Thank you for visiting nature.com. You are using a browser version with limited support for CSS. To obtain the best experience, we recommend you use a more up to date browser (or turn off compatibility mode in Internet Explorer). In the meantime, to ensure continued support, we are displaying the site without styles and JavaScript.
- View all journals
- Explore content
- About the journal
- Publish with us
- Sign up for alerts
- Review Article
- Published: 23 August 2024

Causal inference on human behaviour
- Drew H. Bailey ORCID: orcid.org/0000-0002-7812-1107 1 ,
- Alexander J. Jung ORCID: orcid.org/0000-0003-3699-9066 2 ,
- Adriene M. Beltz ORCID: orcid.org/0000-0001-5754-8083 3 ,
- Markus I. Eronen ORCID: orcid.org/0000-0003-2028-3338 4 ,
- Christian Gische 5 ,
- Ellen L. Hamaker 6 ,
- Konrad P. Kording ORCID: orcid.org/0000-0001-8408-4499 7 , 8 ,
- Catherine Lebel ORCID: orcid.org/0000-0002-0344-4032 9 , 10 ,
- Martin A. Lindquist 11 ,
- Julia Moeller 12 ,
- Adeel Razi ORCID: orcid.org/0000-0002-0779-9439 13 , 14 , 15 , 16 ,
- Julia M. Rohrer ORCID: orcid.org/0000-0001-8564-4523 17 ,
- Baobao Zhang ORCID: orcid.org/0000-0001-7217-5035 18 &
- Kou Murayama ORCID: orcid.org/0000-0003-2902-9600 2 , 19
Nature Human Behaviour volume 8 , pages 1448–1459 ( 2024 ) Cite this article
1604 Accesses
58 Altmetric
Metrics details
- Human behaviour
- Social policy
Making causal inferences regarding human behaviour is difficult given the complex interplay between countless contributors to behaviour, including factors in the external world and our internal states. We provide a non-technical conceptual overview of challenges and opportunities for causal inference on human behaviour. The challenges include our ambiguous causal language and thinking, statistical under- or over-control, effect heterogeneity, interference, timescales of effects and complex treatments. We explain how methods optimized for addressing one of these challenges frequently exacerbate other problems. We thus argue that clearly specified research questions are key to improving causal inference from data. We suggest a triangulation approach that compares causal estimates from (quasi-)experimental research with causal estimates generated from observational data and theoretical assumptions. This approach allows a systematic investigation of theoretical and methodological factors that might lead estimates to converge or diverge across studies.
This is a preview of subscription content, access via your institution
Access options
Access Nature and 54 other Nature Portfolio journals
Get Nature+, our best-value online-access subscription
24,99 € / 30 days
cancel any time
Subscribe to this journal
Receive 12 digital issues and online access to articles
111,21 € per year
only 9,27 € per issue
Buy this article
- Purchase on SpringerLink
- Instant access to full article PDF
Prices may be subject to local taxes which are calculated during checkout
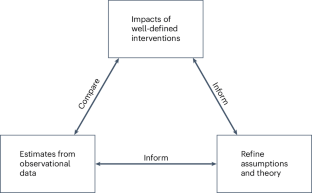
Similar content being viewed by others
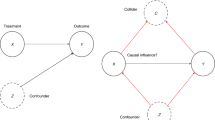
Quantifying causality in data science with quasi-experiments
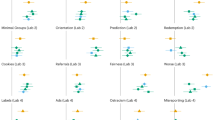
High replicability of newly discovered social-behavioural findings is achievable
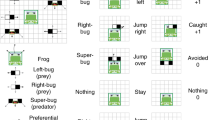
Causal reductionism and causal structures
Angrist, J. D. & Pischke, J.-S. The credibility revolution in empirical economics: how better research design is taking the con out of econometrics. J. Econ. Perspect. 24 , 3–30 (2010).
Article Google Scholar
Hernán, M. A. & Robins, J. M. Causal Inference: What If (Chapman & Hall/CRC, 2020).
Aronow, P. M. & Miller, B. T. Foundations of Agnostic Statistics (Cambridge Univ. Press, 2019).
Keele, L. The statistics of causal inference: a view from political methodology. Polit. Anal. 23 , 313–335 (2015).
Foster, E. M. Causal inference and developmental psychology. Dev. Psychol. 46 , 1454–1480 (2010).
Article PubMed Google Scholar
Marinescu, I. E., Lawlor, P. N. & Kording, K. P. Quasi-experimental causality in neuroscience and behavioural research. Nat. Hum. Behav. 2 , 891–898 (2018).
Rohrer, J. M. Thinking clearly about correlations and causation: graphical causal models for observational data. Adv. Methods Pract. Psychol. Sci. 1 , 27–42 (2018).
Rigoux, L. & Daunizeau, J. Dynamic causal modelling of brain–behaviour relationships. NeuroImage 117 , 202–221 (2015).
Article CAS PubMed Google Scholar
Gangl, M. Causal inference in sociological research. Annu. Rev. Sociol. 36 , 21–47 (2010).
Winship, C. & Morgan, S. L. The estimation of causal effects from observational data. Annu. Rev. Sociol. 25 , 659–706 (1999).
Imbens, G. W. & Rubin, D. B. Causal Inference in Statistics, Social, and Biomedical Sciences (Cambridge Univ. Press, 2015).
Pearl, J. Causality: Models, Reasoning, and Inference 2nd edn (Cambridge Univ. Press, 2009).
Hamaker, E. L. & Wichers, M. No time like the present. Curr. Dir. Psychol. Sci. 26 , 10–15 (2017).
Angrist, J. D. & Pischke, J.-S. Mostly Harmless Econometrics: An Empiricist’s Companion (Princeton Univ. Press, 2009).
Gelman, A. & Imbens, G. Why Ask Why? Forward Causal Inference and Reverse Causal Questions Working Paper No. 19614 (NBER, 2013).
Alvarez-Vargas, D. et al. Hedges, mottes, and baileys: causally ambiguous statistical language can increase perceived study quality and policy relevance. PLoS ONE 18 , e0286403 (2023).
Article CAS PubMed PubMed Central Google Scholar
Haber, N. A. et al. Causal and associational language in observational health research: a systematic evaluation. Am. J. Epidemiol. 191 , 2084–2097 (2022).
Article PubMed PubMed Central Google Scholar
Hernán, M. A. The C-word: scientific euphemisms do not improve causal inference from observational data. Am. J. Public Health 108 , 616–619 (2018).
Rohrer, J. M. & Lucas, R. E. Causal effects of well-being on health: it’s complicated. Preprint at PsyArXiv https://doi.org/10.31234/osf.io/wgbe4 (2020).
Hoemann, K., Devlin, M. & Barrett, L. F. Comment: emotions are abstract, conceptual categories that are learned by a predicting brain. Emot. Rev. 12 , 253–255 (2020).
Young, C. & Holsteen, K. Model uncertainty and robustness: a computational framework for multimodel analysis. Sociol. Methods Res. 46 , 3–40 (2017).
Cinelli, C. & Hazlett, C. Making sense of sensitivity: extending omitted variable bias. J. R. Stat. Soc. B 82 , 39–67 (2020).
Branwen, G. How often does correlation = causality? Gwern.net https://www.gwern.net/Correlation (2022).
Runge, J. Causal network reconstruction from time series: from theoretical assumptions to practical estimation. Chaos 28 , 075310 (2018).
Oster, E. Health recommendations and selection in health behaviors. Am. Econ. Rev. Insights 2 , 143–160 (2020).
VanderWeele, T. J. Constructed measures and causal inference: towards a new model of measurement for psychosocial constructs. Epidemiology 33 , 141–151 (2022).
Greenland, S., Judea, P. & Robins, J. M. Causal diagrams for epidemiologic research. Epidemiology 10 , 37–48 (1999).
Rosenbaum, P. R. From association to causation in observational studies: the role of tests of strongly ignorable treatment assignment. J. Am. Stat. Assoc. 79 , 41–48 (1984).
Hoyle, R. H., Lynam, D. R., Miller, J. D. & Pek, J. The questionable practice of partialing to refine scores on and inferences about measures of psychological constructs. Annu. Rev. Clin. Psychol. 19 , 155–176 (2023).
Cinelli, C., Forney, A. & Pearl, J. A crash course in good and bad controls. Sociol. Methods Res. https://doi.org/10.1177/00491241221099552 (2022).
Wysocki, A. C., Lawson, K. M. & Rhemtulla, M. Statistical control requires causal justification. Adv. Methods Pract. Psychol. Sci. 5 , 251524592210958 (2022).
Elwert, F. & Winship, C. Endogenous selection bias: the problem of conditioning on a collider variable. Annu. Rev. Sociol. 40 , 31–53 (2014).
Knox, D., Lowe, W. & Mummolo, J. Administrative records mask racially biased policing. Am. Polit. Sci. Rev. 114 , 619–637 (2020).
Bryan, C. J., Tipton, E. & Yeager, D. S. Behavioural science is unlikely to change the world without a heterogeneity revolution. Nat. Hum. Behav. 5 , 980–989 (2021).
Haslbeck, J. M. B. & Ryan, O. Recovering within-person dynamics from psychological time series. Multivar. Behav. Res. 57 , 735–766 (2022).
Goldsmith-Pinkham, P., Hull, P. & Kolesár, M. Contamination Bias in Linear Regressions Working Paper No. 30108 (NBER, 2022).
Goodman-Bacon, A. Difference-in-differences with variation in treatment timing. J. Econ. 225 , 254–277 (2021).
Wu, W., Carroll, I. A. & Chen, P.-Y. A single-level random-effects cross-lagged panel model for longitudinal mediation analysis. Behav. Res Methods 50 , 2111–2124 (2018).
Rubin, D. B. Causal inference using potential outcomes. J. Am. Stat. Assoc. 100 , 322–331 (2005).
Article CAS Google Scholar
Altmejd, A. et al. O brother, where start thou? Sibling spillovers on college and major choice in four countries. Q. J. Econ. 136 , 1831–1886 (2021).
Heckman, J. & Karapakula, G. Intergenerational and Intragenerational Externalities of the Perry Preschool Project Working Paper No. 25889 (NBER, 2019).
Karbownik, K. & Özek, U. Setting a Good Example? Examining Sibling Spillovers in Educational Achievement Using a Regression Discontinuity Design Working Paper No. 26411 (NBER, 2019).
Bringmann, L. F. et al. Psychopathological networks: theory, methods and practice. Behav. Res Ther. 149 , 104011 (2022).
Dietrich, J., Schmiedek, F. & Moeller, J. Academic motivation and emotions are experienced in learning situations, so let’s study them: introduction to the special issue. Learn. Instr. 81 , 101623 (2022).
Robins, J. M., Scheines, R., Spirtes, P. & Wasserman, L. Uniform consistency in causal inference. Biometrika 90 , 491–515 (2003).
VanderWeele, T. J. & Hernán, M. A. Causal inference under multiple versions of treatment. J. Causal Inference 1 , 1–20 (2013).
Pearl, J. Does obesity shorten life? Or is it the soda? On non-manipulable causes. J. Causal Inference 6 , 20182001 (2018).
Angrist, J. D. & Pischke, J.-S. Mastering ’Metrics: The Path from Cause to Effect (Princeton Univ. Press, 2014).
Eronen, M. I. Causal discovery and the problem of psychological interventions. N. Ideas Psychol. 59 , 100785 (2020).
Scheines, R. The similarity of causal inference in experimental and non-experimental studies. Phil. Sci. 72 , 927–940 (2005).
Bringmann, L. F., Elmer, T. & Eronen, M. I. Back to basics: the importance of conceptual clarification in psychological science. Curr. Dir. Psychol. Sci. 31 , 340–346 (2022).
Spirtes, P. & Scheines, R. Causal inference of ambiguous manipulations. Phil. Sci. 71 , 833–845 (2004).
Bollen, K. A. & Brand, J. E. A general panel model with random and fixed effects: a structural equations approach. Soc. Forces 89 , 1–34 (2010).
Hamaker, E. L., Kuiper, R. M. & Grasman, R. P. P. P. A critique of the cross-lagged panel model. Psychol. Methods 20 , 102–116 (2015).
Zyphur, M. J. et al. From data to causes I: building a general cross-lagged panel model (GCLM). Organ. Res. Methods 23 , 651–687 (2020).
Voelkle, M. C., Oud, J. H. L., Davidov, E. & Schmidt, P. An SEM approach to continuous time modeling of panel data: relating authoritarianism and anomia. Psychol. Methods 17 , 176–192 (2012).
Frangakis, C. E. & Rubin, D. B. Principal stratification in causal inference. Biometrics 58 , 21–29 (2002).
Beltz, A. M. & Gates, K. M. Network mapping with GIMME. Multivar. Behav. Res. 52 , 789–804 (2017).
Montoya, L. M. et al. The optimal dynamic treatment rule superlearner: considerations, performance, and application to criminal justice interventions. International J. Biostat. 19 , 217–238 (2023).
Gische, C. & Voelkle, M. C. Beyond the mean: a flexible framework for studying causal effects using linear models. Psychometrika 87 , 868–901 (2022).
Imai, K. & Kim, I. S. When should we use unit fixed effects regression models for causal inference with longitudinal data? Am. J. Polit. Sci. 63 , 467–490 (2019).
Sobel, M. E. & Lindquist, M. A. Causal inference for fMRI time series data with systematic errors of measurement in a balanced on/off study of social evaluative threat. J. Am. Stat. Assoc. 109 , 967–976 (2014).
Usami, S. Within-person variability score-based causal inference: a two-step estimation for joint effects of time-varying treatments. Psychometrika 88 , 1466–1494 (2022).
Hamaker, E. L., Mulder, J. D. & van IJzendoorn, M. H. Description, prediction and causation: methodological challenges of studying child and adolescent development. Dev. Cogn. Neurosci. 46 , 100867 (2020).
Lundberg, I., Johnson, R. & Stewart, B. M. What is your estimand? Defining the target quantity connects statistical evidence to theory. Am. Sociol. Rev. 86 , 532–565 (2021).
Rohrer, J. M. & Murayama, K. These are not the effects you are looking for: causality and the within-/between-persons distinction in longitudinal data analysis. Adv. Methods Pract. Psychol. Sci. 6 , 251524592211408 (2023).
Silberzahn, R. et al. Many analysts, one data set: making transparent how variations in analytic choices affect results. Adv. Methods Pract. Psychol. Sci. 1 , 337–356 (2018).
Auspurg, K. & Brüderl, J. Has the credibility of the social sciences been credibly destroyed? Reanalyzing the ‘many analysts, one data set’ project. Socius 7 , 237802312110244 (2021).
Shadish, W. R, Cook, T. D & Campbell, D. T. Experimental and Quasi-Experimental Designs for Generalized Causal Inference (Houghton, Mifflin, 2002).
Rhemtulla, M., van Bork, R. & Borsboom, D. Worse than measurement error: consequences of inappropriate latent variable measurement models. Psychol. Methods 25 , 30–45 (2020).
Westfall, J. & Yarkoni, T. Statistically controlling for confounding constructs is harder than you think. PLoS ONE 11 , e0152719 (2016).
Grosz, M. P., Rohrer, J. M. & Thoemmes, F. The taboo against explicit causal inference in nonexperimental psychology. Perspect. Psychol. Sci. 15 , 1243–1255 (2020).
Deming, D. Early childhood intervention and life-cycle skill development: evidence from Head Start. Am. Econ. J. Appl. Econ. 1 , 111–134 (2009).
Pion, G. M. & Lipsey, M. W. Impact of the Tennessee Voluntary Prekindergarten Program on children’s literacy, language, and mathematics skills: results from a regression-discontinuity design. AERA Open 7 , 233285842110413 (2021).
Ritchie, S. J. & Tucker-Drob, E. M. How much does education improve intelligence? A meta-analysis. Psychol. Sci. 29 , 1358–1369 (2018).
Steiner, P. M., Wong, V. C. & Anglin, K. A causal replication framework for designing and assessing replication efforts. Z. Psychol. 227 , 280–292 (2019).
Google Scholar
Munafò, M. R. & Davey Smith, G. Robust research needs many lines of evidence. Nature 553 , 399–401 (2018).
Colnet, B. et al. Causal inference methods for combining randomized trials and observational studies: a review. Stat. Sci. 39 , 165–191 (2024).
Wan, S., Brick, T. R., Alvarez-Vargas, D. & Bailey, D. H. Triangulating on developmental models with a combination of experimental and nonexperimental estimates. Dev. Psychol. 59 , 216–228 (2022).
Gische, C., West, S. G. & Voelkle, M. C. Forecasting causal effects of interventions versus predicting future outcomes. Struct. Equ. Modeling 28 , 475–492 (2021).
Imai, K., Kim, I. S. & Wang, E. H. Matching methods for causal inference with time‐series cross‐sectional data. Am. J. Polit. Sci. 67 , 587–605 (2021).
Zyphur, M. J. et al. From data to causes II: comparing approaches to panel data analysis. Organ. Res. Methods 23 , 688–716 (2020).
Lüdtke, O. & Robitzsch, A. A comparison of different approaches for estimating cross-lagged effects from a causal inference perspective. Struct. Equ. Modeling 29 , 888–907 (2022).
Usami, S., Murayama, K. & Hamaker, E. L. A unified framework of longitudinal models to examine reciprocal relations. Psychol. Methods 24 , 637–657 (2019).
Bond, T. N. & Lang, K. The evolution of the black–white test score gap in grades K–3: the fragility of results. Rev. Econ. Stat. 95 , 1468–1479 (2013).
Larzelere, R. E., Cox, R. B. & Smith, G. L. Do nonphysical punishments reduce antisocial behavior more than spanking? A comparison using the strongest previous causal evidence against spanking. BMC Pediatr. 10 , 10 (2010).
Oster, E. Unobservable selection and coefficient stability: theory and evidence. J. Bus. Econ. Stat. 37 , 187–204 (2019).
Athey, S., Chetty, R., Imbens, G. W. & Kang, H. The Surrogate Index: Combining Short-Term Proxies to Estimate Long-Term Treatment Effects More Rapidly and Precisely Working Paper No. 26463 (NBER, 2019).
Weidmann, B. & Miratrix, L. Lurking inferential monsters? Quantifying selection bias in evaluations of school programs. J. Policy Anal. Manage. 40 , 964–986 (2021).
Dehejia, R. H. & Wahba, S. Causal effects in nonexperimental studies: reevaluating the evaluation of training programs. J. Am. Stat. Assoc. 94 , 1053–1062 (1999).
LaLonde, R. J. Evaluating the econometric evaluations of training programs with experimental data. Am. Econ. Rev. 76 , 604–620 (1986).
Protzko, J. Effects of cognitive training on the structure of intelligence. Psychon. Bull. Rev. 24 , 1022–1031 (2017).
Schmidt, F. L. Beyond questionable research methods: the role of omitted relevant research in the credibility of research. Arch. Sci. Psychol. 5 , 32–41 (2017).
Meehl, P. E. Why summaries of research on psychological theories are often uninterpretable. Psychol. Rep. 66 , 195–244 (1990).
Chaku, N., Kelly, D. P. & Beltz, A. M. Individualized learning potential in stressful times: how to leverage intensive longitudinal data to inform online learning. Comput. Hum. Behav. 121 , 106772 (2021).
Moeller, J. et al. Generalizability crisis meets heterogeneity revolution: determining under which boundary conditions findings replicate and generalize. Preprint at PsyArXiv https://doi.org/10.31234/osf.io/5wsna (2022).
Dunning, T. et al. (eds). Information, Accountability, And Cumulative Learning: Lessons From Metaketa I (Cambridge Univ. Press, 2019).
Low, H. & Meghir, C. The use of structural models in econometrics. J. Econ. Perspect. 31 , 33–58 (2017).
Todd, P. E. & Wolpin, K. I. Assessing the impact of a school subsidy program in Mexico: using a social experiment to validate a dynamic behavioral model of child schooling and fertility. Am. Econ. Rev. 96 , 1384–1417 (2006).
Pearl, J., Glymour, M. & Jewell, N. P. Causal Inference in Statistics: A Primer (John Wiley & Sons, 2016).
Achen, C. H. Let’s put garbage-can regressions and garbage-can probits where they belong. Confl. Manage. Peace Sci. 22 , 327–339 (2005).
Athey, S. & Imbens, G. Recursive partitioning for heterogeneous causal effects. Proc. Natl Acad. Sci. USA 113 , 7353–7360 (2016).
Geng, E. H., Holmes, C. B., Moshabela, M., Sikazwe, I. & Petersen, M. L. Personalized public health: an implementation research agenda for the HIV response and beyond. PLoS Med. 16 , e1003020 (2019).
Moeller, J. Averting the next credibility crisis in psychological science: within-person methods for personalized diagnostics and intervention. J. Pers. Oriented Res. 7 , 53–77 (2021).
Pearl, J. & Bareinboim, E. Transportability of causal and statistical relations: a formal approach. Proc. AAAI Conf. Artif. Intell. 25 , 247–254 (2011).
Wager, S. & Athey, S. Estimation and inference of heterogeneous treatment effects using random forests. J. Am. Stat. Assoc. 113 , 1228–1242 (2018).
Benjamin-Chung, J. et al. Spillover effects in epidemiology: parameters, study designs and methodological considerations. Int. J. Epidemiol. 47 , 332–347 (2018).
Hudgens, M. G. & Halloran, M. E. Toward causal inference with interference. J. Am. Stat. Assoc. 103 , 832–842 (2008).
Imai, K., Jiang, Z. & Malani, A. Causal inference with interference and noncompliance in two-stage randomized experiments. J. Am. Stat. Assoc. 116 , 632–644 (2021).
Tchetgen, E. J. T. & VanderWeele, T. J. On causal inference in the presence of interference. Stat. Methods Med. Res. 21 , 55–75 (2012).
Zhang, C., Mohan, K. & Pearl, J. Causal inference with non-IID data using linear graphical models. Adv. Neural Inf. Process. Syst. 35 , 13214–13225 (2022).
Eberhardt, F. & Scheines, R. Interventions and causal inference. Phil. Sci. 74 , 981–995 (2007).
Mooij, J. M., Magliacane, S. & Claassen, T. Joint causal inference from multiple contexts. J. Mach. Learn. Res. 21 , 3919–4026 (2020).
Peters, J., Bühlmann, P. & Meinshausen, N. Causal inference by using invariant prediction: identification and confidence intervals. J. R. Stat. Soc. B 78 , 947–1012 (2016).
Aalen, O., Røysland, K., Gran, J., Kouyos, R. & Lange, T. Can we believe the DAGs? A comment on the relationship between causal DAGs and mechanisms. Stat. Methods Med. Res. 25 , 2294–2314 (2016).
Driver, C. C. & Voelkle, M. C. in Continuous Time Modeling in the Behavioral and Related Sciences (eds Van Montfort, K. et al.) 79–109 (Springer International, 2018).
Røysland, K. A martingale approach to continuous-time marginal structural models. Bernoulli 17 , 895–915 (2011).
Ryan, O. & Hamaker, E. L. Time to intervene: a continuous-time approach to network analysis and centrality. Psychometrika 87 , 214–252 (2022).
Download references
Acknowledgements
This Review resulted from a cross-disciplinary workshop discussing such approaches ( https://www.longitudinaldataanalysis.com/ ). The workshop and collaboration were funded by the Jacobs Foundation and CIFAR. The funders had no role in the decision to publish or in the preparation of this manuscript.
Author information
Authors and affiliations.
School of Education, University of California, Irvine, Irvine, CA, USA
Drew H. Bailey
Hector Research Institute of Education Sciences and Psychology, University of Tübingen, Tübingen, Germany
Alexander J. Jung & Kou Murayama
Department of Psychology, University of Michigan, Ann Arbor, MI, USA
Adriene M. Beltz
Department of Theoretical Philosophy, University of Groningen, Groningen, the Netherlands
Markus I. Eronen
Department of Psychology, Humboldt-Universität zu Berlin, Berlin, Germany
Christian Gische
Methodology and Statistics, Faculty of Social and Behavioural Sciences, Utrecht University, Utrecht, the Netherlands
Ellen L. Hamaker
Department of Bioengineering, University of Pennsylvania, Philadelphia, PA, USA
Konrad P. Kording
Department of Neuroscience, University of Pennsylvania, Philadelphia, PA, USA
Alberta Children’s Hospital Research Institute, Calgary, Alberta, Canada
Catherine Lebel
Department of Radiology, University of Calgary, Calgary, Alberta, Canada
Department of Biostatistics, Johns Hopkins University, Baltimore, MD, USA
Martin A. Lindquist
Leipzig University, Leipzig, Germany
Julia Moeller
Turner Institute for Brain and Mental Health, School of Psychological Sciences, Monash University, Clayton, Victoria, Australia
Monash Biomedical Imaging, Monash University, Clayton, Victoria, Australia
Wellcome Trust Centre for Neuroimaging, Institute of Neurology, University College London, London, UK
CIFAR Azrieli Global Scholars Program, CIFAR, Toronto, Ontario, Canada
Wilhelm Wundt Institute for Psychology, Faculty of Life Sciences, Leipzig University, Leipzig, Germany
Julia M. Rohrer
Maxwell School of Citizenship and Public Affairs, Syracuse University, Syracuse, NY, USA
Baobao Zhang
Research Institute, Kochi University of Technology, Kochi, Japan
Kou Murayama
You can also search for this author in PubMed Google Scholar
Corresponding author
Correspondence to Drew H. Bailey .
Ethics declarations
Competing interests.
The authors declare no competing interests.
Peer review
Peer review information.
Nature Human Behaviour thanks Jörn-Steffen Pischke and Rebecca Johnson for their contribution to the peer review of this work.
Additional information
Publisher’s note Springer Nature remains neutral with regard to jurisdictional claims in published maps and institutional affiliations.
Rights and permissions
Springer Nature or its licensor (e.g. a society or other partner) holds exclusive rights to this article under a publishing agreement with the author(s) or other rightsholder(s); author self-archiving of the accepted manuscript version of this article is solely governed by the terms of such publishing agreement and applicable law.
Reprints and permissions
About this article
Cite this article.
Bailey, D.H., Jung, A.J., Beltz, A.M. et al. Causal inference on human behaviour. Nat Hum Behav 8 , 1448–1459 (2024). https://doi.org/10.1038/s41562-024-01939-z
Download citation
Received : 17 March 2023
Accepted : 27 June 2024
Published : 23 August 2024
Issue Date : August 2024
DOI : https://doi.org/10.1038/s41562-024-01939-z
Share this article
Anyone you share the following link with will be able to read this content:
Sorry, a shareable link is not currently available for this article.
Provided by the Springer Nature SharedIt content-sharing initiative
Quick links
- Explore articles by subject
- Guide to authors
- Editorial policies
Sign up for the Nature Briefing newsletter — what matters in science, free to your inbox daily.

Difference-In-Difference Techniques and Causal Inference
- First Online: 14 December 2022
Cite this chapter
- Sue Fu 34 ,
- Katherine Arnow 34 ,
- Amber Trickey 34 &
- Lisa Marie Knowlton 34
Part of the book series: Hot Topics in Acute Care Surgery and Trauma ((HTACST))
346 Accesses
Quasi-experimental study designs seek to measure the effect of an intervention without requiring randomization of study participants, which can be costly, unethical, or impractical. Many quasi-experimental studies utilize naturally occurring phenomena or experiments to determine the difference in outcome between a non-intervention and an intervention group. However, quasi-experimental designs must address the issue of selection bias between the non-intervention and intervention groups to overcome concerns of internal validity. This chapter will discuss one such study design, difference-in-difference, as well as briefly mention other methodologies of causal inference.
This is a preview of subscription content, log in via an institution to check access.
Access this chapter
Subscribe and save.
- Get 10 units per month
- Download Article/Chapter or eBook
- 1 Unit = 1 Article or 1 Chapter
- Cancel anytime
- Available as PDF
- Read on any device
- Instant download
- Own it forever
- Available as EPUB and PDF
- Compact, lightweight edition
- Dispatched in 3 to 5 business days
- Free shipping worldwide - see info
- Durable hardcover edition
Tax calculation will be finalised at checkout
Purchases are for personal use only
Institutional subscriptions
Similar content being viewed by others
Mixed Methods and Causal Analysis
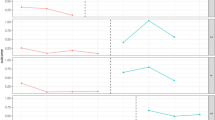
Assessing generalizability and variability of single-case design effect sizes using two-stage multilevel modeling including moderators
Single-Case Designs
CODA Collaborative, et al. A randomized trial comparing antibiotics with appendectomy for appendicitis. N Engl J Med. 2020;383:1907–19. https://doi.org/10.1056/NEJMoa2014320 .
Article Google Scholar
Andreas M, Wiedemann D, Seebacher G, Rath C, Aref T, Rosenhek R, et al. The Ross procedure offers excellent survival compared with mechanical aortic valve replacement in a real-world setting. Eur J Cardiothorac Surg. 2014;46:409–14. https://doi.org/10.1093/ejcts/ezt663 .
David TE, David C, Woo A, Manlhiot C. The Ross procedure: outcomes at 20 years. J Thorac Cardiovasc Surg. 2014;147:85–94. https://doi.org/10.1016/j.jtcvs.2013.08.007 .
Dimick JB, Ryan AM. Methods for evaluating changes in health care policy: the difference-in-difference approach. JAMA. 2014;312:2401. https://doi.org/10.1001/jama.2014.16153 .
Article CAS Google Scholar
Rajaram R, Chung JW, Jones AT, Cohen ME, Dahlke AR, Ko CY, et al. Association of the 2011 ACGME resident duty hour reform with general surgery patient outcomes and with resident examination performance. JAMA. 2014;312:2374–84. https://doi.org/10.1001/jama.2014.15277 .
Zogg CK, Scott JW, Metcalfe D, Gluck AR, Curfman GD, Davis KA, et al. Association of Medicaid expansion with access to rehabilitative care in adult trauma patients. JAMA Surg. 2019;154:402–11. https://doi.org/10.1001/jamasurg.2018.5177 .
Stuart EA, Huskamp HA, Duckworth K, Simmons J, Song Z, Chernew M, et al. Using propensity scores in difference-in-difference models to estimate the effects of a policy change. Health Serv Outcomes Res Methodol. 2014;14:166–82. https://doi.org/10.1007/s10742-014-0123-z .
Card D, Dobkin C, Maestas N. The impact of nearly universal insurance coverage on health care utilization: evidence from Medicare. Am Econ Rev. 2008;98:2242–58. https://doi.org/10.1257/aer.98.5.2242 .
Newgard CD, Schmicker RH, Hedges JR, Trickett JP, Davis DP, Bulger EM, et al. Emergency medical services intervals and survival in trauma: assessment of the “Golden Hour” in a North American Prospective Cohort. Ann Emerg Med. 2010;55:235–246.e4. https://doi.org/10.1016/j.annemergmed.2009.07.024 .
Kurz CF, Rehm M, Holle R, Teuner C, Laxy M, Schwarzkopf L. The effect of bariatric surgery on health care costs: a synthetic control approach using Bayesian structural time series. Health Econ. 2019;28:1293–307. https://doi.org/10.1002/hec.3941 .
Download references
Author information
Authors and affiliations.
S-SPIRE, Department of Surgery, Stanford University, Stanford, CA, USA
Sue Fu, Katherine Arnow, Amber Trickey & Lisa Marie Knowlton
You can also search for this author in PubMed Google Scholar
Corresponding author
Correspondence to Sue Fu .
Editor information
Editors and affiliations.
General and Emergency Surgery Department, School of Medicine and Surgery, Milano-Bicocca University, Monza, Italy
Marco Ceresoli
Department of Surgery, College of Medicine and Health Science, United Arab Emirates University, Abu Dhabi, United Arab Emirates
Fikri M. Abu-Zidan
Department of Surgery, Stanford University, Stanford, CA, USA
Kristan L. Staudenmayer
General and Emergency Surgery Department, Bufalini Hospital, Cesena, Italy
Fausto Catena
Department of General, Emergency and Trauma Surgery, Pisa University Hospital, Pisa, Pisa, Italy
Federico Coccolini
Rights and permissions
Reprints and permissions
Copyright information
© 2022 The Author(s), under exclusive license to Springer Nature Switzerland AG
About this chapter
Fu, S., Arnow, K., Trickey, A., Knowlton, L.M. (2022). Difference-In-Difference Techniques and Causal Inference. In: Ceresoli, M., Abu-Zidan, F.M., Staudenmayer, K.L., Catena, F., Coccolini, F. (eds) Statistics and Research Methods for Acute Care and General Surgeons. Hot Topics in Acute Care Surgery and Trauma. Springer, Cham. https://doi.org/10.1007/978-3-031-13818-8_11
Download citation
DOI : https://doi.org/10.1007/978-3-031-13818-8_11
Published : 14 December 2022
Publisher Name : Springer, Cham
Print ISBN : 978-3-031-13817-1
Online ISBN : 978-3-031-13818-8
eBook Packages : Medicine Medicine (R0)
Share this chapter
Anyone you share the following link with will be able to read this content:
Sorry, a shareable link is not currently available for this article.
Provided by the Springer Nature SharedIt content-sharing initiative
- Publish with us
Policies and ethics
- Find a journal
- Track your research
Causal Inference with Latent Variables: Recent Advances and Future Prospectives
New citation alert added.
This alert has been successfully added and will be sent to:
You will be notified whenever a record that you have chosen has been cited.
To manage your alert preferences, click on the button below.
New Citation Alert!
Please log in to your account
Information & Contributors
Bibliometrics & citations, view options, index terms.
Mathematics of computing
Probability and statistics
Probabilistic representations
Causal networks
Recommendations
Inference in multi-agent causal models.
In this article, we demonstrate the usefulness of causal Bayesian networks as probabilistic reasoning systems. The biggest advantage of causal Bayesian networks over traditional probabilistic Bayesian networks is that they sometimes allow to perform ...
Disentangling causality: assumptions in causal discovery and inference
Causality has been a burgeoning field of research leading to the point where the literature abounds with different components addressing distinct parts of causality. For researchers, it has been increasingly difficult to discern the assumptions ...
Causal inference through a witness protection program
One of the most fundamental problems in causal inference is the estimation of a causal effect when treatment and outcome are confounded. This is difficult in an observational study, because one has no direct evidence that all confounders have been ...
Information
Published in.

- General Chairs:
Northeastern University, USA
CENTAI / Eurecat, Italy
- SIGMOD: ACM Special Interest Group on Management of Data
- SIGKDD: ACM Special Interest Group on Knowledge Discovery in Data
Association for Computing Machinery
New York, NY, United States
Publication History
Check for updates, author tags.
- causal inference
- confounding analysis
- latent variable models
Funding Sources
- The JP Morgan Chase Faculty Research Award
- Snap Gift Funding
- National Science Foundation
- The Cisco Faculty Research Award
- The Commonwealth Cyber Initiative Awards
Acceptance Rates
Contributors, other metrics, bibliometrics, article metrics.
- 0 Total Citations
- 0 Total Downloads
- Downloads (Last 12 months) 0
- Downloads (Last 6 weeks) 0
View options
View or Download as a PDF file.
View online with eReader .
Login options
Check if you have access through your login credentials or your institution to get full access on this article.
Full Access
Share this publication link.
Copying failed.
Share on social media
Affiliations, export citations.
- Please download or close your previous search result export first before starting a new bulk export. Preview is not available. By clicking download, a status dialog will open to start the export process. The process may take a few minutes but once it finishes a file will be downloadable from your browser. You may continue to browse the DL while the export process is in progress. Download
- Download citation
- Copy citation
We are preparing your search results for download ...
We will inform you here when the file is ready.
Your file of search results citations is now ready.
Your search export query has expired. Please try again.
- Health, Fitness & Dieting
- Psychology & Counseling
Sorry, there was a problem.

Download the free Kindle app and start reading Kindle books instantly on your smartphone, tablet, or computer - no Kindle device required .
Read instantly on your browser with Kindle for Web.
Using your mobile phone camera - scan the code below and download the Kindle app.

Image Unavailable

- To view this video download Flash Player

Follow the author

Experimental and Quasi-Experimental Designs for Generalized Causal Inference 2nd Edition
- ISBN-10 0395615569
- ISBN-13 978-0395615560
- Edition 2nd
- Publisher Cengage Learning
- Publication date January 2, 2001
- Language English
- Dimensions 7 x 1 x 8.75 inches
- Print length 656 pages
- See all details
Products related to this item

Editorial Reviews
About the author.
Thomas D. Cook (Ph.D., 1967, Stanford University) is a professor of sociology, psychology, education and social policy, as well as a Faculty Fellow, Institute of Policy Research at Northwestern University. His major research interests include examining routes out of poverty and methodology, dealing with the design and execution of social experiments, methods for promoting causal generalization, and theories of evaluation practice. Dr. Cook has written or edited seven books and has published numerous articles and book chapters. He was the recipient of the Myrdal Prize for Science from the Evaluation Research Society in 1982 and the Donald Campbell Prize for Innovative Methodology from the Policy Sciences Organization in 1988. He is a trustee of the Russell Sage Foundation and a member of its committee on the Future of Work.
Product details
- Publisher : Cengage Learning; 2nd edition (January 2, 2001)
- Language : English
- Paperback : 656 pages
- ISBN-10 : 0395615569
- ISBN-13 : 978-0395615560
- Item Weight : 1.95 pounds
- Dimensions : 7 x 1 x 8.75 inches
- #165 in Medical Psychotherapy TA & NLP
- #199 in Popular Psychology Psychotherapy
- #451 in Medical General Psychology

About the author
William r. shadish.
Discover more of the author’s books, see similar authors, read author blogs and more

Customer reviews
- 5 star 4 star 3 star 2 star 1 star 5 star 70% 11% 6% 6% 7% 70%
- 5 star 4 star 3 star 2 star 1 star 4 star 70% 11% 6% 6% 7% 11%
- 5 star 4 star 3 star 2 star 1 star 3 star 70% 11% 6% 6% 7% 6%
- 5 star 4 star 3 star 2 star 1 star 2 star 70% 11% 6% 6% 7% 6%
- 5 star 4 star 3 star 2 star 1 star 1 star 70% 11% 6% 6% 7% 7%
Customer Reviews, including Product Star Ratings help customers to learn more about the product and decide whether it is the right product for them.
To calculate the overall star rating and percentage breakdown by star, we don’t use a simple average. Instead, our system considers things like how recent a review is and if the reviewer bought the item on Amazon. It also analyzed reviews to verify trustworthiness.
Customers say
Customers find the book great for understanding and strengthening statistical methods. They also appreciate the ease of process and outstanding condition.
AI-generated from the text of customer reviews
Customers find the book easy to understand and useful for strengthening statistical methods. They also say it provides enough details to develop ideas into research questions. Readers say the book is very useful for PhD research. Some find it hard to read and understand, but it's critical for research. Overall, customers are satisfied with the comprehensibility and the author's personable writing style.
"...It is by far the most sophisticated and thoughtful analysis of the experimental approach to social research, and explores in depth some issues..." Read more
"...not been disappointed- Shadish explains the techniques in an easy-to-understand manner , with enough details to develop ideas into research questions...." Read more
"A great reference text , but a very slow read." Read more
"I think this book is the most comprehensive and thoughtful treatment of experimental and quasi-experimental designs...." Read more
Customers appreciate the craftsmanship of the book. They also say it is in outstanding condition.
" good quality and delivered just in time." Read more
" Outstanding condition !" Read more
" Great condition ...." Read more
Customers find the writing style of the book poor, dense, and verbose.
"...So far it’s difficult to stay focused. Wordy and lengthy...." Read more
" Poorly written . Boring." Read more
"Dense, verbose & incredibly boring..." Read more
- Sort reviews by Top reviews Most recent Top reviews
Top reviews from the United States
There was a problem filtering reviews right now. please try again later..

Top reviews from other countries

- About Amazon
- Investor Relations
- Amazon Devices
- Amazon Science
- Sell products on Amazon
- Sell on Amazon Business
- Sell apps on Amazon
- Become an Affiliate
- Advertise Your Products
- Self-Publish with Us
- Host an Amazon Hub
- › See More Make Money with Us
- Amazon Business Card
- Shop with Points
- Reload Your Balance
- Amazon Currency Converter
- Amazon and COVID-19
- Your Account
- Your Orders
- Shipping Rates & Policies
- Returns & Replacements
- Manage Your Content and Devices
- Conditions of Use
- Privacy Notice
- Consumer Health Data Privacy Disclosure
- Your Ads Privacy Choices
All Subjects
1.4 Hypothesis testing
8 min read • august 20, 2024
Hypothesis testing is a crucial statistical method in causal inference. It helps researchers make decisions about population parameters based on sample data, using null and alternative hypotheses to assess the significance of treatment effects and compare groups in experiments.
The process involves formulating hypotheses, selecting appropriate tests, and interpreting results. Key concepts include significance levels, p-values, and different types of errors. Researchers must consider limitations and practical significance when drawing conclusions about causal relationships.
Hypothesis testing overview
- Hypothesis testing is a statistical method used to make decisions about population parameters based on sample data
- It involves formulating a null hypothesis ( H 0 H_0 H 0 ) and an alternative hypothesis ( H a H_a H a ), and using probability to determine whether to reject or fail to reject the null hypothesis
- Hypothesis testing is a crucial tool in causal inference for assessing the significance of treatment effects and comparing groups in experiments
Null and alternative hypotheses
- The null hypothesis ( H 0 H_0 H 0 ) states that there is no significant difference or relationship between variables, or that a parameter equals a specific value
- The alternative hypothesis ( H a H_a H a ) is the opposite of the null hypothesis and represents the claim being tested, such as the existence of a significant difference or relationship
- H 0 H_0 H 0 : The mean weight of a population is 150 lbs; H a H_a H a : The mean weight is not 150 lbs
- H 0 H_0 H 0 : There is no association between smoking and lung cancer; H a H_a H a : There is an association between smoking and lung cancer
Significance level and p-values
- The significance level ( α \alpha α ) is the probability threshold for rejecting the null hypothesis, typically set at 0.05 or 0.01
- The p-value is the probability of observing a test statistic as extreme as or more extreme than the one calculated from the sample data, assuming the null hypothesis is true
- If the p-value is less than the significance level, the null hypothesis is rejected; otherwise, we fail to reject the null hypothesis
- A smaller p-value provides stronger evidence against the null hypothesis
One-tailed vs two-tailed tests
- A one-tailed test is used when the alternative hypothesis specifies a direction (greater than or less than), focusing on one tail of the distribution
- A two-tailed test is used when the alternative hypothesis does not specify a direction (not equal to), considering both tails of the distribution
- The choice between a one-tailed or two-tailed test depends on the research question and prior knowledge about the direction of the effect
Type I and Type II errors
- The probability of a Type I error is equal to the significance level ( α \alpha α )
- The probability of a Type II error is denoted by β \beta β
- The goal is to minimize both types of errors, but there is a trade-off between them
Power of a test
- The power of a test is the probability of rejecting the null hypothesis when it is actually false (i.e., correctly detecting a significant effect)
- Power is calculated as 1 − β 1 - \beta 1 − β , where β \beta β is the probability of a Type II error
- Factors that influence power include sample size, effect size , significance level, and test type
- Higher power reduces the risk of Type II errors and increases the likelihood of detecting true effects
Common hypothesis tests
- Various hypothesis tests are used depending on the type of data, distribution, and research question
- Some common tests include the z-test , t-test , chi-square test , and F-test
- Each test has specific assumptions and is suitable for different scenarios
Z-test for population mean
- The z-test is used to test hypotheses about a population mean when the population standard deviation is known and the sample size is large (n > 30) or the population is normally distributed
- The test statistic ( z z z ) is calculated as: z = x ˉ − μ 0 σ / n z = \frac{\bar{x} - \mu_0}{\sigma / \sqrt{n}} z = σ / n x ˉ − μ 0 , where x ˉ \bar{x} x ˉ is the sample mean, μ 0 \mu_0 μ 0 is the hypothesized population mean, σ \sigma σ is the population standard deviation, and n n n is the sample size
- The z-test assumes that the data are independently and identically distributed (i.i.d.) and that the population is normally distributed
T-test for sample mean
- The t-test is used to test hypotheses about a population mean when the population standard deviation is unknown and the sample size is small (n < 30), or when comparing the means of two independent or paired samples
- The test statistic ( t t t ) is calculated as: t = x ˉ − μ 0 s / n t = \frac{\bar{x} - \mu_0}{s / \sqrt{n}} t = s / n x ˉ − μ 0 , where x ˉ \bar{x} x ˉ is the sample mean, μ 0 \mu_0 μ 0 is the hypothesized population mean, s s s is the sample standard deviation, and n n n is the sample size
- The t-test assumes that the data are i.i.d. and that the population is normally distributed or that the sample size is large enough for the Central Limit Theorem to apply
Chi-square test for independence
- The chi-square test is used to test for the independence of two categorical variables
- It compares the observed frequencies in a contingency table to the expected frequencies under the null hypothesis of independence
- The test statistic ( χ 2 \chi^2 χ 2 ) is calculated as: χ 2 = ∑ ( O − E ) 2 E \chi^2 = \sum \frac{(O - E)^2}{E} χ 2 = ∑ E ( O − E ) 2 , where O O O is the observed frequency and E E E is the expected frequency for each cell
- The chi-square test assumes that the expected frequencies are not too small (usually at least 5) and that the observations are independent
F-test for equality of variances
- The F-test is used to test for the equality of variances between two populations
- It compares the ratio of the sample variances to determine if they are significantly different
- The test statistic ( F F F ) is calculated as: F = s 1 2 s 2 2 F = \frac{s_1^2}{s_2^2} F = s 2 2 s 1 2 , where s 1 2 s_1^2 s 1 2 and s 2 2 s_2^2 s 2 2 are the sample variances of the two populations
- The F-test assumes that the data are i.i.d., the populations are normally distributed, and the samples are independent
Steps in hypothesis testing
- Hypothesis testing follows a systematic procedure to ensure valid and reliable results
- The steps include formulating hypotheses, selecting an appropriate test, calculating the test statistic, determining the critical value, and making a decision based on the p-value
Formulating hypotheses
- Clearly state the null hypothesis ( H 0 H_0 H 0 ) and the alternative hypothesis ( H a H_a H a ) based on the research question and available information
- Ensure that the hypotheses are mutually exclusive and exhaustive
- Consider the implications of rejecting or failing to reject the null hypothesis
Selecting appropriate test
- Choose the appropriate hypothesis test based on the type of data (categorical or numerical), the distribution of the data (normal or non-normal), the sample size, and the research question
- Consider the assumptions of each test and whether they are met by the data
- Determine whether a one-tailed or two-tailed test is appropriate based on the alternative hypothesis
Calculating test statistic
- Calculate the test statistic using the appropriate formula for the selected hypothesis test
- Substitute the sample data and hypothesized values into the formula
- Double-check the calculations to ensure accuracy
Determining critical value
- Determine the critical value(s) based on the significance level ( α \alpha α ) and the type of test (one-tailed or two-tailed)
- Use statistical tables or software to find the critical value(s) for the specific test and degrees of freedom
- The critical value(s) represent the boundary between the rejection and non-rejection regions of the distribution
Making decision to reject or fail to reject
- Compare the calculated test statistic to the critical value(s) or calculate the p-value
- If the test statistic falls in the rejection region or the p-value is less than the significance level, reject the null hypothesis; otherwise, fail to reject the null hypothesis
- Interpret the decision in the context of the research question and the implications for the study
Interpreting results
- After conducting a hypothesis test, it is essential to interpret the results correctly and consider their implications
- Interpretation should include confidence intervals, effect sizes, practical significance, and limitations of the test
Confidence intervals
- A confidence interval is a range of values that is likely to contain the true population parameter with a certain level of confidence (usually 95%)
- Confidence intervals provide more information than a simple hypothesis test by indicating the precision and uncertainty of the estimate
- A narrow confidence interval suggests a more precise estimate, while a wide confidence interval indicates greater uncertainty
Effect size and practical significance
- The effect size measures the magnitude of the difference or relationship between variables, independent of the sample size
- Common effect size measures include Cohen's d, Pearson's r, and odds ratios
- Practical significance refers to the real-world importance or relevance of the effect, beyond statistical significance
- A statistically significant result may not be practically significant if the effect size is small or the consequences are minimal
Limitations of hypothesis testing
- It does not prove causality, only the existence of a significant relationship or difference
- It is sensitive to sample size, with large samples more likely to yield significant results even for small effects
- It can be affected by violations of assumptions, such as non-normality or lack of independence
- It does not account for multiple testing, which can inflate the Type I error rate
- Researchers should be cautious in drawing conclusions based solely on hypothesis tests and consider other factors, such as study design, data quality, and theoretical plausibility
Applications in causal inference
- Hypothesis testing is a key tool in causal inference for assessing the significance of treatment effects and comparing groups in experiments
- It helps researchers determine whether observed differences or relationships are likely due to chance or to a genuine causal effect
Testing for significant treatment effects
- In randomized controlled trials (RCTs) and other experimental designs, hypothesis testing is used to assess the significance of the difference between treatment and control groups
- A significant result suggests that the treatment has a causal effect on the outcome, while a non-significant result indicates that the observed difference could be due to chance
- Example: Testing whether a new drug significantly reduces blood pressure compared to a placebo
Comparing groups in experiments
- Hypothesis testing is also used to compare multiple groups in experiments, such as different treatment conditions or subpopulations
- Tests like ANOVA (analysis of variance) and post-hoc comparisons help determine which groups differ significantly from each other
- Example: Comparing the effectiveness of three different teaching methods on student performance
Assessing validity of causal claims
- Hypothesis testing can be used to assess the validity of causal claims made in observational studies or quasi-experiments
- By testing for significant associations between variables or differences between groups, researchers can evaluate the strength of the evidence for a causal relationship
- However, hypothesis testing alone cannot establish causality, as other factors like confounding and reverse causation must be considered
Hypothesis testing vs estimation approaches
- While hypothesis testing focuses on making decisions about the existence of effects or differences, estimation approaches aim to quantify the size and uncertainty of effects
- Estimation methods, such as confidence intervals and Bayesian analysis, provide more informative results than simple hypothesis tests
- In causal inference, a combination of hypothesis testing and estimation approaches is often used to assess the significance, magnitude, and precision of causal effects
Key Terms to Review ( 24 )
© 2024 fiveable inc. all rights reserved., ap® and sat® are trademarks registered by the college board, which is not affiliated with, and does not endorse this website..

An official website of the United States government
The .gov means it’s official. Federal government websites often end in .gov or .mil. Before sharing sensitive information, make sure you’re on a federal government site.
The site is secure. The https:// ensures that you are connecting to the official website and that any information you provide is encrypted and transmitted securely.
- Publications
- Account settings
Preview improvements coming to the PMC website in October 2024. Learn More or Try it out now .
- Advanced Search
- Journal List
- Am J Epidemiol

The Future of Causal Inference
The past several decades have seen exponential growth in causal inference approaches and their applications. In this commentary, we provide our top-10 list of emerging and exciting areas of research in causal inference. These include methods for high-dimensional data and precision medicine, causal machine learning, causal discovery, and others. These methods are not meant to be an exhaustive list; instead, we hope that this list will serve as a springboard for stimulating the development of new research.
Editor’s note: The opinions expressed in this article are those of the authors and do not necessarily reflect the views of the American Journal of Epidemiology.
Over the past few decades there have been major achievements in the development of causal inference theory and methods and in a range of applications. Foundational advancements in modern causal inference have come from diverse fields, including epidemiology, biostatistics, statistics, computer science, and economics. Seminal work by James Heckman, Judea Pearl, James Robins, Paul Rosenbaum, and Donald Rubin (among others) led to ground-breaking changes in how problems are approached and data are analyzed. For example, researchers who are well versed in causal inference ideas will typically take great care in defining the population of interest, specifying the target causal parameter(s), assessing identifying assumptions using subject matter knowledge (possibly with the help of directed acyclic graphs (DAGs)), designing the study to emulate a target trial, choosing efficient and robust estimators, and carrying out sensitivity analysis. In the past 40 years, novel approaches such as propensity scores, instrumental variables, mediation analysis, and methods for estimating optimal dynamic treatment regimens have been developed and are now commonly used by applied researchers to answer impactful questions.
As codirectors of the Center for Causal Inference (a joint partnership between the University of Pennsylvania and Rutgers University), we are naturally passionate about causal inference. It is heartening to see the exponential growth in causal inference methodology and the explosion of applications in medicine, education, sociology, and public policy. It has also been exciting to see the increase in interest in causal inference among our graduate students. When the 3 of us were in graduate school in the 1990s, there were very few formal courses in causal inference. Now, most doctoral programs in statistics, epidemiology, and biostatistics offer semester-long courses, and there is a nice cadre of excellent textbooks ( 1 – 3 ). There is also now the newly formed Society for Causal Inference (SCI), which will bring together causal researchers across different disciplines to foster research collaborations and enhance training opportunities.
We are delighted to have been invited by the Editor-in-Chief of the Journal to write a commentary on the future of causal inference. Based on our collective experiences and having polled our colleagues currently engaged in cutting-edge causal inference research, we provide here our top-10 list of new and exciting areas of research in causal inference. These are not listed in any specific order of importance and are not meant to be a review of existing methods. Nor do we intend this list to be exhaustive, by any means. Instead, we hope that this list will serve as a springboard for stimulating the development of new research. The future of causal inference is bright. There is much to do and many, many bright new minds to do it.
TOP 10 FUTURE DIRECTIONS FOR CAUSAL INFERENCE RESEARCH
High-dimensional data.
High-dimensional data are increasingly available for researchers in both randomized trials and observational studies. This opens possibilities to learn about a broader range of causal questions more reliably, but it also presents statistical challenges. High-dimensional data can come in many forms, including high-dimensional exposures, confounders, and mediators. In all these cases, statistical approaches could involve variable selection or other types of dimension reduction (along the lines of principal components analysis). Careful consideration needs to be made for the plausibility of the causal identifying assumptions as well as the interpretability. Further, in these high-dimensional data problems there might be tradeoffs between making strong assumptions on the dimension-reduction part of the problem or on the modeling part of the problem.
Arguably, the most common type of high-dimensional data problem in causal inference is high-dimensional confounding. Because valid causal inference often requires an “ignorability” type of an assumption, having access to a large amount of potential confounders is valuable. Variable selection problems here are more complex than in standard prediction modeling problems because, ideally, we will include variables that affect the treatment decision (propensity score) and the outcome. There is a small but growing literature on methods that take both types of relationships into account in variable selection (as discussed elsewhere ( 4 – 6 )). Other types of dimension-reduction approaches run the risk of invalidating the ignorability assumption, although the bias-variance tradeoff might make this acceptable. Examples of high-dimensional exposures could include genetic variants ( 7 ), environmental exposures ( 8 ), or multidimensional treatments with some continuous components ( 9 ). It a distinct challenge to determine how one can reduce the dimension of the exposure while still having interpretable estimands that allow for possible exposure interaction effects. Recent motivating examples in the literature for high-dimensional mediation include genomics data and functional magnetic resonance imaging data as mediating variables. In addition to the challenges mentioned above in terms of defining causal effects and assessing the plausibility of identifying assumptions, high-dimensional mediation also has another challenge. Because the mediators can potentially be affected by the exposure(s), we might want to capture change in the mediators (pre/post exposure). Suppose the mediators are microbiome data or genomic data. How might we account for preexposure values and changes in these variables after treatment?
Precision medicine
Precision medicine is an idea that has received a lot of attention over the past decades, from excitement about the potential impact of the Human Genome Project to President Obama’s Precision Medicine Initiative, through to the present day. It can be thought of as using available data to determine what treatment is best for an individual and delivering it at the right time. Although the idea of precision medicine was largely motivated by choosing medications or other medical interventions based on an individual’s genomic data or other biomarkers, we can also think more generally, beyond just medicine, to tailored interventions and policies.
One of the key statistical questions for precision medicine is, “given a person’s historical data (including prior treatments), what is the best course of action?” Breakthroughs in the statistical literature on estimating optimal dynamic (adaptive) treatment strategies occurred in the early 2000s ( 10 , 11 ). Since then, we have seen many advances in statistical methodology, as well as some real-world applications of the methods. Some recent statistical developments have made it possible to allow frequent, irregular measurement times, and to get even more precise on when to monitor or deliver interventions. For example, taking into account the costs of obtaining data (such as the monetary cost of obtaining biomarker values or the cost of a person’s time or interest in responding to survey questions), one could determine both the optimal monitoring plan and the optimal intervention given data obtained from that monitoring plan ( 12 , 13 ). The opportunities for precision medicine are furthered using mobile devices (mHealth) in health care and research. Microrandomized trials are designed to take advantage of mobile technology to have real-time data collection, randomization, and delivery of interventions ( 14 ). While there have been some statistical advances for estimating dynamic treatment strategies from mHealth data (as in Luckett et al. ( 15 )), we believe there will be a lot of development in the next decade. In addition, there is a particularly strong need here for team science (close collaboration with clinical researchers) and translation (software, training).
Causal machine learning
Causal inference approaches involve study design, defining causal estimands, identifying (causal) assumptions, and statistical modeling. In order to specify, for example, a propensity score model or an outcome model (or both) to make causal inference, we need to learn about observed data distributions or functions (such as mean functions). Machine learning methods allow analysts to avoid making strong parametric assumptions, potentially leading to a reduction in risk of bias due to model misspecification. Causal machine learning differs from standard machine learning in key ways. We are not trying to predict what will happen next given the way the world currently is. In causal machine learning, we are trying to predict what would happen if a particular aspect about the world changed. (For example, what if everyone in our population of interest followed a particular treatment plan?) Because of this, before machine learning methods are implemented, careful thought needs to be given to the design of the study, what variables to include in which models, and so on. There is much to be done in this area of research.
Enriching randomized experiments with real-world data
Randomized experiments provide an unbiased way to estimate treatment effects by dividing units into treatment and control groups in an unbiased manner by “a flip of a coin”. However, randomized experiments are expensive and difficult to conduct, and are often done only among subgroups of the population of interest. Observational studies, which rely on “real-world” data, have opposite advantages and disadvantages: They can be severely biased by confounding because people themselves rather than coins decide on who is in the treatment and control group, but they are less expensive and can be conducted considering the whole population of interest.
An important area of research is how to best combine evidence from observational studies and randomized trials in making causal inferences. This could be seen as a form of meta-analysis that combines evidence from different types of studies. Examples of how such combinations are useful in the context of studying the effect of hormone replacement therapy for postmenopausal women are given by Prentice et al. ( 16 ). An example of work on combining evidence from randomized experiments and observational studies is Kaizar ( 17 ), in which estimates were made of the treatment effect among the part of the population where the randomized experiment was conducted and then the observational study was used to estimate how different the part of the population among which the trial had been conducted was from the part of the population among which the trial had not been conducted. Another way in which real-world data can enrich randomized trials is by enabling improved trial design. An example is Shortreed et al. ( 18 ), which uses electronic health record data to make more realistic sample size calculations in a suicide prevention randomized trial.
Algorithmic fairness and social responsibility
Machine learning, deep learning, and artificial intelligence approaches are often used to develop clinical algorithms to aid with risk prediction and decision making for treating patients. Private industries, government agencies, and the criminal justice system also use these approaches to determine insurance rates and hiring practices, perform facial recognition, and predict recidivism when making sentencing recommendations ( 19 ). These tools, however, are only as good as the data that are used to develop them. Often, the data sources are biased and suffer from deep-rooted social and systemic inequities and injustices. In order to address these issues head-on, careful thought must be given to the underlying causal pathways that give rise to unfair practices, such as disparities in access to health care. Kusner and Loftus ( 20 ) lay out ways in which causal modeling may help assess the fairness or bias of such algorithms by thinking in terms of counterfactuals (e.g., would the prediction from the model change if we changed one feature of an individual, such as their race) and by conducting sensitivity analyses of the algorithms to assess whether they could be biased because of unknown or unmeasured factors. As machine learning approaches are being developed and improved to leverage massive amounts of data, in parallel, principled data collection and causal methods need to be developed to aid in the training and assessment of algorithms to mitigate hidden discrimination and unfair practices. As Kusner and Loftus recommend, epidemiologists and statisticians should work closely with ethicists, social scientists, clinicians, stakeholders (e.g., patients that the algorithm may affect), and others in an interdisciplinary fashion so that algorithms are based on rich and diverse data and incorporate critical features in the causal pathway.
Distributed learning
We have seen the term “distributed learning” used in several different ways, and all of it could end up being important for causal inference problems. For example, it often refers to distributing computational workload across many machines to help with scalability ( 21 ). Deep learning models might require an extremely large number of parameters and enormous amount of training data, and, if centralized, might not be feasible.
Distributed learning aims to distribute the workload while finding cohesive ways to integrate the information. As we discuss in the section on causal machine learning, machine learning methods in general will increasingly play a crucial role in causal inference. Another use of the term has to do with using multiple data sets that cannot be combined or merged. For example, several health-care systems might be willing to allow models to be fitted to their data and to share the output but not to share granular patient data. This kind of privacy-preserving approach will likely be increasingly common in the future. How best to go about causal inference when you can fit models to different data sets from potentially different populations, without having access to the raw data? Many of the other problems that we discuss in this article are also relevant here, such as transportability.
Causal discovery
Much of the causal inference literature begins with assumptions depicted by DAGs and proceeds with methods development given the DAG. Causal discovery, on the other hand, has to do with uncovering causal relationships and structures from observational data using computational and statistical methods. The DAG cannot be identified purely from the data (i.e., it cannot be identified purely from knowing the probabilistic relationships between the observed variables). For example, without any further assumptions, it cannot be known whether an association between X and Y arises from X causing Y , Y causing X , or neither causing the other and instead an unmeasured confounder generating the association. However, under certain assumptions, such as the causal Markov assumption or the causal faithfulness assumption, the DAG can be identified from the data ( 22 ). Under one of these assumptions, traditionally, score-based methods have been used to discover DAGs ( 23 ). In this approach, the space of DAGs is searched for the optimal score using computational algorithms. We anticipate that causal discovery will be an area of substantial development in the coming years. New assumptions under which causal discovery can be undertaken that are motivated by specific applications (e.g., metabolomics, mental health disease pathophysiology) will be needed. Further, new methods may be needed for data gathered under complex conditions, such as having aggregate measurements of causal interactions at a finer scale or data that have a network structure (e.g., some people in the data are friends).
Interference and spillover
In the assessment of public policies, we are often concerned about how to account for the policy “spilling over” to nearby regions. For instance, coronavirus disease 2019 lockdown measures in one city may affect infection rates in nearby towns; residents of the suburbs of Philadelphia may work, go to restaurants, and attend concerts in Philadelphia, and hence, mandates in the city of Philadelphia will affect infection rates in the suburbs in ways that may not be measurable. On the other hand, residents of the suburbs may opt to dine locally rather than venture to Philadelphia. It is also possible that the suburbs may take Philadelphia’s lead and adopt similar behaviors, such as social distancing and masking. Either way, the policy choices of these regions interfere with each other. Assessing the causal effect of spillover and defining relevant estimands of interest are emerging areas of research in causal inference.
When it comes to accounting for spillover, estimands of interest may include the average treatment effect on the treated in the presence of spillover and the average treatment effect on a neighboring control. For instance, there is interest in assessing the causal effect of excise taxes, such as sweetened beverage taxes, on consumption ( 24 ). However, cross-border shopping from a taxed region to a nontaxed neighboring region can mitigate the effect of the tax on the region of interest and also affect the neighboring region’s volume of sales. Similar complexities arise in the assessment of the causal effects of neighborhood policing initiatives on crime ( 25 ). Here, spillover effects of one precinct’s policies on neighboring precincts is critical in understanding the causal effects of such policies. Of course, spillover patterns or behaviors may be influenced by unmeasured confounders complicating the issue but potentially fueling future research. One can imagine an even more complex situation in which the causal effect of air pollution policies on health is of interest. Spillover effects, in this case, must also take into account spatiotemporal correlations ( 26 ). Causal identification and modeling of relevant spillover estimands in these situations are challenges that are yet to be tackled.
Finally, there is currently a vast amount of data being curated, leveraged, and wrangled from social media platforms such as Twitter (San Francisco, California) and Facebook (Menlo Park, California). For instance, there has been interest in studying the association between geographic variability in social media postings and diabetes rates ( 27 ). What if we wanted to assess the causal effects of social media postings on human health? Does the more someone tweets about their diabetes indicate more awareness of their own health as well as influencing others to lead healthier lifestyles? These are social networks that are subject to interference and network dependencies ( 28 ) but are also subject to unmeasured confounding (e.g., sociodemographic factors) and substitution (those who post a lot on Facebook may not post on Twitter or LinkedIn (Sunnyvale, California)).
Transportability
Decision makers are often interested in whether results of a study conducted on a specific population can be transported to another population of interest. This could be in the context of transporting, for instance, the causal average treatment effect from a randomized controlled trial to a different target population. Elegant causal theory and assumptions have been laid out in the setting of transporting causal conclusions from experimental settings to observational settings using a structural causal model framework ( 29 ). There may also be interest in transporting the causal effect of a nonrandomized public health policy from a study population to a target population. For instance, one may ask whether the causal effect of strict gun laws on crime in one state can be transportable to a different state. Methods are needed to account for differences in sociodemographic factors between the study and target populations and also account for spatiotemporal factors. For instance, the target state may be more rural, poorer, and have lower or higher crime rates. Further, the target state may be geographically closer to other states that have weaker gun laws, increasing the likelihood of traveling to another state to purchase guns. Novel methods for transporting causal effects that were originally estimated using, say, difference-in-differences, interrupted time series, or regression discontinuity designs under these complex settings would help investigators to better understand the causal effect of policy interventions in diverse settings. There is also room for developing methods for generalizing causal effects in these complex settings. For example, there may be interest in generalizing the causal effect of a successful drug prevention program in one school to all schools in the district. Careful thought would need to be given to the underlying framework and assumptions, including unmeasured confounding, interference, treatment heterogeneity, and potential mediation.
Quasi-experimental devices
An observational study investigates the effect of a treatment when the treatment is not randomized. A central concern is unmeasured differences between the treatment and control group, other than the treatment. After adjustments have been made for measured covariates in an observational study, an association between treatment and outcome is ambiguous: An association may be an effect caused by the treatment or a reflection of unmeasured differences between the treatment and control groups. Quasi-experimental devices enlarge the set of considered associations with the intention of reducing this ambiguity. Classical quasi-experimental devices include pretreatment outcomes and multiple control groups ( 30 ). Recently, new quasi-experimental devices have been developed such as evidence factors, differential effects, and computerized construction of quasi-experiments ( 31 ). In much of the literature on classical quasi-experimental devices, which was developed by Donald Campbell and collaborators, statistical inference did not play a major role ( 30 , 32 ). One potential future research direction is to work on incorporating statistical inference into the use of quasi-experimental devices. In most of the literature on classical and new quasi-experimental devices, the effect of one or at most a few treatments has been considered. Another important potential research direction is figuring out how current or novel quasi-experimental devices could help to make causal inferences about many treatments working together, as in a gene regulatory network.
Given the massive amount of data that are available today, along with machine learning tools, artificial intelligence tools, and high-performance computing, it is tempting for researchers to take more of a black box approach to answering scientific questions. However, we suggest it is essential for the causal inference community to continue to emphasize the importance of carefully laying out the problem, working with subject-matter experts to understand the data, and thinking carefully about the study design and plausibility of assumptions. We might not be known for flashy branding, but for data science to have the impact we think it can, principled causal inference approaches are critical.
A key consideration that we did not focus on in our list above is advances in estimation of causal estimands. Many of the new approaches we mentioned may benefit from semiparametric, nonparametric, or Bayesian estimation methods that focus on flexibility, multiple robustness, efficiency, and other properties.
We hope that the list above has convinced you that the field of causal inference has a very exciting future ahead. We are sure you can think of other areas of causal inference that would have been justified in claiming a spot in the top 10. We also anticipate that there are areas of research we do not yet know about but that will become critically important in the near future. We are excited to see where the field will go next!
ACKNOWLEDGMENTS
Author affiliations: Department of Biostatistics, Epidemiology and Informatics, University of Pennsylvania, Philadelphia, Pennsylvania, United States (Nandita Mitra); Department of Biostatistics and Epidemiology, Rutgers School of Public Health, Piscataway, New Jersey, United States (Jason Roy); and Department of Statistics, University of Pennsylvania, Philadelphia, Pennsylvania, United States (Dylan Small).
This work was funded by the National Institutes of Health (grants UL1TR003017 (J.R.) and 5R01AG065276-02 (D.S.)).
We thank members of the Center for Causal Inference for their responses to our survey.
The views expressed in this commentary are those of the authors and do not reflect those of the National Institutes of Health.
Conflict of interest: none declared.
arXiv's Accessibility Forum starts next month!
Help | Advanced Search
Statistics > Methodology
Title: anytime-valid inference for double/debiased machine learning of causal parameters.
Abstract: Double (debiased) machine learning (DML) has seen widespread use in recent years for learning causal/structural parameters, in part due to its flexibility and adaptability to high-dimensional nuisance functions as well as its ability to avoid bias from regularization or overfitting. However, the classic double-debiased framework is only valid asymptotically for a predetermined sample size, thus lacking the flexibility of collecting more data if sharper inference is needed, or stopping data collection early if useful inferences can be made earlier than expected. This can be of particular concern in large scale experimental studies with huge financial costs or human lives at stake, as well as in observational studies where the length of confidence of intervals do not shrink to zero even with increasing sample size due to partial identifiability of a structural parameter. In this paper, we present time-uniform counterparts to the asymptotic DML results, enabling valid inference and confidence intervals for structural parameters to be constructed at any arbitrary (possibly data-dependent) stopping time. We provide conditions which are only slightly stronger than the standard DML conditions, but offer the stronger guarantee for anytime-valid inference. This facilitates the transformation of any existing DML method to provide anytime-valid guarantees with minimal modifications, making it highly adaptable and easy to use. We illustrate our procedure using two instances: a) local average treatment effect in online experiments with non-compliance, and b) partial identification of average treatment effect in observational studies with potential unmeasured confounding.
Subjects: | Methodology (stat.ME); Econometrics (econ.EM); Statistics Theory (math.ST); Machine Learning (stat.ML) |
Cite as: | [stat.ME] |
(or [stat.ME] for this version) | |
Focus to learn more arXiv-issued DOI via DataCite |
Submission history
Access paper:.
- HTML (experimental)
- Other Formats

References & Citations
- Google Scholar
- Semantic Scholar
BibTeX formatted citation

Bibliographic and Citation Tools
Code, data and media associated with this article, recommenders and search tools.
- Institution
arXivLabs: experimental projects with community collaborators
arXivLabs is a framework that allows collaborators to develop and share new arXiv features directly on our website.
Both individuals and organizations that work with arXivLabs have embraced and accepted our values of openness, community, excellence, and user data privacy. arXiv is committed to these values and only works with partners that adhere to them.
Have an idea for a project that will add value for arXiv's community? Learn more about arXivLabs .
Experimental Design and Causal Inference
6 units of credit.

IMAGES
VIDEO
COMMENTS
When randomized experiments are infeasible, quasi-experimental designs can be exploited to evaluate causal treatment effects. The strongest quasi-experimental designs for causal inference are regression discontinuity designs, instrumental variable designs, matching and propensity score designs, and comparative interrupted time series designs.
We close by advocating for the cross-pollination of quasi-experimental methods and data science: quasi-experiments can make causal inference possible in typical data science settings, while ...
The randomized control trial (RCT) is the primary experimental design in education research due to its strong internal validity for causal inference. However, in situations where RCTs are not feasible or ethical, quasi-experiments are alternatives to establish causal inference. This paper serves as an introduction to several quasi-experimental designs: regression discontinuity design ...
Techniques for quasi-experimental causal inference are ripe for application in behavioural science and neuroscience. They could fruitfully be applied to existing laboratory data, such as ...
This chapter focuses on quasi-experimental designs that, like that of Bell et al. ( 1995), have both control groups and pretests. The chapter explains how the use of carefully selected comparison groups facilitates causal inference from quasi experiments, but it also argues that such control groups are of minimal advantage unless they are ...
Quasi-experimental designs (QEDs), which first gained prominence in social science research , are increasingly being employed to fill this need ... Previous reviews in this journal have focused on the importance and use of QEDs and other methods to enhance causal inference when evaluating the impact of an intervention that has ...
When randomized experiments are infeasible, quasi-experimental designs can be exploited to evaluate causal treatment effects. The strongest quasi-experimental designs for causal inference are regression discontinuity designs, instrumental variable designs, matching and propensity score designs, and comparative interrupted time series designs. This article introduces for each design the basic ...
We close by advocating for the cross-pollination of quasi-experimental methods and data science: quasi-experiments can make causal inference possible in typical data science settings, while innovations in ML can in turn improve these methods for wider application in complex data domains. ... When applying quasi-experimental methods, like all ...
The strongest quasi-experimental designs for causal inference are regression discontinuity designs, instrumental variable designs, matching and propensity score designs, and comparative interrupted time series designs. This article introduces for each design the basic rationale, discusses the assumptions required for identifying a causal effect ...
A quasi-experiment is an empirical interventional study used to estimate the causal impact of an intervention on target population without random assignment. Quasi-experimental research shares similarities with the traditional experimental design or randomized controlled trial, but it specifically lacks the element of random assignment to ...
Quasi-experimental designs for causal inference: an overview where Y i is participant i 's measured outcome. Simply speak - ing, the ATE, ATT, and ATU are sample mean dierences of the outcome between the treatment and control groups. In quasi-experimental designs where participants are not ran-domly assigned, the independence assumption is ...
We suggest a triangulation approach that compares causal estimates from (quasi-)experimental research with causal estimates generated from observational data and theoretical assumptions.
Experimental and Quasi-Experimental Designs for Generalized Causal Inference. Houghton Mifflin Company. This book covers the design aspect of quasi-experiments and discuses a lot of design elements that are very useful for practical research. However, since it does not cover the analysis of quasi-experiments we will rely on
Experimental and quasi-experimental designs for generalized causal inference. Houghton, Mifflin and Company. Abstract. This is a book for those who have already decided that identifying a dependable relationship between a cause and its effects is a high priority and who wish to consider experimental methods for doing so.
This article is Part 1 of n (depending on how much I end up rambling on) in a series of articles about using quasi-experiments for causal inference. Briefly, Part 1 will explain the whys and hows of quasi-experiments, as well as the nuances involved when applying approaches like PSM. In Part 2, I will talk more about the limitations of quasi ...
Experimental and Quasi-Experimental Designs for Generalized Causal Inference, by William R. Shadish, Thomas D. Cook, and Donald T. Campbell.Boston: Houghton Mifflin ...
Difference-in-difference. Quasi-experimental. Research questions in medicine often center around measuring the effects of new treatments or interventions. We seek to study causal inference, the process of determining why certain outcomes occur. Randomized control trials, or RCTs, have long been considered the gold standard study design to ...
Experimental and quasi-experimental research designs examine whether there is a causal. relationship between independent and dependent variables. Simply de ned, the independent. variable is the ...
The four types of study are: randomized studies, time-series studies, cohort studies, and quasi-experimental studies. Quasi-experimental studies are also referred to as natural experiments (de Vocht et al., 2021) While this is not an exhaustive list of all of the study designs that can inform causal determinations, they are useful to illustrate ...
Causal inference is the process of determining the independent, actual effect of a particular phenomenon that is a component of a larger system. ... Quasi-experimental verification of causal mechanisms is conducted when traditional experimental methods are unavailable. This may be the result of prohibitive costs of conducting an experiment, or ...
Causal inference (CI), which aims to infer intrinsic causal relations among variables of interest, has emerged as a crucial research topic. ... Investigating causality in human behavior from smartphone sensor data: a quasi-experimental approach. EPJ Data Science, Vol. 4, 1 (2015), 24. ... Causal inference for observational and experimental data ...
Amazon.com: Experimental and Quasi-Experimental Designs for Generalized Causal Inference: 8601419432820: Shadish, William R., Cook, Thomas D., Campbell, Donald T.: Books
Experimental and Quasi‐Experimental Designs for Generalized Causal Inference. By William R. Shadish, Thomas D. Cook, and Donald T. Campbell. Boston: Houghton Mifflin, 2002. Pp. 623. $60.36 (paper). ... Jorge Luis Triana EVALUATION OF THE SOCIAL PREVENTION OF CRIME POLICY IN ACAPULCO THROUGH A QUASI-EXPERIMENTAL DESIGN, ...
In causal inference, a combination of hypothesis testing and estimation approaches is often used to assess the significance, magnitude, and precision of causal effects Key Terms to Review ( 24 ) Alternative Hypothesis : The alternative hypothesis is a statement that suggests there is a statistically significant effect or difference in a given ...
3 Active Causal Inference Learning. 3.1 Randomized Controlled Trial; 3.2 Causal inference with contextual bandits. 3.2.1 Estimating ATE from interventional data collected with a bandit method; 3.2.2 Learning a causal graph using a bandit algorithm; 3.2.3 Correcting a bias from unobserved confounders; 4 Passive Causal Inference. 4.1 A Naive ...
The past several decades have seen exponential growth in causal inference approaches and their applications. In this commentary, we provide our top-10 list of emerging and exciting areas of research in causal inference. ... Quasi-experimental devices. An observational study investigates the effect of a treatment when the treatment is not ...
View PDF HTML (experimental) Abstract: Double (debiased) machine learning (DML) has seen widespread use in recent years for learning causal/structural parameters, in part due to its flexibility and adaptability to high-dimensional nuisance functions as well as its ability to avoid bias from regularization or overfitting. However, the classic double-debiased framework is only valid ...
Print Experimental Design and Causal Inference page. bookmark_border. Experimental Design and Causal Inference. MATH3852. 6 Units of Credit. info. open_in_new. eLearning. Information on eLearning, IT support and apps for students. open_in_new. Ask a question. All your UNSW Handbook questions answered here ...