technological advancements. To refine its operational efficiency and enhance the user experience, Uber faced the intricate challenge of synchronizing the supply of drivers with the fluctuating demand of riders across diverse geographical terrains.
The Challenge: Harmonizing Supply and Demand
The core challenge for Uber lies in efficiently balancing the availability of drivers with the dynamically changing needs of customers in different locations. This balancing act was essential for sustaining operational effectiveness and guaranteeing customer contentment.
The Strategic Move: Embracing Real-Time Data Analytics
In response, Uber turned to the power of real-time analytics. This strategic shift involved:
1. Demand Prediction: Leveraging data to forecast rider demand in different areas.
2. Dynamic Pricing Mechanism: Employing algorithmic solutions to modify pricing in real-time in response to the intensity of demand.
3. Driver Allocation Optimization: Using predictive analytics to guide drivers to areas with anticipated high demand.
Results: Measurable Gains in Efficiency and Satisfaction
The results of this approach, grounded in data analytics, were impressive. Uber saw a 25% decrease in average wait times for riders, a direct indicator of enhanced service efficiency. Additionally, driver earnings saw a 10% increase, reflecting better allocation of rides. Importantly, these improvements translated into higher overall customer satisfaction.
Related: Is Becoming a CMO Worth It?
Case Study 8: Spotify – Harnessing Music Analytics for Enhanced Personalization
Backstory: spotify’s pursuit of personalized music experience.
Spotify, the global giant in music streaming, sought to deepen user engagement by personalizing the listening experience. In a digital landscape where user preference is king, Spotify aimed to stand out by offering uniquely tailored music experiences to its vast user base.
The Challenge: Navigating a Sea of Diverse Musical Tastes
With an expansive library of music, Spotify faced the critical task of catering to the incredibly diverse tastes of its users. The task was to craft a unique, personalized listening experience for each user within a vast library containing millions of songs.
The Strategy: Leveraging Machine Learning for Custom Playlists
To address this, Spotify deployed machine learning algorithms in a multifaceted strategy:
1. Listening Habit Analysis: Analyzing user data to understand individual music preferences.
2. Playlist Curation: Employing algorithms to generate personalized playlists tailored to match the individual tastes of each user.
3. Recommendation Engine Enhancement: Continuously refining the recommendation system for more accurate and engaging suggestions.
Results: A Symphony of User Engagement and Loyalty
Implementing these machine-learning strategies led to a remarkable 30% increase in user engagement. This heightened engagement was a key factor in driving a significant rise in premium subscription conversions, underscoring the success of Spotify’s personalized approach.
Related: How Can Creating a Course Lead to Marketing Your Business?
Case Study 9: Airbnb – Advancing Market Positioning and Pricing with Strategic Analytics
Overview: airbnb’s quest for pricing and positioning excellence.
Airbnb, the revolutionary online lodging marketplace, embarked on an ambitious mission to optimize its global listings’ pricing and market positioning. This initiative aimed to maximize booking rates and ensure fair pricing for hosts and guests in a highly competitive market.
The Challenge: Mastering Competitive Pricing in a Diverse Market
Airbnb’s main challenge was pinpointing competitive pricing strategies that would work across its vast array of worldwide listings. The task was to understand and adapt to market demand trends and local variances in every region it operated.
The Strategic Approach: Dynamic Pricing Through Data Analytics
To achieve this, Airbnb turned to the power of analytics, developing a dynamic pricing model that was sensitive to various factors:
1. Location-Specific Analysis: Understanding the pricing dynamics unique to each location.
2. Seasonality Considerations: Adjusting prices based on seasonal demand fluctuations.
3. Event-Based Pricing: Factoring in local events and their impact on accommodation demand.
Results: A Story of Enhanced Performance and Satisfaction
This analytical approach reaped significant rewards. Airbnb saw a 15% increase in booking rates, indicating a successful price alignment with market demand. Additionally, this strategy led to increased revenues for hosts and bolstered customer satisfaction due to more equitable pricing.
Case Study 10: Domino’s – Transforming Pizza Delivery with Analytics-Driven Logistics
Background: domino’s drive for enhanced delivery and service.
Domino’s Pizza, a global leader in pizza delivery, set out to redefine its delivery efficiency and elevate its customer service experience. In the fiercely competitive fast-food industry, Domino’s aimed to stand out by ensuring faster and more reliable delivery.
The Challenge: Streamlining Deliveries in a Fast-Paced Environment
The critical challenge for Domino’s was ensuring timely deliveries while maintaining food quality during transit. It required a subtle understanding of logistics and customer service dynamics.
The Strategy: Optimizing Delivery with Data and Technology
Domino’s responded to this challenge by implementing sophisticated logistics analytics:
1. Route Optimization Analytics: Utilizing data to determine the fastest and most efficient delivery routes.
2. Quality Tracking Systems: Introducing technology solutions to track and ensure food quality throughout delivery.
Results: Measurable Gains in Efficiency and Customer Satisfaction
Adopting these strategies led to a notable 20% reduction in delivery times. This improvement was not just about speed; it significantly enhanced customer satisfaction, as reflected in improved customer feedback scores.
Conclusion: The Transformative Impact of Marketing Analytics in Action
Wrapping up our exploration of these five case studies, one unambiguous insight stands out: the effective application of marketing analytics is pivotal for achieving substantial business gains.
1. Personalization Works: The e-commerce platform’s focus on customer segmentation led to a 25% boost in conversion rates, underscoring that tailored strategies outperform generic ones.
2. Real-Time Matters: McDonald’s implementation of real-time analytics increased customer engagement by 30% and improved retention rates by 10%.
3. Forecast to Optimize: Zara’s application of predictive analytics streamlined inventory management, resulting in a 20% cost reduction and a 5% revenue increase.
4. Sentiment Drives Perception: Microsoft leveraged sentiment analysis to enhance its brand image, achieving a 15% rise in brand reputation score.
5. Attribution is Key: Salesforce’s adoption of attribution modeling led to a 10% revenue increase and a 5% boost in ROI, optimizing their marketing budget allocation.
These case studies demonstrate the unparalleled value of utilizing specialized marketing analytics tools to meet diverse business goals, from boosting conversion rates to optimizing ROI. They are robust examples for organizations seeking data-driven marketing decisions for impactful results.
- Top 30 Finance Leadership Interview Questions and Answers [2024]
- 10 Tips to Crack That CMO Interview [2024]
Team DigitalDefynd
We help you find the best courses, certifications, and tutorials online. Hundreds of experts come together to handpick these recommendations based on decades of collective experience. So far we have served 4 Million+ satisfied learners and counting.
Is Digital Marketing the Right Career Choice for You? [2024]

10 Offline Marketing Ideas for an Online Course [2024]

Is Data Analytics a Safe Career Option? [2024]

Top 30 VP of Marketing Interview Questions and Answers [2024]

10 Alternative Career Paths for Analytics Professionals [2024]

Top 10 Social Media Interview Questions and Answers [2024]
- Español – América Latina
- Português – Brasil
Domino’s: Increasing monthly revenue by 6% with Google Analytics Premium, Google Tag Manager, and Google BigQuery
About Domino’s
Pizza seller and digital innovator, Domino’s is the leading pizza delivery chain in the UK and the Republic of Ireland. It is also the master franchise in Germany and Switzerland. UK Headquarters: Milton Keynes, England.
Tell us your challenge. We're here to help.
DBi marketing technology and data experts enable brands to leverage analytics to drive business performance. DBi is a Google Analytics Certified Partner and a Google Analytics Premium Authorized Reseller. Headquarters: London, England. Learn more at www.dbi.io .
Google Cloud Platform results
- Realizes an immediate 6% increase in monthly revenue
- Saves 80% YOY in ad serving and operations costs
- Increases agility with streamlined tag management
- Obtains easy access to powerful reporting and customized dashboards
Well-known pizza purveyor Domino’s is dominating pizza delivery sales in countries around the world. Today, Domino’s is the most popular pizza delivery chain operating in the UK, the Republic of Ireland, Germany, and Switzerland — and sales just keep growing.
In these regions in 2014, Domino’s sold 76 million pizzas and generated £766.6 million in revenue — a 14.6% increase from the previous year. In the UK and Ireland, online sales are increasing 30% year-over-year and currently account for almost 70% of all sales. Notably, 44% of those online sales are now made via mobile devices.
Multi-device pizza purchasing delivers fresh data opportunities
Domino’s has been a consistent digital innovator. Much of the pizza purveyor’s success stems from its early investments in strong ecommerce and m-commerce platforms that enable customers to purchase pizzas easily.
Domino’s sold its first pizza online in 1999. It launched an iPhone app in 2010, quickly followed by apps for Android and iPad in 2011, and a Windows app in 2012. By late 2014, Domino’s customers could even order pizzas from their Xboxes. The Domino’s marketing team had assembled a variety of tools to measure marketing performance, keeping pace with the company’s rapid innovations. Unfortunately, dealing with siloed analytics data from channel-focused tools was restricting the team’s ability to gain insights across all devices and channels.
The Domino’s team knew that valuable data insights were waiting just out of reach. To drive success, the team knew they must break down silos, connect datasets, and gain efficient reporting to get a more holistic and actionable view of customer behavior.
Better tag management propels agility across channels
The company's three main goals:
- Integrate marketing measurement across devices
- Connect CRM and digital data to create a holistic view of customer behavior
- Make cross-channel marketing performance analysis easy and efficient
The approach they decided would help them get there:
- Use Google Analytics Premium , Google Tag Manager, and BigQuery to integrate digital data sources and CRM data
Having taken strategic steps in its partnership with DBi, a Google Analytics Premium Authorized Reseller, Domino’s has turned its team goal of unified marketing measurement, holistic insights, and efficient actionability into a day-to-day reality.
For all of this to be possible, DBi leveraged the power of the data layer, a repository of information written into the page code used to store and send information to Google Tag Manager. Because the data layer stays independent of the HTML page structure, it remains consistent when the page content is updated and provides reliable, unchanging data sources for Google Tag Manager containers to pull from.
DBi deployed Google Tag Manager across many of Domino’s apps and websites, setting customized tags for all of the company’s ecommerce tracking and reporting needs. Despite there being a large number of unique containers, data layer consistency makes it easy to duplicate tags and rules — a significant time-saver and error preventor for Domino’s.
Connecting datasets provides holistic customer insights
Next, Domino’s and DBi turned their efforts toward connecting valuable datasets. Although Domino’s had extensive customer data, including demographic information, order frequency, and order method, the company needed to merge this data with digital analytics to enable deeper analysis of consumer behaviors and preferences.
With that goal in mind, DBi developed a custom solution using BigQuery to store and query Domino’s massive datasets in a fast, efficient, and affordable way. Using the BigQuery export feature in Google Analytics Premium, Domino’s can automatically export raw data to a BigQuery project on a daily basis. A secured FTP location and the BigQuery API enable daily automated uploads of CRM data into the BigQuery database on the Google Cloud.
Following the process described above, CRM data became easily merged with Google Analytics digital data via transaction IDs. Because BigQuery can process gigabytes of data in seconds, reporting queries are easy to build and automate. A report examining customer type by marketing channel, for example, reveals which marketing channels or keywords influence customer segments the most.
Google Analytics Premium drives significant results
Since implementing Google Analytics Premium, the ability to access a single Google Analytics account to evaluate web and app performance has made reporting easier and more efficient, and it has furthered the company’s ability to analyze and capture opportunities.
Integrated cross-device tracking has uncovered new insights into customer behavior, allowing the Domino’s marketing team to save 80% year-over-year in ad serving and operations costs.
The new Google Tag Manager implementation allows Domino’s to act fast. Tags can now be created, reviewed, and published in days, rather than having to wait months to catch the next development cycle. In fact, Domino’s used Google Tag Manager to quickly implement an on-site targeting tool that captured and realized an immediate 6% increase in monthly revenue — percentage points that would have been lost each month the project was delayed.
Lastly, connecting CRM data with digital analytics data provided Domino’s with greater visibility into how marketing efforts influence customers. This has enabled the Domino’s marketing team to make better budget allocation decisions and further improve ROI. The team can also customize powerful reports and dashboards to communicate its successes to business stakeholders.
With Google Analytics Premium in place, Domino’s benefits from data-informed decision-making. Going forward, DBi will continue to help Domino’s leverage every ounce of value made possible by Google Analytics Premium. Customized solutions, including Google Tag Manager and BigQuery, drive deeper customer understanding and better marketing strategies.
“Google Analytics Premium, combined with Google Tag Manager and BigQuery, has become an integral solution that gives us the technical agility and the analytics power we need to advance our marketing strategies."

FOR EMPLOYERS
Top 10 real-world data science case studies.
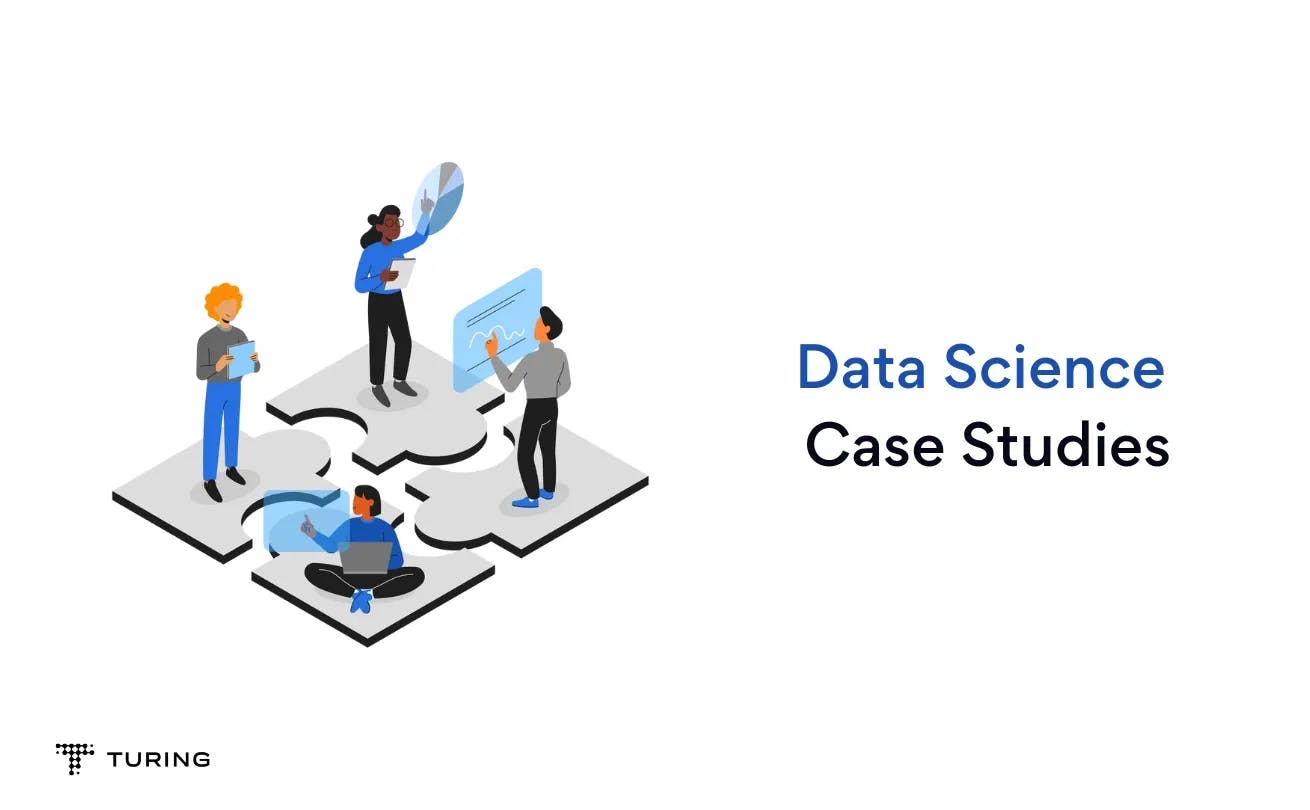
Aditya Sharma
Aditya is a content writer with 5+ years of experience writing for various industries including Marketing, SaaS, B2B, IT, and Edtech among others. You can find him watching anime or playing games when he’s not writing.
Frequently Asked Questions
Real-world data science case studies differ significantly from academic examples. While academic exercises often feature clean, well-structured data and simplified scenarios, real-world projects tackle messy, diverse data sources with practical constraints and genuine business objectives. These case studies reflect the complexities data scientists face when translating data into actionable insights in the corporate world.
Real-world data science projects come with common challenges. Data quality issues, including missing or inaccurate data, can hinder analysis. Domain expertise gaps may result in misinterpretation of results. Resource constraints might limit project scope or access to necessary tools and talent. Ethical considerations, like privacy and bias, demand careful handling.
Lastly, as data and business needs evolve, data science projects must adapt and stay relevant, posing an ongoing challenge.
Real-world data science case studies play a crucial role in helping companies make informed decisions. By analyzing their own data, businesses gain valuable insights into customer behavior, market trends, and operational efficiencies.
These insights empower data-driven strategies, aiding in more effective resource allocation, product development, and marketing efforts. Ultimately, case studies bridge the gap between data science and business decision-making, enhancing a company's ability to thrive in a competitive landscape.
Key takeaways from these case studies for organizations include the importance of cultivating a data-driven culture that values evidence-based decision-making. Investing in robust data infrastructure is essential to support data initiatives. Collaborating closely between data scientists and domain experts ensures that insights align with business goals.
Finally, continuous monitoring and refinement of data solutions are critical for maintaining relevance and effectiveness in a dynamic business environment. Embracing these principles can lead to tangible benefits and sustainable success in real-world data science endeavors.
Data science is a powerful driver of innovation and problem-solving across diverse industries. By harnessing data, organizations can uncover hidden patterns, automate repetitive tasks, optimize operations, and make informed decisions.
In healthcare, for example, data-driven diagnostics and treatment plans improve patient outcomes. In finance, predictive analytics enhances risk management. In transportation, route optimization reduces costs and emissions. Data science empowers industries to innovate and solve complex challenges in ways that were previously unimaginable.
Hire remote developers
Tell us the skills you need and we'll find the best developer for you in days, not weeks.
10 Real World Data Science Case Studies Projects with Example
Top 10 Data Science Case Studies Projects with Examples and Solutions in Python to inspire your data science learning in 2023.
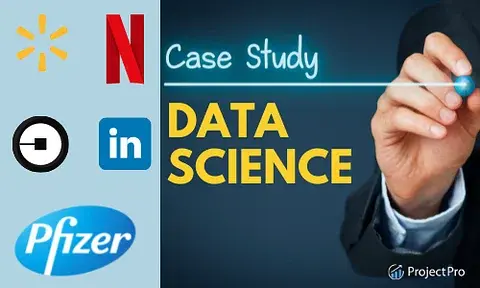
BelData science has been a trending buzzword in recent times. With wide applications in various sectors like healthcare , education, retail, transportation, media, and banking -data science applications are at the core of pretty much every industry out there. The possibilities are endless: analysis of frauds in the finance sector or the personalization of recommendations on eCommerce businesses. We have developed ten exciting data science case studies to explain how data science is leveraged across various industries to make smarter decisions and develop innovative personalized products tailored to specific customers.

Walmart Sales Forecasting Data Science Project
Downloadable solution code | Explanatory videos | Tech Support
Table of Contents
Data science case studies in retail , data science case study examples in entertainment industry , data analytics case study examples in travel industry , case studies for data analytics in social media , real world data science projects in healthcare, data analytics case studies in oil and gas, what is a case study in data science, how do you prepare a data science case study, 10 most interesting data science case studies with examples.
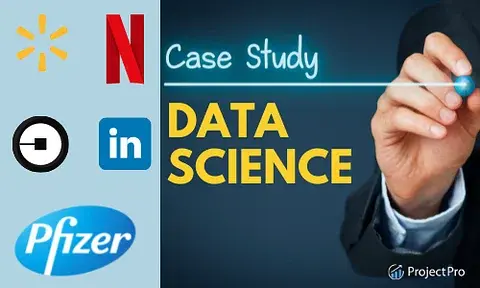
So, without much ado, let's get started with data science business case studies !
With humble beginnings as a simple discount retailer, today, Walmart operates in 10,500 stores and clubs in 24 countries and eCommerce websites, employing around 2.2 million people around the globe. For the fiscal year ended January 31, 2021, Walmart's total revenue was $559 billion showing a growth of $35 billion with the expansion of the eCommerce sector. Walmart is a data-driven company that works on the principle of 'Everyday low cost' for its consumers. To achieve this goal, they heavily depend on the advances of their data science and analytics department for research and development, also known as Walmart Labs. Walmart is home to the world's largest private cloud, which can manage 2.5 petabytes of data every hour! To analyze this humongous amount of data, Walmart has created 'Data Café,' a state-of-the-art analytics hub located within its Bentonville, Arkansas headquarters. The Walmart Labs team heavily invests in building and managing technologies like cloud, data, DevOps , infrastructure, and security.
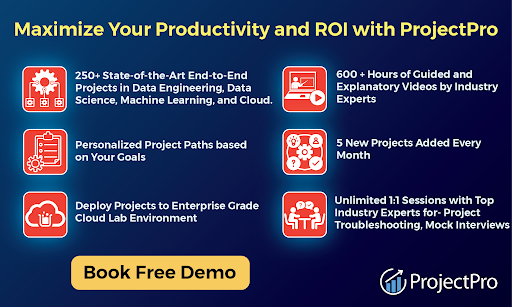
Walmart is experiencing massive digital growth as the world's largest retailer . Walmart has been leveraging Big data and advances in data science to build solutions to enhance, optimize and customize the shopping experience and serve their customers in a better way. At Walmart Labs, data scientists are focused on creating data-driven solutions that power the efficiency and effectiveness of complex supply chain management processes. Here are some of the applications of data science at Walmart:
i) Personalized Customer Shopping Experience
Walmart analyses customer preferences and shopping patterns to optimize the stocking and displaying of merchandise in their stores. Analysis of Big data also helps them understand new item sales, make decisions on discontinuing products, and the performance of brands.
ii) Order Sourcing and On-Time Delivery Promise
Millions of customers view items on Walmart.com, and Walmart provides each customer a real-time estimated delivery date for the items purchased. Walmart runs a backend algorithm that estimates this based on the distance between the customer and the fulfillment center, inventory levels, and shipping methods available. The supply chain management system determines the optimum fulfillment center based on distance and inventory levels for every order. It also has to decide on the shipping method to minimize transportation costs while meeting the promised delivery date.
Here's what valued users are saying about ProjectPro
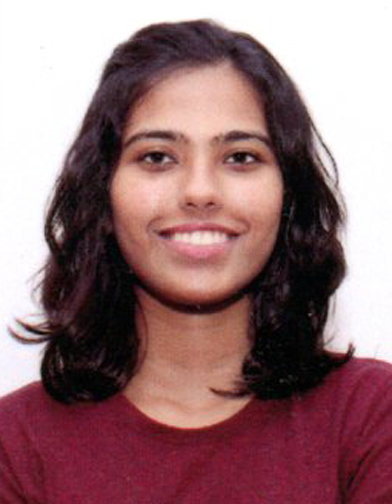
Savvy Sahai
Data Science Intern, Capgemini
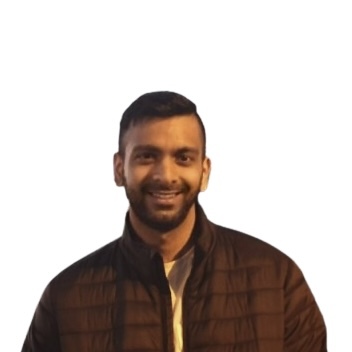
Gautam Vermani
Data Consultant at Confidential
Not sure what you are looking for?
iii) Packing Optimization
Also known as Box recommendation is a daily occurrence in the shipping of items in retail and eCommerce business. When items of an order or multiple orders for the same customer are ready for packing, Walmart has developed a recommender system that picks the best-sized box which holds all the ordered items with the least in-box space wastage within a fixed amount of time. This Bin Packing problem is a classic NP-Hard problem familiar to data scientists .
Whenever items of an order or multiple orders placed by the same customer are picked from the shelf and are ready for packing, the box recommendation system determines the best-sized box to hold all the ordered items with a minimum of in-box space wasted. This problem is known as the Bin Packing Problem, another classic NP-Hard problem familiar to data scientists.
Here is a link to a sales prediction data science case study to help you understand the applications of Data Science in the real world. Walmart Sales Forecasting Project uses historical sales data for 45 Walmart stores located in different regions. Each store contains many departments, and you must build a model to project the sales for each department in each store. This data science case study aims to create a predictive model to predict the sales of each product. You can also try your hands-on Inventory Demand Forecasting Data Science Project to develop a machine learning model to forecast inventory demand accurately based on historical sales data.
Get Closer To Your Dream of Becoming a Data Scientist with 70+ Solved End-to-End ML Projects
Amazon is an American multinational technology-based company based in Seattle, USA. It started as an online bookseller, but today it focuses on eCommerce, cloud computing , digital streaming, and artificial intelligence . It hosts an estimate of 1,000,000,000 gigabytes of data across more than 1,400,000 servers. Through its constant innovation in data science and big data Amazon is always ahead in understanding its customers. Here are a few data analytics case study examples at Amazon:
i) Recommendation Systems
Data science models help amazon understand the customers' needs and recommend them to them before the customer searches for a product; this model uses collaborative filtering. Amazon uses 152 million customer purchases data to help users to decide on products to be purchased. The company generates 35% of its annual sales using the Recommendation based systems (RBS) method.
Here is a Recommender System Project to help you build a recommendation system using collaborative filtering.
ii) Retail Price Optimization
Amazon product prices are optimized based on a predictive model that determines the best price so that the users do not refuse to buy it based on price. The model carefully determines the optimal prices considering the customers' likelihood of purchasing the product and thinks the price will affect the customers' future buying patterns. Price for a product is determined according to your activity on the website, competitors' pricing, product availability, item preferences, order history, expected profit margin, and other factors.
Check Out this Retail Price Optimization Project to build a Dynamic Pricing Model.
iii) Fraud Detection
Being a significant eCommerce business, Amazon remains at high risk of retail fraud. As a preemptive measure, the company collects historical and real-time data for every order. It uses Machine learning algorithms to find transactions with a higher probability of being fraudulent. This proactive measure has helped the company restrict clients with an excessive number of returns of products.
You can look at this Credit Card Fraud Detection Project to implement a fraud detection model to classify fraudulent credit card transactions.
New Projects
Let us explore data analytics case study examples in the entertainment indusry.
Ace Your Next Job Interview with Mock Interviews from Experts to Improve Your Skills and Boost Confidence!
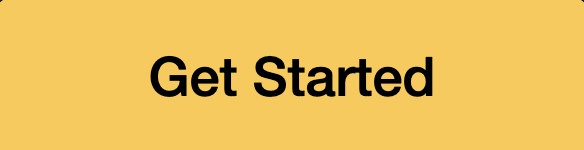
Netflix started as a DVD rental service in 1997 and then has expanded into the streaming business. Headquartered in Los Gatos, California, Netflix is the largest content streaming company in the world. Currently, Netflix has over 208 million paid subscribers worldwide, and with thousands of smart devices which are presently streaming supported, Netflix has around 3 billion hours watched every month. The secret to this massive growth and popularity of Netflix is its advanced use of data analytics and recommendation systems to provide personalized and relevant content recommendations to its users. The data is collected over 100 billion events every day. Here are a few examples of data analysis case studies applied at Netflix :
i) Personalized Recommendation System
Netflix uses over 1300 recommendation clusters based on consumer viewing preferences to provide a personalized experience. Some of the data that Netflix collects from its users include Viewing time, platform searches for keywords, Metadata related to content abandonment, such as content pause time, rewind, rewatched. Using this data, Netflix can predict what a viewer is likely to watch and give a personalized watchlist to a user. Some of the algorithms used by the Netflix recommendation system are Personalized video Ranking, Trending now ranker, and the Continue watching now ranker.
ii) Content Development using Data Analytics
Netflix uses data science to analyze the behavior and patterns of its user to recognize themes and categories that the masses prefer to watch. This data is used to produce shows like The umbrella academy, and Orange Is the New Black, and the Queen's Gambit. These shows seem like a huge risk but are significantly based on data analytics using parameters, which assured Netflix that they would succeed with its audience. Data analytics is helping Netflix come up with content that their viewers want to watch even before they know they want to watch it.
iii) Marketing Analytics for Campaigns
Netflix uses data analytics to find the right time to launch shows and ad campaigns to have maximum impact on the target audience. Marketing analytics helps come up with different trailers and thumbnails for other groups of viewers. For example, the House of Cards Season 5 trailer with a giant American flag was launched during the American presidential elections, as it would resonate well with the audience.
Here is a Customer Segmentation Project using association rule mining to understand the primary grouping of customers based on various parameters.
Get FREE Access to Machine Learning Example Codes for Data Cleaning , Data Munging, and Data Visualization
In a world where Purchasing music is a thing of the past and streaming music is a current trend, Spotify has emerged as one of the most popular streaming platforms. With 320 million monthly users, around 4 billion playlists, and approximately 2 million podcasts, Spotify leads the pack among well-known streaming platforms like Apple Music, Wynk, Songza, amazon music, etc. The success of Spotify has mainly depended on data analytics. By analyzing massive volumes of listener data, Spotify provides real-time and personalized services to its listeners. Most of Spotify's revenue comes from paid premium subscriptions. Here are some of the examples of case study on data analytics used by Spotify to provide enhanced services to its listeners:
i) Personalization of Content using Recommendation Systems
Spotify uses Bart or Bayesian Additive Regression Trees to generate music recommendations to its listeners in real-time. Bart ignores any song a user listens to for less than 30 seconds. The model is retrained every day to provide updated recommendations. A new Patent granted to Spotify for an AI application is used to identify a user's musical tastes based on audio signals, gender, age, accent to make better music recommendations.
Spotify creates daily playlists for its listeners, based on the taste profiles called 'Daily Mixes,' which have songs the user has added to their playlists or created by the artists that the user has included in their playlists. It also includes new artists and songs that the user might be unfamiliar with but might improve the playlist. Similar to it is the weekly 'Release Radar' playlists that have newly released artists' songs that the listener follows or has liked before.
ii) Targetted marketing through Customer Segmentation
With user data for enhancing personalized song recommendations, Spotify uses this massive dataset for targeted ad campaigns and personalized service recommendations for its users. Spotify uses ML models to analyze the listener's behavior and group them based on music preferences, age, gender, ethnicity, etc. These insights help them create ad campaigns for a specific target audience. One of their well-known ad campaigns was the meme-inspired ads for potential target customers, which was a huge success globally.
iii) CNN's for Classification of Songs and Audio Tracks
Spotify builds audio models to evaluate the songs and tracks, which helps develop better playlists and recommendations for its users. These allow Spotify to filter new tracks based on their lyrics and rhythms and recommend them to users like similar tracks ( collaborative filtering). Spotify also uses NLP ( Natural language processing) to scan articles and blogs to analyze the words used to describe songs and artists. These analytical insights can help group and identify similar artists and songs and leverage them to build playlists.
Here is a Music Recommender System Project for you to start learning. We have listed another music recommendations dataset for you to use for your projects: Dataset1 . You can use this dataset of Spotify metadata to classify songs based on artists, mood, liveliness. Plot histograms, heatmaps to get a better understanding of the dataset. Use classification algorithms like logistic regression, SVM, and Principal component analysis to generate valuable insights from the dataset.
Explore Categories
Below you will find case studies for data analytics in the travel and tourism industry.
Airbnb was born in 2007 in San Francisco and has since grown to 4 million Hosts and 5.6 million listings worldwide who have welcomed more than 1 billion guest arrivals in almost every country across the globe. Airbnb is active in every country on the planet except for Iran, Sudan, Syria, and North Korea. That is around 97.95% of the world. Using data as a voice of their customers, Airbnb uses the large volume of customer reviews, host inputs to understand trends across communities, rate user experiences, and uses these analytics to make informed decisions to build a better business model. The data scientists at Airbnb are developing exciting new solutions to boost the business and find the best mapping for its customers and hosts. Airbnb data servers serve approximately 10 million requests a day and process around one million search queries. Data is the voice of customers at AirBnB and offers personalized services by creating a perfect match between the guests and hosts for a supreme customer experience.
i) Recommendation Systems and Search Ranking Algorithms
Airbnb helps people find 'local experiences' in a place with the help of search algorithms that make searches and listings precise. Airbnb uses a 'listing quality score' to find homes based on the proximity to the searched location and uses previous guest reviews. Airbnb uses deep neural networks to build models that take the guest's earlier stays into account and area information to find a perfect match. The search algorithms are optimized based on guest and host preferences, rankings, pricing, and availability to understand users’ needs and provide the best match possible.
ii) Natural Language Processing for Review Analysis
Airbnb characterizes data as the voice of its customers. The customer and host reviews give a direct insight into the experience. The star ratings alone cannot be an excellent way to understand it quantitatively. Hence Airbnb uses natural language processing to understand reviews and the sentiments behind them. The NLP models are developed using Convolutional neural networks .
Practice this Sentiment Analysis Project for analyzing product reviews to understand the basic concepts of natural language processing.
iii) Smart Pricing using Predictive Analytics
The Airbnb hosts community uses the service as a supplementary income. The vacation homes and guest houses rented to customers provide for rising local community earnings as Airbnb guests stay 2.4 times longer and spend approximately 2.3 times the money compared to a hotel guest. The profits are a significant positive impact on the local neighborhood community. Airbnb uses predictive analytics to predict the prices of the listings and help the hosts set a competitive and optimal price. The overall profitability of the Airbnb host depends on factors like the time invested by the host and responsiveness to changing demands for different seasons. The factors that impact the real-time smart pricing are the location of the listing, proximity to transport options, season, and amenities available in the neighborhood of the listing.
Here is a Price Prediction Project to help you understand the concept of predictive analysis which is widely common in case studies for data analytics.
Uber is the biggest global taxi service provider. As of December 2018, Uber has 91 million monthly active consumers and 3.8 million drivers. Uber completes 14 million trips each day. Uber uses data analytics and big data-driven technologies to optimize their business processes and provide enhanced customer service. The Data Science team at uber has been exploring futuristic technologies to provide better service constantly. Machine learning and data analytics help Uber make data-driven decisions that enable benefits like ride-sharing, dynamic price surges, better customer support, and demand forecasting. Here are some of the real world data science projects used by uber:
i) Dynamic Pricing for Price Surges and Demand Forecasting
Uber prices change at peak hours based on demand. Uber uses surge pricing to encourage more cab drivers to sign up with the company, to meet the demand from the passengers. When the prices increase, the driver and the passenger are both informed about the surge in price. Uber uses a predictive model for price surging called the 'Geosurge' ( patented). It is based on the demand for the ride and the location.
ii) One-Click Chat
Uber has developed a Machine learning and natural language processing solution called one-click chat or OCC for coordination between drivers and users. This feature anticipates responses for commonly asked questions, making it easy for the drivers to respond to customer messages. Drivers can reply with the clock of just one button. One-Click chat is developed on Uber's machine learning platform Michelangelo to perform NLP on rider chat messages and generate appropriate responses to them.
iii) Customer Retention
Failure to meet the customer demand for cabs could lead to users opting for other services. Uber uses machine learning models to bridge this demand-supply gap. By using prediction models to predict the demand in any location, uber retains its customers. Uber also uses a tier-based reward system, which segments customers into different levels based on usage. The higher level the user achieves, the better are the perks. Uber also provides personalized destination suggestions based on the history of the user and their frequently traveled destinations.
You can take a look at this Python Chatbot Project and build a simple chatbot application to understand better the techniques used for natural language processing. You can also practice the working of a demand forecasting model with this project using time series analysis. You can look at this project which uses time series forecasting and clustering on a dataset containing geospatial data for forecasting customer demand for ola rides.
Explore More Data Science and Machine Learning Projects for Practice. Fast-Track Your Career Transition with ProjectPro
7) LinkedIn
LinkedIn is the largest professional social networking site with nearly 800 million members in more than 200 countries worldwide. Almost 40% of the users access LinkedIn daily, clocking around 1 billion interactions per month. The data science team at LinkedIn works with this massive pool of data to generate insights to build strategies, apply algorithms and statistical inferences to optimize engineering solutions, and help the company achieve its goals. Here are some of the real world data science projects at LinkedIn:
i) LinkedIn Recruiter Implement Search Algorithms and Recommendation Systems
LinkedIn Recruiter helps recruiters build and manage a talent pool to optimize the chances of hiring candidates successfully. This sophisticated product works on search and recommendation engines. The LinkedIn recruiter handles complex queries and filters on a constantly growing large dataset. The results delivered have to be relevant and specific. The initial search model was based on linear regression but was eventually upgraded to Gradient Boosted decision trees to include non-linear correlations in the dataset. In addition to these models, the LinkedIn recruiter also uses the Generalized Linear Mix model to improve the results of prediction problems to give personalized results.
ii) Recommendation Systems Personalized for News Feed
The LinkedIn news feed is the heart and soul of the professional community. A member's newsfeed is a place to discover conversations among connections, career news, posts, suggestions, photos, and videos. Every time a member visits LinkedIn, machine learning algorithms identify the best exchanges to be displayed on the feed by sorting through posts and ranking the most relevant results on top. The algorithms help LinkedIn understand member preferences and help provide personalized news feeds. The algorithms used include logistic regression, gradient boosted decision trees and neural networks for recommendation systems.
iii) CNN's to Detect Inappropriate Content
To provide a professional space where people can trust and express themselves professionally in a safe community has been a critical goal at LinkedIn. LinkedIn has heavily invested in building solutions to detect fake accounts and abusive behavior on their platform. Any form of spam, harassment, inappropriate content is immediately flagged and taken down. These can range from profanity to advertisements for illegal services. LinkedIn uses a Convolutional neural networks based machine learning model. This classifier trains on a training dataset containing accounts labeled as either "inappropriate" or "appropriate." The inappropriate list consists of accounts having content from "blocklisted" phrases or words and a small portion of manually reviewed accounts reported by the user community.
Here is a Text Classification Project to help you understand NLP basics for text classification. You can find a news recommendation system dataset to help you build a personalized news recommender system. You can also use this dataset to build a classifier using logistic regression, Naive Bayes, or Neural networks to classify toxic comments.
Get confident to build end-to-end projects
Access to a curated library of 250+ end-to-end industry projects with solution code, videos and tech support.
Pfizer is a multinational pharmaceutical company headquartered in New York, USA. One of the largest pharmaceutical companies globally known for developing a wide range of medicines and vaccines in disciplines like immunology, oncology, cardiology, and neurology. Pfizer became a household name in 2010 when it was the first to have a COVID-19 vaccine with FDA. In early November 2021, The CDC has approved the Pfizer vaccine for kids aged 5 to 11. Pfizer has been using machine learning and artificial intelligence to develop drugs and streamline trials, which played a massive role in developing and deploying the COVID-19 vaccine. Here are a few data analytics case studies by Pfizer :
i) Identifying Patients for Clinical Trials
Artificial intelligence and machine learning are used to streamline and optimize clinical trials to increase their efficiency. Natural language processing and exploratory data analysis of patient records can help identify suitable patients for clinical trials. These can help identify patients with distinct symptoms. These can help examine interactions of potential trial members' specific biomarkers, predict drug interactions and side effects which can help avoid complications. Pfizer's AI implementation helped rapidly identify signals within the noise of millions of data points across their 44,000-candidate COVID-19 clinical trial.
ii) Supply Chain and Manufacturing
Data science and machine learning techniques help pharmaceutical companies better forecast demand for vaccines and drugs and distribute them efficiently. Machine learning models can help identify efficient supply systems by automating and optimizing the production steps. These will help supply drugs customized to small pools of patients in specific gene pools. Pfizer uses Machine learning to predict the maintenance cost of equipment used. Predictive maintenance using AI is the next big step for Pharmaceutical companies to reduce costs.
iii) Drug Development
Computer simulations of proteins, and tests of their interactions, and yield analysis help researchers develop and test drugs more efficiently. In 2016 Watson Health and Pfizer announced a collaboration to utilize IBM Watson for Drug Discovery to help accelerate Pfizer's research in immuno-oncology, an approach to cancer treatment that uses the body's immune system to help fight cancer. Deep learning models have been used recently for bioactivity and synthesis prediction for drugs and vaccines in addition to molecular design. Deep learning has been a revolutionary technique for drug discovery as it factors everything from new applications of medications to possible toxic reactions which can save millions in drug trials.
You can create a Machine learning model to predict molecular activity to help design medicine using this dataset . You may build a CNN or a Deep neural network for this data analyst case study project.
Access Data Science and Machine Learning Project Code Examples
9) Shell Data Analyst Case Study Project
Shell is a global group of energy and petrochemical companies with over 80,000 employees in around 70 countries. Shell uses advanced technologies and innovations to help build a sustainable energy future. Shell is going through a significant transition as the world needs more and cleaner energy solutions to be a clean energy company by 2050. It requires substantial changes in the way in which energy is used. Digital technologies, including AI and Machine Learning, play an essential role in this transformation. These include efficient exploration and energy production, more reliable manufacturing, more nimble trading, and a personalized customer experience. Using AI in various phases of the organization will help achieve this goal and stay competitive in the market. Here are a few data analytics case studies in the petrochemical industry:
i) Precision Drilling
Shell is involved in the processing mining oil and gas supply, ranging from mining hydrocarbons to refining the fuel to retailing them to customers. Recently Shell has included reinforcement learning to control the drilling equipment used in mining. Reinforcement learning works on a reward-based system based on the outcome of the AI model. The algorithm is designed to guide the drills as they move through the surface, based on the historical data from drilling records. It includes information such as the size of drill bits, temperatures, pressures, and knowledge of the seismic activity. This model helps the human operator understand the environment better, leading to better and faster results will minor damage to machinery used.
ii) Efficient Charging Terminals
Due to climate changes, governments have encouraged people to switch to electric vehicles to reduce carbon dioxide emissions. However, the lack of public charging terminals has deterred people from switching to electric cars. Shell uses AI to monitor and predict the demand for terminals to provide efficient supply. Multiple vehicles charging from a single terminal may create a considerable grid load, and predictions on demand can help make this process more efficient.
iii) Monitoring Service and Charging Stations
Another Shell initiative trialed in Thailand and Singapore is the use of computer vision cameras, which can think and understand to watch out for potentially hazardous activities like lighting cigarettes in the vicinity of the pumps while refueling. The model is built to process the content of the captured images and label and classify it. The algorithm can then alert the staff and hence reduce the risk of fires. You can further train the model to detect rash driving or thefts in the future.
Here is a project to help you understand multiclass image classification. You can use the Hourly Energy Consumption Dataset to build an energy consumption prediction model. You can use time series with XGBoost to develop your model.
10) Zomato Case Study on Data Analytics
Zomato was founded in 2010 and is currently one of the most well-known food tech companies. Zomato offers services like restaurant discovery, home delivery, online table reservation, online payments for dining, etc. Zomato partners with restaurants to provide tools to acquire more customers while also providing delivery services and easy procurement of ingredients and kitchen supplies. Currently, Zomato has over 2 lakh restaurant partners and around 1 lakh delivery partners. Zomato has closed over ten crore delivery orders as of date. Zomato uses ML and AI to boost their business growth, with the massive amount of data collected over the years from food orders and user consumption patterns. Here are a few examples of data analyst case study project developed by the data scientists at Zomato:
i) Personalized Recommendation System for Homepage
Zomato uses data analytics to create personalized homepages for its users. Zomato uses data science to provide order personalization, like giving recommendations to the customers for specific cuisines, locations, prices, brands, etc. Restaurant recommendations are made based on a customer's past purchases, browsing history, and what other similar customers in the vicinity are ordering. This personalized recommendation system has led to a 15% improvement in order conversions and click-through rates for Zomato.
You can use the Restaurant Recommendation Dataset to build a restaurant recommendation system to predict what restaurants customers are most likely to order from, given the customer location, restaurant information, and customer order history.
ii) Analyzing Customer Sentiment
Zomato uses Natural language processing and Machine learning to understand customer sentiments using social media posts and customer reviews. These help the company gauge the inclination of its customer base towards the brand. Deep learning models analyze the sentiments of various brand mentions on social networking sites like Twitter, Instagram, Linked In, and Facebook. These analytics give insights to the company, which helps build the brand and understand the target audience.
iii) Predicting Food Preparation Time (FPT)
Food delivery time is an essential variable in the estimated delivery time of the order placed by the customer using Zomato. The food preparation time depends on numerous factors like the number of dishes ordered, time of the day, footfall in the restaurant, day of the week, etc. Accurate prediction of the food preparation time can help make a better prediction of the Estimated delivery time, which will help delivery partners less likely to breach it. Zomato uses a Bidirectional LSTM-based deep learning model that considers all these features and provides food preparation time for each order in real-time.
Data scientists are companies' secret weapons when analyzing customer sentiments and behavior and leveraging it to drive conversion, loyalty, and profits. These 10 data science case studies projects with examples and solutions show you how various organizations use data science technologies to succeed and be at the top of their field! To summarize, Data Science has not only accelerated the performance of companies but has also made it possible to manage & sustain their performance with ease.
FAQs on Data Analysis Case Studies
A case study in data science is an in-depth analysis of a real-world problem using data-driven approaches. It involves collecting, cleaning, and analyzing data to extract insights and solve challenges, offering practical insights into how data science techniques can address complex issues across various industries.
To create a data science case study, identify a relevant problem, define objectives, and gather suitable data. Clean and preprocess data, perform exploratory data analysis, and apply appropriate algorithms for analysis. Summarize findings, visualize results, and provide actionable recommendations, showcasing the problem-solving potential of data science techniques.
|
|
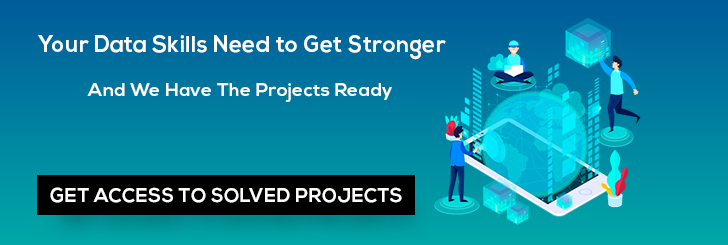
About the Author

ProjectPro is the only online platform designed to help professionals gain practical, hands-on experience in big data, data engineering, data science, and machine learning related technologies. Having over 270+ reusable project templates in data science and big data with step-by-step walkthroughs,

© 2024
© 2024 Iconiq Inc.
Privacy policy
User policy
Write for ProjectPro
- Marketing Analytics Case Studies
- Social Media Management
- Social Media Marketing
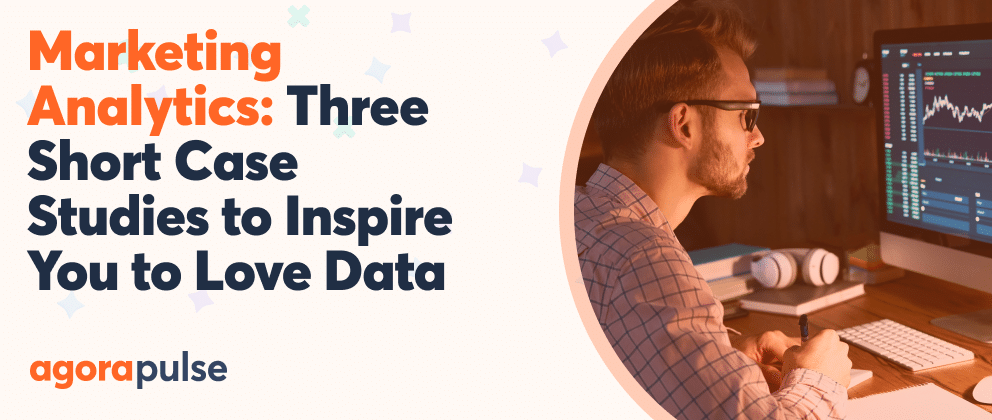
Three Short Marketing Analytics Case Studies to Inspire You to Love Data
Written by Anna Sonnenberg
Published Feb. 28 2022

Table of Contents
From engagement statistics to content analytics to conversion metrics, data is a big part of most social media managers’ responsibilities. But that doesn’t necessarily mean you enjoy processing marketing data or drawing conclusions from it.
If data isn’t exactly your favorite part of the job, these marketing analytics case studies may change your mind.
Find out how marketing analytics helped three major brands grow their businesses—and you might develop a whole new appreciation for marketing data in the process.
What Is Marketing Analytics?
Marketing analytics is the process of collecting and evaluating metrics to understand how much value marketing efforts generate. With analytics, you can assess the return on investment (ROI) of anything from social media posts and ad campaigns to landing pages and native platform features.
For many organizations and their marketing team, marketing analytics are essential for improving offerings and driving growth.
Here are common goals you can achieve with marketing analytics.
Improving marketing campaigns
Some social media marketing campaigns are more successful than others. Analytics can help your organization pinpoint exactly what works. By analyzing metrics like engagement, click-through rate (CTR), conversions, and ROI, you can determine what resonates best with its audience. By using data science, you can craft a marketing strategy that gets you better results from your campaigns.
Decreasing expenses
Ineffective marketing campaigns, usability issues, and poorly optimized algorithms can all lead to dissatisfied customers and unnecessarily high retention costs.
By investing in marketing analytics, your organization can take steps to identify points of friction and reduce expenses.
Forecasting results
Reviewing past outcomes is useful, but forecasting the results your campaigns are likely to generate is even more valuable. With marketing analytics, you can model results and get a better sense of how marketing initiatives can impact growth over time.
Marketing Analytics Case Studies: Progressive Insurance
In the early 2000s, Progressive’s website was routinely considered one of the best in the insurance industry. When the insurance provider’s customers began switching to mobile devices a decade later, the organization aimed to develop a mobile app as effective as its desktop site.
But what did that mean exactly? And what was the insurance provider’s mobile app missing?
To determine what would make the mobile app more successful, Progressive pursued an in-depth analysis of the organization’s marketing data.
As Progressive Data & Analytics Business Leader Pawan Divakarla explains , the insurance provider’s analytics team has always sought insight into how customers are using the company’s tools.
In his words, “At Progressive, we sell insurance. But if you think about it, our product is actually data.”
After launching the mobile app, Progressive began looking for ways to optimize the user experience. As this Progressive case study explains, the organization aimed to streamline the login process and improve user satisfaction to meet its ultimate goals of increasing customer loyalty and new customer acquisition.
Because Progressive’s mobile app generated so much information, the organization needed data visualization tools for collection and processing. To analyze customers’ experiences and actions, the company opted to use a combination of Google Analytics 360 and Google Tag Manager 360.
This choice was a relatively simple one for Progressive because the company already used these tools to run A/B tests and optimize its website.
Using Google’s analytical tools to review the company’s mobile app would allow Progressive to understand what features to test and how to optimize the user experience across countless mobile devices and operating systems.
Progressive used the two Google tools for separate yet complementary functions:
- With Google Analytics 360, Progressive could track user sessions and demographics. The company integrated BigQuery for more insight into user behaviors.
- With Google Tag Manager 360, Progressive could easily implement tracking tags to measure various actions, conversions, and navigation patterns.
To get the insights the company needed to improve its mobile app, Progressive took a three-pronged approach:
User device data
First, Progressive aimed to identify which devices and operating systems were most common among the app’s user base. With this information, the company would be able to develop more effective tests for its mobile app.
App crash data
Next, Progressive wanted to analyze app crash data. The company planned to use Google Analytics 360 and BigQuery data to understand the cause for the crash and how users reacted when the app stopped working abruptly.
Login and security data
Finally, Progressive aimed to learn how users responded when failed login attempts locked them out of the app. The company planned to use Google Analytics 360 and BigQuery to see what actions users took. It planned to then test new prompts that would guide users more effectively.
Outcome of this marketing analytics case study
Using marketing analytics tools , Progressive was able to process customer behavior, identify appropriate tests, and implement successful solutions.
Here’s how each of the three approaches generated useful results that helped Progressive reach its ultimate acquisition and loyalty goals.
First, Progressive developed session-based reports that reflected the most common mobile devices and operating systems for the app’s user base. With those insights, the company identified which device and operating system combinations to prioritize for its mobile app tests.
As a result, the company reduced testing time by 20% for its mobile app—allowing the organization to find solutions much more quickly than its typical timeline would have allowed.
Next, Progressive reviewed the actions customers took right before the app crashed. The company pinpointed a server issue as the cause of a major crash that disrupted countless mobile app sessions.
Using this data, Progressive could address the server issue and prevent it from happening again.
Finally, Progressive created a custom funnel in Google Analytics 360 to evaluate users’ typical login path. After learning that many users who became locked out of their accounts never attempted to log in again, the company developed a workflow that provided better guidance.
The new workflow sends users to a Forgot Password page, which has increased logins by 30%.
Marketing Analytics Case Studies: Netflix
When companies take a digital-first approach to customer loyalty, they can collect an incredible amount of user data. With these marketing analytics, companies can improve their products, build better marketing campaigns, and drive more revenue.
As this Netflix case study shows, the online content streaming platform has leveraged its user data in a variety of helpful ways.
By using data to improve its content recommendation engine, develop original content, and increase its customer retention rate, Netflix has positioned itself far ahead of the competition.
With so much data to leverage, Netflix had wide-ranging goals for the company’s marketing analytics. However, all of the organization’s goals contributed to the company’s larger business objectives—which focus on customer retention.
Netflix aimed to go beyond basic user demographics and understand what customers want from a streaming platform—and what was likely to convince them to stay. With this knowledge, Netflix could create better products and services for happier customers.
Access issues, service outages, and platform flaws can all lead to unhappy customers and negative sentiment—which can cause customers to seek out an alternative solution.
By identifying problems early through marketing analytics, Netflix could improve its products and continue to innovate.
To work toward its customer retention objective, Netflix collected data from virtually every interaction with its 150+ million subscribers. The company then used marketing analytics tools to process this native data and evaluate everything from how customers navigate the platform to what they watch.
By creating such detailed customer profiles, Netflix could make much more personalized recommendations for each user. The more data the company collected, the more it could tailor its algorithm to suggest the ideal content to each individual viewer.
To better understand the platform’s users, Netflix collected such data as:
- The devices viewers used to stream content
- Day of week and time of day when users viewed content
- Number of serial episodes viewers watched in a row
- Whether viewers paused and resumed content
- Number and type of searches users performed
Netflix also welcomed user feedback on content . The company incorporated these content ratings into their analysis to better understand viewer preferences.
According to the streaming platform, the Netflix algorithm is responsible for about 80% of viewer activity . The company has successfully collected relevant data and used marketing analytics to generate recommendations that encourage viewers to continue watching and subscribing.
The revenue metrics suggest that Netflix’s focus on marketing analytics has been hugely beneficial to the company. The company estimates that its algorithm generates $1 billion in value every year, largely due to customer retention.
In recent years, Netflix’s customer retention rate has far surpassed competitors like Hulu and Amazon Prime. Netflix has an impressive 90% retention rate , meaning the vast majority of viewers continue to subscribe to the service month after month. (In contrast, Amazon Prime’s retention rate is 75%, and Hulu’s is 64%.)
For Netflix, customer retention means more than happy viewers. It also means more data, a continually improving algorithm, and substantial business growth.
Netflix has emerged as the world’s most highly valued company, with a total valuation of over $160 billion. Netflix can continue to increase this valuation. It leverages its data by producing original media and recommending the ideal content to viewers every time they access the streaming platform.
Marketing Analytics Case Studies: Allrecipes
As the world’s biggest digital food brand, Allrecipes has 18 websites and more than 85 million users. But the brand also has plenty of competition from other food-focused apps and websites.
To stay ahead of other recipe sites and ensure that it continues to provide all the solutions that users want, Allrecipes relies on marketing analytics.
With marketing analytics, the digital brand can better understand the customer journey and analyze trends as they emerge. As this Allrecipes case study explains, the brand can expand its audience and attract even more lucrative demographics using these insights.
To continue to gain ground as the world’s top digital food brand, Allrecipes established several wide-ranging goals.
Some of the brand’s primary objectives included the following.
Improve user experience
With more than a billion and a half visitors across the brand’s sites every year, Allrecipes generates a ton of traffic. But the company needed a way to understand how visitors were using the site, so it could improve the user experience and gauge the health of the sites.
Increase video engagement
To take advantage of a demand for video content, Allrecipes had decided to invest heavily in video. However, the video production team needed strategic guidance. The brand needed to know what types of content would drive the most engagement.
Drive mobile engagement
To continue to meet the needs of its user base, Allrecipes had to look beyond its websites. As more and more people began using mobile devices to access the brand’s content, Allrecipes realized that the company needed to optimize its mobile app.
Inform product strategy
To promote new features and integrations or pursue partner programs, Allrecipes needed to know what its community wanted. Had they adopted the new integrations yet? Did they need new features to use the site or app more effectively?
Expand user base
Cooking and dining trends come and go, and Allrecipes needed a simple yet effective way to identify these developments.
By responding quickly to trends, the brand would be able to capture a larger user base, including elusive millennials.
Grow advertising revenue
Like many digital brands, Allrecipes has a native advertising program that allows the company to monetize its website. The company aimed to increase its advertising revenue, yet the organization didn’t want to compromise the user experience. To find the right partners to grow this program, Allrecipes needed deeper insights into its audience.
Although the brand’s goals were varied, the approach was relatively straightforward. To process marketing analytics from a wide range of channels, the brand opted to use Tableau, a business intelligence platform.
With Tableau, Allrecipes could establish a single platform for visualizing data from Adobe Marketing Cloud, Hitwise, and comScore. By linking Adobe Marketing Cloud to Tableau, the brand could pull in all of its website and marketing analytics. By linking Hitwise and comScore, the brand could source demographic data.
Using Tableau allowed Allrecipes to build custom dashboards and develop tailored reports to answer all of the brand’s questions. This tool also allowed the brand to pursue collaboration options across the organization.
In fact, departments ranging from marketing and design to product and finance contributed to the tool. Teams used Tableau Server to publish dashboards, creating a single space where stakeholders could visualize or analyze data.
With Tableau, Allrecipes was able to visualize the brand’s data successfully, enabling smarter decisions and making progress toward key goals. Here’s what the brand accomplished using marketing analytics:
Using insights from Tableau, Allrecipes was able to see how visitors typically used the site—including how they submit recipes, share content, and post links on social media channels. The organization then used this data to devise a plan for improving the site.
Knowing how visitors were already engaging with the site allowed the brand to make data-driven, goal-focused decisions.
With Tableau’s marketing analytics, Allrecipes found that out of all types of recipes, dessert typically generated more views and attracted more comments and photos. As a result, the brand opted to focus on this highly engaging niche, creating a separate video hub for dessert recipes.
To increase engagement on mobile devices, Allrecipes devised an A/B test that displayed the brand’s mobile site on all devices. Then the organization used the analytics to identify what drove interactions on mobile. The brand then used insights to improve the mobile site, including optimizing content and encouraging photo uploads.
Tableau’s data visualizations helped Allrecipes understand trends in its user community and respond to preferences more efficiently. Using these insights, the brand was able to promote integrations and features while gathering data for future product enhancements.
By using Tableau’s insights to process trends, Allrecipes was able to segment audiences for various recipe types, ultimately identifying millennial users’ interests and preferences. The brand was then able to create more content geared toward this growing user base—likely responding much more quickly than competitors could.
By tapping into real-time marketing analytics, Allrecipes was able to share popular recipe searches and trending content with its advertising partners during a recent holiday season. Advertisers could then create ads tailored to these interests, generating a better ROI and creating a more appealing experience for users.
What We Learned From These Marketing Analytics Case Studies
As these marketing analytics case studies show, data can tell you a lot about what your customers want—and where your organization succeeds or has room for improvement. Using insights from marketing analytics, a digital marketer can make data-driven decisions to cultivate customer loyalty, generate more revenue, and ultimately grow your business.
Get started on saving time and energy on your own social media management! Check out our free trial of Agorapulse to help you schedule, track, and measure all your social media efforts.
Keep up to date with social media marketing!
Our newsletter is packed with the hottest posts and latest news in social media.
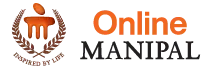
UGC-entitled
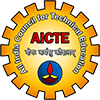
AICTE approved
Admissions open for online degrees from top Manipal universities
Manipal universities prove it again.
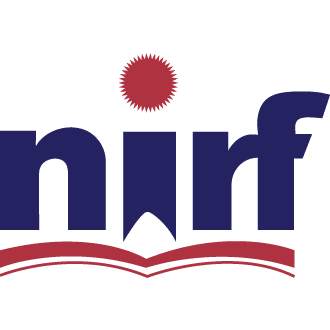
AMONGST ALL UNIVERSITIES
Also, No. 1 Ranked Private University in India
Also, No. 1 Ranked State Pvt. University in Rajasthan
Get more than just a degree!
Complimentary Access To 10K+ Courses & Certifications From Top Global Universities
A 70-year legacy of educational excellence, with 70K+ online learners from 60+ nationalities
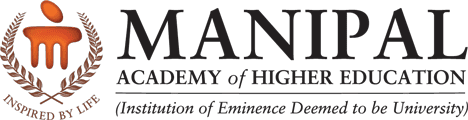
Manipal Academy of Higher Education (MAHE)
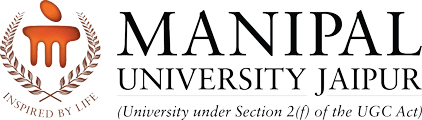
Manipal University Jaipur (MUJ)
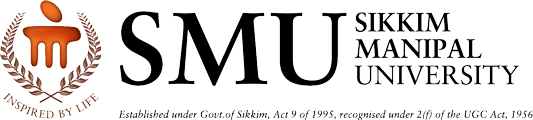
Sikkim Manipal University (SMU)
Explore our online degrees & certifications
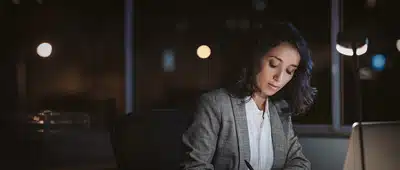
Master of Business Administration

Bachelor of Computer Applications
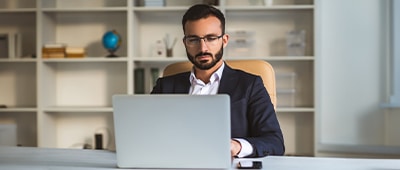
PGCP in Entrepreneurship & Innovation
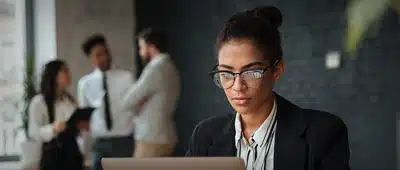
Bachelor of Arts
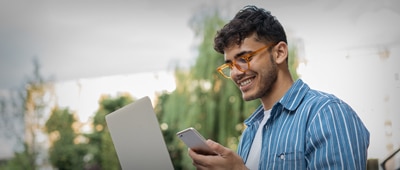
Bachelor of Business Administration
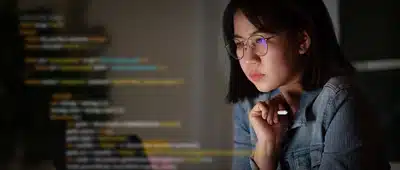
Master of Computer Applications
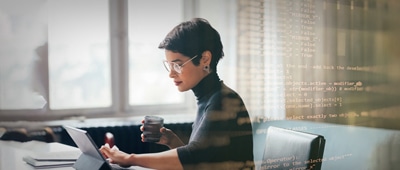
Bachelor of Commerce
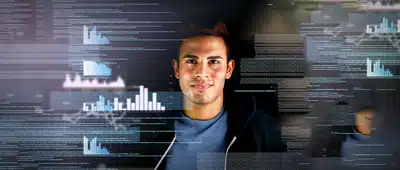
M.Sc. in Data Science
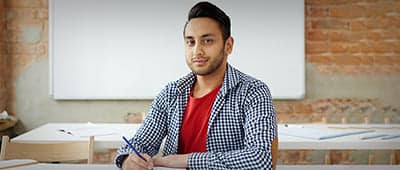
Master of Arts in English
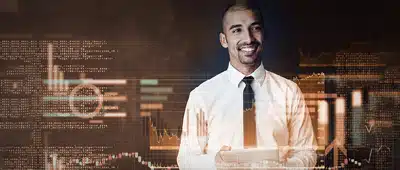
M.Sc. in Business Analytics
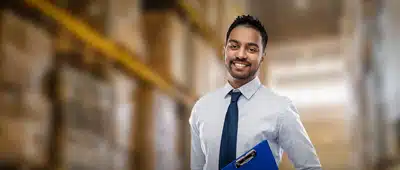
PGCP in Logistics and Supply Chain Management
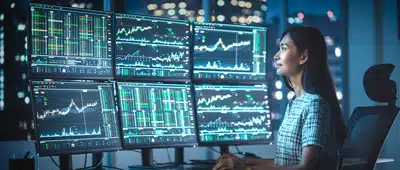
PGCP in Business Analytics
Online manipal benefits.
Graduate with UGC-entitled & AICTE approved online degrees which are at par with on-campus degree programs.
Avail scholarship benefits under merit, defense, Divyaang, alumni, and other categories.
Become a part of a 1,50,000+ global Manipal alumni network.
Improved affordability with flexible financing and no-cost EMI options.
Boost your employability quotient by acquiring job-ready skills & preparing for interviews to perform well in placement drives.
Stay updated on key industry trends through our weekly webinars by industry experts.
Free access to 10K+ courses & professional certifications from top global universities.
Participate in exclusive in-person meetups at campus with your batchmates and faculty.
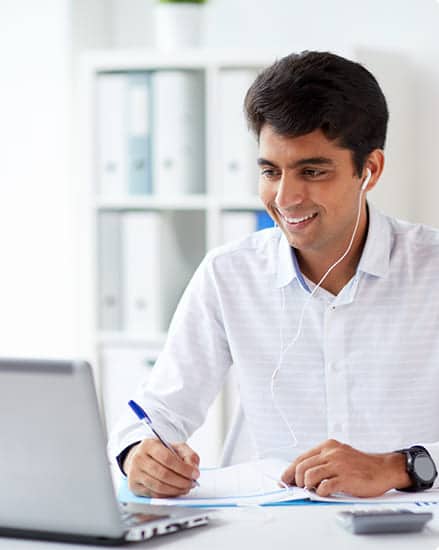
Note: The above offerings may differ based on university & program
Career support services
Our experienced team helps you choose the right career path that aligns with your goals, interests, and skills by providing you valuable guidance and support.
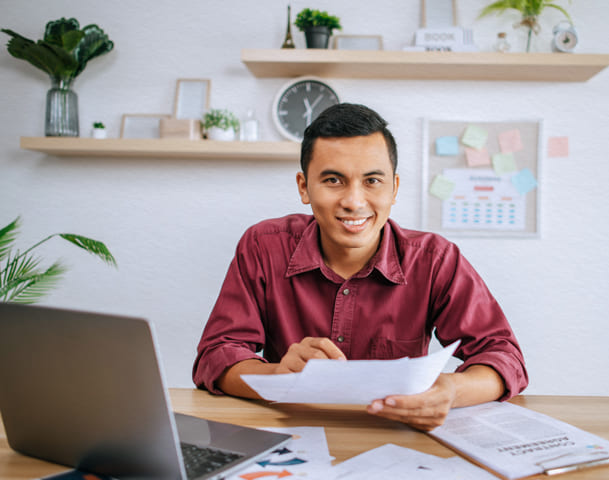
Alumni interactions during & after program
Interact and receive first-hand information & guidance from alumni during and after the program..
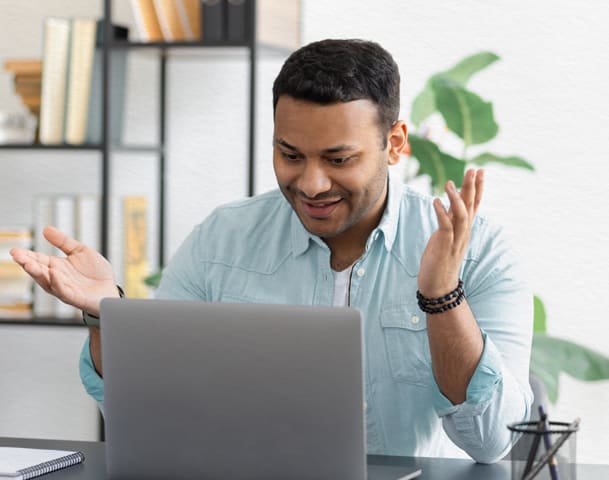
Career advisory & counselling by industry experts
Make informed decisions while choosing your career path by gaining valuable insights on various career opportunities from our expert career counsellors..
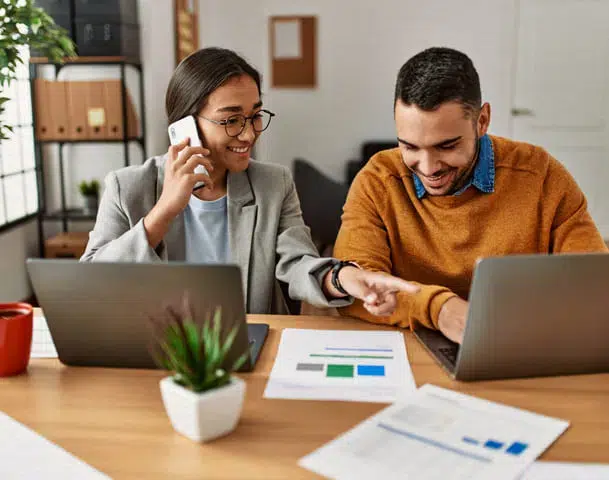
Industry-readiness sessions
Familiarize yourself with industry trends, organizational expectations, and recruiter behavior to develop relevant skills and become job ready..
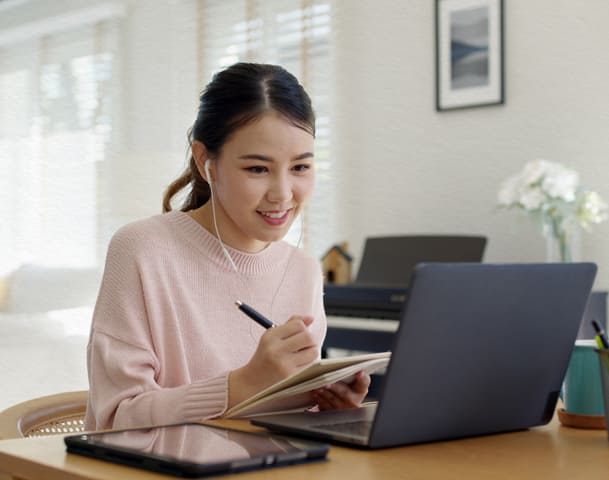
Employability skill assessment & enhancement
Identify your strengths & weaknesses through skill assessments and build competencies to improve your employability quotient., student speak.
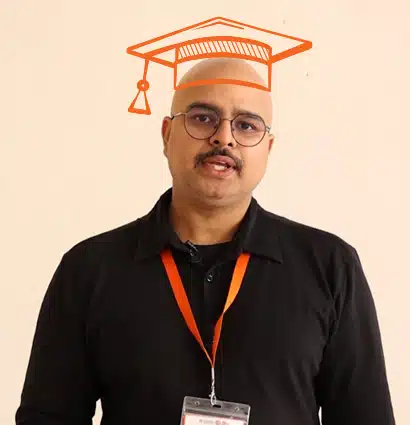
I always wanted to pursue my higher education dream without quitting my job, and MUJ has made it possible for me through their online degrees. My online MCA degree has given me wings to fly and chase my career aspirations.
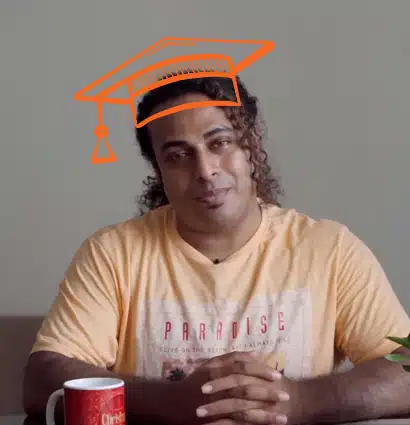
With one year of work experience in a hospital, I wanted to hone my managerial skills. So, I decided to pursue an online MBA in Healthcare Management. Since I’m also preparing for UPSC, pursuing an online MBA is the perfect choice and Online Manipal is playing a key role in enhancing my knowledge.
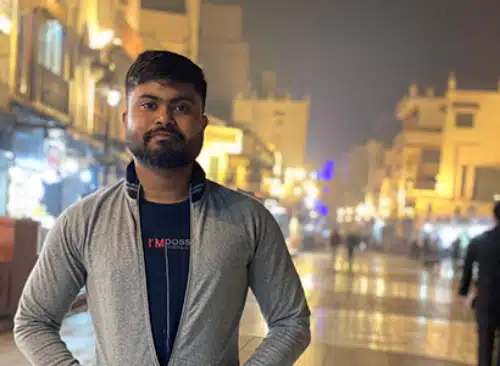
I wanted to specialize in marketing, which is why I decided to start by pursuing an online BBA. As a working professional, an online degree was the best choice for me. The faculty at MUJ are experienced & guide us well and the student portal is user-friendly.
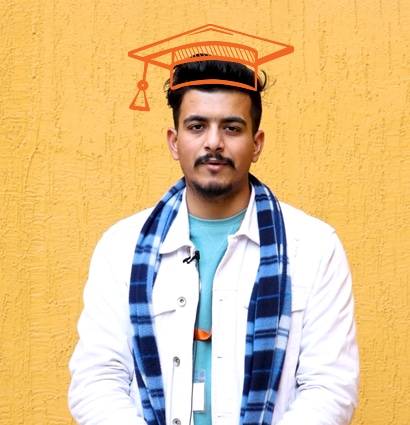
I have 2 years of work experience in IT as an Application Engineer. Through this program, I hope to expand my knowledge in business analytics and apply it to my current job role. Online Manipal has enabled me to learn at my convenience and the free access to Coursera content has helped me gain industry-relevant skills.
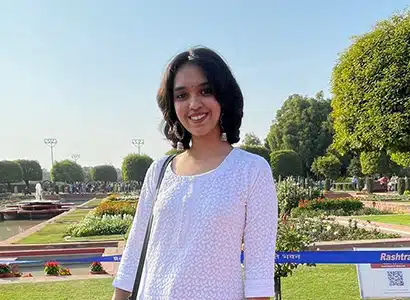
Having completed my master’s in business, I wanted to switch to the in-demand domain of business analytics, and I found MAHE’s certification program to be one of the best picks for me. The best part about this online certification program is that I can study at my own pace.
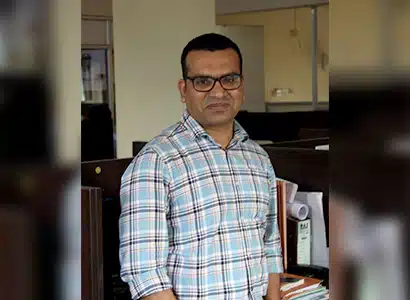
With 12 years of work experience in procurement and supply chain, I wanted to upskill in this domain. The curriculum of the online PGCP program by MAHE is industry-relevant and is helping me in applying my skills on the job. The e-tutorials are very helpful and cover in-depth topics.
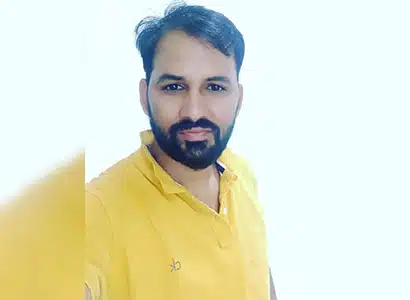
I have been working as a lab technician in Manipal University Jaipur for 8 years, I have good technical skills like video recording and editing. However, I wanted to improve my knowledge, so I decided to pursue an online MA JMC. I want to pursue my PhD after this online program, and I also hope to become a news anchor one day.
Our stories
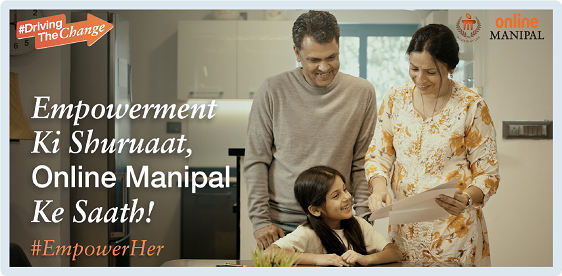
Empowerment Ki Shuruaat, Online Manipal Ke Saath | International Women's Day | Online Manipal
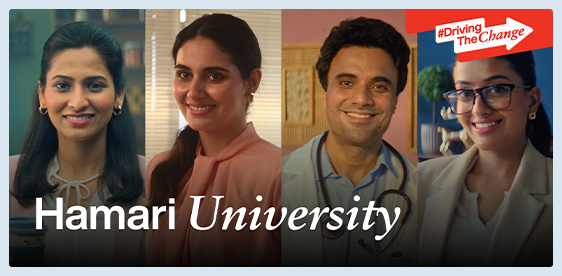
Hamari University; Apke Aur Apke Sapnon Ke Liye | #DrivingTheChange | Online Manipal
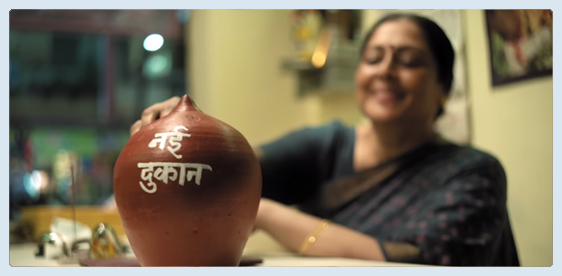
Brand Film | Online Manipal | #AzadiWaliDegree
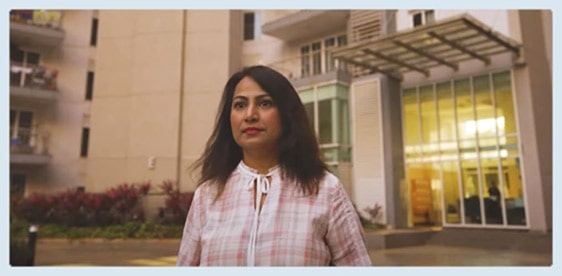
Learner Testimonial: Devyani's Journey With Online BBA (MUJ)
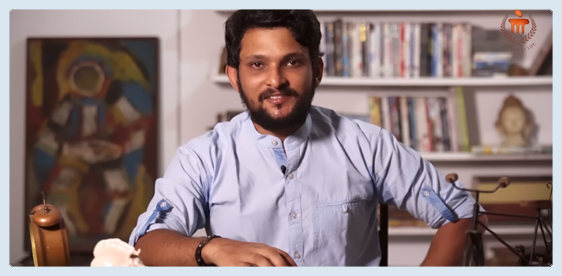
Learner Testimonial: Akhil's Journey With Online MBA (MUJ)
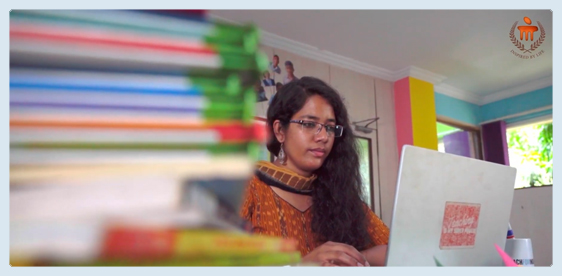
Learner Testimonial: Romila's Journey With Online MA JMC (MUJ)
Read our blogs
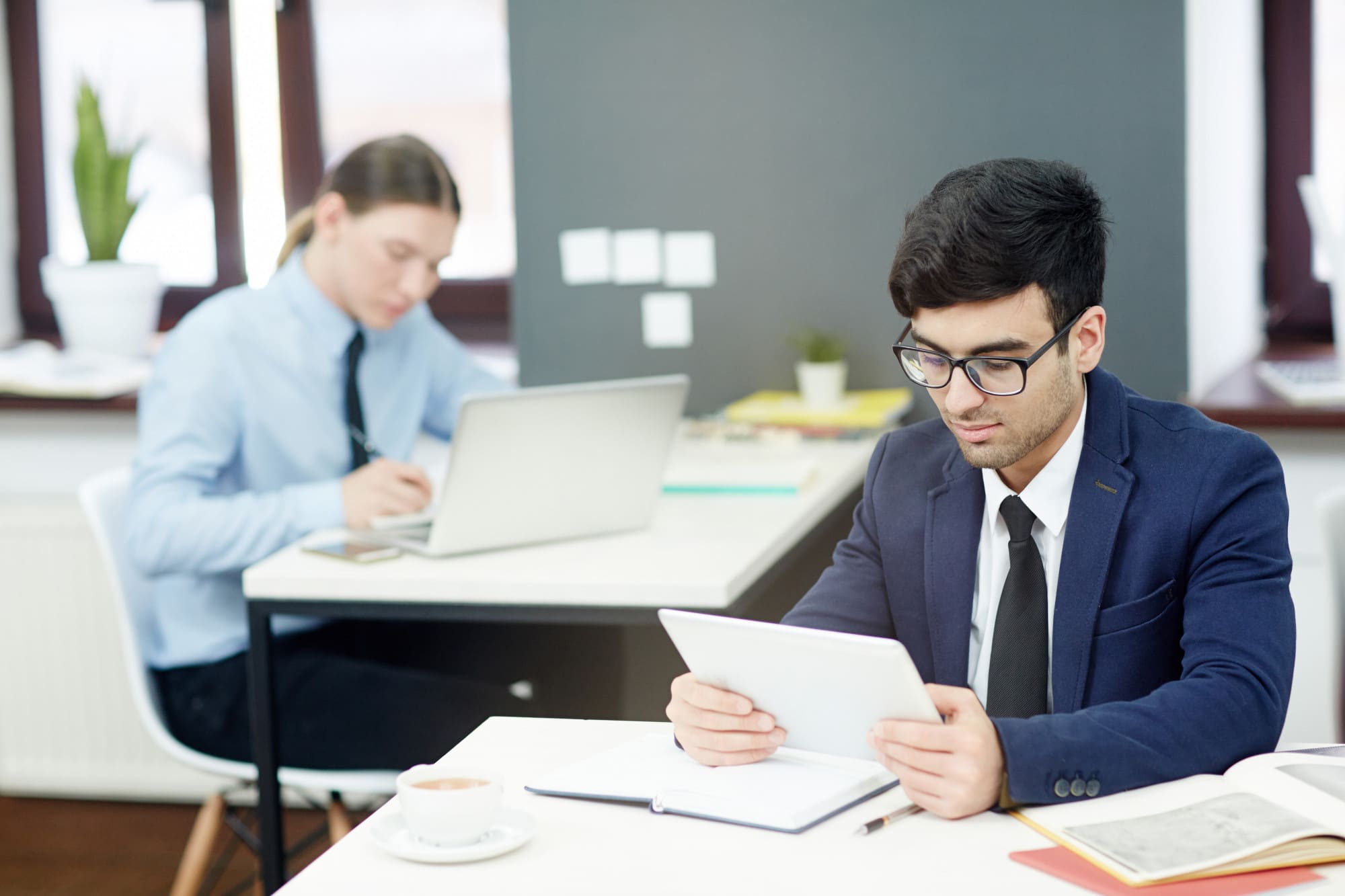
Top MBA degree courses in detail to prepare for a successful career

Executive MBA vs. Online MBA: Which one is better?
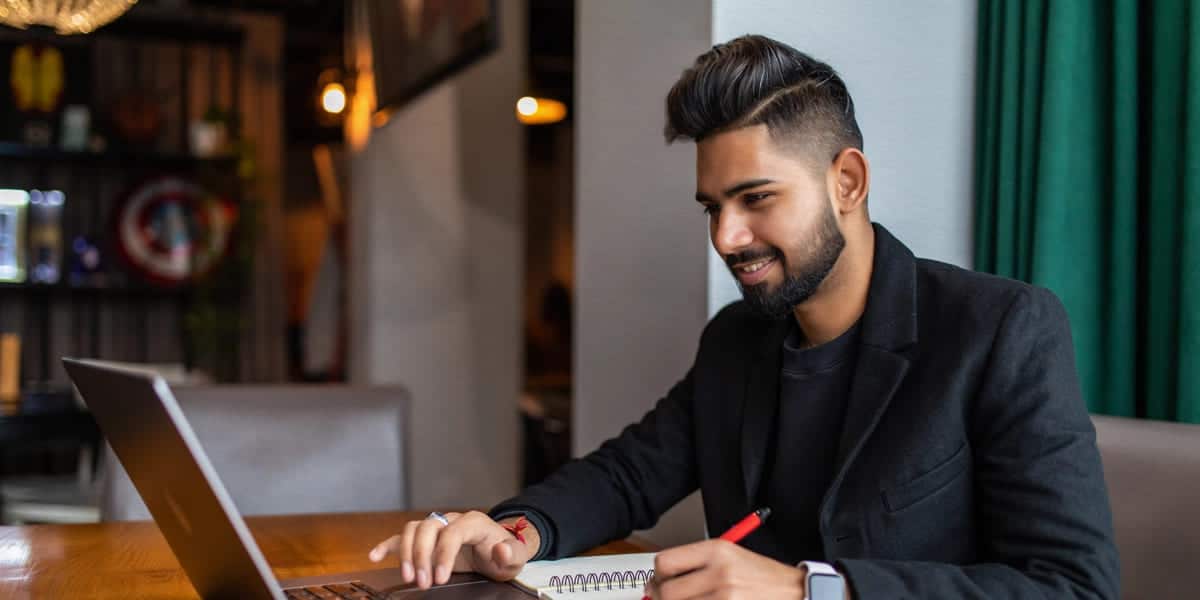
Why pursue an online MBA from Sikkim Manipal University
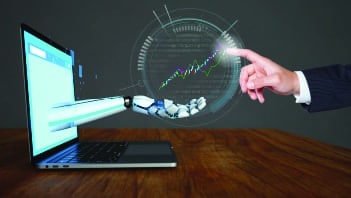
Internet of Things: Applications and advantages
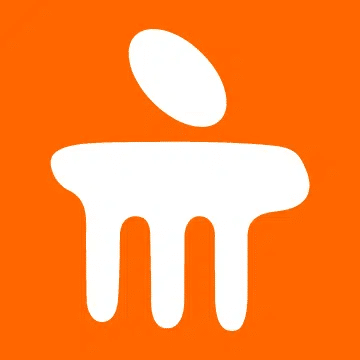
Interested in our courses? Share your details and we'll get back to you.
Master of Business Administration Bachelor of Business Administration Bachelor of Computer Applications Bachelor of Commerce Master of Computer Applications Master of Commerce Master of Arts in Journalism & Mass Communication MSc Data Science MSc Business Analytics PGCP Business Analytics PGCP Logistics and Supply Chain PGCP in Entrepreneurship and Innovation Bachelor of Arts MA in English MA in Sociology MA in Political Science
Manipal University Jaipur Manipal Academy of Higher Education Manipal Institute of Technology Sikkim Manipal University
I authorize Online Manipal and its associates to contact me with updates & notifications via email, SMS, WhatsApp, and voice call. This consent will override any registration for DNC / NDNC.
Enter the code sent to your phone number to proceed with the application form
COURSE SELECTED Edit
Bachelor of Business Administration (BBA) Manipal University Jaipur
Please leave this field empty. Submit

An official website of the United States government
The .gov means it’s official. Federal government websites often end in .gov or .mil. Before sharing sensitive information, make sure you’re on a federal government site.
The site is secure. The https:// ensures that you are connecting to the official website and that any information you provide is encrypted and transmitted securely.
- Publications
- Account settings
- My Bibliography
- Collections
- Citation manager
Save citation to file
Email citation, add to collections.
- Create a new collection
- Add to an existing collection
Add to My Bibliography
Your saved search, create a file for external citation management software, your rss feed.
- Search in PubMed
- Search in NLM Catalog
- Add to Search
CuPCA: a web server for pan-cancer association analysis of large-scale cuproptosis-related genes
Affiliations.
- 1 College of Biological Sciences and Technology, Beijing Forestry University, Beijing 100083, China.
- 2 College of Computer Science and Technology, Beijing Forestry University, Beijing 100083, China.
- 3 Department of Clinical Laboratory, Beijing Ditan Hospital, Capital Medical University, Beijing 100015, China.
- 4 College of Art, Beijing Forestry University, Beijing 100083, China.
- PMID: 39231258
- PMCID: PMC11373563
- DOI: 10.1093/database/baae075
Copper-induced cell death is a novel mechanism of cell death, which is defined as cuproptosis. The increasing level of copper can produce toxicity in cells and may cause the occurrence of cell death. Several previous studies have proved that cuproptosis has a tight association with various cancers. Thus, the discovery of relationships between cuproptosis-related genes (CRGs) and human cancers is of great importance. Pan-cancer analysis can efficiently help researchers find out the relationship between multiple cancers and target genes precisely and make various prognostic analyses on cancers and cancer patients. Pan-cancer web servers can provide researchers with direct results of pan-cancer prognostic analyses, which can greatly improve the efficiency of their work. However, to date, no web server provides pan-cancer analysis about CRGs. Therefore, we introduce the cuproptosis pan-cancer analysis database (CuPCA), the first database for various analysis results of CRGs through 33 cancer types. CuPCA is a user-friendly resource for cancer researchers to gain various prognostic analyses between cuproptosis and cancers. It provides single CRG pan-cancer analysis, multi-CRGs pan-cancer analysis, multi-CRlncRNA pan-cancer analysis, and mRNA-circRNA-lncRNA conjoint analysis. These analysis results can not only indicate the relationship between cancers and cuproptosis at both gene level and protein level, but also predict the conditions of different cancer patients, which include their clinical condition, survival condition, and their immunological condition. CuPCA procures the delivery of analyzed data to end users, which improves the efficiency of wide research as well as releases the value of data resources. Database URL: http://cupca.cn/.
© The Author(s) 2024. Published by Oxford University Press.
PubMed Disclaimer
Conflict of interest statement
None declared.
Outlines show the acquisition of…
Outlines show the acquisition of data and data output for the CuPCA visualization…
Top navigation of CuPCA functions…
Top navigation of CuPCA functions and the initial interface of CuPCA. (a) The…
Pan-cancer analysis of single CRG.…
Pan-cancer analysis of single CRG. (a) Differential analysis. (b) Survival analysis. (c) Clinical…
Pan-cancer analysis of multi-CRGs. (a)…
Pan-cancer analysis of multi-CRGs. (a) Differential analysis. (b) Presentation of the prognostic model…
Pan-cancer analysis of CRlncRNA. (a)…
Pan-cancer analysis of CRlncRNA. (a) Presentation of the prognostic model. (b) Verification results…
Pan-cancer conjoint analysis of mRNA–lncRNA–circRNA.…
Pan-cancer conjoint analysis of mRNA–lncRNA–circRNA. (a) Differential analysis. (b) Regulatory internet. (c) KEGG…
- Duan WJ, He RR. Cuproptosis: copper-induced regulated cell death. Sci China Life Sci 2022;65:1680–82. 10.1007/s11427-022-2106-6 - DOI - PubMed
- Wang D, Tian Z, Zhang P. et al. The molecular mechanisms of cuproptosis and its relevance to cardiovascular disease. Biomed Pharmacother 2023;163:114830. 10.1016/j.biopha.2023.114830 - DOI - PubMed
- Tsvetkov P, Coy S, Petrova B. et al. Copper induces cell death by targeting lipoylated TCA cycle proteins. Science 2022;375:1254–61. 10.1126/science.abf0529 - DOI - PMC - PubMed
- Xie J, Yang Y, Gao Y. et al. Cuproptosis: mechanisms and links with cancers. Mol Cancer 2023;22:46. 10.1186/s12943-023-01732-y - DOI - PMC - PubMed
- Chen L, Min J, Wang F. Copper homeostasis and cuproptosis in health and disease. Signal Transduct Target Ther 2022;7:378. 10.1038/s41392-022-01229-y - DOI - PMC - PubMed
- Search in MeSH
LinkOut - more resources
Full text sources.
- Silverchair Information Systems
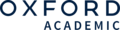
- Citation Manager
NCBI Literature Resources
MeSH PMC Bookshelf Disclaimer
The PubMed wordmark and PubMed logo are registered trademarks of the U.S. Department of Health and Human Services (HHS). Unauthorized use of these marks is strictly prohibited.
Log in using your username and password
- Search More Search for this keyword Advanced search
- Latest content
- For authors
- Browse by collection
- BMJ Journals
You are here
- Volume 14, Issue 9
- Evaluating the impact of artificial intelligence-assisted image analysis on the diagnostic accuracy of front-line clinicians in detecting fractures on plain X-rays (FRACT-AI): protocol for a prospective observational study
- Article Text
- Article info
- Citation Tools
- Rapid Responses
- Article metrics

- http://orcid.org/0000-0002-5880-8235 Alex Novak 1 ,
- Max Hollowday 2 ,
- http://orcid.org/0000-0003-0967-3554 Abdala Trinidad Espinosa Morgado 1 ,
- Jason Oke 3 ,
- http://orcid.org/0000-0001-6642-9967 Susan Shelmerdine 4 , 5 , 6 ,
- http://orcid.org/0000-0001-9598-189X Nick Woznitza 7 , 8 ,
- David Metcalfe 2 ,
- Matthew L Costa 2 , 3 , 9 ,
- http://orcid.org/0000-0003-3964-0809 Sarah Wilson 10 ,
- Jian Shen Kiam 2 ,
- http://orcid.org/0000-0002-0513-7220 James Vaz 2 ,
- http://orcid.org/0000-0002-6123-9838 Nattakarn Limphaibool 2 ,
- Jeanne Ventre 11 ,
- Daniel Jones 11 ,
- Lois Greenhalgh 12 ,
- Fergus Gleeson 13 ,
- Nick Welch 12 ,
- Alpesh Mistry 14 , 15 ,
- Natasa Devic 2 ,
- James Teh 16 ,
- http://orcid.org/0000-0001-9614-5033 Sarim Ather 2
- 1 Emergency Medicine Research Oxford , Oxford University Hospitals NHS Foundation Trust , Oxford , UK
- 2 Oxford University Hospitals NHS Foundation Trust , Oxford , UK
- 3 Nuffield Department of Primary Care Health Sciences , University of Oxford , Oxford , UK
- 4 Clinical Radiology , Great Ormond Street Hospital for Children , London , UK
- 5 Radiology , UCL GOSH ICH , London , UK
- 6 NIHR Great Ormond Street Hospital Biomedical Research Centre , London , UK
- 7 Radiology , University College London Hospitals NHS Foundation Trust , London , UK
- 8 Canterbury Christ Church University , Canterbury Christ Church University , Canterbury , UK
- 9 Nuffield Department of Orthopaedics, Rheumatology and Musculoskeletal Sciences (NDORMS), Oxford Trauma & Emergency Care (OxTEC) , University of Oxford , Oxford , UK
- 10 Frimley Health NHS Foundation Trust , Frimley , UK
- 11 Gleamer SAS , Paris , France
- 12 Patient and Public Involvement Member , Oxford , UK
- 13 Department of Oncology , University of Oxford , Oxford , UK
- 14 Liverpool University Hospitals NHS Foundation Trust , Liverpool , UK
- 15 North West MSK Imaging , Liverpool , UK
- 16 Nuffield Orthopaedic Centre , Oxford University Hospitals NHS Foundation Trust , Oxford , UK
- Correspondence to Dr Alex Novak; Alex.Novak{at}ouh.nhs.uk
Introduction Missed fractures are the most frequent diagnostic error attributed to clinicians in UK emergency departments and a significant cause of patient morbidity. Recently, advances in computer vision have led to artificial intelligence (AI)-enhanced model developments, which can support clinicians in the detection of fractures. Previous research has shown these models to have promising effects on diagnostic performance, but their impact on the diagnostic accuracy of clinicians in the National Health Service (NHS) setting has not yet been fully evaluated.
Methods and analysis A dataset of 500 plain radiographs derived from Oxford University Hospitals (OUH) NHS Foundation Trust will be collated to include all bones except the skull, facial bones and cervical spine. The dataset will be split evenly between radiographs showing one or more fractures and those without. The reference ground truth for each image will be established through independent review by two senior musculoskeletal radiologists. A third senior radiologist will resolve disagreements between two primary radiologists. The dataset will be analysed by a commercially available AI tool, BoneView (Gleamer, Paris, France), and its accuracy for detecting fractures will be determined with reference to the ground truth diagnosis. We will undertake a multiple case multiple reader study in which clinicians interpret all images without AI support, then repeat the process with access to AI algorithm output following a 4-week washout. 18 clinicians will be recruited as readers from four hospitals in England, from six distinct clinical groups, each with three levels of seniority (early-stage, mid-stage and later-stage career). Changes in the accuracy, confidence and speed of reporting will be compared with and without AI support. Readers will use a secure web-based DICOM (Digital Imaging and Communications in Medicine) viewer ( www.raiqc.com ), allowing radiograph viewing and abnormality identification. Pooled analyses will be reported for overall reader performance as well as for subgroups including clinical role, level of seniority, pathological finding and difficulty of image.
Ethics and dissemination The study has been approved by the UK Healthcare Research Authority (IRAS 310995, approved on 13 December 2022). The use of anonymised retrospective radiographs has been authorised by OUH NHS Foundation Trust. The results will be presented at relevant conferences and published in a peer-reviewed journal.
Trial registration numbers This study is registered with ISRCTN ( ISRCTN19562541 ) and ClinicalTrials.gov ( NCT06130397 ). The paper reports the results of a substudy of STEDI2 (Simulation Training for Emergency Department Imaging Phase 2).
- Artificial Intelligence
- Diagnostic Imaging
- Fractures, Closed
- Emergency Service, Hospital
- RADIOLOGY & IMAGING
This is an open access article distributed in accordance with the Creative Commons Attribution 4.0 Unported (CC BY 4.0) license, which permits others to copy, redistribute, remix, transform and build upon this work for any purpose, provided the original work is properly cited, a link to the licence is given, and indication of whether changes were made. See: https://creativecommons.org/licenses/by/4.0/ .
https://doi.org/10.1136/bmjopen-2024-086061
Statistics from Altmetric.com
Request permissions.
If you wish to reuse any or all of this article please use the link below which will take you to the Copyright Clearance Center’s RightsLink service. You will be able to get a quick price and instant permission to reuse the content in many different ways.
STRENGTHS AND LIMITATIONS OF THIS STUDY
This study uses a detailed artificial intelligence-assisted fracture detection algorithm with a National Health Service-derived dataset.
A broad set of health professionals will be recruited as participants, including under-represented groups such as nurse practitioners and physiotherapists.
The enhanced dataset will allow evaluation of a broad range of pathologies, including rare but significant fractures.
The dataset will have an abnormally high disease prevalence (50%) to include a broad range of pathologies.
The small number of readers may reduce the statistical power for comparison between professional groups.
Introduction
Missed fractures are a source of serious harm for patients attending the emergency departments (EDs) and represent the most common diagnostic error in that clinical setting. 1 Almost 2 million fractures occur annually in the UK with a lifetime prevalence of nearly 40%, 2 while 5.1% of all ED attendances are for fractures or dislocations. National Health Service (NHS) Resolution has identified that misinterpretation of plain radiographs was the most common error leading to a successful claim for negligent ED care, leading to significant impacts on the lives of affected patients. 3 Reported consequences include death, disability, deformity, need for further or prolonged treatments, chronic pain, emotional distress and loss of trust in the health service. 4 Furthermore, the need for further attendances and prolonged or corrective treatment leads to significant excess healthcare costs. 5
Most acute fractures are diagnosed by ED clinicians using plain radiographs as the first-line imaging investigation (National Clinical Guideline Centre, 2016), a task which requires time, skill and expertise. However, few of the clinicians fulfilling this role have any formal image interpretation training, and they vary significantly in experience. 6 Furthermore, a workforce shortage of radiologists in the UK means that they are rarely able to undertake the primary evaluation of plain radiographs in ED. 7 The high service pressures in UK EDs combined with a highly transient workforce results in a busy and distracting clinical environment that predispose to error and missing fractures on plain radiographs. An estimated 3.3% of fractures are missed on initial interpretation by ED staff. 8 The error rate is higher on radiographs interpreted outside daytime working hours, which suggests that fatigue, workload and shift patterns may impact clinician performance. 9
Over the last decade, advances in computer vision and machine learning have been used to augment interpretation of medical imaging. 10 Several artificial intelligence (AI) algorithms have been developed that are able to detect fractures on plain radiographs with a high degree of accuracy. 11 One such algorithm is the Gleamer BoneView (Gleamer, Paris, France) (see figure 1 ), which is currently the mostly widely used fracture detection algorithm in the NHS as well as worldwide (>800 sites in 30 countries). This algorithm estimates the likelihood of a fracture being present on a radiograph and provides users with three outcomes: fracture , no fracture and uncertain . If the likelihood has been estimated to be above a designated cut-off value, the area of abnormality is highlighted as a region of interest on a secondary image, which is made available to clinicians via their picture archive and communication system. If no abnormality is detected, this is also stated on the secondary image. 12 13 Prior studies have demonstrated that the algorithm is highly accurate at detecting abnormalities, and it is already in use in a number of European centres, having received regulatory approval for use to support clinicians interpreting plain radiographs. Previous research has suggested that the algorithm is highly accurate at detecting abnormalities, and it is already in use in a number of European centres, having received regulatory approval for use to support clinicians interpreting X-rays. Moreover, recent studies have suggested that the use of AI software for detecting bone fractures 14 15 can drastically decrease the rate of missed fractures. However, this software has not yet been fully tested in a UK setting using a locally derived dataset, and it is unclear to what degree such systems would affect the diagnostic performance of certain staff groups specific to the NHS, such as reporting radiographers and specialist nurse practitioners.
- Download figure
- Open in new tab
- Download powerpoint
Image of Gleamer Boneview showing artificial intelligence-assisted overlay.
This study will evaluate the impacts of a commercially available AI-assisted image interpretation tool (Gleamer BoneView) on the diagnostic performance of the full range of clinicians (including nurses and allied health professionals) who routinely diagnose fractures in the NHS. It will address this evidence gap in the current evidence base, in line with the NICE (National Institute for Health and Care Excellence) Evidence Standards Framework for Digital Health Technologies, and recent Early Value Assessments which highlight the dearth of prospective evidence to support the use of AI-assisted image interpretation algorithms in the UK healthcare setting. Automation bias (the propensity for humans to favour suggestions from automated decision-making systems) is a known source of error in human-machine interaction 16 and has been one of a number of causes for concern regarding the increasing usage of AI in radiology. 17 A recent reader study in mammography, 18 suggested significant automation bias presence across all levels of experience, noting that it was only the high-experienced reporters that consistently picked up on AI error. During our study, we will also assess the impact of incorrect advice given by the algorithm on the clinical end users. 19
To evaluate the impact of AI-enhanced imaging on the diagnostic performance, efficiency and confidence of clinicians in detecting fractures on plain radiographs (primary).
To determine the stand-alone diagnostic accuracy of the BoneView AI tool with respect to the reference standard (secondary).
To determine associations between professional background and level of experience when determining the impact of AI support on clinician fracture detection (secondary).
To explore which imaging factors influence clinicians’ reporting accuracy and efficiency, and algorithm performance, for example, category of abnormality, size of abnormality, image quality, presence of multiple abnormalities (secondary).
To measure whether clinicians are more likely to make a mistake when AI provides an incorrect diagnosis (secondary).
Methods and analysis
Study design.
This study employs a multiple reader multiple case (MRMC) methodology. This approach involves multiple readers of various specialties and experience levels interpreting a large set of radiographs with and without AI assistance. The study processes are summarised in the flowchart in figure 2 , with the dataflows represented in figure 3 . The study design encompasses several key elements, including participant selection, case reading procedures, ground truthing process, case selection and AI algorithm inference on cases, which will be described in detail in the following subtitles.
Study flowchart for artificial intelligence-assisted image analysis on the diagnostic accuracy of front-line clinicians in detecting fractures on plain X-rays multicase multireader study. AI, artificial intelligence; XRs, X-rays.
Artificial intelligence-assisted image analysis on the diagnostic accuracy of front-line clinicians in detecting fractures on plain X-rays study dataflows. AI, artificial intelligence; RAIQC, Report and Image Quality Control; XR, X-rays.
Participants
In order to explore the effects of using the algorithm on the full range of clinicians who diagnose fractures in routine practice and minimise selection bias, we have created a balanced matrix of readers in terms of specialty and seniority. 18 readers will be recruited from the following specialties (six specialities with three readers from each):
Emergency physicians.
Trauma and orthopaedic surgeons.
Emergency nurses practitioners.
Physiotherapists.
General radiologists.
Reporting radiographers.
Each specialty group will consist of a reader each fulfilling one of the following three levels of seniority:
Consultant/senior/equivalent: >10 years experience.
Registrar/equivalent: 5–10 years experience.
Senior house officer/equivalent: <5 years experience.
Each specialty reader group will include one reader at each level of experience. Readers will be excluded if they have significant radiology experience in excess of their current specialty or grade. Prior use of fracture detection software does not exclude participation, as it is not expected in itself to confer a change in performance unless actively used during interpretation.
Readers will be recruited from across four NHS organisations that comprise the Thames Valley Emergency Medicine Research Network ( www.TaVERNresearch.org ):
Oxford University Hospitals (OUH) NHS Foundation Trust.
Royal Berkshire NHS Foundation Trust.
Frimley Health NHS Foundation Trust.
Milton Keynes University Hospital NHS Foundation Trust.
Participants will be recruited through a structured invitation process coordinated by the research team. A designated team member will collaborate with clinical leads and research coordinators at each participating site within the Thames Valley Emergency Medicine Research Network to identify potential participants based on predetermined criteria. These criteria include fulfilment of the required specialty and experience level categories, demonstrated commitment to professional development and research, and ability to commit to the full duration of the study.
All invitations will be extended based on the aforementioned criteria, and participation will be voluntary, maintaining objectivity throughout the recruitment process.
The reads will be performed using a secure web-based DICOM viewer ( www.raiqc.com ). The platform allows readers to view radiographs and identify the site of an abnormality with a mouse click. The images will be viewable through a web browser on desktop or laptop devices, reflecting standard real-world hospital practice in which radiographs are typically interpreted by clinicians without dedicated high-resolution viewing stations.
Prior to beginning each phase of the study, the readers will undergo a training module that includes reading 5 practice images (not part of the 500-image dataset) to familiarise themselves with the use of the study platform and the output of the AI tool.
Case selection and composition
The image dataset will include anonymised radiographs of adult patients (≥18 years) who presented to the EDs of OUH NHS Foundation Trust with a suspicion of fracture after injury to the limbs, pelvis or thoracolumbar spine. As CT is the investigation of choice for skull and many cervical spine injuries, these will be excluded from the study. Paediatric patients will be excluded from the dataset as their fracture types differ from those in adults, and there is an ongoing study evaluating this aspect (FRACTURE study; Fast Reporting using Artificial Intelligence for Children's TraUmaticRadiology Examinations 12 ). Obvious fractures (defined as fractures including any of the following: displacement>5 mm, shortening>5 mm or angulation>5°) will also be excluded.
To constitute the dataset, radiology reports will be screened from the radiology information system to develop an enriched dataset of the 500 standard clinical examinations evenly split between normal and abnormal, with one or more fractures. The ratio of radiographs from each anatomical location has been informed by the proportion of missed fractures mentioned in the NHS Resolution report ( table 1 ).
- View inline
Proportion of radiographs of each anatomical location, based on the proportion of missed fractures mentioned in the National Health Service Resolution report
To ensure a like-for-like comparison, image finding for abnormal cases will be performed first. The normal images will be age and sex matched per body part. We will aim to include representation of the different image views, system type (mobile or fixed), system vendors and patient demographics (eg, age, sex) without any prespecified quota.
The dataset will then be anonymised and uploaded to the Report and Image Quality Control platform under an existing data governance approval from the OUH NHS Foundation Trust Caldicott guardian.
Case inclusion and exclusion summary
Plain radiographs of adult patients (age>18 years) presenting to the OUH ED with a suspected fracture.
Plain skull radiographs.
Plain cervical spine radiographs.
Follow-up radiographs for known fracture.
Paediatric radiographs (age<18).
Obvious fractures defined as:
Displacement>5 mm.
Shortening>5 mm.
Angulation>5°.
Inferencing the image dataset
The entire dataset of images will then be separately analysed using BoneView, creating a duplicate dataset of radiographs with alerts and regions of interest indicated.
Radiographic interpretation
All readers will review all 500 radiographs individually across 2 reporting rounds.
In the first round, they will interpret the images as per clinical practice without any AI assistance. After a washout period of a month to mitigate the effects of recall bias, they will review the same 500 radiographs a second time with the assistance of the algorithm, which will contribute its suggestions as to abnormality presence and location. In both sessions, clinicians will be blinded to the ground truth established by the MSK (musculoskeletal) radiologists.
Clinician readers will be asked to identify the presence or absence of fracture by placing a marker on the image at the location of the fracture (if present) and to rank their confidence for fracture identification. Confidence rating will take the form of a Likert scale from 1 to 5 with 1 being least confident and 5 most confident.
Ground truthing
The gold standard reference process will be conducted by two experienced musculoskeletal radiologists (>10 years’ experience) who will independently review and annotate each of the 500 radiographs in the dataset. They will draw bounding boxes around each detected fracture and grade the images on both image quality and difficulty of abnormality detection using a 5-point Likert scale.
In cases of disagreement between the two primary radiologists regarding the presence or absence of abnormalities, a third senior musculoskeletal radiologist will review the contentious images and make a final decision.
All annotations, gradings and arbitration decisions will be documented within the secure web-based DICOM viewer platform, establishing a reliable reference standard for evaluating both human reader performance and AI assistance.
In the event of significant discrepancies persisting after the initial arbitration process, a consensus meeting will be agreed. This meeting will include the primary ground truth radiologists, the arbitrator and key members of the research team. The purpose of this meeting will be to review and resolve any remaining discrepancies, ensuring the integrity and consistency of the final reference standard. This collaborative approach will be employed only for cases where substantial disagreement remains, thereby maintaining the overall objectivity of the ground truth process while addressing complex or ambiguous cases.
Study timeline
This study commenced on 8 February 2024 and is actively collecting data. The data collection and analysis phase is projected to finish by the end of September 2024 with write up and publication anticipated later in the year.
Outcome measures
Reader and AI performance will be evaluated using sensitivity, specificity, positive predictive value (PPV), negative predictive value (NPV) and area under receiver operating characteristic curve (AUC). Reader performance will be evaluated with and without AI assistance.
Reader speed will be evaluated as the mean review time per scan, with and without AI assistance.
Reader confidence will be evaluated as self-reported diagnostic confidence on a 5-point Likert scale, with and without AI assistance.
Data statement and management
Radiographs selected for the study will be anonymised in accordance with OUH NHS Foundation Trust information governance protocol and uploaded to the secure image viewing platform ( www.raiqc.com ). Access to the radiographs will be controlled via the study platform using separate user accounts for each reader.
All study data will be entered into a password-protected and secure database. Individual reader accuracy scores will be anonymised, and the study team will not have access to the identifying link between the participants’ personal details and the data. Data about the participants’ seniority level and professional group will be retained to allow group comparisons.
Sample size and power calculation
The study’s sample size of 500 images, evenly split between normal and abnormal cases, was determined using the Multi-Reader Sample Size Program for Diagnostic Studies. This tool, developed by Hillis, 20 is specifically designed for MRMC study power calculations. Based on parameters derived from our previous MRMC study on pneumothorax detection, the programme calculated that with 18 readers and 500 cases, our study will achieve 85% power to detect a 10% difference in accuracy between unassisted and AI-assisted readings, with a 5% type 1 error rate (See output from software below).
The chosen sample size of 500 images ensures sufficient statistical power and adequate representation of fracture types and anatomical locations. This robust sample size, combined with our substantial and diverse reader pool, should enable the detection of clinically significant improvements in fracture detection accuracy and allow for subgroup analyses across specialties and experience levels. By using this rigorously calculated sample size, we aim to produce statistically robust and clinically relevant results that can inform the potential integration of AI assistance in fracture detection across various clinical settings, while adequately addressing our study objectives and maintaining statistical validity.
Statistical analyses
The performance of the algorithm will be compared with the ground truth generated by the musculoskeletal radiologist panel. The continuous probability score from the algorithm will be used for the AUC analyses, while binary classification results with three different operating cut-offs will be used for evaluation of sensitivity, specificity, PPV and NPV. Sensitivity and specificity of readers with and without AI will be tested based on the Obuchowski-Rockette model for MRMC analysis which will model the data using a two-way mixed effects analysis of variance (ANOVA) model treating readers and cases (images) as random effects and effect of AI as a fixed effect with recommended adjustment to df by Hillis. 21
The difference in diagnostic characteristics (sensitivity, specificity, accuracy, area under the receiver operating characteristic (ROC) curve) of readers as compared with ground truth with and without AI assistance will be the primary outcome on a per image and per abnormality basis. The main analysis will be performed as a single pooled analysis including all groups and sites. Secondary outcomes will include comparison between the performance of subgroups by specialty (emergency medicine, trauma/orthopaedics, physiotherapy, nurse practitioner, radiologist, radiographer), level of seniority (senior, middle grade, junior), degree of difficulty of the image and by anatomical region. Reader-reported confidence with and without the AI assistance will be compared. Secondary outcomes include the diagnostic characteristics of the AI algorithm alone. Surveys will be conducted throughout the study to measure the satisfaction, adoption and confidence in the AI algorithm of the study participants. Per-patient sensitivity will be defined as the proportion of reads in which all true fractures were marked as a proportion of the reads having at least one fracture. Per-patient specificity will be defined as the proportion of reads in which no fracture was marked by the reader as a proportion of the reads that did not show a fracture. These definitions disregard the detection of multiple fractures thus we will define the fracture-wise sensitivity as the proportion of fractures correctly detected as a proportion of all fractures. The two coprimary outcomes will be patient-wise sensitivity and patient-wise specificity. The stand-alone algorithm performance will be assessed by calculating the area under the curve (AUC) of the ROC and free-response ROC curves plotted with their variance. To account for correlated errors arising from readers interpreting the same images with and without AI, the Obuchowski and Rockette, Dorfman-Berbaum-Metz 22 procedure; a modality-by-reader random effects ANOVA model will be used for estimation. Analyses will be carried out using R and the MRMCaov library.
Strengths and limitations
This study uses a CE (Conformité Européenne)-marked AI-assisted fracture detection algorithm with an NHS-derived dataset. The enhanced dataset will allow evaluation of a broad range of pathologies, including rare but significant fractures and its composition is mapped to mirror the proportions of missed fracture locations seen in the NHS Resolution report. A broad set of health professionals will be recruited as participants, including under-represented groups such as nurse practitioners and physiotherapists, from multiple hospital sites across the region—these reflect a reader group not yet explored in the literature, and one directly applicable to the NHS.
In terms of limitations, while the overall study group is large in comparison to other similar reader studies, the small number of readers in subgroups may reduce the statistical power for comparison between professional groups. The dataset will include an abnormally high disease prevalence (50%) to include a broad range of pathologies to facilitate meaningful statistical comparison, meaning that while the reader study will effectively explore the impact of the algorithm on readers interpreting a broad and detailed dataset, the results will not mirror the prevalence of pathologies encountered in normal clinical practice and further prospective study will be required to determine efficacy in this regard.
Patient and public involvement (PPI)
This protocol has been reviewed by the Oxford ACUTECare PPI group and PPI representatives on the artificial intelligence-assisted image analysis on the diagnostic accuracy of front-line clinicians in detecting fractures on plain X-rays steering group. They have supported the study and its aims, were involved in the grant application, design and data management stages and have advised on dissemination strategies.
Ethics and dissemination
The study has been approved by the UK Health Research Authority (IRAS number 310995, approved on 13 December 2022). The use of anonymised retrospective radiographs has been authorised by the Caldicott Guardian and information governance team at OUH NHS Foundation Trust. Readers will provide written informed consent and will be able to withdraw at any time.
The study is registered at Clinicaltrials.gov ( NCT06130397 ) and the ISRCTN ( ISRCTN19562541 ) registry (approval pending reference 44612). The results of the study will be presented at relevant conferences and published in peer-reviewed journals. The detailed study protocol will be freely available on request to the corresponding author. Further dissemination strategy will be strongly guided by our PPIE (Patient and Public Involvement and Engagement) activities. This will be based on co-productions between patient partners and academics and will involve media pieces (mainstream and social media) as well as communication through charity partners. Key target audiences will include non-specialist clinicians routinely involved in fracture detection, as well as hospital managers, health policy-makers and academics working in AI-assisted image analysis.
Ethics statements
Patient consent for publication.
Consent obtained directly from patient(s).
Acknowledgments
The authors would link to thank FRACT-AI steering committee: Matthew Costa, Natasa Devic, Fergus Gleeson, Divyansh Guilati, Daniel Jones, Jian Shen Kiam, Nattakarn Limphaibool, David Metcalfe, Jason Oke, Ravi Shashikala, Susan Shelmerdine, James Teh, Simon Triscott, Jeanne Ventre, James Vaz, Nick Welch, Sarah Wilson, Nicholas Woznitza.
- Hussain F ,
- Carson-Stevens A , et al
- Donaldson LJ ,
- Reckless IP ,
- Scholes S , et al
- Bousson V ,
- Benoist N , et al
- Hayashi D ,
- Kompel AJ ,
- Ventre J , et al
- NHS Resolution
- Mitchell D ,
- Jenkins PJ ,
- van Leeuwen KG ,
- Schalekamp S ,
- Rutten MJCM , et al
- Bollard SM , et al
- Shelmerdine SC ,
- Liu H , et al
- Fracture Study
- Ducarouge A ,
- Gillibert A , et al
- Guermazi A ,
- Tannoury C ,
- Kompel AJ , et al
- Challen R ,
- Pitt M , et al
- Coppola F , et al
- Dratsch T ,
- Rezazade Mehrizi M , et al
- Harrison C ,
- Curran TA , et al
- Hillis SL ,
- Berbaum KS ,
X @SusieShels, @xray_nick
Contributors AN and SA led the conception and overall study design, contributed to protocol review and are co-chief investigators of the study. Both will carry out the analysis and interpretation of the results, independently write up the findings and handle publication. AN led the NIHR grant application and provided specialist emergency medicine input. SA provided specialist radiology input. MH and ATEM contributed to protocol drafting, study registration and recruitment. JO will carry out the independent statistical analysis. FG, SS, NW, DM, MLC, SW, JSK, JVaz, NL, JVentre and DJ were involved in study design and provided steering group inputs. ND and AM serve as ground truthers for the study, and JT serves as the arbitrator. LG and NW are PPI representatives. All authors contributed to the writing of the protocol and reviewed the manuscript. The guarantor of the study is AN; accepts full responsibility for the finished work and/or the conduct of the study, had access to the data, and controlled the decision to publish.
Funding This work was supported by the NIHR Research for Patient Benefit in Health and Care Award (NIHR204982).
Competing interests JV and DJ of the Steering Committee are employees of Gleamer SAS, France. SA is a shareholder of RAIQC, UK. All other authors declare no competing interests.
Patient and public involvement Patients and/or the public were involved in the design, or conduct, or reporting, or dissemination plans of this research. Refer to the Methods and analysis section for further details.
Provenance and peer review Not commissioned; externally peer reviewed.
Author note Transparency Declaration: The lead author, AN, affirms that this manuscript is an honest, accurate and transparent account of the study being reported; that no important aspects of the study have been omitted; and that any discrepancies from the study as planned have been explained.
Read the full text or download the PDF:

- Entertainment
- Life & Style

To enjoy additional benefits
CONNECT WITH US

R.G. Kar hospital rape-murder case: Calcutta High Court seeks CBI report on nasty social media posts on victim
The court noted that nasty comments have been made with the photograph of the victim as found in the copies of the offending posts provided by the petitioner.
Updated - September 05, 2024 04:53 pm IST - Kolkata

A view of the Calcutta High Court in Kolkata. File | Photo Credit: The Hindu
The Calcutta High Court on Thursday (September 5, 2024) directed the Central Bureau of Investigation (CBI) to file a report by September 18 on detestable posts being made on social media regarding the rape-murder victim of R.G. Kar Medical College and Hospital.
The court noted that nasty comments, which are far from being acceptable to any member of the society, have been made with the photograph of the victim as found in the copies of the offending posts provided by the petitioner.
Also Read : Kolkata doctor rape and murder case: The complete coverage
The body of the on-duty trainee doctor was found in the seminar room of the State-run hospital on August 9, triggering nationwide outrage. The CBI is investigating the case from the second week of August on an order of the High Court.
The petitioner prayed in the PIL that the CBI be directed to investigate the cyber offences in connection with the "unfortunate and brutal incident." The court asked the Additional Solicitor General Asok Kumar Chakrabarti, representing the Central agency, whether it could find a way to block these types of posts.
The division Bench, presided by Chief Justice T. S. Sivagnanam, directed the joint director, CBI, Kolkata, to look into the grievance expressed by the petitioner with regard to the issue of such abominable social media posts.
The Bench, also comprising Justice Hiranmay Bhattacharyya, directed the CBI joint director or any other competent officer to file a report in this regard by September 18, when the PIL will come up for hearing again along with other petitions relating to the August 9 rape-murder of the doctor.
Also Read : Kolkata doctor’s rape-murder: Parents allege police tried to hush up case, bribe them
After the order was dictated, Mr. Chakrabarti submitted before the court that the CBI does not have any separate wing to investigate such cyber crimes.
Maintaining that the State police has a special unit to monitor such matters, he prayed that the West Bengal government's cyber crime branch be also directed to file a separate report. The court said that it would consider the prayer on the next date of hearing.
Published - September 05, 2024 01:49 pm IST
Related Topics
West Bengal / Kolkata / The fight against rape / sexual assault & rape / murder / crime, law and justice / crime
Top News Today
- Access 10 free stories every month
- Save stories to read later
- Access to comment on every story
- Sign-up/manage your newsletter subscriptions with a single click
- Get notified by email for early access to discounts & offers on our products
Terms & conditions | Institutional Subscriber
Comments have to be in English, and in full sentences. They cannot be abusive or personal. Please abide by our community guidelines for posting your comments.
We have migrated to a new commenting platform. If you are already a registered user of The Hindu and logged in, you may continue to engage with our articles. If you do not have an account please register and login to post comments. Users can access their older comments by logging into their accounts on Vuukle.
- Open access
- Published: 05 September 2024
The relationship between salivary cytokines and oral cancer and their diagnostic capability for oral cancer: a systematic review and network meta-analysis
- Lijun Huang 1 , 2 ,
- Fen Luo 1 , 2 ,
- Mingsi Deng 1 , 2 &
- Jie Zhang 1 , 2
BMC Oral Health volume 24 , Article number: 1044 ( 2024 ) Cite this article
Metrics details
Oral cancer (OC) is a common malignancy in clinical practice. Saliva testing is a convenient and noninvasive early diagnostic technique for OC. Several salivary cytokines have been identified as potential biomarkers for OC, including IL-8, IL-6, TNF-α, IL-1β, and IL-10. Nonetheless, the optimal cytokine for OC diagnosis remains inconclusive and highly contentious.
PubMed, Embase, Web of Science, and Cochrane Library databases were comprehensively retrieved to collect all case–control studies on OC. A meta-analysis was performed to compare the levels of salivary IL-8, IL-6, IL-10, TNF-α, and IL-1β in OC patients and healthy controls. Network meta-analysis (NMA) was carried out to probe into the accuracy of these salivary cytokines in diagnosing OC.
This analysis included 40 studies, encompassing 1280 individuals with OC and 1254 healthy controls. Significantly higher levels of salivary IL-8, IL-6, TNF-α, IL-1β, and IL-10 were observed in patients with OC in comparison to healthy controls. The results of NMA showed that TNF-α had the highest diagnostic accuracy for OC, with a sensitivity of 79% and a specificity of 92%, followed by IL-6 (sensitivity: 75%, specificity: 86%) and IL-8 (sensitivity: 80%, specificity: 80%).
This study suggests that IL-8, IL-6, IL-10, TNF-α, and IL-1β may be potential diagnostic biomarkers for OC. Among them, TNF-α, IL-6, and IL-8 are highly accurate in the diagnosis of OC. Nevertheless, further studies that eliminate other confounding factors are warranted, and more standardized procedures and large-scale studies are needed to support the clinical use of saliva testing.
Peer Review reports
Introduction
Oral cancer (OC) is one of the most frequent aggressive malignancies worldwide. It was estimated that there were 377,713 new OC cases (approximately 2% of all cancer cases) and 177,757 deaths (1.8% of all cancer-related deaths) in 2020 around the world [ 1 ]. This cancer may locally invade the tongue, lips, lower and upper gums, hard palate, retromolar trigone, and floor of the mouth, and even metastasize to distant sites at an advanced stage [ 2 ]. Squamous cell carcinoma (OSCC) represents approximately 90% of OC cases [ 3 ], and other OC types encompass salivary gland tumors, lymphomas, and sarcomas [ 4 ]. Due to asymptomatic characteristics, around 50% of individuals with OC are diagnosed at a late stage. Accordingly, the treatment of such patients is often aggressive and mutilating, adversely affecting their quality of life [ 5 ]. The 5-year relative survival rate is about 85.1% for localized OC, and is as low as 69.1% and 39.3% for lymphatic metastasis and distant metastasis, respectively [ 6 ]. Therefore, early diagnosis of OC is essential to reduce the mortality rate and ameliorate the quality of life of OC patients.
Currently, commonly-used diagnostic approaches for OC include traditional oral visual examination (VOE), classical biopsy followed by histopathological assessment, vital staining (such as toluidine blue), and radiographic imaging [ 7 ]. Among them, biopsy and histopathological examinations are still the standard procedures for the diagnosis of OC [ 8 ]. Besides, the analysis of body fluids, especially saliva, is a promising and potential alternative to biopsy for early OC detection since it is adjacent to cancer cells, readily available, non-invasive, and inexpensive [ 9 ]. Human saliva consists of cytokines, circulating cells, DNA and RNA molecules, and derivatives of tissue and extracellular vesicles (EVs) [ 10 ]. Cytokines, as key mediators of cell communication, can control complex and dynamic cell–cell interactions and regulate various cancer-related pathways in the tumor microenvironment [ 11 ]. In histiocytology, cytokines such as IL-6 and IL-8 that are important in pro-inflammatory and pro-angiogenic responses can be detected in cell lines, tissue specimens, and serum of patients with Head and Neck squamous cell carcinoma (HNSCC includes OSCC, pharyngeal squamous cell carcinoma, laryngeal squamous cell carcinoma, nasal squamous cell carcinoma, paranasal sinuses squamous cell carcinoma etc. [ 12 , 13 ]. Moreover, a large scale gene expression profiling assisted by laser capture microdissection and microarray analysis was carried out, identifying the expression of 2 cellular genes: interleukin (IL)6 and IL-8 which are uniquely associated with OSCC [ 14 ]. Besides, a direct link between oral inflammation and cancer invasion was established by showing that neutrophils increase OSCC invasion through a tumor necrosis factor (TNFα)-dependent mechanism [ 15 ]. According to many case–control studies and previous systematic reviews and meta-analyses, the average levels of salivary cytokines such as IL-6, IL-8, TNF-α, IL-1β, and IL-10 are significantly different between OSCC, oral potentially malignant disorders (OPMD), oral leukoplakia (OL) and control saliva. Previous studies also showed that IL-6 and IL-8 concentrations in saliva were associated with different stages of OSCC and the presence of cervical metastasis [ 6 , 16 , 17 ]. Taken together, these findings suggest that these salivary cytokines may be potential diagnostic biomarkers for OC.
Nevertheless, there is no consensus on which biomarkers have the best diagnostic value for OC. Network meta-analysis for diagnostic tests (NMA-DT) is a new analysis approach that allows simultaneously comparing multiple diagnostic tests, at multiple test thresholds [ 18 ]. This novel technique can lessen bias and enhance statistical accuracy in the comparison of the diagnostic performance of multiple tests by borrowing strength from indirect evidence [ 19 ]. Therefore, this network meta-analysis was implemented to evaluate and compare the accuracy of five common salivary cytokines (IL-8, IL-6, TNF-α, IL-1β, and IL-10) in diagnosing OC and to rank these diagnostic tests based on a superiority index.
Protocol and registration
This study was conducted following the Preferred Reporting Items for Systematic Reviews and Meta-Analyses for Diagnostic Test Accuracy (PRISMA-DTA) Checklist (The prisma-DTA checklist is in the Supplementary Table S5) [ 20 ]. The study protocol was registered on the International Prospective Register of Systematic Reviews (PROSPERO; registration ID: CRD42023430533).
Inclusion and exclusion criteria
The following studies were included: (1) case–control studies on human subjects; (2) patients diagnosed with OC; (3) OC was confirmed pathologically; (4) the controls were healthy subjects without systemic diseases; (5) studies that reported at least one of the diagnostic sensitivity and specificity of IL-8, IL-6, IL-10, TNF-α, or IL-1β in OC patients compared to healthy controls with no systemic disease and the concentrations of these salivary biomarkers.
The exclusion criteria were as follows: (1) duplicate publications; (2) reviews, systematic reviews, meta-analyses; (3) conference summaries/abstracts, case reports, guidelines, letters to editors, editorials, study protocols, brief correspondences, animal experiments; (4) full texts unavailable; (5) outcome data unextractable; (6) non-English articles.
Literature search
As of 10 April 2023, two independent investigators (Lijun, Huang (L, H) and Mingsi, Deng (M, D)) extensively retrieved electronic databases, including PubMed, Embase, Web of Science, and Cochrane Library. No restrictions were imposed on study type, date/time, or publication status. Search terms encompassed “cytokine”, “saliva”, and “oral cancer” in combination with “interleukin” or “interferon”. The specific search strategy is delineated in Table S1. Besides, the reference lists of related studies and reviews were manually retrieved.
Study selection
All retrieved studies were imported into EndNote 20 to eliminate duplicate records. Two investigators (L, H and Fen, Luo (F, L)) independently checked the titles and abstracts to remove irrelevant articles. A full-text review was then conducted to select eligible studies. A third reviewer (M, D) was consulted to settle any disagreements that arose throughout the literature screening process.
Data extraction and quality assessment
Relevant data were independently extracted from included articles by two investigators, encompassing title, first author, publication year, country, study design, sample size, gender, patient age, the outcomes (levels of different cytokines in saliva, sensitivity and specificity, true positives (TP), false positives (FP), true negatives (TN) and false negatives (FN) in predicting OC). Dissents, if any, were settled by consulting a third investigator.
The Newcastle–Ottawa Scale (NOS) [ 21 ] was employed to evaluate the quality of the included articles. Two researchers (L, H and M, D) independently assessed 8 items in three domains: selection of case and control groups, comparability between groups, and exposure factors. Except for comparability, which has 2 points, each item in the remaining domains has 1 point. The total score ranges from 0 to 9 points. Studies rated 8 or higher were regarded as high quality, while those rated 4 or less were deemed to be of low quality. Meanwhile, studies with a score of 5–7 were considered to have a medium quality. The Quality Assessment on Diagnostic Accuracy Studies (QUADAS) 2 tool were used to assess the risk of bias [ 22 ], too. The risk of bias was assessed in four key domains including patient selection, index test(s), reference standard, and flow and timing. Concerns regarding applicability (patient selection, index test(s), and reference standard) were determined. The degree of bias and applicability were expressed as high, low, or unclear, in accordance with the guidance documents. The quality assessment was implemented independently by two researchers. Any dissents were resolved by a third researcher (F, L).
Statistical analyses
A traditional meta-analysis was carried out to pool data from studies comparing the levels of salivary IL-8, IL-6, IL-10, TNF-α, and IL-1β in OC patients and controls (non-OC). Standardized mean difference (SMD) with 95% confidence intervals (CIs) was used as the effect size. A p < 0.05 signals statistical significance. Forest plots were generated to visually present the results. The I 2 statistic was utilized to examine heterogeneity among studies. I 2 > 50% indicates statistically significant heterogeneity, and therefore, a random-effects model was applied for data analysis. Potential publication bias was determined by using a funnel plot and Egger’s test.
Furthermore, a network meta-analysis for diagnostic tests (NMA-DT) was conducted to delve into which saliva cytokine is the most accurate for predicting OC. NMA-DT enables us to concurrently compare several diagnostic tests of saliv a cytokines with the gold standard, at various thresholds [ 23 ]. The relative performance, sensitivity, and specificity of the index tests were assessed in relation to standard diagnostic method for OC, and these tests were ranked utilizing the diagnostic odds ratios (DORs) and superiority index (Table S2 in the Supplementary Materials explains all statistical terms). Higher DOR and superiority values indicate higher accuracy of tests in detecting diseases. This network meta-analysis was conducted using the R package “rstan” (version 4.1.3; R Foundation for Statistical Computing, Vienna, Austria). Analysis of Variance (ANOVA) model based on the Bayesian algorithm was applied to exhibit network meta-analysis among four systems by utilizing two independent binomial distributions to describe the true positive and true negative rates between OC and non-OC patients, meantime considering the correlation between sensitivity and specificity [ 24 ]. In order to improve accuracy and compare diagnostic assays one by one, calculations were repeated 7 times (model_code = model, chains = 2, iterations = 10,000, warmup = 5000, thin = 5), and then, league tables for relative comparations were drawn. Review manager version 5.4 was employed to calculate summary receiver operating characteristic curve (SROC) values.
Initially, 3075 articles were obtained from the database search. After removing 1082 duplicates, 1993 articles remained. After screening the titles and abstracts, 58 articles were potentially eligible. Based on a full-text review, 18 studies were further excluded, including 4 studies without healthy-control group, 8 studies without required experimental group, 4 studies without cytokines of interest, 1 study with no outcome of interest, and 1 commentary. Finally, 40 articles were included in the traditional meta-analysis and 12 articles in the network meta-analysis. The study selection process is delineated in Fig. 1 .
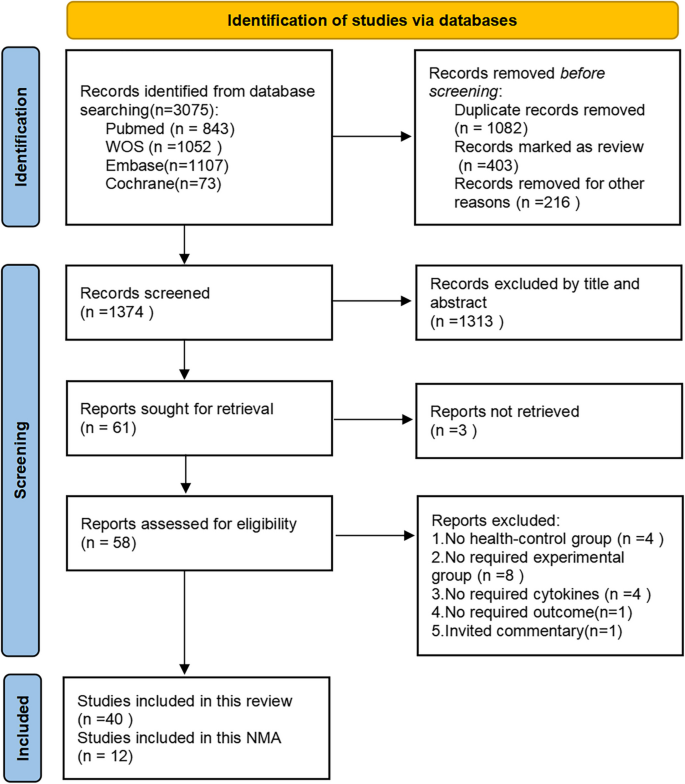
PRISMA flow diagram for search and selection of eligible studies included in the network meta-analysis
Study characteristics
The characteristics of the 40 included studies are presented in Table 1 . These studies were published between 2004 and 2023. 19 studies were conducted in Asia [ 25 , 26 , 27 , 28 , 29 , 30 , 31 , 32 , 33 , 34 , 35 , 36 , 37 , 38 , 39 , 40 , 41 , 42 , 43 ], 11 studies in Europe [ 16 , 44 , 45 , 46 , 47 , 48 , 49 , 50 , 51 , 52 , 53 ], and 10 studies in North America [ 54 , 55 , 56 , 57 , 58 , 59 , 60 , 61 , 62 , 63 ]. A total of 1280 patients with OC (most of the patients had OSCC) and 1254 healthy controls were included. The sample size of the included studies ranged from 9 to 100, with a mean age of 46 to 73 years. All the case–control studies enrolled both adult males and females. The enzyme-linked immunosorbent assay (ELISA) was the most often utilized detection method for determining the levels of salivary cytokines, followed by the Luminex-based immunoassay, the bead-based multiplex immunoassay, and the chemiluminescent enzyme immunoassay. For example, in the study by Piyarathne et al. [ 44 ], protein levels of ILs were quantified using a commercially available sandwich enzyme-linked immune-sorbent assay (ELISA), with pre-coated plates: E-EL-H0149, E-EL-H0102 and E-EL-H0048 kits (Elabscience; Wuhan, Hubei, China). In the study by Laliberté et al. [ 54 ], cytokines were analyzed according to the immunoassay protocol for the Millipore Human Cytokine/Chemokine Magnetic Bead Assay Panel (HCYTMAG-60K-PX30 by EMD Millipore, USA) and Luminex detection. In the study by Sato, J. et al. [ 42 ], IL-6 concentrations were measured using a highly sensitive chemiluminescent enzyme immunoassay (Fujirebio Inc., Tokyo, Japan).
Ten studies reported salivary IL-1β concentrations [ 25 , 35 , 36 , 44 , 47 , 53 , 54 , 55 , 57 , 60 ], 4 of which reported its sensitivity and specificity in the diagnosis of OC [ 25 , 36 , 44 , 60 ]. 24 studies reported salivary IL-6 concentrations [ 16 , 31 , 33 , 34 , 35 , 36 , 37 , 38 , 39 , 40 , 41 , 42 , 44 , 45 , 46 , 49 , 50 , 52 , 53 , 54 , 56 , 58 , 61 , 63 ], 5 studies reported sensitivity and specificity [ 16 , 36 , 44 , 46 , 47 ]. 20 studies reported salivary IL-8 concentrations [ 16 , 25 , 29 , 32 , 34 , 35 , 36 , 39 , 44 , 45 , 47 , 54 , 55 , 56 , 57 , 58 , 59 , 60 , 61 , 63 ] , 8 studies reported sensitivity and specificity [ 16 , 25 , 29 , 36 , 44 , 55 , 60 , 62 ]. 15 studies reported salivary TNF-α concentrations [ 16 , 26 , 27 , 28 , 29 , 34 , 36 , 45 , 48 , 52 , 53 , 54 , 58 , 61 , 63 ], 5 of which reported sensitivity and specificity [ 16 , 27 , 28 , 36 , 47 ]. 5 studies reported salivary IL-10 concentrations [ 36 , 43 , 48 , 51 , 54 ], but none of them investigated its sensitivity and specificity.
Quality assessment
The NOS scores are provided in Table 1 . The quality assessment results revealed that 1 study [ 31 ] was of high quality and the other 39 studies were of medium quality. Besides, the mean score of all included studies was 6.5. Table S3 in the Supplementary Materials illustrates the thorough point-by-point evaluation. For the risk of bias and applicability of diagnostic accuracy studies, we considered the overall risk of bias to be relatively low, and all included studies generated only low concern in all aspects (details in the Supplementary Figure S1). To be specific, there was a high risk of bias in participant selection due to case–control designs and inappropriate exclusions, with unclear consecutive sample of patients enrolled in some studies. In the index test assessment, 2 [ 16 , 44 ] out of 12 studies had a low risk of bias, 10 studies [ 25 , 27 , 28 , 29 , 36 , 46 , 47 , 55 , 60 , 62 ] were judged to be unclear. Regarding reference standard tests, all studies had a low risk of bias. For the flow and timing aspects, all studies demonstrated a low risk of bias in statements regarding the interval time between the reference test and the index test.
Meta -analysis for comparing saliva cytokines between OC patients and healthy controls
IL-6, as the most extensively investigated cytokine, was reported in 24 studies [ 16 , 31 , 33 , 34 , 35 , 36 , 37 , 38 , 39 , 40 , 42 , 44 , 45 , 46 , 49 , 52 , 53 , 54 , 56 , 58 , 61 , 62 , 63 , 64 ], including 708 OC cases and 652 controls. The meta-analysis suggested an obvious increase in salivary IL-6 levels in OC patients (SMD = 2.32, 95% CI (1.61, 3.03), p < 0.001). There was a high degree of heterogeneity (I 2 = 95.8%, Fig. 2 A). However, the sensitivity analysis did not find the source of heterogeneity (Supplementary Figure S2) Furthermore, subgroup analyses were conducted depending on patients’ age (> 60, ≤ 60, or not grouped), the assay kits used (ELISA, Luminex-based Multiplex immunoassay or chemiluminescent enzyme immunoassay), and the geographic locations among the included studies (Europe, north America, or Asia), and the results showed that patients’ age and the assay kits were the source of heterogeneity (> 60, I 2 = 0%, ≤ 60, I 2 = 90.7%; ELISA kit, I 2 = 96.5%; the Luminex-based Multiplex kit and the chemiluminescent enzyme kit, I 2 = 0%, details see Supplementary Table S4). Egger's test pointed to the evidence of publication bias across these 24 studies (p < 0.001).
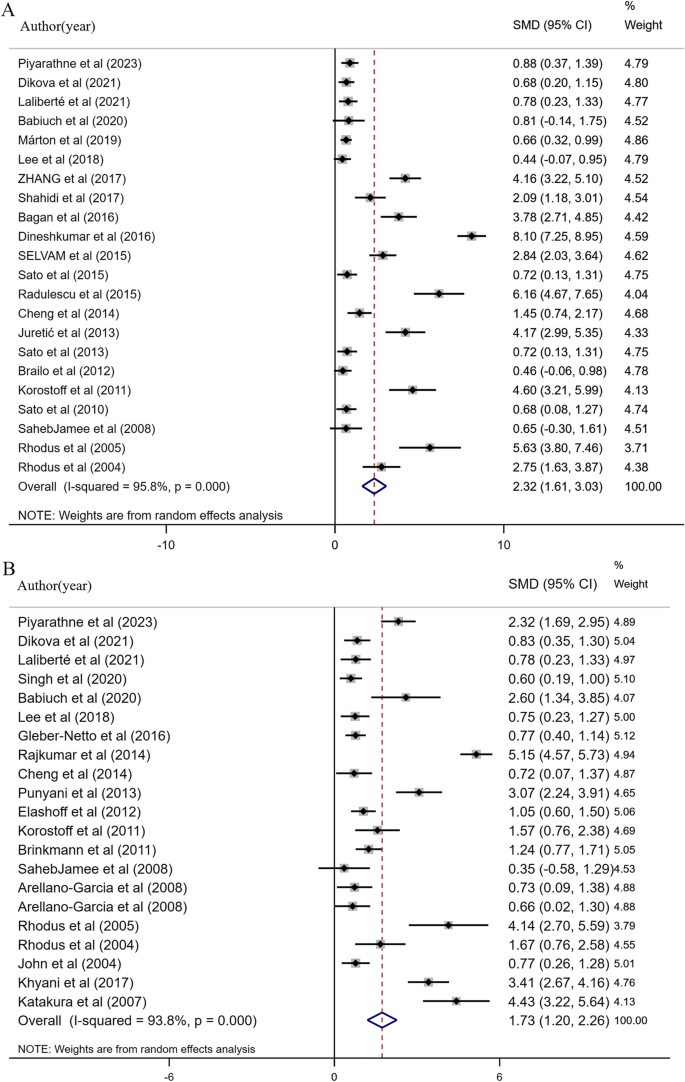
Forest plot of the saliva cytokine levels in OSCC patients versus healthy controls A IL-6 levels B IL-8 levels
The second most commonly studied cytokine was IL-8, which was investigated in 20 studies [ 16 , 25 , 29 , 32 , 34 , 35 , 36 , 39 , 44 , 45 , 54 , 55 , 56 , 57 , 58 , 59 , 60 , 61 , 62 , 65 ] encompassing 691 OC patients and 750 controls. According to the pooled analysis (Fig. 2 B), salivary IL-8 levels were found to be markedly increased in the OC population (SMD = 1.73, 95%CI [1.20, 2.26], p < 0.001). The heterogeneity between studies was significant (I 2 = 93.8%). The exclusion of individual studies did not alter the analysis results (Supplementary Figure S2). The results of subgroup analyses indicated that the assay kits were the source of heterogeneity (ELISA kit, I 2 = 94.9%; the Luminex-based Multiplex kit and the chemiluminescent enzyme kit, I 2 = 0%, details see Supplementary Table S4). Egger's test revealed significant publication bias (p = 0.03).
The salivary TNF-α level (Fig. 3 A) was discussed in 15 studies [ 16 , 26 , 27 , 29 , 34 , 36 , 45 , 48 , 52 , 53 , 54 , 58 , 61 , 62 , 65 ], covering 460 cases and 435 controls. The pooled analysis showed significantly elevated TNF-α levels in OSCC patients (SMD = 2.27, 95% CI (1.27, 3.26), p < 0.001). Heterogeneity was high (I 2 = 96.3%). Sensitivity analysis suggested that the exclusion of each study did not alter the pooled effect size (Supplementary Figure S2). Subgroup analyses showed that participants’ age and the assay kits were the source of heterogeneity (> 60, I 2 = 0%, ≤ 60, I 2 = 91.2%; ELISA kit, I 2 = 96.3%; the Luminex-based Multiplex kit, I 2 = 0%, Supplementary Table S4). Egger's tests (p = 0.065) revealed no publication bias.
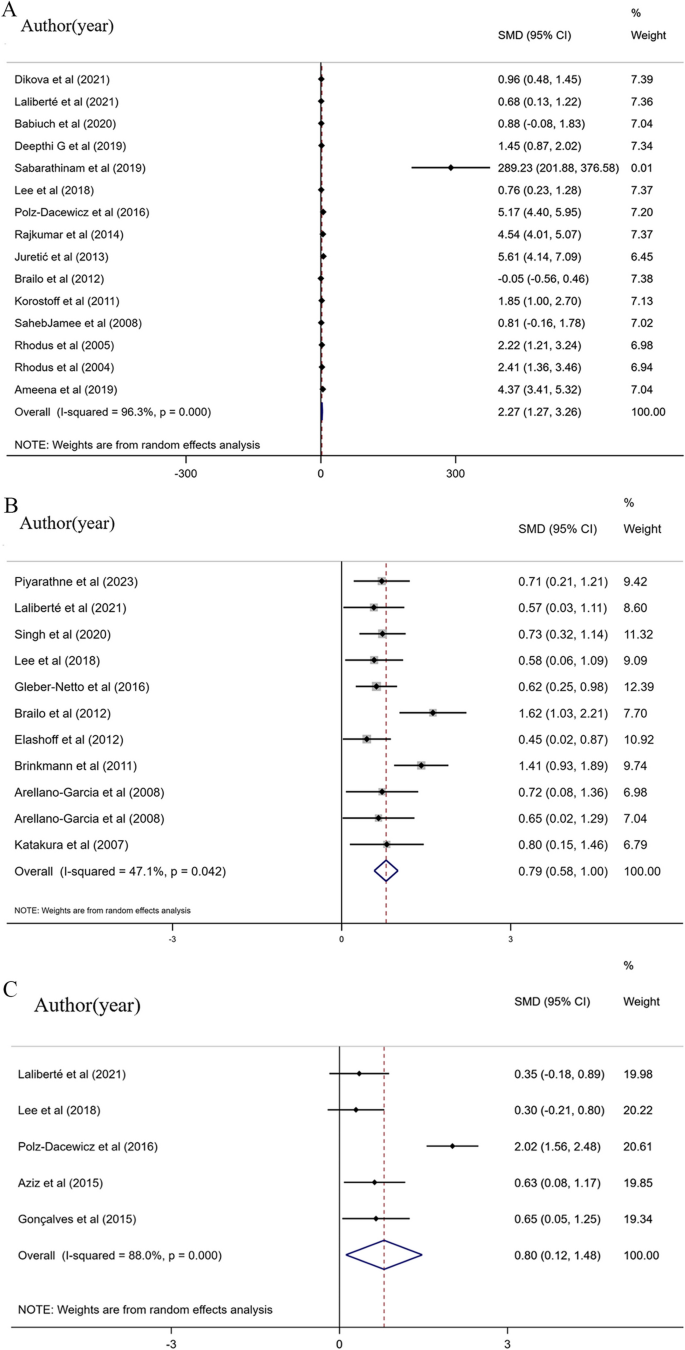
Forest plot of the salivary cytokine levels in OC patients versus healthy controls A TNF-α levels B IL-1βlevels C IL-10 levels
IL-1β was evaluated in 10 studies [ 25 , 35 , 36 , 44 , 53 , 54 , 55 , 57 , 59 , 60 ], including 381 OC cases and 392 controls. The meta-analysis found that OC patients exhibited a considerably higher IL-1β level in comparison to the healthy controls (SMD = 0.79, 95% CI (0.58, 1.00), p < 0.001, Fig. 3 B). Heterogeneity was low (I 2 = 47.1%). The funnel plot (Supplementary Figure S3) and the Egger’s test suggested no publication bias ( p = 0.393).
IL-10 levels were reported in 5 included studies [ 36 , 43 , 48 , 51 , 54 ], involving 99 OC cases and 139 controls. Higher levels of IL-10 were noted in OC patients in comparison to the control group (SMD = 0.80, 95% CI (0.12, 1.48), p = 0.022, Fig. 3 C). High heterogeneity was observed (I 2 = 88%). The sensitivity analysis demonstrated that leaving out any one study did not have an impact on the pooled results (Supplementary Figure S2), while the assay kits and the geographic locations were the source of heterogeneity (ELISA kit, I 2 = 92.0%; the Luminex-based Multiplex kit, I 2 = 0%; Asia, I 2 = 0%; Europe, I 2 = 92.0%, Supplementary Table S4). Egger's test and the funnel plot (Supplementary Figure S3) indicated no publication bias ( p = 0.231).
Diagnostic accuracy estimate
Since no studies have mentioned the sensitivity and specificity of IL-10 in the diagnosis of oral cancer only the remaining 4 kinds of saliva cytokines (i.e., IL-6, IL-8, TNF-α, and IL-1β) were included in this network meta-analysis. 12 studies [ 16 , 25 , 27 , 28 , 29 , 36 , 44 , 46 , 47 , 55 , 60 , 62 ] provided their sensitivity and specificity, involving 1058 participants, of whom 574 (54.3%) were OC patients. Among the included studies, 5 studies [ 16 , 36 , 44 , 46 , 47 ] assessed the diagnostic accuracy of IL-6 for OC, with the sensitivity varying from 0.75 to 1.00 and specificity from 0.49 to 0.80 (Fig. 4 ). The pooled sensitivity and specificity of IL-6 were 0.75 (95% CI: 0.71, 0.81) and 0.86 (95%CI: 0.82, 0.90), respectively (Table 2 ). 8 studies [ 16 , 25 , 29 , 36 , 44 , 55 , 60 , 62 ] assessed the diagnostic accuracy of IL-8 for OSCC, with the sensitivity varying from 0.67 to 0.97, and specificity from 0.58 to 0.97 (Fig. 4 ). The pooled sensitivity and specificity of IL-8 were 0.80 (95%CI: 0.77, 0.83) and 0.80 (95%CI: 0.77, 0.84), respectively (Table 2 ). 5 studies [ 16 , 27 , 28 , 36 , 47 ] assessed the diagnostic accuracy of TNF-α for OC, with the sensitivity varying from 0.83 to 1.00, and specificity from 0.49 to 1.00 (Fig. 4 ). The pooled sensitivity and specificity of TNF-α were 0.79 (95%CI: 0.76, 0.84) and 0.92 (95%CI: 0.90, 0.95) (Table 2 ), respectively. 4 studies [ 25 , 36 , 60 , 65 ] assessed the diagnostic accuracy of IL-1β for OC, with the sensitivity varying from 0.61 to 0.74, and specificity from 0.76 to 0.84 (Fig. 4 ). The pooled sensitivity and specificity of IL-1β were 0.66 (95%CI: 0.61, 0.72) and 0.75 (95%CI: 0.70, 0.81), respectively (Table 2 ).
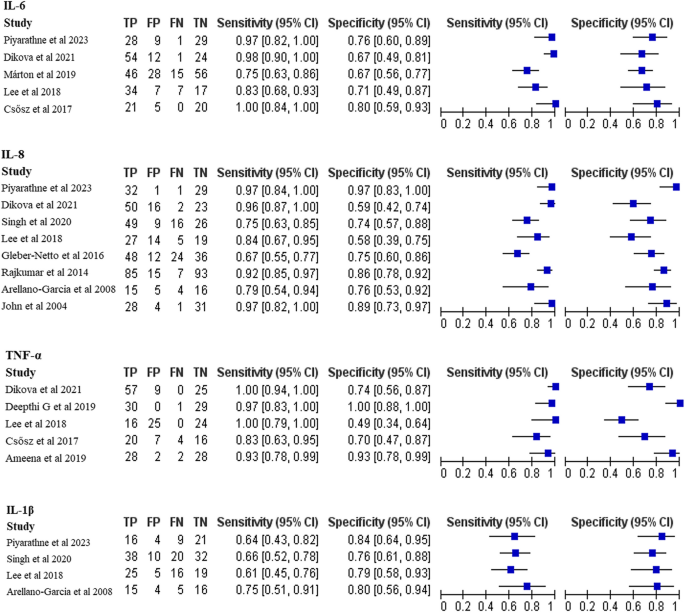
Forest plots for the diagnostic accuracy of saliva cytokines. TP true positive, FP false positive, FN false negative, TN true negative, CI confidence interval
The network plot for the diagnostic accuracy of salivary cytokines for OC is illustrated in Fig. 5 A. The NMA suggested that TNF-α ranked first, with the highest DOR (72.42, 95%CI: 34.00, 89.45), the second highest sensitivity (0.79, 95%CI: 0.76, 0.84), highest specificity (0.97, 95%CI: 0.69, 1.00), and the highest superiority index (Table 2 ). IL-6 ranked second, and the pooled sensitivity, specificity and DOR of IL-6 were 0.75 (95% CI: 0.71, 0.81), 0.86 (95% CI: 0.82, 0.90) and 25.13 (95% CI:13.48, 31.77), respectively, followed by IL-8 (sensitivity: 0.80, 95% CI: 0.77,0.83; specificity: 0.80, 95% CI: 0.77, 0.84; DOR: 19.09, 95% CI: 12.71, 23.33) and IL-1β (sensitivity: 0.66, 95% CI: 0.61, 0.72; specificity: 0.75, 95% CI: 0.70, 0.81; DOR: 7.57, 95% CI: 4.22, 9.42). Summary ROC results are presented in Fig. 5 B.
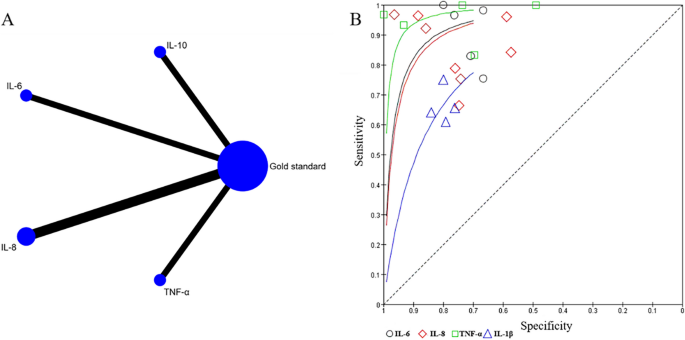
A Evidence network plot of diagnostic accuracy of saliva cytokines for OC B Summary ROC plot of saliva tests
A traditional meta-analysis was carried out based on all available evidence from 40 case–control studies to compare the levels of salivary IL-8, IL-6, IL-10, TNF-α, and IL-1β in OC patients versus controls. Our research indicated that OC patients exhibited considerably higher levels of salivary IL-8, IL-6, TNF-α, IL-1β and IL-10 than healthy controls. To our knowledge, this is the first network meta-analysis to delve into the diagnostic accuracy of these cytokines for OC. Our NMA found that IL-8 had the highest sensitivity (0.80), followed by TNF-α (0.79) and IL6 (0.75). TNF-α had the highest specificity (0.92), followed by IL6 (0.86) and IL8 (0.80). Overall, the DOR results indicated that TNF-α had the highest accuracy for the diagnosis of OC.
In the tumor microenvironment, there are a large number of cytokines, which inhibit tumor-specific immune response and promote the proliferation of tumor cells [ 66 , 67 ], thus contributing to the tumorigenesis and progression of tumors. Our results suggested that OC patients exhibited considerably higher levels of salivary IL-8, IL-6, TNF-α, IL-1β and IL-10 in comparison to healthy controls, which were consistent with previous studies [ 17 , 68 ]. Specifically, Rezaei's meta-analysis implied that levels of IL-6 and IL-8 in saliva were markedly elevated in OC patients [ 68 ]. The meta-analysis by Chiamulera demonstrated significantly higher levels of salivary IL-8, IL-6, TNF-α, IL-1β and IL-10 in OC patients [ 17 ]. It has been proposed that cytokines in oral chronic/acute inflammation recruit neutrophils to form a feedback loop with OC cells, resulting in a pro-tumor phenotype [ 15 ]. Our research further supports this finding, suggesting that these cytokines can be used as potential biomarkers for OC.
The main purpose of our study was to compare common saliva cytokines so as to identify the best cytokine for diagnosing OC. According to our results, TNF-α was the most accurate and ranked first with a specificity of 0.92 and a sensitivity of 0.79, and its DOR value was much higher than others. TNF-α, a member of the enormous TNF cytokine family, plays a key role in numerous physiological and pathological cellular processes, such as cell proliferation, differentiation, and death, regulation of immune response to various cells and molecules, local and vascular invasion of tumors, and destruction of the tumor vascular system [ 69 ]. In both in vitro and in vivo models, as well as in patients with OC, the upregulation of TNF-α has been shown to enhance cell proliferation, whereas its downregulation inhibits the proliferation and migration of tumors [ 70 , 71 ]. Moreover, elevated TNF-α in the OC tumor microenvironment has been reported to facilitate invasion through two mechanisms: (i) it fosters the pro-inflammatory and pro-invasive phenotype of OC cells; (ii) it acts as a paracrine mediator to promote the recruitment and activation of inflammatory cells [ 15 , 72 ]. Simultaneously, TNF-α gene polymorphisms are strongly associated with an elevated risk of oral pre-cancer [ 73 ]. Based on our results, TNF-α could be a preferred biomarker for the diagnosis of OSCC.
Furthermore, our NMA implied that IL-6 and IL-8 ranked second (sensitivity: 75%; specificity: 86%) and third (sensitivity: 80%; specificity: 77%), respectively, while IL-1β ranked last (sensitivity: 66%; specificity: 75%). The diagnostic performance of IL-10 was not analyzed because the included studies did not provide the specificity and sensitivity of IL-10 for the diagnosis of OC. Previous studies and the results of the present study have shown that the IL-6 level in saliva is significantly elevated in OC patients [ 17 ], and there is a statistical difference in the concentration of IL-6 between pre-cancer state and the normal population [ 31 , 63 ]. The concentration of IL-6 in saliva may be used as a biological marker for the early diagnosis of OC. For instance, an elevated IL-6 concentration indicates a higher probability of local OC recurrence [ 31 ]. Moreover, IL-6, as a member of the IL-6 cytokine family, is involved in the recruitment of neutrophils and macrophages, which is related to the pathogenesis of chronic inflammatory diseases. It not only contributes to the tumorigenesis and rapid progression of tumors, but also promotes the metastasis and spread of aggressive cancer cells [ 74 ]. Hence, IL-6 may serve as a major contributor to the occurrence and development of OC. IL-8, as a member of the CXC chemokine family, is a pro-inflammatory chemokine produced by immune cells under inflammatory conditions [ 75 ]. In the tumor microenvironment, IL-8 can not only enhance tumor cell proliferation or transformation into a migratory or stromal phenotype but also foster tumor angiogenesis or recruit additional immunosuppressive cells to the tumor, thereby promoting tumor progression [ 76 ]. In addition, cancer cells secrete IL-8, thus up-regulating the expression of matrix metalloproteinase-7 (MMP-7), which also contributes to OSCC invasion [ 77 ].
IL-1β stimulates the tyrosine phosphorylation of epidermal growth factor receptor (EGFR) through the chemokine ligand 1-receptor 2(CXCL1-CXCR2) axis, and regulates EGFR signal to promote the proliferation of dysplasia oral mucosa keratinocyte (DOK) and OSCC cells. However, a significant decrease in tyrosine phosphorylation of EGFR and a sharp decrease in DOK cell proliferation were observed by transfecting CXCL1-targeted short hairpin RNA (shRNA) with lentivirus or by using CXCR2 antagonists [ 78 ]. Lee et al. (2015) also find that IL-1β can promote the proliferation of DOK and OSCC cells, enhance the angiogenesis ability and the expression of epithelial-mesenchymal transition (EMT)-related genes Snail and Slug , and down-regulate expression of cadherin E, thereby resulting in OCC invasion and metastasis [ 79 ]. It is worth noting that IL-1β can activate the nuclear factor-κB (NF-κB) pathway and foster the expression and secretion of IL-6 and IL-8. As a powerful pro-inflammatory cytokine, IL-1β has been widely demonstrated to be upregulated in ovarian, lung, and gastrointestinal cancers, which are often associated with poor prognosis [ 80 ]. IL-10 is a representative anti-inflammatory and immunosuppressive factor that promotes immune escape of tumor cells [ 81 ]. A previous study has shown that in most OC samples, the expression of IL-10 is higher in tumor cells and stromal cells than in controls [ 82 ]. Based on our research results, IL6, IL8 and IL1β could be used as biomarkers of OC and serve as auxiliary diagnostic methods. Nonetheless, further studies are needed to explore the specific role of these cytokines in OSCC and investigate their diagnostic accuracy.
Strength, limitations, and Inspiration for future research
First of all, saliva detection provides a low-cost method for early diagnosis of oral cancer due to its advantages of non-invasive, convenient collection, processing and storage. And because saliva is in constant contact with oral lesions, it may be superior to blood or other body fluids. In our study, we found that several salivary cytokines (salivary cytokines) have strong diagnostic ability in oral cancer diagnosis. This suggests that in future studies, we can perform multiple cytokine tests on these three cytokines.
However, the study still has several limitations. The heterogeneity of the included studies was significant, similar to a previous meta-analysis [ 17 ]. As a result, the operating procedures for saliva collection, storage, and cytokine quantification should be standardized in the future. Additionally, a multicenter study with a larger sample size is warranted to rule out possible bias. Future studies should also consider some lifestyle factors, such as smoking and drinking, which may also influence IL levels (between cases and controls), although their influence may be wakened upon the occurrence of cancer [ 44 , 65 ]. Few studies provided data on the sensitivity and specificity of IL-10 in diagnosing OC, so no relevant NMA was conducted. Further studies are desired to provide a more accurate evaluation of IL-10 in the diagnosis of OC. Some included studies conducted subgroup analysis by the stages of OC [ 16 , 36 , 45 ], but the correlation between cancer stages and cytokines was not investigated in our analysis. Since the early diagnosis of OSCC is closely related to the clinical treatment and prognosis of patients, further research in this area is necessary.
Previous studies [ 6 ] observed that IL-8 and il-6 concentrations in OC patients were significantly higher than those in OPMD patients, and also significantly increased compared with healthy subjects. This suggests that the amount of the increase may distinguish between oral cancer and precancerous states, lichen planus, periodontitis, or other inflammatory and infectious diseases. We look forward to conducting more control studies in the future to compare oral cancer with potential oral malignancies, oral inflammatory diseases, and even systemic inflammatory states to further improve its specificity in the diagnosis of oral cancer.
In addition, many studies have demonstrated the effects of cytokines in the treatment of cancers [ 83 , 84 , 85 , 86 ], so these cytokines provide an insight into future clinical treatment of OC.
This study suggests that salivary cytokines can be used as potential biomarkers for early diagnosis of OC. Given its high diagnostic specificity and sensitivity, TNF-α is recommended, followed by IL-6 and IL-8. Notably, salivary cytokine levels may be affected by other factors, such as potential malignant states, chronic local inflammation, and autoimmune diseases. Therefore, it is necessary to further distinguish the diagnostic accuracy of these cytokines in different disease states and compare them with OC and different OC stages. At the same time, more standard operating procedures and large-scale multi-center studies are needed to reduce bias and heterogeneity. It is believed that the TNF-α, IL-6, and IL-8 saliva test is an affordable technique for early clinical diagnosis of OC, which can provide novel insights into the targeted therapy of OC.
Availability of data and materials
All data supporting the findings of this study are available within the paper and its Supplementary Materials.
Sung H, et al. Global Cancer Statistics 2020: GLOBOCAN Estimates of Incidence and Mortality Worldwide for 36 Cancers in 185 Countries. CA Cancer J Clin. 2021;71(3):209–49.
Article PubMed Google Scholar
Sand L, Jalouli J. Viruses and oral cancer. Is there a link? Microbes Infect. 2014;16(5):371–8.
Article CAS PubMed Google Scholar
Chamoli A, et al. Overview of oral cavity squamous cell carcinoma: Risk factors, mechanisms, and diagnostics. Oral Oncol. 2021;121: 105451.
Montero PH, Patel SG. Cancer of the oral cavity. Surg Oncol Clin N Am. 2015;24(3):491–508.
Article PubMed PubMed Central Google Scholar
Warnakulasuriya S. Global epidemiology of oral and oropharyngeal cancer. Oral Oncol. 2009;45(4–5):309–16.
Ferrari E, et al. Salivary Cytokines as Biomarkers for Oral Squamous Cell Carcinoma: A Systematic Review. Int J Mol Sci. 2021;22(13):6795.
Article CAS PubMed PubMed Central Google Scholar
Su YF, et al. Current Insights into Oral Cancer Diagnostics. Diagnostics (Basel). 2021;11(7):1287.
Chen XJ, et al. Nanotechnology: a promising method for oral cancer detection and diagnosis. J Nanobiotechnology. 2018;16(1):52.
Meleti M. Salivary biomarkers for diagnosis of systemic diseases and malignant tumors. A systematic review. Med Oral Patol Oral Cir Bucal. 2020;25(2):e299–e31.
CAS PubMed PubMed Central Google Scholar
Cristaldi M, et al. Salivary Biomarkers for Oral Squamous Cell Carcinoma Diagnosis and Follow-Up: Current Status and Perspectives. Front Physiol. 2019;10:1476.
Nisar S. Chemokine-Cytokine Networks in the Head and Neck Tumor Microenvironment. Int J Mol Sci. 2021;22(9):4584.
Chen Z, et al. Expression of proinflammatory and proangiogenic cytokines in patients with head and neck cancer. Clin Cancer Res. 1999;5(6):1369–79.
CAS PubMed Google Scholar
Chow LQM. Head and Neck Cancer. N Engl J Med. 2020;382(1):60–72.
Alevizos I, et al. Oral cancer in vivo gene expression profiling assisted by laser capture microdissection and microarray analysis. Oncogene. 2001;20(43):6196–204.
Goertzen C, et al. Oral inflammation promotes oral squamous cell carcinoma invasion. Oncotarget. 2018;9(49):29047–63.
Dikova V, Jantus-Lewintre E, Bagan J. Potential Non-Invasive Biomarkers for Early Diagnosis of Oral Squamous Cell Carcinoma. J Clin Med. 2021;10(8):1658.
Chiamulera MMA, et al. Salivary cytokines as biomarkers of oral cancer: a systematic review and meta-analysis. BMC Cancer. 2021;21(1):205.
O’Sullivan JW. Network meta-analysis for diagnostic tests. BMJ Evid Based Med. 2019;24(5):192–3.
Veroniki AA, et al. Diagnostic test accuracy network meta-analysis methods: A scoping review and empirical assessment. J Clin Epidemiol. 2022;146:86–96.
Moher D, et al. Preferred reporting items for systematic reviews and meta-analyses: the PRISMA statement. BMJ. 2009;339: b2535.
Stang A. Critical evaluation of the Newcastle-Ottawa scale for the assessment of the quality of nonrandomized studies in meta-analyses. Eur J Epidemiol. 2010;25(9):603–5.
Whiting PF, et al. QUADAS-2: a revised tool for the quality assessment of diagnostic accuracy studies. Ann Intern Med. 2011;155(8):529–36.
Ma X, et al. A Bayesian hierarchical model for network meta-analysis of multiple diagnostic tests. Biostatistics. 2018;19(1):87–102.
Nyaga VN, Aerts M, Arbyn M. ANOVA model for network meta-analysis of diagnostic test accuracy data. Stat Methods Med Res. 2018;27(6):1766–84.
Singh P, Verma JK, Singh JK. Validation of Salivary Markers, IL-1β, IL-8 and Lgals3bp for Detection of Oral Squamous Cell Carcinoma in an Indian Population. Sci Rep. 2020;10(1):7365.
Sabarathinam J, Selvaraj J, Devi S. Estimation of Levels of Glutathione Peroxidase (Gpx), Malondialdehyde (Mda), Tumor Necrosis Factor Alpha (Tnf Alpha) and Alpha Feto Protein (Afp) In Saliva of Potentially Malignant Disorders and Oral Squamous Cell Carcinoma. Biomed Pharmacol J. 2019;12(4):1881–6.
Article CAS Google Scholar
Deepthi G, Nandan SRK, Kulkarni PG. Salivary Tumour Necrosis Factor-α as a Biomarker in Oral Leukoplakia and Oral Squamous Cell Carcinoma. Asian Pac J Cancer Prev. 2019;720(7):2087–93.
Google Scholar
Ameena M, Rathy R. Evaluation of tumor necrosis factor: Alpha in the saliva of oral cancer, leukoplakia, and healthy controls - A comparative study. Journal of International Oral Health. 2019;11(2):92–9.
Article Google Scholar
Rajkumar K, et al. Validation of the diagnostic utility of salivary interleukin 8 in the differentiation of potentially malignant oral lesions and oral squamous cell carcinoma in a region with high endemicity. Oral Surg Oral Med Oral Pathol Oral Radiol. 2014;118(3):309–19.
Krishnan R, et al. Association of serum and salivary tumor necrosis factor-α with histological grading in oral cancer and its role in differentiating premalignant and malignant oral disease. Asian Pac J Cancer Prev. 2014;15(17):7141–8.
Sato J, et al. Correlation between salivary interleukin-6 levels and early locoregional recurrence in patients with oral squamous cell carcinoma: preliminary study. Head Neck. 2013;35(6):889–94.
Punyani SR, Sathawane RS. Salivary level of interleukin-8 in oral precancer and oral squamous cell carcinoma. Clin Oral Investig. 2013;17(2):517–24.
Sato J, et al. Changes in saliva interleukin-6 levels in patients with oral squamous cell carcinoma. Oral Surg Oral Med Oral Pathol Oral Radiol Endod. 2010;110(3):330–6.
SahebJamee M, et al. Salivary concentration of TNFalpha, IL1 alpha, IL6, and IL8 in oral squamous cell carcinoma. Med Oral Patol Oral Cir Bucal. 2008;13(5):E292–5.
PubMed Google Scholar
Katakura A, et al. Comparison of salivary cytokine levels in oral cancer patients and healthy subjects. Bull Tokyo Dent Coll. 2007;48(4):199–203.
Lee LT, et al. Evaluation of saliva and plasma cytokine biomarkers in patients with oral squamous cell carcinoma. Int J Oral Maxillofac Surg. 2018;47(6):699–707.
Zhang S, et al. Variation and significance of secretory immunoglobulin A, interleukin 6 and dendritic cells in oral cancer. Oncol Lett. 2017;13(4):2297–303.
Shahidi, M, et al. Predictive value of salivary microRNA-320a, vascular endothelial growth factor receptor 2, CRP and IL-6 in Oral lichen planus progression . Inflammopharmacology. 2017.
Khyani IAM, et al. Detection of interleukins-6 and 8 in saliva as potential biomarkers of oral pre-malignant lesion and oral carcinoma: A breakthrough in salivary diagnostics in Pakistan. Pak J Pharm Sci. 2017;30(3):817–23.
Dineshkumar T, et al. Salivary and Serum Interleukin-6 Levels in Oral Premalignant Disorders and Squamous Cell Carcinoma: Diagnostic Value and Clinicopathologic Correlations. Asian Pac J Cancer Prev. 2016;17(11):4899–906.
PubMed PubMed Central Google Scholar
Panneer Selvam N, Sadaksharam J. Salivary interleukin-6 in the detection of oral cancer and precancer. Asia Pac J Clin Oncol. 2015;11(3):236–41.
Sato J, et al. Differences in sequential posttreatment salivary IL-6 levels between patients with and patients without locoregional recurrences of oral squamous cell carcinoma: Part III of a cohort study. Oral Surg Oral Med Oral Pathol Oral Radiol. 2015;120(6):751–60.e2.
Aziz S, et al. Salivary Immunosuppressive Cytokines IL-10 and IL-13 Are Significantly Elevated in Oral Squamous Cell Carcinoma Patients. Cancer Invest. 2015;33(7):318–28.
Piyarathne NS, et al. Salivary Interleukin Levels in Oral Squamous Cell Carcinoma and Oral Epithelial Dysplasia: Findings from a Sri Lankan Study. Cancers (Basel). 2023;15(5):1510.
Babiuch K, et al. Evaluation of Proinflammatory, NF-kappaB Dependent Cytokines: IL-1α, IL-6, IL-8, and TNF-α in Tissue Specimens and Saliva of Patients with Oral Squamous Cell Carcinoma and Oral Potentially Malignant Disorders. J Clin Med. 2020;9(3):867.
Márton, IJ. et al. Salivary IL-6 mRNA is a Robust Biomarker in Oral Squamous Cell Carcinoma . J Clin Med. 2019;8(11):1958.
Csősz É, et al. Proteomics investigation of OSCC-specific salivary biomarkers in a Hungarian population highlights the importance of identification of population-tailored biomarkers. PLoS ONE. 2017;12(5): e0177282.
Polz-Dacewicz M, et al. Salivary and serum IL-10, TNF-α, TGF-β, VEGF levels in oropharyngeal squamous cell carcinoma and correlation with HPV and EBV infections. Infect Agent Cancer. 2016;11:45.
Bagan L, et al. Salivary and serum interleukin-6 levels in proliferative verrucous leukoplakia. Clin Oral Investig. 2016;20(4):737–43.
Radulescu R, et al. Biomarkers of Oxidative Stress, Proliferation, Inflammation and Invasivity in Saliva from Oral Cancer Patients. Journal of Analytical Oncology. 2015;4:52–7.
Gonçalves AS, et al. Immunosuppressive mediators of oral squamous cell carcinoma in tumour samples and saliva. Hum Immunol. 2015;76(1):52–8.
Juretić M, et al. Salivary levels of TNF-α and IL-6 in patients with oral premalignant and malignant lesions. Folia Biol (Praha). 2013;59(2):99–102.
Brailo V, et al. Salivary and serum interleukin 1 beta, interleukin 6 and tumor necrosis factor alpha in patients with leukoplakia and oral cancer. Med Oral Patol Oral Cir Bucal. 2012;17(1):e10–5.
Laliberté C, et al. Characterization of Oral Squamous Cell Carcinoma Associated Inflammation: A Pilot Study. Front Oral Health. 2021;2: 740469.
Gleber-Netto FO, et al. Salivary Biomarkers for Detection of Oral Squamous Cell Carcinoma in a Taiwanese Population. Clin Cancer Res. 2016;22(13):3340–7.
Lisa Cheng YS, et al. Salivary interleukin-6 and -8 in patients with oral cancer and patients with chronic oral inflammatory diseases. J Periodontol. 2014;85(7):956–65.
Elashoff D, et al. Prevalidation of salivary biomarkers for oral cancer detection. Cancer Epidemiol Biomarkers Prev. 2012;21(4):664–72.
Korostoff A, et al. The role of salivary cytokine biomarkers in tongue cancer invasion and mortality. Oral Oncol. 2011;47(4):282–7.
Brinkmann O, et al. Oral squamous cell carcinoma detection by salivary biomarkers in a Serbian population. Oral Oncol. 2011;47(1):51–5.
Arellano-Garcia ME, et al. Multiplexed immunobead-based assay for detection of oral cancer protein biomarkers in saliva. Oral Dis. 2008;14(8):705–12.
Rhodus NL, et al. The feasibility of monitoring NF-kappaB associated cytokines: TNF-alpha, IL-1alpha, IL-6, and IL-8 in whole saliva for the malignant transformation of oral lichen planus. Mol Carcinog. 2005;44(2):77–82.
St John MA, et al. Interleukin 6 and interleukin 8 as potential biomarkers for oral cavity and oropharyngeal squamous cell carcinoma. Arch Otolaryngol Head Neck Surg. 2004;130(8):929–35.
Rhodus NL, et al. NF-kappaB dependent cytokine levels in saliva of patients with oral preneoplastic lesions and oral squamous cell carcinoma. Cancer Detect Prev. 2005;29(1):42–5.
Selvam NP, Sadaksharam J. Salivary interleukin-6 in the detection of oral cancer and precancer. Asia Pac J Clin Oncol. 2015;11(3):236–41.
Piyarathne NS, et al. Diagnostic salivary biomarkers in oral cancer and oral potentially malignant disorders and their relationships to risk factors - A systematic review. Expert Rev Mol Diagn. 2021;21(8):789–807.
Galdiero MR., Marone G, Mantovani A. Cancer Inflammation and Cytokines. Cold Spring Harb Perspect Biol,. 2018;10(8):a028662.
Li L, et al. Effects of immune cells and cytokines on inflammation and immunosuppression in the tumor microenvironment. Int Immunopharmacol. 2020;88: 106939.
Rezaei F, et al. Evaluation of Serum and Salivary Interleukin-6 and Interleukin-8 Levels in Oral Squamous Cell Carcinoma Patients: Systematic Review and Meta-Analysis. J Interferon Cytokine Res. 2019;39(12):727–39.
Mahdavi Sharif P, et al. Importance of TNF-alpha and its alterations in the development of cancers. Cytokine. 2020;130: 155066.
Sun Z, et al. Effect of interleukin-1β and tumor necrosis factor α gene silencing on mouse gastric cancer cell proliferation and migration. Oncol Lett. 2016;11(4):2559–65.
Ho MY, et al. TNF-α induces epithelial-mesenchymal transition of renal cell carcinoma cells via a GSK3β-dependent mechanism. Mol Cancer Res. 2012;10(8):1109–19.
Glogauer JE, et al. Neutrophils Increase Oral Squamous Cell Carcinoma Invasion through an Invadopodia-Dependent Pathway. Cancer Immunol Res. 2015;3(11):1218–26.
Serefoglou Z, et al. Genetic association of cytokine DNA polymorphisms with head and neck cancer. Oral Oncol. 2008;44(12):1093–9.
Jones SA, Jenkins BJ. Recent insights into targeting the IL-6 cytokine family in inflammatory diseases and cancer. Nat Rev Immunol. 2018;18(12):773–89.
Matsushima K, Yang D, Oppenheim JJ. Interleukin-8: An evolving chemokine. Cytokine. 2022;153: 155828.
Fousek K, Horn LA, Palena C. Interleukin-8: A chemokine at the intersection of cancer plasticity, angiogenesis, and immune suppression. Pharmacol Ther. 2021;219: 107692.
Watanabe H, et al. Role of interleukin-8 secreted from human oral squamous cell carcinoma cell lines. Oral Oncol. 2002;38(7):670–9.
Lee CH, et al. Interleukin-1 beta transactivates epidermal growth factor receptor via the CXCL1-CXCR2 axis in oral cancer. Oncotarget. 2015;6(36):38866–80.
Lee CH, et al. IL-1β promotes malignant transformation and tumor aggressiveness in oral cancer. J Cell Physiol. 2015;230(4):875–84.
Zhang D, et al. Association of IL-1beta gene polymorphism with cachexia from locally advanced gastric cancer. BMC Cancer. 2007;7:45.
Kondoh N, et al. Immunomodulatory aspects in the progression and treatment of oral malignancy. Jpn Dent Sci Rev. 2019;55(1):113–20.
Arantes DA, et al. Overexpression of immunosuppressive cytokines is associated with poorer clinical stage of oral squamous cell carcinoma. Arch Oral Biol. 2016;61:28–35.
Nguyen KG, et al. Localized Interleukin-12 for Cancer Immunotherapy. Front Immunol. 2020;11: 575597.
Jaén M, et al. Interleukin 13 receptor alpha 2 (IL13Rα2): Expression, signaling pathways and therapeutic applications in cancer. Biochim Biophys Acta Rev Cancer. 2022;1877(5): 188802.
Raeber ME, Sahin D, Boyman O. Interleukin-2-based therapies in cancer. Sci Transl Med. 2022;14(670):eabo5409.
Han Y, et al. IL-1β-associated NNT acetylation orchestrates iron-sulfur cluster maintenance and cancer immunotherapy resistance. Mol Cell. 2023;83(11):1887–1902.e8.
Download references
Acknowledgements
Not applicable.
1. Changsha Natural Science Foundation. (No: kq2208484).
2. Research Program Project of Hunan Provincial Health and Wellness Commission. (No: 202108030155).
Author information
Authors and affiliations.
Department of Orthodontics, Changsha Stomatological Hospital, Changsha Hunan, 410006, China
Lijun Huang, Fen Luo, Mingsi Deng & Jie Zhang
School of Stomatology, Hunan University of Traditional Chinese Medicine, Changsha Hunan, 410208, China
You can also search for this author in PubMed Google Scholar
Contributions
All authors contributed to the study conception and design. Lijun Huang: Conceptualization, Methodology, Software, Writing- Original draft, Data curation, Visualization were performed; Fen Luo: Investigation, Writing - Original Draft, Writing – Reviewing and Editing, Funding acquisition were performed; Mingsi Deng: Methodology, Software, Writing- Original draft were performed; Jie Zhang: Conceptualization, Supervision, Project administration, Funding acquisition were performed. All authors read and approved the final manuscript.
Corresponding author
Correspondence to Jie Zhang .
Ethics declarations
Ethics approval and consent to participate, consent for publication, competing interests.
The authors declare no competing interests.
Additional information
Publisher's note.
Springer Nature remains neutral with regard to jurisdictional claims in published maps and institutional affiliations.
Supplementary Information
Supplementary material 1., rights and permissions.
Open Access This article is licensed under a Creative Commons Attribution-NonCommercial-NoDerivatives 4.0 International License, which permits any non-commercial use, sharing, distribution and reproduction in any medium or format, as long as you give appropriate credit to the original author(s) and the source, provide a link to the Creative Commons licence, and indicate if you modified the licensed material. You do not have permission under this licence to share adapted material derived from this article or parts of it. The images or other third party material in this article are included in the article’s Creative Commons licence, unless indicated otherwise in a credit line to the material. If material is not included in the article’s Creative Commons licence and your intended use is not permitted by statutory regulation or exceeds the permitted use, you will need to obtain permission directly from the copyright holder. To view a copy of this licence, visit http://creativecommons.org/licenses/by-nc-nd/4.0/ .
Reprints and permissions
About this article
Cite this article.
Huang, L., Luo, F., Deng, M. et al. The relationship between salivary cytokines and oral cancer and their diagnostic capability for oral cancer: a systematic review and network meta-analysis. BMC Oral Health 24 , 1044 (2024). https://doi.org/10.1186/s12903-024-04840-3
Download citation
Received : 06 December 2023
Accepted : 29 August 2024
Published : 05 September 2024
DOI : https://doi.org/10.1186/s12903-024-04840-3
Share this article
Anyone you share the following link with will be able to read this content:
Sorry, a shareable link is not currently available for this article.
Provided by the Springer Nature SharedIt content-sharing initiative
- Oral cancer
- Network meta-analysis
BMC Oral Health
ISSN: 1472-6831
- General enquiries: [email protected]
- Open access
- Published: 31 August 2024
Modified thoracoabdominal nerve block via perichondral approach: an alternative for perioperative pain management in laparoscopic cholecystectomy in a middle-income country
- Luisa Fernanda Castillo-Dávila 1 ,
- Carlos Jesús Torres-Anaya 1 ,
- Raquel Vazquez-Apodaca 1 ,
- Hector Borboa-Olivares 2 ,
- Salvador Espino-y-Sosa 3 &
- Johnatan Torres-Torres 3
BMC Anesthesiology volume 24 , Article number: 304 ( 2024 ) Cite this article
Metrics details
Laparoscopic cholecystectomy is known for its minimally invasive nature, but postoperative pain management remains challenging. Despite the enhanced recovery after surgery (ERAS) protocol, regional analgesic techniques like modified perichondral approach to thoracoabdominal nerve block (M-TAPA) show promise. Our retrospective study evaluates M-TAPA’s efficacy in postoperative pain control for laparoscopic cholecystectomy in a middle-income country.
This was a retrospective case-control study of laparoscopic cholecystectomy patients at Hospital General de Mexico in which patients were allocated to the M-TAPA or control group. The data included demographic information, intraoperative variables, and postoperative pain scores. M-TAPA blocks were administered presurgery. Outcomes: opioid consumption, pain intensity, adverse effects, and time to rescue analgesia. Analysis of variance (ANOVA) compared total opioid consumption between groups, while Student’s t test compared pain intensity and time until the first request for rescue analgesia.
Among the 56 patients, those in the M-TAPA group had longer surgical and anesthetic times ( p < 0.001), higher ASA 3 scores (25% vs. 3.12%, p = 0.010), and reduced opioid consumption ( p < 0.001). The M-TAPA group exhibited lower postoperative pain scores ( p < 0.001), a lower need for rescue analgesia ( p = 0.010), and a lower incidence of nausea/vomiting ( p = 0.010).
Bilateral M-TAPA offers effective postoperative pain control after laparoscopic cholecystectomy, especially in middle-income countries, by reducing opioid use and enhancing recovery.
Peer Review reports
Introduction
Laparoscopic cholecystectomy is a cornerstone procedure in modern surgery and is renowned for its minimally invasive approach and swift recovery compared to open surgery [ 1 ]. However, managing postoperative pain, which can range from moderate to severe within the first 24 h, remains a clinical challenge [ 2 ]. This pain, driven primarily by somatic components followed by neuropathic and shoulder-referred pain, exhibits considerable variability among patients [ 3 ].
To address this challenge, the enhanced recovery after surgery (ERAS) protocol advocates for a multimodal analgesic strategy [ 4 ]. However, the efficacy of nonopioid analgesics and adjuvant anesthetics has limitations, spurring exploration of regional analgesic techniques such as ultrasound-guided nerve blocks or local infiltration [ 5 , 6 ].
By targeting the anterolateral abdominal wall innervated by the thoracoabdominal nerves, the modified perichondral approach to thoracoabdominal nerve block (M-TAPA) has emerged as a promising analgesic modality [ 7 , 8 , 9 ]. M-TAPA, introduced by Tulgar et al. in 2019, offers broader coverage of both anterior and lateral cutaneous branches of the thoracoabdominal nerves, surpassing the limitations of conventional techniques such as the transversus abdominis plane block [ 10 ]. While initial studies have demonstrated its efficacy in abdominal surgeries, including laparoscopic cholecystectomy, further research is needed to establish its clinical utility and compare it with existing regional analgesic methods [ 11 , 12 ].
Healthcare in middle-income countries faces considerable challenges due to resource limitations and underdeveloped healthcare infrastructure. These nations often lack access to advanced treatments and pain management techniques, underscoring the importance of developing effective and economically viable approaches to address perioperative pain. In this context, our study aimed to assess the efficacy of M-TAPA for postoperative pain management compared to that of conventional analgesia in laparoscopic cholecystectomy patients in a middle-income country.
Study design and participants
This retrospective case-control study involved adult patients who underwent laparoscopic cholecystectomy at the Hospital General de Mexico “Dr. Eduardo Liceaga” in Mexico City from January 2023 to July 2023. Patients were retrospectively categorized into two groups based on the analgesic technique they received during surgery: those who received M-TAPA were assigned to the M-TAPA group, while those who received local infiltration were allocated to the control group. The study was conducted with the approval of the institutional research and bioethics boards at the Hospital General de Mexico “Dr. Eduardo Liceaga” (Approval number: 1384 − 295/23). Exclusion criteria included allergies to local anesthetics or contraindications to nerve block procedures.
Data collection
The data, including demographic information; intraoperative variables such as surgical and anesthetic duration; intraoperative opioid consumption; and postoperative pain scores assessed using the visual analog scale (VAS) [ 13 , 14 ], which ranges from 0 to 10, at awake, 30, and 120 min postoperatively, were extracted from the patients’ medical records. Each of these variables of interest was then transferred to an electronic database for analysis. Adverse effects related to analgesia, such as nausea and vomiting, were also recorded. Furthermore, our analysis incorporated variables such as time to mobilization and time to complete oral intake to assess postoperative recovery. Additionally, the time until the first request for rescue analgesia was documented as a measure of postoperative pain management efficacy.
Anesthesia and perioperative management protocol
No preoperative premedication was administered to the patients. The anesthesia technique was standardized for all patients in the operating room, involving electrocardiography, non-invasive blood pressure monitoring, capnography, peripheral oxygen saturation, neuromonitoring, a multi-gas analyzer, and temperature monitoring, alongside the initiation of a 0.9% NaCl infusion at a rate of 4 ml/kg.
Anesthesia induction comprised propofol (1.2 mg/kg), rocuronium (0.6 mg/kg), and fentanyl (4 mcg/kg) based on ideal body weight. Following endotracheal intubation, maintenance anesthesia involved a blend of 2% sevoflurane and 50% air in 50% O2 (3.5 L/min). Mechanical ventilation was executed in volume-controlled mode, with tidal volume set at 6–8 ml/kg according to ideal body weight to maintain end-tidal carbon dioxide at 30 to 35 mm Hg.
During surgery, anesthesia depth was regulated using end-tidal sevoflurane, maintaining sevoflurane concentration at 0.8-1 MAC.
In the M-TAPA group, the regional blockade was bilaterally administered by a single anesthesiologist post-general anesthesia induction and pre-surgical procedure. Sevoflurane maintained anesthesia and in most cases as heart rate and blood pressure values remained within a 20% variation, there was no need for additional boluses of fentanyl (1 mcg/kg).
In the control group, intraoperative management involved fentanyl infusion titration, supplemented in some cases with dexmedetomidine or lidocaine infusion, while ensuring non-invasive blood pressure and heart rate remained within 20% variation.
Patients were transferred to the post-anesthesia care unit upon completion of surgery, once neuromuscular functions were fully restored, and adequate tidal volume was observed.
Standard analgesia comprised intravenous administration of paracetamol 1 g every 8 h, metamizole 1 g every 8 h, or ketorolac 60 mg every 8 h. The initial doses of paracetamol and metamizol or ketorolac were administered in the last 30 min of surgery. Additionally, 100 mg of tramadol was intravenously administered to all patients in the no-block group before surgery termination. Pain intensity was assessed using a 0–10 numeric rating scale (NRS) at rest and during movement. Tramadol 50–100 mg was administered intravenously as a rescue analgesic to patients with a resting NRS > 4.
Description of the M-TAPA block technique
After administering anesthesia and before surgery began, an experienced anesthesiologist performed the M-TAPA block while the patient laid on their back. Using a high-frequency linear transducer (7–14 MHz, SonoSite M-Turbo), the anesthesiologist located the external, internal, and transverse abdominal muscles near the tenth rib cartilage, aiming to clearly visualize the underside of the cartilage.
Once the injection site was prepped, a 22G x 100 mm peripheral nerve block needle (Stimuplex ® Ultra 360 ® , B-Braun, USA) was carefully guided toward the underside of the tenth rib cartilage between the internal oblique and transverse abdominal muscles using real-time ultrasound imaging. After confirming the correct placement of the saline solution and ensuring that there was no blood aspiration, 20 ml of 0.375% ropivacaine was injected, ensuring effective anesthetic spread between the muscle layers. The same process was performed bilaterally. All blocks were conducted by an experienced anesthesiologist [ 12 , 15 ].
The primary outcome measure was total opioid consumption during the first postoperative hour. The secondary outcomes included postoperative pain intensity, incidence of adverse effects related to analgesia, and time to the first request for rescue analgesia.
Statistical analysis
Continuous variables are expressed as medians and interquartile ranges (IQRs), and inferential testing was performed using the Mann‒Whitney U test; categorical data are expressed as numbers and percentages and were analyzed using the chi‒square test or Fisher’s exact test. Analysis of variance (ANOVA) was used to compare total opioid consumption between groups, and Student’s t test was used to compare pain intensity and time until the first request for rescue analgesia. A p -value of less than 0.05 was considered to indicate statistical significance. (StataCorp. 2020, Stata Statistical Software: Release 17. College Station, TX: StataCorp LLC).
Characteristics of the study population
A total of 56 patients were analyzed in this study, with a predominance of 69.6% females and 30.4% males. The mean age of the patients was 38.18 ± 9.57 years. Clinical characteristics such as age, sex, and body mass index were significantly similar between the study groups ( Table 1 ) . However, compared with those in the control group, a significantly greater proportion of patients in the M-TAPA group underwent ASA 3 assessment (25% vs. 3.12%, p = 0.010).
Characteristics of the surgical event
Surgical and anesthetic times were significantly longer in the M-TAPA group than in the control group (119.75 versus 81.25, p = 0.0004 and 153.25 versus 107.06, p = 0.0001, respectively). Additionally, there was a greater use of anesthetic adjuvants in the control group (8.33% versus 78.12%, p = 0.0001), as did increased opioid consumption (341.58 versus 577.53, p = 0.0001) ( Tables 1 and Fig. 1 ) .
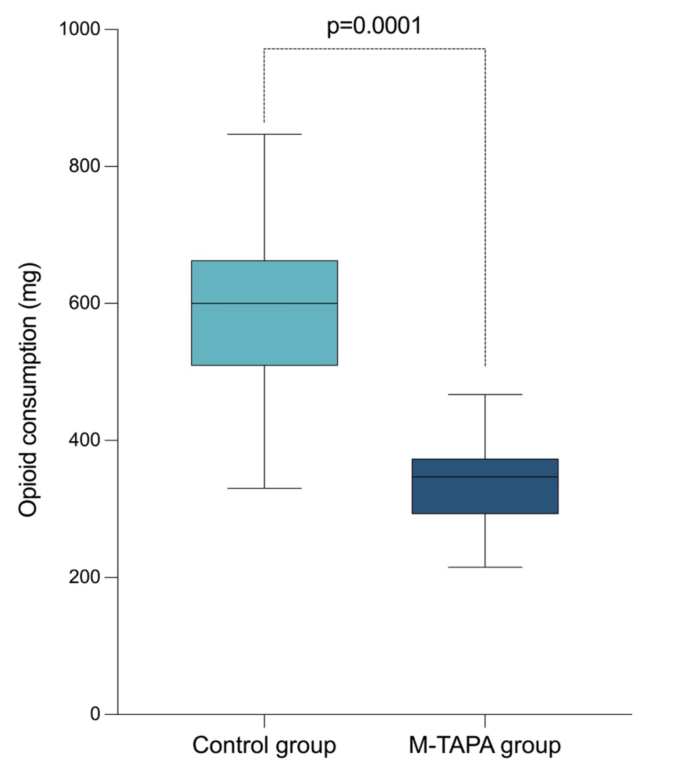
Opioid consumption between groups
Postoperative evaluation between groups
There were no significant differences in the VAS scores at awakening or 30 min after the operation between the study groups ( Table 2 ) . However, upon recovery, the M-TAPA group exhibited significantly lower pain scores than did the control group (1.25 versus 3.88, p = 0.0001) ( Fig. 2 ) . In Fig. 3 , the Kaplan-Meier survival curve compares the probability of patients in the control group and the M-TAPA group requiring tramadol rescue analgesia over time post-surgery. The log-rank test indicated a statistically significant difference between the survival curves (χ² =21.30, df = 1, p < 0.0001), demonstrating a longer duration until rescue analgesia in the M-TAPA group compared to the control group. This finding underscores the efficacy of M-TAPA in providing prolonged pain relief following laparoscopic cholecystectomy. Additionally, the hazard ratio (HR) calculated using the Mantel-Haenszel method was 8.333 (95%CI: 3.973 to 17.48), indicating a significantly lower risk of requiring rescue analgesia in the M-TAPA group compared to the control group. Furthermore, consistent with these survival analysis results, there was a reduced need for rescue analgesia with tramadol at 60 min post-surgery in the M-TAPA group compared to the control group (16.67% versus 50%, p = 0.010). A greater incidence of nausea and/or vomiting was recorded in the control group than in the M-TAPA group (15.62% versus 0, p = 0.010) ( Table 2 ) . Furthermore, analysis revealed that the M-TAPA group had significantly shorter times for early mobilization (8.2 h versus 10.6 h, p = 0.010), and quicker resumption of oral intake (17.3 h versus 24.7 h, p = 0.001) compared to the control group.
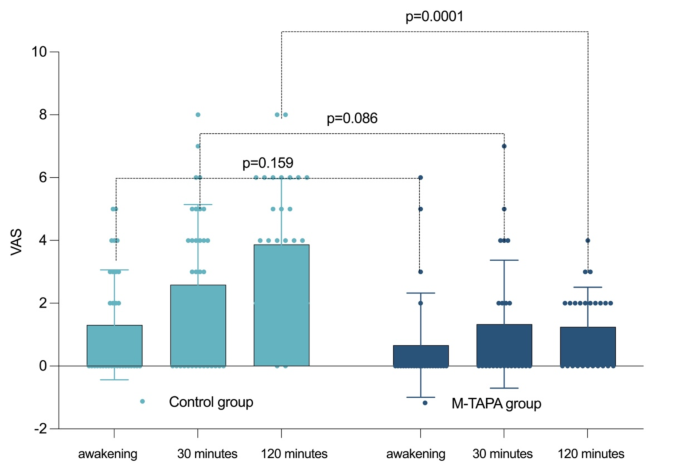
Postoperative pain assessment in the recovery area
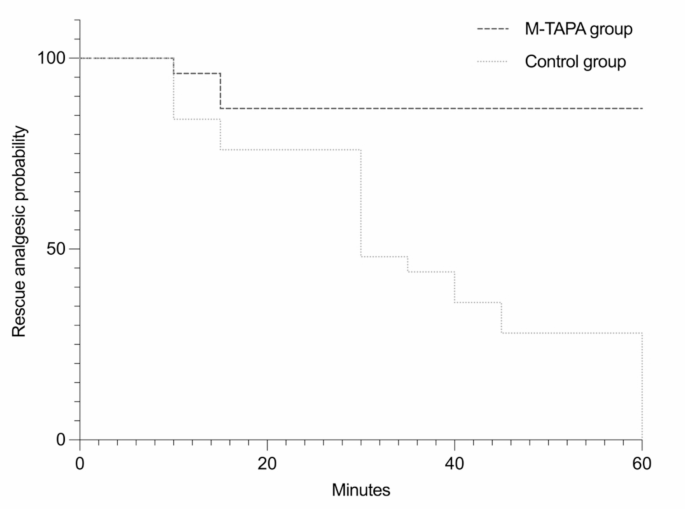
Depicts a Kaplan-Meier survival curve illustrating the time to rescue analgesia following laparoscopic cholecystectomy, with a focus on the need for tramadol rescue analgesia at 60 min post-surgery
The findings of our study underscore the efficacy of the M-TAPA block in alleviating postoperative pain following laparoscopic cholecystectomy. While the analgesic effectiveness of this technique is independent of the socioeconomic context, conducting the study in a middle-income country enhances the universal applicability and external validity of the M-TAPA block. It demonstrates that this approach can be particularly beneficial in settings with limited resources, where cost-effective and easily implementable pain management strategies are crucial. In our study, the administration of M-TAPA post anesthesia led to a significant reduction in postoperative pain levels, decreased opioid consumption, and a lower requirement for rescue analgesia during the recovery period. These outcomes not only highlight the effectiveness of M-TAPA in improving patient comfort but also emphasize its potential as a valuable tool in resource-limited environments.
This study contributes to the growing body of evidence supporting the use of M-TAPA in abdominal surgeries. In line with the findings of Bilge et al. and Güngör et al. [ 11 , 12 ]. , we found that M-TAPA provides effective analgesia, evidenced by a significant reduction in the need for rescue analgesia and lower postoperative pain scores compared to control groups receiving alternative analgesic approaches. Additionally, we observed a higher proportion of ASA 3 patients in the M-TAPA group, suggesting that this technique may be particularly beneficial for patients with more complex physical statuses.
Our results also align with Tulgar et al.‘s research [ 15 ], which underscores M-TAPA’s efficacy in abdominal surgeries, supporting our own observation of decreased opioid consumption and improved patient satisfaction. Furthermore, our study aligns with Chen et al.‘s findings, emphasizing the versatility of M-TAPA, as we illustrate its potential efficacy, even in obese patients [ 16 ].
The cadaveric studies by Ciftci et al. and the clinical cases presented by Aikawa et al. provide a robust anatomical and clinical basis for M-TAPA efficacy [ 17 , 18 ]. Our findings support this understanding by demonstrating a reduced need for rescue analgesia, lower opioid consumption, and better postoperative recovery in the M-TAPA group compared to the control group. Our study did not directly assess the impact on recovery using instruments like the QoR-40 questionnaire employed in Bilge et al.‘s study or the QoR-15 questionnaire used in Suzuka et al.‘s research [ 12 , 19 ]. Nonetheless, we have emphasized the potential for improved recovery based on objective metrics, such as notably shorter durations for early mobilization and faster resumption of oral intake observed in the M-TAPA group compared to the control group. These specific findings regarding functional aspects of recovery, namely mobilization and oral intake, serve as objective indicators suggesting that the M-TAPA technique may contribute to a swifter and smoother recovery process following laparoscopic cholecystectomy.
The consistent findings across several studies, including our own, underscore the reliability and practicality of this technique for postoperative pain management in abdominal surgeries.
Strengths and limitations
Our study utilized objective and quantitative measures, such as intraoperative opioid consumption and postoperative pain intensity assessed using the visual analog scale, thereby enhancing the reliability and validity of our findings. The inclusion of demographic and clinical variables also allowed for a more comprehensive analysis and appropriate comparison between study groups.
However, several limitations must be acknowledged. The sample size and retrospective design of the present study may introduce selection and confounding biases, potentially compromising its internal validity. Uncontrolled factors such as variations in surgical technique, team experience, and anesthesiologist expertise could influence outcomes. Furthermore, long-term follow-up data for assessing surgical complications and late-stage recovery were lacking. The single-center design limits generalizability, and the absence of definitive recommendations on the optimal LA concentration underscores the need for prospective studies. Despite these limitations, our findings strongly support the efficacy of M-TAPA blockade for perioperative pain management, warranting further multicenter investigations.
Clinical implications
Our study findings suggest that integrating M-TAPA into standard anesthesia protocols for laparoscopic cholecystectomy, particularly in a middle-income country setting, can notably enhance pain management outcomes. By decreasing intraoperative opioid usage and postoperative pain intensity, M-TAPA represents a promising strategy for improving patient comfort, safety, and overall surgical experience. This approach not only helps mitigate opioid-related side effects but also holds potential for expediting recovery, reducing hospital stays, and optimizing resource utilization in healthcare settings. These results highlight the importance of developing accessible and effective pain management strategies in resource-constrained environments, underscoring the need for greater consideration of middle-income countries in research and clinical practice related to perioperative pain management to improve outcomes and enhance the quality of care in these challenging settings.
Our study highlights the effectiveness of M-TAPA for managing postoperative pain after laparoscopic cholecystectomy, especially in middle-income countries. By reducing opioid use and providing prolonged analgesia, MWA offers a safer alternative to traditional methods, potentially leading to the transformation of perioperative care. However, further research is needed to validate its broad applicability in diverse surgical settings.
Data availability
The datasets used and/or analyzed during the current study are available upon reasonable request. Access to the data can be requested via the following Google Drive link: https://drive.google.com/file/d/1uQWopPm2vmltwrh52_TKzjEvL1Z_Cqu-/view? usp=drivesdk. Please contact [email protected] or [email protected] to request access.
Kurtulus I, Culcu OD, Degerli MS. Which is more effective: laparoscopic or open partial cholecystectomy? J Laparoendosc Adv Surg Tech A. 2022;32(5):476–84.
Article PubMed Google Scholar
Breazu C, Margarit S, Bartos A, Ionescu D. Postoperative analgesia after laparoscopic cholecystectomy - prospective, randomized, double Blind, Control Trial. Chirurgia (Bucur). 2022;117(5):563–71.
Bisgaard T, Klarskov B, Rosenberg J, Kehlet H. Characteristics and prediction of early pain after laparoscopic cholecystectomy. Pain. 2001;90(3):261–9.
Ni X, Jia D, Guo Y, Sun X, Suo J. The efficacy and safety of enhanced recovery after surgery (ERAS) program in laparoscopic digestive system surgery: a meta-analysis of randomized controlled trials. Int J Surg. 2019;69:108–15.
Avci O, Gundogdu O, Balci F, Tekcan MN. Effects of modified thoracoabdominal nerve Block through Perichondrial Approach on Postoperative Pain and Analgaesic Consumption in patients undergoing laparoscopic cholecystectomy. J Coll Physicians Surg Pak. 2024;34(1):5–10.
Wills VL, Hunt DR. Pain after laparoscopic cholecystectomy. Br J Surg. 2000;87(3):273–84.
Article PubMed CAS Google Scholar
Cho HY, Hwang IE, Lee M, Kwon W, Kim WH, Lee HJ. Comparison of modified thoracoabdominal nerve block through perichondral approach and subcostal transversus abdominis plane block for pain management in laparoscopic cholecystectomy: a randomized-controlled trial. Korean J Pain. 2023;36(3):382–91.
Article PubMed PubMed Central Google Scholar
Altıparmak B, Korkmaz Toker M, Uysal AI, Kuşçu Y, Gümüş Demirbilek S. Ultrasound-guided erector spinae plane block versus oblique subcostal transversus abdominis plane block for postoperative analgesia of adult patients undergoing laparoscopic cholecystectomy: Randomized, controlled trial. J Clin Anesth. 2019;57:31–6.
Chen Y, Shi K, Xia Y, Zhang X, Papadimos TJ, Xu X, et al. Sensory Assessment and Regression rate of bilateral Oblique Subcostal Transversus Abdominis Plane Block in volunteers. Reg Anesth Pain Med. 2018;43(2):174–9.
PubMed Google Scholar
Tulgar S, Senturk O, Selvi O, Balaban O, Ahiskalioğlu A, Thomas DT, et al. Perichondral approach for blockage of thoracoabdominal nerves: anatomical basis and clinical experience in three cases. J Clin Anesth. 2019;54:8–10.
Güngör H, Ciftci B, Alver S, Gölboyu BE, Ozdenkaya Y, Tulgar S. Modified thoracoabdominal nerve block through perichondrial approach (M-TAPA) vs local infiltration for pain management after laparoscopic cholecystectomy surgery: a randomized study. J Anesth. 2023;37(2):254–60.
Bilge A, Başaran B, Et T, Korkusuz M, Yarımoğlu R, Toprak H, et al. Ultrasound-guided bilateral modified-thoracoabdominal nerve block through a perichondrial approach (M-TAPA) in patients undergoing laparoscopic cholecystectomy: a randomized double-blind controlled trial. BMC Anesthesiol. 2022;22(1):329.
Huskisson EC. Measurement of pain. Lancet. 1974;2(7889):1127–31.
Reed MD, Van Nostran W. Assessing pain intensity with the visual analog scale: a plea for uniformity. J Clin Pharmacol. 2014;54(3):241–4.
Tulgar S, Selvi O, Thomas DT, Deveci U, Özer Z. Modified thoracoabdominal nerves block through perichondrial approach (M-TAPA) provides effective analgesia in abdominal surgery and is a choice for opioid sparing anesthesia. J Clin Anesth. 2019;55:109.
Chen J, Ma C, Guo C. Out-of-plane technique may be the better method for the blockage of thoracoabdominal nerves through perichondrial approach. J Clin Anesth. 2021;69:110155.
Ciftci B, Alici HA, Ansen G, Sakul BU, Tulgar S. Cadaveric investigation of the spread of the thoracoabdominal nerve block using the perichondral and modified perichondral approaches. Korean J Anesthesiol. 2022;75(4):357–9.
Aikawa K, Tanaka N, Morimoto Y. Modified thoracoabdominal nerves block through perichondrial approach (M-TAPA) provides a sufficient postoperative analgesia for laparoscopic sleeve gastrectomy. J Clin Anesth. 2020;59:44–5.
Suzuka T, Tanaka N, Kadoya Y, Ida M, Iwata M, Ozu N et al. Comparison of Quality of Recovery between Modified Thoracoabdominal nerves Block through Perichondrial Approach versus Oblique Subcostal Transversus Abdominis Plane Block in patients undergoing total laparoscopic hysterectomy: a pilot randomized controlled trial. J Clin Med. 2024;13(3).
Download references
Acknowledgements
We would like to extend our sincere gratitude to the Instituto Nacional de Perinatología “Isidro Espinosa de los Reyes” for their financial support, which covered the publication charges for this study. Their generous contribution has been invaluable in facilitating the dissemination of our research findings.
This research did not receive any specific grant from funding agencies in the public, commercial, or not-for-profit sectors.
Author information
Authors and affiliations.
Anesthesiology Department, Hospital General de México Dr. Eduardo Liceaga, Mexico City, Mexico
Luisa Fernanda Castillo-Dávila, Carlos Jesús Torres-Anaya & Raquel Vazquez-Apodaca
Community Interventions Research Branch, Instituto Nacional de Perinatología “Isidro Espinosa de los Reyes”, Mexico City, Mexico
Hector Borboa-Olivares
Department of Reproductive and Perinatal Health Research, Instituto Nacional de Perinatología Isidro Espinosa de los Reyes, Mexico City, Mexico
Salvador Espino-y-Sosa & Johnatan Torres-Torres
You can also search for this author in PubMed Google Scholar
Contributions
LFCD: Conceptualization, Writing – original draft and editing. LFCD and CJTA: Methodology and Investigation. RVA: Writing – the original draft. HBO and SEyS: Methodology and editing. JTT: Conceptualization, Methodology, Formal analysis, Writing – original draft and editing.
Corresponding author
Correspondence to Johnatan Torres-Torres .
Ethics declarations
Ethics approval and consent to participate.
The study was conducted in accordance with the Declaration of Helsinki and approved by the Institutional Review Board of Hospital General de Mexico “Dr. Eduardo Liceaga” (protocol code 1384 − 295/23). Informed consent was obtained from all subjects involved in the study.
Consent for publication
Not applicable.
Competing interests
The authors declare no competing interests.
Additional information
Publisher’s note.
Springer Nature remains neutral with regard to jurisdictional claims in published maps and institutional affiliations.
Rights and permissions
Open Access This article is licensed under a Creative Commons Attribution 4.0 International License, which permits use, sharing, adaptation, distribution and reproduction in any medium or format, as long as you give appropriate credit to the original author(s) and the source, provide a link to the Creative Commons licence, and indicate if changes were made. The images or other third party material in this article are included in the article’s Creative Commons licence, unless indicated otherwise in a credit line to the material. If material is not included in the article’s Creative Commons licence and your intended use is not permitted by statutory regulation or exceeds the permitted use, you will need to obtain permission directly from the copyright holder. To view a copy of this licence, visit http://creativecommons.org/licenses/by/4.0/ .
Reprints and permissions
About this article
Cite this article.
Castillo-Dávila, L.F., Torres-Anaya, C.J., Vazquez-Apodaca, R. et al. Modified thoracoabdominal nerve block via perichondral approach: an alternative for perioperative pain management in laparoscopic cholecystectomy in a middle-income country. BMC Anesthesiol 24 , 304 (2024). https://doi.org/10.1186/s12871-024-02690-8
Download citation
Received : 14 April 2024
Accepted : 22 August 2024
Published : 31 August 2024
DOI : https://doi.org/10.1186/s12871-024-02690-8
Share this article
Anyone you share the following link with will be able to read this content:
Sorry, a shareable link is not currently available for this article.
Provided by the Springer Nature SharedIt content-sharing initiative
- Laparoscopic cholecystectomy
- Modified thoracoabdominal nerve block (M-TAPA)
- Perioperative pain management
- Opioid consumption
- Postoperative analgesia
BMC Anesthesiology
ISSN: 1471-2253
- General enquiries: [email protected]

IMAGES
VIDEO
COMMENTS
Sales Analytics. Improving their online sales by understanding user pre-purchase behaviour. New line of designs in the website contributed to 6% boost in sales. 60% increase in checkout to the payment page. Google Analytics. Enhanced Ecommerce. *. Marketing Automation. Marketing.
Result: 130% growth in conversions and 31.5% improvement in conversion rate. 8. Top Talents flow to "Teach For America". Teach for america. This is one of the best Google Analytics case studies. Teach For America is an organization focused on providing quality education to underprivileged children of America.
Combining different tools can provide a more comprehensive view of your data. For example, pairing Google Analytics with tools like Hotjar for heatmaps or SEMrush for SEO analytics can offer deeper insights. As of 2023, over 60% of marketers use multiple analytics tools to gain better insights. 3.
Case Studies Web Analytics. 5 months ago 11 Oleksandr Konivnenko. 2349 2 0 Case Study: Analytical Tool for Ticket Sales Service - Hundreds of Event Advertising Budgets Managed in Real Time Case Studies Web Analytics. a year ago 5 Alexey Seleznev. 4565 8 0 ...
In this blog, we learnt, about Predictive Web Analytics, various metrics used for this , took a case study, performed Data Visualizations, made clusters based on customer behaviors, built two ...
In this blog post, we'll explore 10 inspiring case studies showcasing the power of product analytics. Real-world examples of how data-driven insights transformed businesses. 1. Netflix 's Content Recommendation System: Personalized Engagement. Delve into the realm of data-driven innovation as you uncover the inner workings of Netflix 's ...
15 Business Analytics Case Studies [2024] In today's data-driven world, the strategic application of business analytics stands as a cornerstone for enterprise success across various industries. From retail giants optimizing inventory through predictive algorithms to healthcare systems enhancing patient care with personalized treatments, the ...
Case Studies Success Stories Powered by Data: Discover Our Real-World Impact June 6, 2019; High Volume ClickStream Analytics to Monetize Search Page Ads ... Web Analytics. INDUSTRIES. Technology. CPG. Retail. Financial-services. Industrials (Re)Think Data Think LatentView. Services. Technical Expertise. Domain Expertise. Advisory Services ...
How to create an impactful web analytics strategy in 6 steps. We've brought it back to the basics and created this six-step guide to help you develop a strategy that puts your customer at the forefront, so you can keep making the right decisions for the right reasons. Let's dive in! 1. Collect website data and customer insights.
Explore web analytics case studies. Find the path toward becoming data‑driven. Our clients have become confident about making the right digital marketing decisions. Their teams understand their business priorities and the path to reach their targets. Agata continues to keep improving our data in ways we didn't think were possible.
Cloud case studies. Global insurer embraces advanced analytics to improve predictability and service. Interactive gaming publisher uses analytics to transform its data model. Leading beverage producer uses exploratory analytics to uncover actionable opportunities. Global consumer products company uses visualization and advanced analytics to ...
In this case, the way the Google Analytics scripts were implemented was causing additional problems. At some point in those 3 pageviews, the user's traffic source was being dropped, and some of the user visits were being attributed to "Direct to site", as if they had typed in the domain name directly into the browser and visited directly.
Here are 10 case studies of companies that used insights from web analytics and drove ROI. BT FINANCIAL GROUP: Is a leading provider of superannuation, investment, and insurance products. The BT website focuses on service and usability with an online application form as one of the key conversion points. Landing pages with different combinations ...
As a consequence, there is a better knowledge of social media traffic. Panasonic: Renowned firms, including Panasonic, have recognized the value of digital marketing. Panasonic and the firm NRI Netcom agreed to combine all of their marketing data from all of their websites into a single platform, Google Analytics 360.
Google Analytics Performance Marketing Case Studies. When you change the way data is collected and analyzed, you gain insights into your customers and their purchase behaviors. The brands in the section below, including Westwing, Travelocity and PBS, did just that with products such as Google Analytics Premium and Universal Analytics. Case Study.
A case study of Web analytics usage in a large multinational airline company demonstrates an application of the theory to a practical context with a company that invests significant resources in ...
2. Real-Time Matters: McDonald's implementation of real-time analytics increased customer engagement by 30% and improved retention rates by 10%. 3. Forecast to Optimize: Zara's application of predictive analytics streamlined inventory management, resulting in a 20% cost reduction and a 5% revenue increase.
Explore case studies and videos. Solutions close. Industry Solutions Reduce cost, increase operational agility, and capture new market opportunities. ... Since implementing Google Analytics Premium, the ability to access a single Google Analytics account to evaluate web and app performance has made reporting easier and more efficient, and it ...
Discover the power of data science through 10 intriguing case studies, including GE, PayPal, Amazon, IBM Watson Health, Uber, NASA, Zendesk, John Deer, etc. ... predictive analytics enhances risk management. In transportation, route optimization reduces costs and emissions. Data science empowers industries to innovate and solve complex ...
Here are a few data analytics case study examples at Amazon: i) Recommendation Systems. Data science models help amazon understand the customers' needs and recommend them to them before the customer searches for a product; this model uses collaborative filtering. Amazon uses 152 million customer purchases data to help users to decide on ...
to improv e a website's performance using Web analytics. Based on academic papers, official sources, white papers, and best. practices, the main research objective of this paper is to establish ...
From engagement statistics to content analytics to conversion metrics, data is a big part of most social media managers' responsibilities. But that doesn't necessarily mean you enjoy processing marketing data or drawing conclusions from it. If data isn't exactly your favorite part of the job, these marketing analytics case studies may change your mind. Find out how marketing analytics ...
Business analytics case studies involve the logical investigation of an organization's data utilizing various forms of business intelligence and statistical data analysis. Konverse AI - AI Chatbot, Team Inbox, WhatsApp Campaign, Instagram ... When you turn on re-marketing for a web property, you can use the data from Analytics to build re ...
When conducting QLR, time is the lens used to inform the overall study design and processes of data collection and analysis. While QLR is an evolving methodology, spanning diverse disciplines (Holland et al., 2006), a key feature is the collection of data on more than one occasion, often described as waves (Neale, 2021).Thus, researchers embarking on designing a new study need to consider ...
Copper-induced cell death is a novel mechanism of cell death, which is defined as cuproptosis. The increasing level of copper can produce toxicity in cells and may cause the occurrence of cell death. Several previous studies have proved that cuproptosis has a tight association with various cancers. …
Methods and analysis A dataset of 500 plain radiographs derived from Oxford University Hospitals (OUH) NHS Foundation Trust will be collated to include all bones except the skull, facial bones and cervical spine. The dataset will be split evenly between radiographs showing one or more fractures and those without. The reference ground truth for each image will be established through independent ...
The Binding Antibody Multiplex Assay (BAMA) antigens were selected for this study through an analysis of the vaccine immunogen sequence and available sequence reagents to maximally capture breadth in Southern Africa (Appendix p 3, Figure S1). The two T-cell markers are percent of CD4+ (or CD8+) T cells expressing IFN-g and/or IL-2 upon ...
R.G. Kar hospital rape-murder case: Calcutta High Court directs CBI to investigate detestable social media posts on rape-murder victim by R G Kar Medical College.
PubMed, Embase, Web of Science, and Cochrane Library databases were comprehensively retrieved to collect all case-control studies on OC. A meta-analysis was performed to compare the levels of salivary IL-8, IL-6, IL-10, TNF-α, and IL-1β in OC patients and healthy controls. Network meta-analysis (NMA) was carried out to probe into the ...
Background Laparoscopic cholecystectomy is known for its minimally invasive nature, but postoperative pain management remains challenging. Despite the enhanced recovery after surgery (ERAS) protocol, regional analgesic techniques like modified perichondral approach to thoracoabdominal nerve block (M-TAPA) show promise. Our retrospective study evaluates M-TAPA's efficacy in postoperative pain ...