ACM Transactions on Cyber-Physical Systems

Volume 8, Issue 3

- Computation Abstractions
- System Modeling and Languages
- System Compositionality and Integration
- Design Automation and Tool Chains
- Trustworthy System Designs
- Resilient and Robust System Designs
- Human in the Loop


Subject Areas
Announcements.
ACM Updates Its Peer Review Policy
ACM is pleased to announce that its Publications Board has approved an updated Peer Review Policy . If you have any questions regarding the update, the associated FAQ addresses topics such as confidentiality, the use of large language models in the peer review process, conflicts of interest, and several other relevant concerns. If there are any issues that are not addressed in the FAQ, please contact ACM’s Director of Publications, Scott Delman .
New ACM Policy on Authorship ACM has a new Policy on Authorship , covering a range of key topics, including the use of generative AI tools. Please familiarize yourself with the new policy and the associated list of Frequently Asked Questions .
Most Frequent Affiliations
Most cited authors, latest issue.
- Volume 8, Issue 3 July 2024 ISSN: 2378-962X EISSN: 2378-9638 View Table of Contents
DistressNet-NG: A Resilient Data Storage and Sharing Framework for Mobile Edge Computing in Cyber-Physical Systems
Department of Computer Science and Engineering, Texas A&M University, College Station, USA
Department of Computer Science and Software Engineering, Miami University, Oxford, USA
Department of Computer Engineering, University of Sharjah, Sharjah, UAE
National Institute of Standards and Technology (NIST), Boulder, USA
Experimentation and Implementation of the BFT++ Cyber-Attack Resilience Mechanism for Cyber-Physical Systems
MITRE, McLean, USA
CSAFA Labs, SCP, Georgia Institute of Technology, Atlanta, USA
Recent Award Winners
Most popular, other acm journals.

Volume 2, Issue 2

Volume 3, Issue 2
April-June 2024

Volume 56, Issue 12
December 2024

Volume 5, Issue 2

Volume 36, Issue 2
Export Citations
- Please download or close your previous search result export first before starting a new bulk export. Preview is not available. By clicking download, a status dialog will open to start the export process. The process may take a few minutes but once it finishes a file will be downloadable from your browser. You may continue to browse the DL while the export process is in progress. Download
- Download citation
- Copy citation
We are preparing your search results for download ...
We will inform you here when the file is ready.
Your file of search results citations is now ready.
Your search export query has expired. Please try again.
- Email Alert
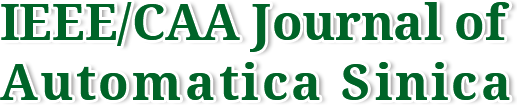
论文 全文 图 表 新闻
- Abstracting/Indexing
- Journal Metrics
- Current Editorial Board
- Early Career Advisory Board
- Previous Editor-in-Chief
- Past Issues
- Current Issue
- Special Issues
- Early Access
- Online Submission
- Information for Authors
- Share facebook twitter google linkedin
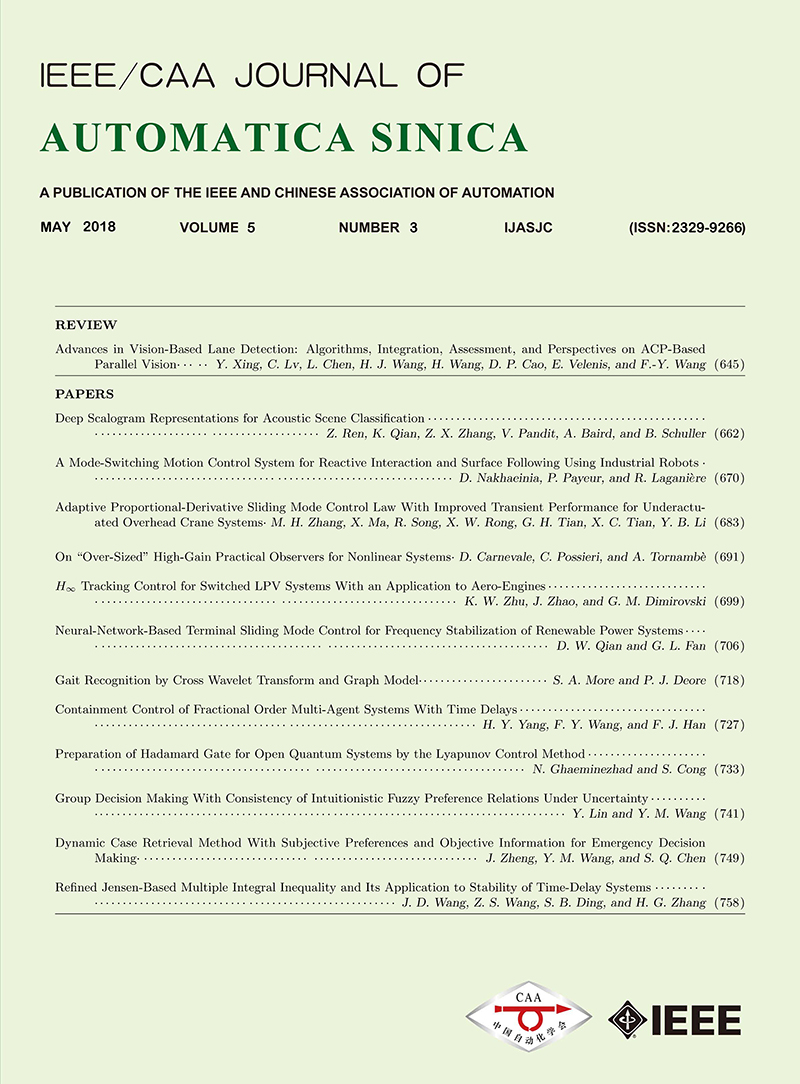
IEEE/CAA Journal of Automatica Sinica
- JCR Impact Factor: 15.3 , Top 1 (SCI Q1) CiteScore: 23.5 , Top 2% (Q1) Google Scholar h5-index: 77, TOP 5
Xinping Guan, Bo Yang, Cailian Chen, Wenbin Dai and Yiyin Wang, "A Comprehensive Overview of Cyber-Physical Systems: From Perspective of Feedback System," , vol. 3, no. 1, pp. 1-14, 2016. |
Xinping Guan, Bo Yang, Cailian Chen, Wenbin Dai and Yiyin Wang, "A Comprehensive Overview of Cyber-Physical Systems: From Perspective of Feedback System," , vol. 3, no. 1, pp. 1-14, 2016. |
A Comprehensive Overview of Cyber-Physical Systems: From Perspective of Feedback System
- Xinping Guan ,
- Bo Yang ,
- Cailian Chen ,
- Wenbin Dai ,
Department of Automation, Shanghai Jiao Tong University, and Key Laboratory of System Control and Information Processing, Ministry of Education of China, Shanghai 200240, China
This work was supported by National Natural Science Foundation of China (61221003, 61174127, 61573245, 61273181, 61503247, 61301223), and Shanghai Municipal Science and Technology Commission (15QA1402300, 14511107903).
- Revised Date: 2015-08-18
- Cyber-physical systems (CPS) ,
- system modeling ,
- information acquisition ,
- communication ,
- control ,
Proportional views
通讯作者: 陈斌, [email protected]
沈阳化工大学材料科学与工程学院 沈阳 110142
Article Metrics
- PDF Downloads( 144 )
- Abstract views( 1662 )
- HTML views( 28 )
- Copyright © 2022 IEEE/CAA Journal of Automatica Sinica
- 京ICP备14019135号-24
- E-mail: [email protected] Tel: +86-10-82544459, 10-82544746
- Address: 95 Zhongguancun East Road, Handian District, Beijing 100190, China
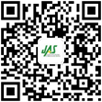
Export File

Thank you for visiting nature.com. You are using a browser version with limited support for CSS. To obtain the best experience, we recommend you use a more up to date browser (or turn off compatibility mode in Internet Explorer). In the meantime, to ensure continued support, we are displaying the site without styles and JavaScript.
- View all journals
- Explore content
- About the journal
- Publish with us
- Sign up for alerts
- Open access
- Published: 13 May 2022
Quantum cyber-physical systems
- Javier Villalba-Diez ORCID: orcid.org/0000-0002-2423-1474 1 , 2 ,
- Ana González-Marcos 3 &
- Joaquín Ordieres-Meré ORCID: orcid.org/0000-0002-9677-6764 4
Scientific Reports volume 12 , Article number: 7964 ( 2022 ) Cite this article
2727 Accesses
3 Citations
12 Altmetric
Metrics details
- Quantum simulation
This paper aims to promote a quantum framework that analyzes Industry 4.0 cyber-physical systems more efficiently than traditional simulations used to represent integrated systems. The paper proposes a novel configuration of distributed quantum circuits in multilayered complex networks that enable the evaluation of industrial value creation chains. In particular, two different mechanisms for the integration of information between circuits operating at different layers are proposed, where their behavior is analyzed and compared with the classical conditional probability tables linked to the Bayesian networks. With the proposed method, both linear and nonlinear behaviors become possible while the complexity remains bounded. Applications in the case of Industry 4.0 are discussed when a component’s health is under consideration, where the effect of integration between different quantum cyber-physical digital twin models appears as a relevant implication.
Similar content being viewed by others

Recovery coupling in multilayer networks
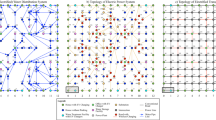
A tensor-based formulation of hetero-functional graph theory

Quantum certification and benchmarking
Introduction.
Cyber-physical systems (CPS) are integrations of computational and physical components that can interact with humans through new and different modalities. A key to future technological development is precisely this new and different capacity of interaction together with the new possibilies that these systems pose for expanding the capabilities of the physical world through computation, communication and control 1 . When CPS are understood within the industrial practice fueled by additional technologies such as Internet of Things (IoT), people refer to the Industry 4.0 paradigm 2 . The design of many industrial engineering systems has been performed by separately considering the control system design from the hardware and/or software implementation details. That is, after a first design and verification stage in which the control system is exhaustively simulated, the uncertainty of the generated model and random disturbances are addressed by means of ad hoc adjustment methods, which has been time-consuming and costly in the maintenance of functional and operational systems when several subsystems are integrated 1 .
Leading Industry 4.0 processes to the coordinated achievement of objectives is a probabilistic process in which decision–makers can never be certain that the choice being made is the correct one 3 . As a consequence, value-creating networks can be considered as decision networks or probabilistic directed acyclic graphical models 4 , 5 with known conditional probabilities, and such network per process, when considered as an ensemble, is nothing else than a multiple complex system. Multiple complex systems can be found as well in many other fields such as the human nervous system 6 , forests 7 , city transportation systems 8 , social networks 9 or insect colonies 10 , which present a multilayered hierarchical network structure. The emergence of this type of configuration confers on the system a series of evolutionary fitness advantages 11 such as the development of distributed swarm intelligence 12 , systemic learning through information aggregation 13 , effective goal achievement and complex problem solving 14 , or greater resilience to changes 15 . Indeed, the strategic design of organizations takes such complexity into account 16 for cyber-physical systems of Industry 4.0 17 , where they start to be understood as socio-technical complex networked configurations at various levels of complexity 18 , 19 , 20 , 21 .
The behavioral simulation of individual systems in Industry 4.0 is frequently addressed by means of digital twins (DTs). Although the DT concept is well established, it is usually crafted in different ways depending on the application and discipline, and they have the vision for representing physical assets, allowing different component models 22 . Most of the efforts in creating DTs are spent in gathering data and training models. However, little efforts have been done to exploit the hierarchical relationship between systems in an integrated way. Therefore, a significant gap regarding DTs is the lack of integration 23 , not only at the same level, losing the capability of promoting their interactions fostering the information value chain, but even vertically, where the upper hierarchical levels are not aware of the status of subsystems or components, relevant for their processing status. The main reason is because of the high complexity involved, which makes it hard to consider all components at all hierarchical levels.
Quantum computation processes information using the laws of quantum mechanics, which endows it with a high computational capacity compared to classical computers 24 . It has opened new ways of solving some problems, e.g., in machine learning 25 , finance 26 , or human interaction 27 . Industry 4.0 problems using machine learning are likely to benefit from quantum models of computation 3 . A quantum simulation may also be used to optimize the configuration of the cyber-physical resources that conform to these systems. It has already been shown how quantum simulations can be used to describe networks of interdependent resources 28 , 29 , 30 .
While a CPS has both physical and software components deeply intertwined in such a way it operates on different spatial and temporal scales, interacting with each other in ways that change with context, we define Quantum Cyber Physical Systems (QCPS) as a CPS in which its mechanism is controlled or monitored by quantum-based algorithms.
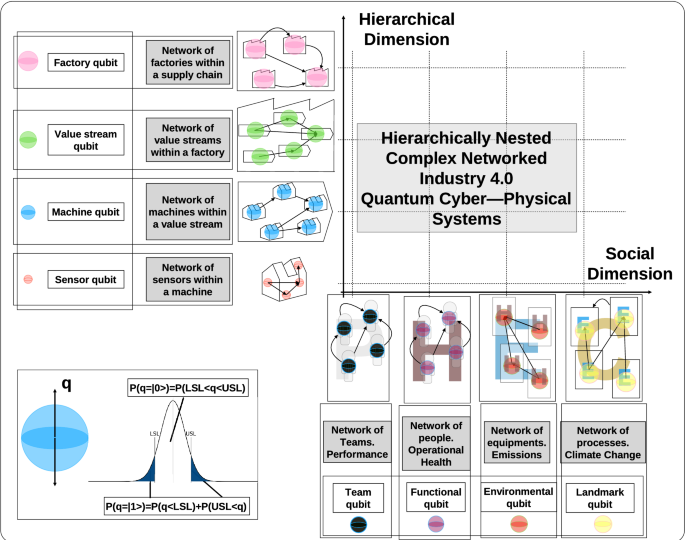
Quantum industry 4.0 cyber-physical systems.
In this article, we combine the system theory as well as its digital translation and the use of quantum decision networks to propose a quantum framework for Industry 4.0 cyber-physical systems, able to deal with the hierarchical interdependent DT. As shown in the graphical abstract of Fig. 1 , we propose a two-dimensional socio-technical multilayered network framework in which the resources that make up the cyber-physical system are modeled as a network of qubits or, in their simplest configuration, through qubits. Each of these elements presents a probability of being in the desired process specifications that is represented by the qubit position.
To clarify the proposed architecture, for the exhibited example in the Fig. 1 , it is important to understand that the interest was to model and to represent two different behaviors for the elements or subsystems, such as normal (inside the expected range) or abnormal (outside of it). Such a status can be described as a probability at a specific time and it can be represented by a quantum bit (qubit), in a similar way to other approaches that use quantum computing to simulate diffusion through networks 31 .
Therefore, such representation is used on the technical axis to describe the status of a sensor or network of sensors, of a machine or network of machines, of a value stream of resources, or a network of value streams, etc.
On the other axis, this time talking about the nontechnical human or social dimensions of the organization, different interests can be also described in a probabilistic way. In the case of a team (or a network of teams), we could be interested in estimating their performance, or their alignment as normal (inside the expected range through time) or abnormal. However, the focus could be a group of people, where the relevant parameter being described in a probabilistic way could be their operational health, or other dimensions. Indeed, the interest can also be the effect of devices or processes over social agents at a short scale (emissions) or at a larger scale (contribution to climate change).
Each system component is independent and connected to others as defined by the users, facilitating the assembly of a multilayered network structure. This allows users to assess different strategies according to various situations or configurations. In the example used as reference, the interest is to model how abnormalities at specific level impact in higher levels of hierarchy. Furthermore, the paper develops methods to evaluate the effects of resource or system failure at a specific level as well as its propagation and impact. This proposal can be seen as another perspective of the utilization of quantum computing, a similar hierarchical perspective presented by Ref. 32 in their vision for Quantum Internet.
This paper is organized as follows. Second Section describes quantum multilayered networks. The third section evaluates the implementation of the hierarchical relationship. The fourth section discusses the results. Finally, the fifth section presents our conclusions, limitations, and future research steps.
Quantum multilayered networks
Reading the proposed framework, each of the two dimensions of the socio-technical phase space can be understood as a specific space, then different layers are considered inside each space. We have separated the aggregation levels as follows:
At the social level, each layer is defined by a network of agents reporting to each other 33 , where performance, operational health, emissions, and carbon footprint have been identified 22 , 34 , 35 as core elements.
Similarly, at the technical level, the machine state is defined by a network of sensors 36 , the value stream state is defined by a network of machines 37 , the factory state is defined by a network of value streams 38 , and the supply chain state is defined by a network of factories defining the supra-organizational state 39 , 40 .
In general, we can state that Industry 4.0 systems can be designed as a network of processes 41 and the failure of one of these processes leads to a performance loss of the system 42 . We aim to inspect the behavior of the system when some of its elements are not operating within the standard operating procedure tolerances 43 . Industrial processes are designed following strict standard operating procedures that constrain them within certain limits, to ensure the competitiveness and quality of the products 44 , 45 .
The information describing Industry 4.0 systems is typically managed by a series of key performance indicators (KPIs). Such KPIs are interdependent and describe certain characteristics of the processes that conform to the value creation of certain products 46 . To ensure the necessary conditions of quality, cost, etc., demanded by customers, these KPIs and their respective statistical distributions are constantly monitored by control processes. Our proposal assigns one qubit \(q\) to each of the cyber-physical resources of the multilayered socio-technical network. Specifically, the probability that the cyber-physical resource is within the specifications defined in the standard operating procedure will be assigned as the probability of \(P(q=|0\rangle )\) and \(P(q=|1\rangle )\) otherwise (see Fig. 1 ). These resources will be linked to others to build a system, depending on the aspect under consideration in such a way that multilayer network becomes a natural architecture. Indeed, the state space of a composite physical system is the tensor product of the state spaces of the component physical systems 47 , and this principle can be easily translated to the quantum environment. Therefore, in this work it is proposed a hybrid framework for analyzing classical data coming from different sources through quantum networks.
Bloch’s sphere is commonly used to geometrically represent a qubit 48 . A qubit can be represented as a point on the Bloch sphere with the help of two parameters ( \(\theta\) , \(\phi\) ), as expressed by \(|\Psi \rangle =cos\left( \frac{\theta }{2} \right) |0\rangle + e^{i\phi }sin\left( \frac{\theta }{2} \right) |1\rangle\) . When several qubits are utilized in a circuit describing a layer of \(M\) elements, their aggregated state can be determined utilizing the tensorial product given by \(|\Psi \rangle =|\Psi _{1}\rangle \otimes |\Psi _{2}\rangle \otimes ...\otimes |\Psi _{M}\rangle\) 47 . This tensorial product maps the entry \(x \in \mathbb {C}^n\) in a complex Hilbert space \(\mathscr {H}\) , which for \(n\) qubits is the \(\mathbb {C}^{2^{n}}\) . For simplicity reasons, we will. For the sake of simplicity, we will henceforth dispense with the notation by mentioning the entry \(x\) .
A multilayer network \(\mathscr {M}\) is given by the quadruplet \(\mathscr {M}=(\Gamma _{M},\mathscr {E}_{M},V ,\mathscr {L})\) , in which \(\Gamma _{M}\) indicates the set of node-layer tuplets related to a set of nodes \(V\) , and \(\mathscr {L}\) representing the set of perspectives built upon a set of elementary layers being relevant to the set of aspects \(\mathscr {A}\) 49 . Therefore, a multilayer network can have N \(_{A}\) number of aspects, being N \(_{A}\) the cardinality of \(\mathscr {A}\) . Based on those aspects a sequence of sets of layers is defined as \(\mathscr {L}\) = \(\{\) L \(_{\alpha }\) \(\}\) , \(\alpha \in (1,2,\dots\) ,N \(_{A}\) ) , where L \(_{\alpha }\) represents the set of layers related to the \(\alpha\) aspect. The whole group of layers are built based on the cartesian product of the sets as per aspect, \(L_{1} \times L_{2} \times \dots \times L_{N_{A}}\) , and then \(\Gamma _{M} \subseteq V \times L_{1} \times L_{2} \times \dots \times L_{N_{A}}\) . The nodes can be connected pairwise by means of edges, \(\mathscr {E}_{M} \subseteq \Gamma _{M} \times \Gamma _{M}\) where according to the definition, connections can happen inside layers or inter-layers. The multilayer network can still be represented as graph \(\mathscr {G}_{M} = (\Gamma _{M},\mathscr {E}_{M})\) .
For any given time \(t\) complex cyber-physical networks have been formally described 21 as time-dependent graphs given by Eq. ( 1 ):
which can be understood as lists of \(\Gamma _{M}(t)\) human and cyber-physical nodes and its standard communication \(\mathscr {E}_{M}(t)\subset (\Gamma _{M}(t)x\Gamma _{M}(t))\) edges 43 . Continuous improvement-oriented standardization of business communication protocols between network elements (i.e., organizational network edges) is the only way to produce lean structural networks from complex networked organizational design configurations 43
Within this time interval \(\Delta t\) , the graph \(\mathscr {G}_{M}(t)\) described in Eq. ( 1 ) converts into a decision network \(\mathscr {G}_{M}(t)'=(\Gamma _{M}(t)',\mathscr {E}_{M}(t)')\) formed by a set of \(\Gamma _{M}(t)'\) nodes and \(\mathscr {E}_{M}(t)'\) edges, where \(\Gamma _{M}(t)'=(\gamma _{M1}(t), \gamma _{M2}(t), \ldots , \gamma _{MN}(t))\) represents the set of all the nodes being part of the network in \(\Delta t\) , and the edges represent interactions between nodes. Let \(\gamma _{Mi}(t)\) be a parent node and \(\gamma _{Mj}(t)\) a child node. The edge between nodes \(\gamma _{Mi}(t)\) and \(\gamma _{Mj}(t)\) are determined by the known probabilistic dependence occurrence on node \(\gamma _{Mj}(t)\) related to the occurrence on \(\gamma _{Mi}(t)\) . Subsequently, as described by Ref. 50 , the joint probability of the nodes can be decomposed into the product of the marginal probabilities given by Eq. ( 2 ):
This property shall be used later on for a proper representation of lean complex cyber-physical networks through quantum circuits.
These qubits form decision networks that can be simulated with quantum circuits 3 , 27 , 29 , 30 . In this approach, an amplitude encoding feature map is implemented. For a root node (qubit) with no parents—i.e., it does not depend on any other node, there are two possible states: \(|0\rangle\) and \(|1\rangle\) . The initialization of these qubits is implemented by translating the conditional probabilities depending on their decision network dependencies into qubit rotation angles. Therefore the rotation angle \(\theta\) required to calculate the probabilities of being in state \(|0\rangle\) and \(|1\rangle\) can be expressed by Eq. ( 3 ):
In general, for a child node \(\Gamma _{i}\) with m parents there are \(2^{m}\) possible states \(\prod \Gamma ^{*}_{i}\) and the rotation angle is given by Eq. ( 4 ):
This rotation angle can be seen as a special case of the rotation angle proposed by Ref. 51 for initializing a quantum register to an arbitrary superposed quantum state and used in 52 for the preparation stage in their estimation on the upper bound of quantum cloning machine. Similarly, the rotation angle given in Eqs. ( 3 ) and ( 4 ) provides appropriate amplitudes for the basis states \(|0\rangle\) and \(|1\rangle\) .
Implementation of the hierarchical relationship
According to the already introduced multilayer network configuration, this paper proposes a quantum multilayered network that presents several computational advantages: on the one hand, the state of each qubit can be fully computed as a wave function of the quantum circuit that conforms it at a lower level. This allows for an effective computation of the interactions between different layers and greatly reduces the computational resources needed to elaborate virtual representations of the system as compared with other approaches such as twin factories 53 . On the other hand, it allows for a distributed ledger computation of organizational states and can therefore flexibly and securely evaluate different decision network configurations and aggregate them into greater settings, hence enabling researchers and organizational designers to use advanced quantum simulations to accelerate managerial decision making.
Without loss of generality, in the modeling of Industry 4.0 processes, we can always add a qubit at the end of the quantum circuit that measures a characteristic of the circuit that we are interested in measuring (i.e. quality, cost,...) and that condenses the conditional probabilities of the rest of the circuit. As a consequence, the aggregation of the wave function \(|\Psi _{\alpha _{j},i}^{l}\rangle\) to the next level \(l+1\) in the position \(j\) is performed by two nodes: one that describes the initial rotation that represents the \(|0\rangle\) probability of the last node of the circuit \(P(|\Psi _{\alpha _{j},N_{j}}^{l}\rangle =|0\rangle )\) which absorbs the initial rotation of the level \(l\) , and a new qubit that contains the conditional probabilities of the node in the level \(l+1\) . Since the root nodes at level l+1 do not have conditional probabilities, they only present the initial rotation. This is shown in Fig. 2 a.
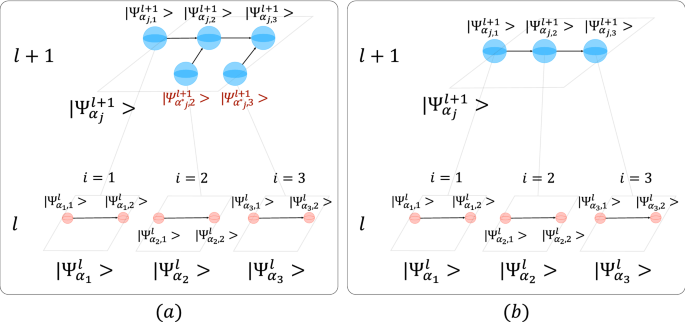
Aggregation of two layers network. Three qubits case. ( a ) First approach ( b ) Second approach. Each node (circle) of the network is represented by a qubit (e.g., sensor qubit in level \(l\) and machine qubit in level \(l+1\) ). Interactions between nodes (qubits) are represented by edges in the direction of influence.
Therefore, the number of additional qubits required to represent the interlayer connections is equal to the number of child nodes in the level \(l+1\) . For illustration, the quantum circuit at level \(l+1\) represented in Fig. 2 a uses two additional qubits ( \(|\Psi _{\alpha ^{*}_{j},2}^{l+1}\rangle\) and \(|\Psi _{\alpha ^{*}_{j},3}^{l+1}\rangle\) ). The initial state of all the qubits in the quantum circuit is \(|0\rangle\) (state of no failure). Then, an initial rotation with \(\theta\) angles that are conditioned on the states of the last node of the circuits at level \(l\) is applied to the root nodes at level \(l+1\) and the new qubits that represent the interlayer connections:
\(P(|\Psi _{\alpha _{j},1}^{l+1}\rangle =|0\rangle ) = P(|\Psi _{\alpha _{1},2}^{l}\rangle =|0\rangle )\)
\(P(|\Psi _{\alpha ^{*}_{j},2}^{l+1}\rangle =|0\rangle ) = P(|\Psi _{\alpha _{2},2}^{l}\rangle =|0\rangle )\)
\(P(|\Psi _{\alpha ^{*}_{j},3}^{l+1}\rangle =|0\rangle ) = P(|\Psi _{\alpha _{3},2}^{l}\rangle =|0\rangle )\) .
The calculation of the initial rotation angles is given by Eq. ( 3 ).
In the case of child nodes \(|\Psi _{\alpha _{j},2}^{l+1}\rangle\) and \(|\Psi _{\alpha _{j},3}^{l+1}\rangle\) , it is necessary to define a set of probabilities conditioned on the values of the corresponding parent nodes (e.g., \(|\Psi _{\alpha ^{*}_{j},2}^{l+1}\rangle\) and \(|\Psi _{\alpha _{j},1}^{l+1}\rangle\) for node \(|\Psi _{\alpha _{j},2}^{l+1}\rangle\) , and \(|\Psi _{\alpha ^{*}_{j},3}^{l+1}\rangle\) and \(|\Psi _{\alpha _{j},2}^{l+1}\rangle\) for node \(|\Psi _{\alpha _{j},3}^{l+1}\rangle\) ). Thus, \(P(|\Psi _{\alpha _{j},i}^{l+1}\rangle =|1\rangle \vert |\Psi _{\alpha ^{*}_{j},i}^{l+1},\Psi _{\alpha _{j},i-1}^{l+1}\rangle =|ab\rangle )\) , where \(i\in \{2,3\}\) and \(|ab\rangle \in \{|11\rangle ,|10\rangle ,|01\rangle ,|00\rangle \}\) , represents the probability of failure of node \(|\Psi _{\alpha _{j},i}^{l+1}\rangle\) conditioned to the state of failure and/or no-failure of nodes \(|\Psi _{\alpha ^{*}_{j},i}^{l+1}\rangle\) and \(|\Psi _{\alpha _{j},i-1}^{l+1}\rangle\) .
The quantum circuit designed with this first approach can be represented by a Bayesian network. Rotation gates in the quantum circuit represent the marginal probabilities associated with root nodes, and controlled rotation gates represent the conditional probability tables associated with child nodes 5 . Thus, the results of the application of this strategy can be compared with the results of the equivalent Bayesian network. As shown in Figs. 3 and 4 , the results obtained with both implementations, i.e, the quantum circuit and the classical Bayesian network, are the same. Failure probability propagation from the root nodes—which represent the state of the systems at level \(l\) —towards the final node—which represents the state of the system at level \(l+1\) —depends on:
The set of conditional probabilities that quantifies the effect of the parent nodes on a child. As Fig. 3 illustrates, higher probabilities of failure conditioned on the state of failure of both parent nodes (from \(0.7\) to \(0.95\) in our example) lead to a greater performance loss of the system.
The distance between the root node in a state of failure and the final child node. In our example, the root node \(|\Psi _{\alpha ^{*}_{j},3}^{l+1}\rangle\) has the greatest impact on the performance loss of the system (see Fig. 3 c,e or f).
The number of root nodes, i.e., systems at level \(l\) , in a state of failure.
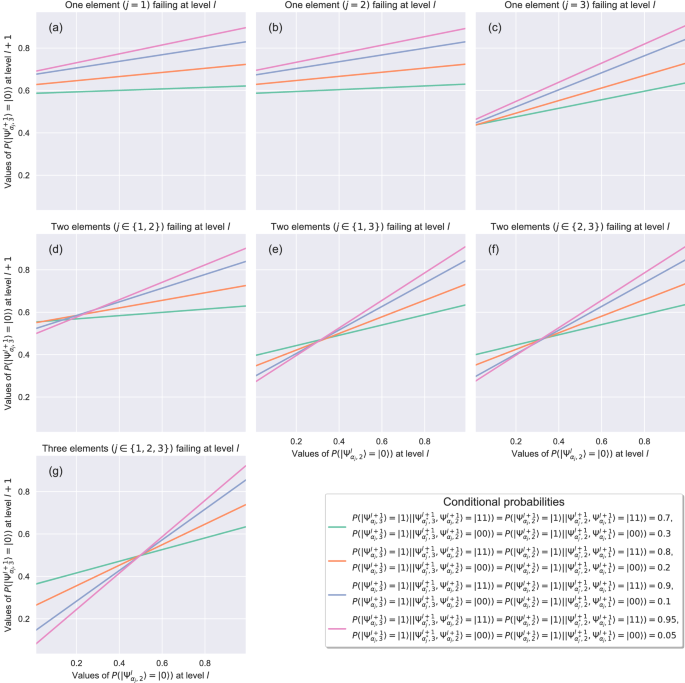
Performance loss of the system at level \(l+1\) for different failure behaviors at level \(l\) , different combinations of \(P(|\Psi _{\alpha _{j},i}^{l+1}\rangle =|1\rangle \vert |\Psi _{\alpha ^{*}_{j},i}^{l+1},\Psi _{\alpha _{j},i-1}^{l+1}\rangle =|ab\rangle )\) , where \(|ab\rangle \in \{|11\rangle ,|00\rangle \}\) , and \(P(|\Psi _{\alpha _{j},i}^{l+1}\rangle =|1\rangle \vert |\Psi _{\alpha ^{*}_{j},i}^{l+1},\Psi _{\alpha _{j},i-1}^{l+1}\rangle =|10\rangle )=P(|\Psi _{\alpha _{j},i}^{l+1}\rangle =|1\rangle \vert |\Psi _{\alpha ^{*}_{j},i}^{l+1},\Psi _{\alpha _{j},i-1}^{l+1}\rangle =|01\rangle )=0.5\) . First approach. Three qubits case.
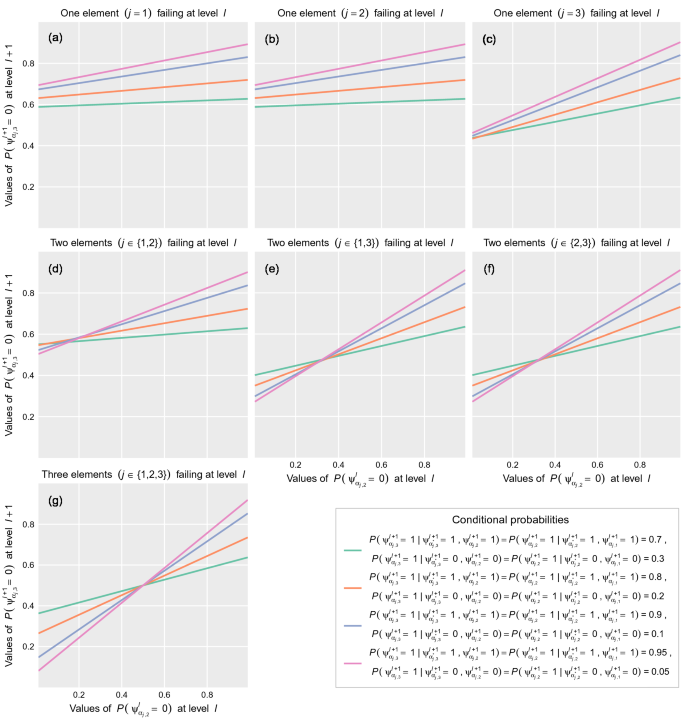
Performance loss of the system at level \(l+1\) for different failure behaviors at level \(l\) , different combinations of \(P(\Psi _{\alpha _{j},i}^{l+1}=1\vert (\Psi _{\alpha ^{*}_{j},i}^{l+1},\Psi _{\alpha _{j},i-1}^{l+1})=(a,b))\) , where \((a,b)\in \{(1,1),(0,0)\}\) , and \(P(\Psi _{\alpha _{j},i}^{l+1}=1\vert (\Psi _{\alpha ^{*}_{j},i}^{l+1},\Psi _{\alpha _{j},i-1}^{l+1})=(1,0))=P(\Psi _{\alpha _{j},i}^{l+1}=1\vert (\Psi _{\alpha ^{*}_{j},i}^{l+1},\Psi _{\alpha _{j},i-1}^{l+1})=(0,1))=0.5\) . First approach. Equivalent Bayesian network.
A different proposed approach to connect different layers in the network is to use the state of the last node of the circuit in the level \(l\) as initial state of nodes in the level \(l+1\) instead of being initialized to \(|0\rangle\) . In this case, the rotation angle can be expressed by Eq. ( 5 ):
Thus, \(\theta =0\) if the last node of the circuit in the level \(l\) has a no-failure state, \((P(|\Psi _{\alpha _{j},N_{j}}^{l}\rangle =|0\rangle )=1)\) , and the initial state of the qubits in the level \(l+1\) is set to \(|0\rangle\) , i.e, state of no-failure. On the other hand, as long as the system in the level \(l\) is not operating properly \((P(|\Psi _{\alpha _{j},N_{j}}^{l}\rangle =|0\rangle ) \in [0,1))\) , the value of \(\theta\) increases from \(0\) to \(\pi /2\) , which leads the initial state of the qubits in the level \(l+1\) towards a state of failure.
This second approach, which is represented in Fig. 2 b, eliminates the use of additional qubits as in the first approach. After the computation of the initial state of nodes in level \(l+1\) by applying internal rotation with angles based on Eq. ( 5 ), the wave function of the quantum circuit is calculated. To describe the case showed in Fig. 2 b, the following probabilities associated with each node need to be defined:
\(P(|\Psi _{\alpha _{j},1}^{l+1}\rangle =|1\rangle )\) . Probability of failure of node \(|\Psi _{\alpha _{j},1}^{l+1}\rangle\) .
\(P(|\Psi _{\alpha _{j},i}^{l+1}\rangle =|1\rangle \vert |\Psi _{\alpha _{j},i-1}^{l+1}\rangle =|a\rangle )\) , where \(i\in \{2,3\}\) and \(|a\rangle \in \{|1\rangle ,|0\rangle \}\) . Probability of failure of node \(|\Psi _{\alpha _{j},i}^{l+1}\rangle\) conditioned to the state of failure or no-failure of node \(|\Psi _{\alpha _{j},i-1}^{l+1}\rangle\) .
The results of the application of this second strategy (Fig. 5 ) show a non-linear behavior of the system when some of its elements are in a state of failure. In this case, the initialization performed by applying internal rotations modifies the initial probability amplitude of quantum states and changes how the information propagates through the quantum circuit. Figure 5 shows how the dominating factors for the performance loss of the system are both the increasing number of nodes (i.e., systems at level \(l\) ) in a state of failure and their decreasing distance to the final node. Furthermore, as the almost parallel lines in Fig. 5 c,e,f,g illustrate, the impact of conditional probabilities on the state of the final node decreases with both increasing number of nodes in a state of failure and decreasing distance between failure nodes and the final one, which results in a saturation point where the performance loss of the system becomes flat.
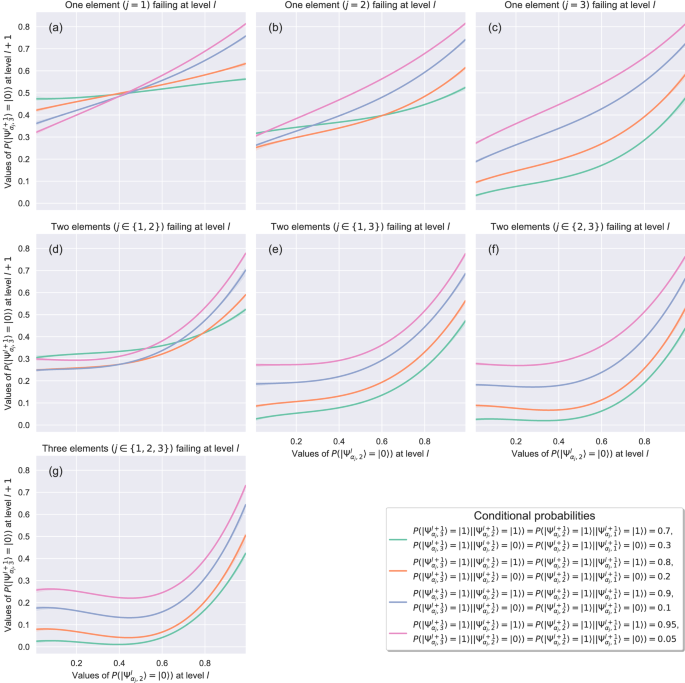
Performance loss of the system at level \(l+1\) for different failure behaviors at level \(l\) and different combinations of \(P(|\Psi _{\alpha _{j},i}^{l+1}\rangle =|1\rangle \vert |\Psi _{\alpha _{j},i-1}^{l+1}\rangle =|a\rangle )\) , where \(i\in \{2,3\}\) and \(|a\rangle \in \{|1\rangle ,|0\rangle \}\) . Second approach. Three qubits case.
An alternative representation of the quantum state is applied to an entry \(x\) \(\langle \Psi (x|\) is given by an Hermitian operator \(\rho (x)=|\Psi (x)\rangle \langle \Psi (x)|\) called density matrix which contains all the observable information of the quantum state. Quantum circuits map therefore the input into a high-dimensional feature space in which statistical properties of the measurement \(M\) are interpreted as output of the quantum circuit. These measurements, which correspond to a Hermitian operator \(\mathscr {M}\) acting on vectors in the Hilbert space of the quantum circuit \(\mathscr {H}\) and live in a subspace of the data-encoding feature space \(\mathscr {F}\) are in general not linear in the Hilbert space \(\mathscr {H}\) of the quantum circuit 54 . However, according to the celebrated representer theorem 55 , an optimal quantum kernel can be found that allows describing the quantum circuits as linear models in the space of the Hermitian operator \(\rho (x)\) with the form \(tr\left[ \left( \sum _{m=1}^{M}\alpha _{m}\rho (x^m) \right) \rho (x)\right]\) where \(x^m\) , \(m=1, \ldots ,M\) is the input data and \(\alpha _{m}\in \mathbb {R}\) . In other words, if we find a linear transformation of our quantum state vector \(|\Psi (x)\rangle\) , we are guaranteed that the best measurements for our quantum circuit only has \(M<<2^{2n}\) degrees of freedom, rather than the \(\mathscr {O}(2^{2n})\) degrees of freedom of a quantum circuit with n qubits.
As shown in Fig. 2 a, and the related results in Fig. 3 , the first quantum model presented in this work does exactly this: by understanding the data-encoding density matrices \(\rho (x)\) as feature vectors, it describes a novel quantum kernel that allows the aggregation of hierarchical networks of qubits in such a way that the quantum models behave linearly in the space of the resulting operator when describing the observable information of the quantum state \(|\Psi (x)\rangle\) . In contrast, the non-linearity of the second model, shown in Fig. 2 b, and the related results depicted in Fig. 5 , shows dissipating effects in the translation of the failure probabilities from one level \(l\) to the next level \(l+1\) derived from the internal rotation imposed on the qubits in the hierarchical aggregation 56 . This means that while the circuit has a high-dimensional state space, the quantum model with additional qubits can be operated in a low-dimensional subspace without dissipation, while the model with internal rotations cannot. This representation can be very useful for several applications, but perhaps the most important one is that it allows us to study the temporal evolution of multilayered networked qubit systems given by Eq. ( 1 ) from an optimization point of view: minimizing the cost functions represented in the space of quantum circuits would be equivalent to minimizing the same cost functions of the resulting system after the proposed transformation.
Conclusions
In this paper, a comprehensive framework for articulating different behaviors of components of systems has been proposed. Feasibility of the integrated behavior is obtained through quantum computing circuits describing the connected interaction between elements of those components at every significant level. The proposed framework allows to represent cyber-physical complex networks of components and systems in Industry 4.0 applications, enabling a systematic and quantitative analysis of these systems. This takes into consideration that Industry 4.0 resources can be flexibly activated in different layers inside different processes to create different products. The flexibility of the presented approach comes as it enables the description of different perspectives attending a variety of socio-technical dimensions of the system, preserving its integrated hierarchical description.
The application to the Industry 4.0 case has a particular added value, as it can contribute to efficiently bring vertical and horizontal integration to the digital representation of subsystems, reached through the DT concept, but where every single subsystem on every single dimension requires its particular DT. However, describing the behavior of the whole system requires strong integration capabilities from the quantitative point of view, as more sources of variability need to be considered.
To illustrate the capabilities of the proposed framework, a detailed analysis of the vertical integration between two hierarchical layers of components of a CPS interacting horizontally has been discussed. The adopted criteria of interest are the operational health of the system and its components (non-operating failures), where the failure of components can occur both horizontally and vertically and influence in a different way the system reliability. Without the lack of generalization capability, simple topology configurations were adopted for the multilayered network of components.
This research has also proposed two different effective alternatives for scaling up the aggregated behavior between layers, bringing linear and nonlinear behaviors. The performed simulations associated with this work—quantum simulations have been performed in Python 3.7 and the Bayesian networks have been simulated in R- are available 57 . Explanation of behavior for both alternatives including sensitiveness to failure transmission levels was also undertaken. The results have shown how Industry 4.0 multilayered networks, at any desired scale, can be represented through quantum simulations associated with the conditional probabilities of failure of their cyber-physical elements. They can successfully represent the health of the system, where the computational advantages are relevant, and the possibility of integration of this quantum simulation paradigm in DT Industry 4.0 environments has been demonstrated.
Although Industry 4.0 manufacturing systems are one of the most relevant fields for DTs exploitation, the adoption of DTs can also play a promising role for decision making in alternative fields, such as smart farming or smart cities, among others. Thus, the proposed framework can be easily applied to other contexts, such as organizational lean management, where it can facilitate the representation of communications as well as representing the variability of related processes at different organizational levels.
The adoption of the proposed framework will allow the investigation of alternative descriptions for the components, out of the probability representing its health status, which can include a hybrid representation of interests.
In the future, quantum research on complex hierarchical networks should evolve from the discrete representation of systems as ‘extended qubits’ towards a more ‘continuous’ description through variables representing functions. In mathematical terms, instead of representing as the descriptive basis of the density operator, the complex Hilbert space in which it is located, tends towards more complex Hilbert spaces such as that of bounded L2 functions, or other similar ones. Another pending research topic is the associated optimization problems linked to multilayered networks, where cost functions become defined over the network. Finally, it is worth mentioning that the proposed approach is not a new design of quantum neural networks, such as the many proposals and ideas that can be found in the literature 58 , 59 . It can be seen as another perspective of quantum complex networks, which have been shown to be more vulnerable to some network attacks than others 60 , 61 . In this sense, more research is needed to improve information transmission, e.g., quantum spin networks 62 , as well as to allow device independence of security protocols in multisource networks 61 .
Baheti, R. & Gill, H. Cyber-physical systems. Impact Control Technol. 12 , 161–166 (2011).
Google Scholar
Lee, J., Bagheri, B. & Kao, H.-A. A Cyber-Physical Systems architecture for Industry 4.0-based manufacturing systems. Manuf. Lett. 3 , 18–23. https://doi.org/10.1016/j.mfglet.2014.12.001 (2015).
Article Google Scholar
Villalba-Diez, J. & Zheng, X. Quantum strategic organizational design: Alignment in industry 4.0 complex-networked cyber-physical lean management systems. Sensors. https://doi.org/10.3390/s20205856 (2020).
Article PubMed PubMed Central Google Scholar
Nielsen, T. D. & Jensen, F. V. Bayesian Networks and Decision Graphs (Springer Science & Business Media, 2009).
Borujeni, S. E., Nannapaneni, S., Nguyen, N. H., Behrman, E. C. & Steck, J. E. Quantum circuit representation of Bayesian networks. Expert Syst. Appl. 176 , 114768. https://doi.org/10.1016/j.eswa.2021.114768 (2021).
Sporns, O. Networks of the Brain (The MIT Press, 2011).
MATH Google Scholar
Wohlleben, P. Das geheime Leben der Bäume: Was sie fühlen, wie sie kommunizieren. (The hidden life of trees) (Ludwig Buchverlag, 2016).
Aleta, A., Meloni, S. & Moreno, Y. A multilayer perspective for the analysis of urban transportation systems. Sci. Rep. 7 , 1–9. https://doi.org/10.1038/srep44359 (2017) ( ISBN: 2045-2322 Publisher: Nature Publishing Group ).
Oro, E., Pizzuti, C., Procopio, N. & Ruffolo, M. Detecting topic authoritative social media users: A multilayer network approach. IEEE Trans. Multimed. 20 , 1195–1208. https://doi.org/10.1109/TMM.2017.2763324 (2017) ( ISBN: 1520-9210 Publisher: IEEE ).
Finn, K. R., Silk, M. J., Porter, M. A. & Pinter-Wollman, N. The use of multilayer network analysis in animal behaviour. Anim. Behav. 149 , 7–22. https://doi.org/10.1016/j.anbehav.2018.12.016 (2019).
Bianconi, G. Multilayer Networks: Structure and Function (Oxford University Press, 2018).
Book Google Scholar
Cao, M. & Fang, W. Swarm intelligence algorithms for weapon-target assignment in a multilayer defense scenario: A comparative study. Symmetry. https://doi.org/10.3390/sym12050824 (2020).
Baltakys, K., Kanniainen, J. & Emmert-Streib, F. Multilayer aggregation with statistical validation: Application to investor networks. Sci. Rep. 8 , 8198. https://doi.org/10.1038/s41598-018-26575-2 (2018).
Article ADS CAS PubMed PubMed Central Google Scholar
Boccaletti, S. et al. The structure and dynamics of multilayer networks. Phys. Rep. 544 , 1–122. https://doi.org/10.1016/j.physrep.2014.07.001 (2014).
Article ADS MathSciNet CAS PubMed PubMed Central Google Scholar
Bertoni, V. B., Saurin, T. A., Fogliatto, F. S., Falegnami, A. & Patriarca, R. Monitor, anticipate, respond, and learn: Developing and interpreting a multilayer social network of resilience abilities. Saf. Sci. 136 , 105148. https://doi.org/10.1016/j.ssci.2020.105148 (2021).
Burton, R. M., Øbel, B. & Håkonsson, D. D. Organizational Design: A Step-by-Step Approach , 3 edn. (Cambridge University Press, 2015).
Bauernhansl, T., Hompel, M. T. & Vogel-Heuser, B. (eds.) Industrie 4.0 in Produktion, Automatisierung und Logistik: Anwendung \(\cdot\) Technologien \(\cdot\) Migration (Springer Vieweg, 2014).
Baxter, G. & Sommerville, I. Socio-technical systems: From design methods to systems engineering. Interact. Comput. 23 , 4–17 (2011).
Davies, R., Coole, T. & Smith, A. Review of socio-technical considerations to ensure successful implementation of industry 4.0. Proc. Manuf. 11 , 1288–1295. https://doi.org/10.1016/j.promfg.2017.07.256 (2017).
Bogoviz, A. V. Industry 4.0 as a new vector of growth and development of knowledge economy. In Industry 4.0: Industrial Revolution of the 21st Century (eds. Popkova, E. G., Ragulina, Y. V. & Bogoviz, A. V.) 85–91. https://doi.org/10.1007/978-3-319-94310-7_8 (Springer International Publishing, 2019).
Villalba-Diez, J. et al. Geometric deep lean learning: deep learning in industry 4.0 cyber-physical complex networks. Sensors. https://doi.org/10.3390/s20030763 (2020).
Zheng, X., Wang, M. & Ordieres-Mere, J. Comparison of data preprocessing approaches for applying deep learning to human activity recognition in the context of industry 4.0. Sensors 18 , 2146 (2018).
Article ADS Google Scholar
Singh, S. et al. Challenges of Digital Twin in High Value Manufacturing (Tech. Rep, SAE Technical Paper, 2018).
Nielsen, M. A. & Chuang, I. Quantum Computation and Quantum Information (Cambridge University Press, 2010).
Biamonte, J. et al. Quantum machine learning. Nature 549 , 195–202. https://doi.org/10.1038/nature23474 (2017).
Article ADS CAS PubMed Google Scholar
Woerner, S. & Egger, D. J. Quantum risk analysis. NPJ Quantum Inf. 5 , 15. https://doi.org/10.1038/s41534-019-0130-6 (2019).
Villalba-Diez, J., Benito, R. M. & Losada, J. C. Industry 4.0 quantum strategic organizational design configurations. The case of two qubits: One reports to one. Sensors. https://doi.org/10.3390/s20236977 (2020).
Wittek, P. & Gogolin, C. Quantum enhanced inference in Markov logic networks. Sci. Rep. 7 , 45672. https://doi.org/10.1038/srep45672 (2017).
Moreira, C. & Wichert, A. Are quantum-like Bayesian networks more powerful than classical Bayesian networks?. J. Math. Psychol. 82 , 73–83. https://doi.org/10.1016/j.jmp.2017.11.003 (2018).
Article MathSciNet MATH Google Scholar
Moreira, C., Tiwari, P., Pandey, H. M., Bruza, P. & Wichert, A. Quantum-like influence diagrams for decision-making. Neural Netw. 132 , 190–210. https://doi.org/10.1016/j.neunet.2020.07.009 (2020).
Article PubMed MATH Google Scholar
Britt, B. C. Modeling viral diffusion using quantum computational network simulation. Quantum Eng. 2 , e29. https://doi.org/10.1002/que2.29 (2020).
Wehner, S., Elkouss, D. & Hanson, R. Quantum internet: A vision for the road ahead. Science 362 , eaam9288 (2018).
Article ADS MathSciNet Google Scholar
Villalba-Diez, J. The Hoshin Kanri Forest. Lean Strategic Organizational Design , 1st edn (CRC Press. Taylor and Francis Group LLC, 2017).
Villalba-Diez, J., Zheng, X., Schmidt, D. & Molina, M. Characterization of industry 4.0 lean management problem-solving behavioral patterns using EEG sensors and deep learning. Sensors. https://doi.org/10.3390/s19132841 (2019).
Schmidt, D. et al. Industry 4.0 lean shopfloor management characterization using EEG sensors and deep learning. Sensors. https://doi.org/10.3390/s20102860 (2020) ( Publisher: Multidisciplinary Digital Publishing Institute ).
Villalba-Diez, J. et al. Deep learning for industrial computer vision quality control in the printing industry 4.0. Sensors. https://doi.org/10.3390/s19183987 (2019).
Huang, Z., Kim, J., Sadri, A., Dowey, S. & Dargusch, M. S. Industry 4.0: Development of a multi-agent system for dynamic value stream mapping in SMEs. J. Manuf. Syst. 52 , 1–12. https://doi.org/10.1016/j.jmsy.2019.05.001 (2019).
Article CAS Google Scholar
Tortorella, G. L. et al. Designing lean value streams in the fourth industrial revolution era: Proposition of technology-integrated guidelines. Int. J. Prod. Res. 58 , 5020–5033. https://doi.org/10.1080/00207543.2020.1743893 (2020) ( Publisher: Taylor & Francis ).
Cross, R., Singer, J., Colella, S., Thomas, R. & Silverstone, Y. The organizational network fieldbook: Best practices, techniques and exercises to drive organizational innovation and performance , 1 edn (Jossey-Bass, 2010).
Manavalan, E. & Jayakrishna, K. A review of Internet of Things (IoT) embedded sustainable supply chain for industry 4.0 requirements. Comput. Ind. Eng. 127 , 925–953. https://doi.org/10.1016/j.cie.2018.11.030 (2019).
Schuh, G., Potente, T., Varandani, R. & Schmitz, T. Methodology for the assessment of structural complexity in global production networks. Proc. CIRP 7 , 67–72 (2013).
Del Gaudio, D. & Hirmer, P. Seamless integration of devices in industry 4.0 environments. Internet Things 12 , 100321. https://doi.org/10.1016/j.iot.2020.100321 (2020).
Villalba-Diez, J. & Ordieres-Mere, J. Improving manufacturing operational performance by standardizing process management. Trans. Eng. Manag. 62 , 351–360. https://doi.org/10.1109/TEM.2015.2424156 (2015).
Shin, W. S., Dahlgaard, J. J., Dahlgaard-Park, S. M. & Kim, M. G. A quality scorecard for the era of industry 4.0. Total Qual. Manag. Bus. Excell. 29 , 959–976. https://doi.org/10.1080/14783363.2018.1486536 (2018) ( Publisher: Routledge ).
Gunasekaran, A., Subramanian, N. & Ngai, W. T. E. Quality management in the 21st century enterprises: Research pathway towards Industry 4.0. Int. J. Prod. Econ. 207 , 125–129. https://doi.org/10.1016/j.ijpe.2018.09.005 (2019).
Villalba-Diez, J., Ordieres-Meré, J., Chudzick, H. & López-Rojo, P. Nemawashi: Attaining value stream alignment within complex organizational networks. Proc. CIRP 37 , 134–139 (2015).
Khrennikov, A. Y. Ubiquitous Quantum Structure (Springer, 2014).
Mosseri, R. & Dandoloff, R. Geometry of entangled states, Bloch spheres and Hopf fibrations. J. Phys. A Math. Gen. 34 , 10243–10252. https://doi.org/10.1088/0305-4470/34/47/324 (2001) ( Publisher: IOP Publishing ).
Article ADS MathSciNet MATH Google Scholar
Kivelä, M. et al. Multilayer networks. J. Complex Netw. 2 , 203–271 (2014).
Article MathSciNet Google Scholar
Bossomaier, T., Barnett, L., Harré, M. & Lizier, J. T. An Introduction to Transfer Entropy Vol. 65 (Springer International Publishing, 2016).
Long, G.-L. & Sun, Y. Efficient scheme for initializing a quantum register with an arbitrary superposed state. Phys. Rev. A 64 , 014303. https://doi.org/10.1103/PhysRevA.64.014303 (2001).
Article ADS CAS Google Scholar
Maruyama, K. & Knight, P. L. Upper bounds for the number of quantum clones under decoherence. Phys. Rev. A 67 , 032303. https://doi.org/10.1103/PhysRevA.67.032303 (2003).
Qi, Q. & Tao, F. Digital twin and big data towards smart manufacturing and industry 4.0: 360 degree comparison. IEEE Access 6 , 3585–3593. https://doi.org/10.1109/ACCESS.2018.2793265 (2018).
Havlíček, V. et al. Supervised learning with quantum-enhanced feature spaces. Nature 567 , 209–212. https://doi.org/10.1038/s41586-019-0980-2 (2019).
Kimeldorf, G. & Wahba, G. Some results on Tchebycheffian spline functions. J. Math. Anal. Appl. 33 , 82–95. https://doi.org/10.1016/0022-247X(71)90184-3 (1971).
Gupta, S. & Zia, R. Quantum Neural Networks. arXiv:quant-ph/0201144 (2002).
Agmarcos. agmarcos/qcps: Qicps code. https://doi.org/10.5281/ZENODO.4837469 (2021).
Zhang, Y. & Ni, Q. Design of quantum neuron model for quantum neural networks. Quantum Eng. https://doi.org/10.1002/que2.75 (2021).
Wei, S., Chen, Y., Zhou, Z. & Long, G. A quantum convolutional neural network on NISQ devices. AAPPS Bull. https://doi.org/10.1007/s43673-021-00030-3 (2022).
Sundar, B., Walschaers, M., Parigi, V. & Carr, L. D. Response of quantum spin networks to attacks. J. Phys. Complex. 2 , 032008. https://doi.org/10.1088/2632-072X/abf5c2 (2020).
Zhang, C. et al. Experimental observation of quantum nonlocality in general networks with different topologies. Fundam. Res. 1 , 22–26. https://doi.org/10.1016/J.FMRE.2020.11.002 (2021).
Bogdan, P., Jonckheere, E. & Schirmer, S. Multi-fractal geometry of finite networks of spins: Nonequilibrium dynamics beyond thermalization and many-body-localization. Chaos Solitons Fractals 103 , 622–631. https://doi.org/10.1016/J.CHAOS.2017.07.008 (2017).
Download references
Acknowledgements
The authors want to thank the “Programa Estatal de I+D+i Orientada a los Retos de la Sociedad”, and through the Ministerio de Ciencia e Innovación Agencia Estatal de Investigación with the Grant RTI2018-094614-B-I00 (SMASHING). J.V.-D. would like to thank Professor Rosa Maria Benito Zafrilla and Professor Juan Carlos Losada González from Universidad Politécnica de Madrid for their valuable contributions and support.
Open Access funding enabled and organized by Projekt DEAL.
Author information
Authors and affiliations.
Hochschule Heilbronn, Fakultät Management und Vertrieb, Campus Schwäbisch Hall, 74523, Schwäbisch Hall, Germany
Javier Villalba-Diez
Complex Systems Group, Universidad Politécnica de Madrid, Av. Puerta de Hierro 2, 28040, Madrid, Spain
Department of Mechanical Engineering, Universidad de La Rioja, 26004, Logroño, Spain
Ana González-Marcos
Escuela Técnica Superior de Ingenieros Industriales (ETSII), Universidad Politécnica de Madrid, José Gutiérrez Abascal 2, 28006, Madrid, Spain
Joaquín Ordieres-Meré
You can also search for this author in PubMed Google Scholar
Contributions
J.V.-D., A.G.-M. and J.O.-M. conceived the experiment(s), J.V.-D., A.G.-M. and J.O.-M. conducted the experiment(s), J.V.-D., A.G.-M. and J.O.-M. analyzed the results. All authors reviewed the manuscript.
Corresponding author
Correspondence to Javier Villalba-Diez .
Ethics declarations
Competing interests.
The authors declare no competing interests.
Additional information
Publisher's note.
Springer Nature remains neutral with regard to jurisdictional claims in published maps and institutional affiliations.
Rights and permissions
Open Access This article is licensed under a Creative Commons Attribution 4.0 International License, which permits use, sharing, adaptation, distribution and reproduction in any medium or format, as long as you give appropriate credit to the original author(s) and the source, provide a link to the Creative Commons licence, and indicate if changes were made. The images or other third party material in this article are included in the article's Creative Commons licence, unless indicated otherwise in a credit line to the material. If material is not included in the article's Creative Commons licence and your intended use is not permitted by statutory regulation or exceeds the permitted use, you will need to obtain permission directly from the copyright holder. To view a copy of this licence, visit http://creativecommons.org/licenses/by/4.0/ .
Reprints and permissions
About this article
Cite this article.
Villalba-Diez, J., González-Marcos, A. & Ordieres-Meré, J. Quantum cyber-physical systems. Sci Rep 12 , 7964 (2022). https://doi.org/10.1038/s41598-022-11691-x
Download citation
Received : 07 June 2021
Accepted : 22 April 2022
Published : 13 May 2022
DOI : https://doi.org/10.1038/s41598-022-11691-x
Share this article
Anyone you share the following link with will be able to read this content:
Sorry, a shareable link is not currently available for this article.
Provided by the Springer Nature SharedIt content-sharing initiative
By submitting a comment you agree to abide by our Terms and Community Guidelines . If you find something abusive or that does not comply with our terms or guidelines please flag it as inappropriate.
Quick links
- Explore articles by subject
- Guide to authors
- Editorial policies
Sign up for the Nature Briefing newsletter — what matters in science, free to your inbox daily.

Human-in-the-loop Cyber-Physical Systems
Loading... Editorial 14 November 2022 Editorial: Human-in-the-loop cyber-physical systems Kok-Lim Alvin Yau 1,249 views 0 citations
Original Research 26 September 2022 Research on the dynamic viseme of the lip shape based on facial motion capture technology Shengyin Zhu 1,487 views 0 citations
Loading... Original Research 18 July 2022 DeepMatch: Toward Lightweight in Point Cloud Registration Lizhe Qi , 2 more and Yuquan Sun 4,743 views 3 citations
Loading... Original Research 06 July 2022 Spatial-Temporal Attention Mechanism and Graph Convolutional Networks for Destination Prediction Cong Li , 3 more and Fei Yang 6,419 views 6 citations
Original Research 01 July 2022 An Augmented Neural Network for Sentiment Analysis Using Grammar Baohua Zhang , 2 more and Jiahao Cai 3,706 views 0 citations
Loading... Original Research 23 June 2022 Application of Intelligent Inspection Robot in Coal Mine Industrial Heritage Landscape: Taking Wangshiwa Coal Mine as an Example Yan Shen , 1 more and Zengping Li 3,305 views 5 citations
Loading... Original Research 08 April 2022 Color Constancy via Multi-Scale Region-Weighed Network Guided by Semantics Fei Wang , 2 more and Guowang Gao 3,793 views 7 citations
Cyber-Physical Systems
The term Cyber-Physical System (CPS) is used to describe dynamic systems which combine components characterized by a physical state (e.g., the location, power level, and temperature of a mobile robot) with components (mostly digital devices empowered by software) characterized by an operational state or mode (e.g., on/off, transmitting/receiving). From a modeling point of view, physical states evolve according to time-driven dynamics commonly described through differential (or difference) equations, while operational states have event-driven dynamics where events may be controllable (e.g., a turn on command) or uncontrollable (e.g., a random failure). Imparting intelligence to a CPS implies the presence of multiple additional events that correspond to actions such as “start moving” for a mobile robot or “change sampling rate” for a sensor. These physical and operational states generally interact to give rise to a hybrid dynamic system. For example, a sensor with autonomous control capabilities may switch to a data transmitting mode as a result of a particular physical state change (e.g., its residual energy drops below a certain threshold). Examples of CPS include smart grid, autonomous vehicle systems, medical monitoring, industrial control systems, and robotics systems, and among others.
Collaborative Research: CPS: Medium: An Online Learning Framework for Socially Emerging Mixed Mobility
Emerging mobility systems, e.g., connected and automated vehicles and shared mobility, provide the most intriguing opportunity for enabling users to better monitor transportation network conditions and make better decisions for improving safety and transportation efficiency. However, different levels of vehicle automation in the transportation network can significantly alter transportation efficiency metrics (travel times, energy, environmental […]
CNS Core: Small: Collaborative Research: HEECMA: A Hybrid Elastic Edge-Cloud Application Management Architecture
Application software is becoming increasingly abundant in functionality and increasingly demanding of resources, e.g., memory and compute power. This project examines how application software, e.g., a Virtual Reality (VR) based drone control application, can be partitioned and deployed over different parts of a distributed computing infrastructure, i.e., resources are managed by a hybrid of service […]
Decentralized Optimal Control of Cooperating Networked Multi-agent Systems
Multi-agent systems encompass a broad spectrum of applications, ranging from connected autonomous vehicles and the emerging internet of cars, where the spatial domain may be hundreds of miles with time horizons over hours of days, to micro-air vehicles which operate over meter length and minute time scales, and down to nano-manipulation with nanometer spatial microsecond […]
CPS: Breakthrough: Collaborative Research: A Framework for Extensibility-Driven Design of Cyber-Physical Systems
A longstanding problem in the design of cyber-physical systems is the inability and ineffectiveness in coping with software and hardware evolutions over the lifetime of a design or across multiple versions in the same product family. The objective of this project is to develop a systematic framework for designing extensible cyber-physical systems that can enable […]
Workshop on Smart Cities, Arlington, Virginia, December 3-4, 2015
This Workshop will bring together researchers and technical leaders from academia, industry, and municipal government in order to set a short and long-term research agenda for Smart Cities aiming to integrate the three broad fields of Engineering, Computer Science, and Social Science. The Workshop topics cover a variety of fields and specialized disciplines including: transportation, […]
CPS: FRONTIER: COLLABORATIVE RESEARCH: BIOCPS FOR ENGINEERING LIVING CELLS
Recent developments in nanotechnology and synthetic biology have enabled a new direction in biological engineering: synthesis of collective behaviors and spatio-temporal patterns in multi-cellular bacterial and mammalian systems. This will have a dramatic impact in such areas as amorphous computing, nano-fabrication, and, in particular, tissue engineering, where patterns can be used to differentiate stem cells […]
PFI:BIC A Smart-city Cloud-based Open Platform and Ecosystem (SCOPE)
This NSF Partnerships for Innovation: Building Innovation Capacity (PFI:BIC) project from Boston University will research, prototype, and evaluate novel “smart-city” services for the city of Boston and for the Commonwealth of Massachusetts. The centerpiece of the project is a Smart-city Cloud-based Open Platform and Ecosystem (SCOPE), which creates a multisided marketplace for smart-city services based […]
Sensor Research Wins $1M NSF Award
Enhancing the functionality of cyber-physical systems—those that integrate physical processes with networked computing—could significantly improve our quality of life, from reducing car collisions to upgrading robotic surgeries to mounting more effective search and rescue missions. Recognizing Boston University as a key contributor to this effort, the National Science Foundation has awarded Professors Venkatesh Saligrama (ECE, SE) […]
CPS: Synergy: Collaborative Research: A Cyber-Physical Infrastructure for the “Smart City”
The project aims at making cities “smarter” by engineering processes such as traffic control, efficient parking services, and new urban activities such as recharging electric vehicles. To that end, the research will study the components needed to establish a Cyber-Physical Infrastructure for urban environments and address fundamental problems that involve data collection, resource allocation, real-time […]

An official website of the United States government
The .gov means it’s official. Federal government websites often end in .gov or .mil. Before sharing sensitive information, make sure you’re on a federal government site.
The site is secure. The https:// ensures that you are connecting to the official website and that any information you provide is encrypted and transmitted securely.
- Publications
- Account settings
Preview improvements coming to the PMC website in October 2024. Learn More or Try it out now .
- Advanced Search
- Journal List
- Sensors (Basel)
- PMC10490255

A Holistic Review of Cyber–Physical–Social Systems: New Directions and Opportunities
Associated data.
No new data were created or analyzed in this study. Data sharing is not applicable to this article.
A Cyber–Physical–Social System (CPSS) is an evolving subset of Cyber–Physical Systems (CPS), which involve the interlinking of the cyber, physical, and social domains within a system-of-systems mindset. CPSS is in a growing state, which combines secure digital technologies with physical systems (e.g., sensors and actuators) and incorporates social aspects (e.g., human interactions and behaviors, and societal norms) to facilitate automated and secure services to end-users and organisations. This paper reviews the field of CPSS, especially in the scope of complexity theory and cyber security to determine its impact on CPS and social media’s influence activities. The significance of CPSS lies in its potential to provide solutions to complex societal problems that are difficult to address through traditional approaches. With the integration of physical, social, and cyber components, CPSS can realize the full potential of IoT, big data analytics, and machine learning, leading to increased efficiency, improved sustainability and better decision making. CPSS presents exciting opportunities for innovation and advancement in multiple domains, improving the quality of life for people around the world. Research challenges to CPSS include the integration of hard and soft system components within all three domains, in addition to sociological metrics, data security, processing optimization and ethical implications. The findings of this paper note key research trends in the fields of CPSS, and recent novel contributions, followed by identified research gaps and future work.
1. Introduction
Cyber–Physical Systems (CPS) are increasingly becoming integration points for computation and are a significant part of people’s daily lives in their homes, businesses, governments, and industry. CPS were developed in order to address modeling challenges for control systems and embedded computing, focusing on efficiency optimization and secure components [ 1 ]. Not only have CPS come to represent industrial control systems, but a wide variety of systems at the interface of the digital and physical worlds, including medical devices, education devices, Internet of Things (IoT), smart cities, and transportation systems, among others [ 2 , 3 , 4 ]. A CPS integrates the computational and physical capabilities of systems, enabling those embedded systems to interact with sensors and actuators that exist in the physical world [ 4 , 5 ]. A CPS is characterized by its ability to interact with the physical world through a combination of control and computation, enabled through communication [ 5 ] (p. 1) and [ 1 ]. The term is broad, spanning several generations of technological growth and application development, and has evolved with technologies such as the Internet of Things (IoT).
CPS have traditionally been focused on systems that involve purely technical and physical components, with humans existing as external entities to the system, considering cyber aspects, including confidentiality, integrity and availability [ 6 ]. A CPS architecture is closely aligned with IoT, consisting of three layers: the perception layer, the network layer, and the application layer [ 4 , 6 , 7 ]. The perception layer is concerned with sensors and actuators that measure and collect data. The network layer is responsible for the communication of data between agents. Finally, the application layer is concerned with the processing of information and decision making. As the field of CPS grows and system boundaries extend beyond traditional Cyber–Physical components, there is the potential need for new architectural paradigms to be considered, especially including a security-by-design layer to be embedded in order to safeguard these layers efficiently.
With the prevalence of social media, a Cyber–Physical–Social System (CPSS) has emerged, concerned with identifying and understanding how humans integrate within systems that encompass the cyber, physical and social space [ 6 , 8 ]. Such systems extend beyond human-in-the-loop systems, where people interact with the system in switch-case-like predefined actions or decision support scenarios [ 9 ]. Instead, CPSSs include an understanding of the social ramifications and their feedback loops within the scope of the system boundary [ 6 ]. CPSSs are designed to offer seamless communication, coordination, and integration between the cyber, physical, and social elements, resulting in more effective system performance. This integration allows systems to adapt and respond to changing conditions, optimise resource allocation, and improve overall system performance and user experiences. A CPSS was defined as a Cyber–Physical System that exhibits the coordination, conjoining and integration of both human and social characteristics within a larger CPS [ 10 ] (p. 85). This further highlighted the complexity of CPSS, being exacerbated by the social system’s inclusion in the wider system boundary. This definition would be linked with three hypotheses, where the physical, mental, and artificial worlds combine, and highlighted the internet as a vector for this integration [ 10 , 11 ]. Pervasive Intelligent Spaces (PIS) would be also added in the definition to enable the interaction of all agents in real time and heavily rely on pervasive IoT technologies later expanded [ 10 ] (p. 86). This research highlights that social media serves as the new revolution for CPSSs, changing the way that societies and industries may function [ 10 ]. Each of the domains incorporated into CPSSs—social, physical, and cyber—individually meet the definition of complex systems. The large-scale and significant numbers of interactions between each of these domains create a strong system-of-systems dynamic [ 12 ]. Considering CPSS approaches therefore requires the use of a system-of-systems mindset, and will continue to do so in the future [ 13 ].
The field of CPSS attempts to bridge the gap between traditional CPS research and social complexity research. As CPS become more integrated into society within a system-of-systems mindset, social factors have the potential to effect the functioning of Cyber–Physical components in unpredictable ways. CPSS provides researchers with the opportunity to solve complex societal problems that are difficult to address using traditional methods. These include attempts to understand and model human behavior in online social media environments in addition to modeling systems that include real-time human feedback such as seen in smart cities and smart transportation [ 14 , 15 ].
Some of the emerging trends in CPSS include the development of smart cities, intelligent transportation systems, and smart healthcare systems [ 16 , 17 , 18 , 19 ]. The significance of CPSS lies in its potential to provide solutions to complex societal problems that are difficult to address through traditional approaches. CPSS can be used in numerous domains such as healthcare, transportation, energy conservation, disaster management, and environmental monitoring [ 17 , 20 , 21 , 22 ]. With the integration of physical, social, and cyber systems, CPSS can realize the full potential of IoT, big data analytics, and machine learning, leading to increased efficiency, improved sustainability and better decision making. Therefore, CPSS presents exciting opportunities for innovation and advancement in multiple domains, improving the quality of life for people around the world. In a CPSS, the cyber component involves the seamless integration of computational algorithms, communication networks, and software applications. The physical component includes physical infrastructure, devices, and sensors that collect and provide data to the system. The social component encompasses human interactions, preferences, and behaviors, which influence the system’s operation and outcomes
In a CPSS, sensors and actuators in the physical domain are often underpinned by IoT technologies. Security challenges posed by IoT-enabling technologies include signal integrity and accurate event actuation [ 23 ]. Thapa et al. addresses concerns related to these fundamental units in a complex CPS context, proposing security solutions for autonomous vehicle case studies to enable secure design [ 24 ]. However, the perception layer of a CPSS has the potential to exist conceptually beyond the physical domain, and within the cyber and/or social ones. For example, a human agent within a social network acts as a sensor to its environment, which may then inform feedback loops into the wider CPSS [ 25 ].
A CPSS represents a field that encompasses a complex system of systems. Social factors have the potential to create emergent behaviors that can induce instability into critical CPSS networks within the world. CPSS effects exist beyond the boundaries of traditional CPS. This can be exemplified with two case examples—Non-Fungible Tokens (NFTs) and the historical events of the Arab Spring. These case studies highlight the importance of CPSSs and the need to understand their interactions within a complex system-of-systems due to their potential wide-scale consequences across multiple domains.
The economic value of Non-Fungible Tokens (NFTs) is derived through social factors. They exist within the cyber domain, but have real physical-world consequences including economic and market impacts. The value of NFTs is created based on social perception of the cost/benefit trade-off of that token, and is heavily influenced by aesthetic human psychological values [ 26 ]. Of course, NFTs and similar digital ledger technologies exist within the cyber domain, but they also have physical-world impacts through economic returns and potential changes to the labor market [ 27 , 28 , 29 ]. Thus, the existence and financial impacts of NFTs demonstrate the consequences of CPSSs on the large scale system-of-systems that effects large-scale world dynamics.
The Arab Spring [ 30 ] also highlighted how CPSSs can induce emergent behaviors that have multi-domain impacts. Protests that initially began in Tunisia spread to other countries including Libya, Egypt, Yemen, Syria, and Bahrain. This information spread was fueled through social media platforms including Facebook and Twitter, enabling real-time dissemination of information across national boundaries and organization and mobilization of protests based on shared ideologies. During the Arab Spring, features of complexity were exemplified through sensitivity to initial conditions with the initial protest suicide of Tunisian Mohamed Bouazizi, the self-organization of political groups via the internet, adaptive interaction of protests as events evolved, and emergent behaviors including the coup of the Egyptian President Mohamed Morsi [ 31 , 32 , 33 , 34 , 35 , 36 ]. The three CPSS domains were also clearly present throughout the Arab Spring. The cyber component was a key enabler to the dissemination of information between protesters via social media. The social component manifested through the sharing of ideologies and political engagement by both citizens and governments. Finally, the physical domain manifested through physical protests, the Egyptian coup, the Tremeseh massacre, the Syrian civil war, and diverse government reactions and reform.
A CPSS poses several challenges from a scientific perspective. One of the main challenges is the integration of various components of the CPS within the CPSS context. These components include sensors, network components, and actuators [ 37 ]. The nature of CPSS components further requires that resource synchronization and optimization occur for performance—a complex task [ 38 , 39 ]. The development of secure and reliable communication protocols that can safely transmit data between different components is an issue [ 4 , 40 ]. The new trends in CPSSs are characterized by the use of advanced technologies and methods such as artificial intelligence, machine learning, blockchain, and IoT [ 41 , 42 , 43 ]. These technologies allow CPSSs to interact with their environment more intelligently, accurately, and efficiently. As a result, CPSSs can improve the performance of physical and social systems, enhance decision-making capabilities, and unlock new applications.
CPSSs face a variety of cyber security challenges. Some challenges are common with those underlying the IoT, such as node capture, routing attacks and data theft [ 44 ]. Because CPSSs include the social landscape, this provides greater opportunity for social engineering attacks that exploit human vulnerabilities, using tactics such as deception, manipulation, or influence [ 45 , 46 , 47 ]. CPSSs present unique cyber security challenges, where threats may arise through any of the cyber, physical or social agents within the system. Subsequently, conscious development of CPSSs within a secure digital technology mindset is essential to assuring system operation in the face of cyber threats [ 48 ]. Ensuring the security and privacy of CPSSs is crucial because of the high volume of sensitive data being processed and shared [ 49 ]. Protecting against cyber attacks, asserting data integrity, and maintaining user privacy are significant challenges. CPSSs include various interconnected components, such as sensors, devices, and networks [ 50 ]. Ensuring seamless interoperability between these components is a challenge because of differences in protocols, standards, and technologies. CPSSs would also need to handle large amounts of data, users, and interconnected systems, ensuring scalability to accommodate such growth while maintaining system performance and efficiency, which is a problem. A CPSS should be resilient to failures, disruptions, and adverse events [ 51 ]. Ensuring robustness and fault tolerance to maintain system functionality and minimize potential damages is a significant challenge [ 52 ]. CPSSs raise ethical concerns, such as the potential amplification of social biases, discriminatory practices, and the misuse of personal data. Addressing these challenges and promoting responsible and inclusive CPSS design and usage is essential, which we attempt to highlight in this study.
The ongoing advancements in CPSSs are expected to have a significant impact on various industries and aspects of daily lives [ 52 ]. As a consequence, understanding the scope of current CPSS research within these topics is essential for the development of future work and innovation in the research field. This is enhanced by CPSS’s potential for impact in diverse and interconnected domains, ranging from politics to economics, infrastructure and algorithm design [ 18 , 51 , 53 ]. This paper aims to holistically review CPSSs from three angles—cyber, physical and social—in order to determine their impact on system performance and how they offer secure and automated services to end-users and organisations in the era of artificial intelligence (AI). We define a CPSS as a system that integrates cyber, physical, and social elements to interact and collaborate with each other.
The key contributions of this work are structured as follows:
- We determine a CPS and its interaction with social media and its components, and to what level it influences human behaviors.
- We examine the distribution and intersection of research associated with CPSS, complex systems, social media, influence and cyber security applications.
- We discuss the recent advancements of CPSSs and how they enhance human activities and system performances.
- We describe the recent challenges and lessons learned and future research directions of CPSSs.
This paper discusses Cyber–Physical–Social Systems within the larger context of Cyber–Physical Systems and the impact of social complexity on these systems in Section 2 . In Section 3 , we discuss the related studies mixed between CPS, social media, and CPSS. The recent technologies with CPSS are explained in Section 4 . This is followed by examining the most influential terms in Section 5 . Section 6 describes the role of CPSS with recent smart applications. Research challenges and lessons learned are explained in Section 7 . Finally, we conclude the paper in Section 8 .
2. Cyber Physical Social System (CPSS)
We define a CPSS in a similar way to Wang [ 10 ], but in addition, we include security-by-design to each component as an extension of a Cyber–Physical-System that includes human social systems within its holistic system boundary. A CPSS, therefore, includes social and cognitive functions not considered within traditional CPS [ 54 ]. We define a CPSS as a systematic architecture that connects social features with human and network elements, with social attributes disseminating in accordance with node relationships, considering a secure design to the elements and fractures. In this way, the social space exists beyond just people, but also within their interactions, networks, and interactions.
We do not consider any of the competing definitions of CPSS that do not model social systems within their system boundary, and instead note that these systems interface with the CPS, as seen in the work of Zhou and Lin [ 55 ]. Competing definitions include considering CPSS as battery-supplied low-energy devices [ 55 ] or systems that highly conjoin communication, sensing, computing, and C2 (Command and Control) within larger human societal contexts [ 56 ]. The application of CPSSs spans various domains, including smart cities, transportation systems, healthcare, manufacturing, and entertainment. By combining cyber, physical, and social elements, CPSSs can enable advancements such as autonomous vehicles, smart grid systems, personalized healthcare, and immersive virtual environments. Overall, CPSSs represent an interdependent and interconnected system that leverages digital technologies, physical infrastructure, and social dynamics to create intelligent and adaptive systems capable of addressing complex societal challenges.
A further distinction should be made between the concept of CPSS and Cyber–Physical–Human Systems (CPHS). CPHS include humans in the loop and focus on the interaction between people and Cyber–Physical Systems [ 9 ]. CPHS play a crucial role in various scientific fields, including robotics, artificial intelligence, and human–computer interaction. They enable the development of advanced technologies such as autonomous vehicles, wearable devices, smart homes, and interactive virtual reality platforms. By leveraging CPHS, researchers aim to enhance human productivity, safety, and well-being in various domains such as healthcare, transportation, manufacturing, and entertainment. For example, in healthcare, CPHS can be used to monitor patients’ vital signs remotely, enabling early detection of health issues and prompt intervention. In transportation, CPHS can facilitate the development of self-driving cars that optimize traffic flow and improve road safety. However, CPHS rely on people who feature cognition, predictability and motivation without considering complex social factors [ 9 ]. CPHS are suited for applications where the human element of the CPS behaves in a defined way using defined options, and has been used heavily in modelling human-in-the-loop systems such as those at the National Aeronautics and Space Administration [ 9 ].
2.1. Cyber–Physical System
The ontology of CPSSs is difficult to define for two reasons. The first is that the boundaries between these agents or sub-systems are unclear. The second reason is that a CPSS includes human social systems within its holistic system boundary, as shown in Figure 1 . Subsequently, modelling and measuring people’s social feelings and attitudes is more difficult than placing sensors in traditional Cyber–Physical Systems, because these sensors may need to exist at the interface or within the human mind itself [ 57 ]. The collection of data from social sensors is therefore a challenge within this field, as accurate metrics regarding social or emotional human states pose obstacles in terms of collection accuracy. In a traditional CPS, sensors can be implemented technically through technology paradigms such as IoT.

Evolution of the CPSS.
The connection between CPS IoT lies in their shared objective of connecting and exchanging data between physical devices. CPS involves the integration of sensors, actuators, and control systems, while IoT extends this concept by connecting a vast array of everyday objects or “things” through the internet. IoT acts as the backbone for CPS, providing the network infrastructure and communication protocols necessary to connect and exchange data between CPS components. This allows remote monitoring and control of physical processes in real time, enabling more efficient and autonomous decision making.
Converting human interactions and and behaviors in the social space into calculations inputted to sensors is a challenge within CPSS. Intrinsic social calculations that occur within the human mind are difficult to effectively record and calculate, and thus developing mechanisms to appropriately measure these social metrics is a field of continued CPSS research [ 57 ].
The combination of CPS with IoT leads to numerous applications across various domains, such as smart cities, transportation systems, healthcare, manufacturing, and energy management. By leveraging the capabilities of CPS and IoT, organizations can achieve improved efficiency, increased productivity, enhanced safety, and better resource management. The introduction of humans and their associated social contexts presents a unique challenge for CPSS research, as sensors to monitor these conditions need to quantify social data points [ 57 ]. An example of a CPS in this context would be a modern manufacturing facility, which would have computational sensors, actuators, and humans that would be making predefined or limited decisions based on the system state. By contrast, an example of a CPSS would be a humanitarian assistance or disaster relief effort, which would be considering multiple sensors and unstructured reports from groups of people. This latter example has a greater role for people, social systems and their interactions. This is an evolving area, as the forms of human interaction are increasing, especially as new communication platforms appear. There has been some development into ontologies for CPSS modelling, although much of this is sourced from a few limited authors [ 8 , 58 ].
2.2. Social Media Complexity
Social media exemplifies the construct of a Cyber–Physical–Social System. Social systems, regardless of technological integration, are regarded as complex systems [ 31 ] (p. 29). Complex systems are entities that consist of “many interacting parts”, and exist on the “edge of chaos” [ 59 ] (p. 1) and [ 60 ]. Social media is inherently reliant on layers of complex systems, often driven by people, and is fed by these principles [ 61 , 62 ]. Terms such as “the Internet of Minds” and “Humans as Sensors” have also contributed to understanding social interaction with CPS through an IoT perspective [ 63 , 64 ]. Furthermore, Maier et al. [ 65 ] discussed the role of humans as “neurons in a hive mind” as part of Society 5.0, enabled by the token economy. Subsequently, there is a strong link between the concept of social media and its intersection with the field of CPSS.
Complex systems are characterized by several behaviors and features. These aid in distinguishing complex systems from both chaos and complicated systems. Complicated systems contain a multitude of parts that are defined by ordered and simple rules [ 66 ]. Complex systems exist at the intersection between chaos and complicated systems, with sub-units potentially including both chaotic and complicated dynamics. Complex adaptive systems are a more specialized case of complex systems, although sometimes the terms are used interchangeably. Complex adaptive systems dynamically evolve with and exhibit adaptation within changing environments, meaning that the system will not always react consistently to the same stimuli [ 67 ] (p. 2). They are distinct from the features of systems of equilibrium or homeostasis. Complex adaptive systems contain agents within their system boundaries, which are semi-autonomous units that seek to optimize based on a schema of prescriptive actions and rules [ 68 ] (p. 18) and [ 69 ] (p. 85). The behavior of these agents can subsequently display patterns at the system level, not all of which are predictable or repeatable. The degree of complexity experienced by a system can be measured by its difficulty in description, its difficulty in creation, and its degree of organization [ 70 ]. Many complex adaptive systems change over time, with changing optimized organization depending on the current context or environment. Many human and social interactions form parts of larger complex adaptive systems, with wider environment changes influencing both individual and system behavior [ 71 ].
The features of complex systems identified in the literature are varied; however, there are some key commonalities between interpretations [ 31 , 32 , 33 , 34 ]. The boundaries between these systems and their sub-units are sometimes blurred [ 34 ]. Complex systems often contain the features of self-organization; interdependence; hierarchy and scale; evolution, feedback and adaptive interaction; unpredictability and non-linearity; sensitivity to initial conditions; and emergence [ 31 , 32 , 33 , 34 ]. Social media is clearly established in the literature as a complex system, which creates additional challenges for the conceptualization and modelling of the system within the scope of CPSS [ 72 ] (p. 374). Social media case studies have exemplified how its emergence has had far-reaching consequences on political, economic, and social outcomes.
Conceptual frameworks and analysis of historic social and economic events fueled by complex social media interactions have been studied, including such diverse events as the Arab Spring, Euromaidan, and Gamestop [ 73 , 74 , 75 , 76 , 77 ]. Furthermore, the evolution of news consumption through social media has increased opportunities for the generation of ‘Fake News’. This has been a contributing factor to real-world phenomena including politically influenced ideological polarization within the social population [ 78 , 79 , 80 ]. CPS theory is an opportunity to examine emergence within social media, and therefore model the complexity in CPSS. There are further epistemological questions regarding complex CPSS, with challenges in identifying and understanding the inputs, processes, and outputs that social agents have within these systems. Subsequently, modelling and understanding social media as a form of complex CPSS is a field warranting further investigation. This study attempts to understand the distribution of research relating to this topic and determine where research gaps and opportunities exist to further its conceptual development.
3. Current State of the Art
This study was conducted via a mixed methods approach, with both quantitative and qualitative data analysis. It involved surveying search results from the Scopus database related to the research topic, statistical analysis of those results, exporting result metadata to file for topic cluster analysis in VOSViewer, and finally a conceptual analysis of key research papers identified. VOSViewer and similar visualization tools have been widely used in the preliminary analysis and identification research opportunities in several academic domains [ 81 ], including specific IoT environments [ 82 ] and computational physical chemistry [ 83 ]. There are also several other tools designed to perform similar meta-analysis of academic media via citations, keywords and themes. These include CitNetExplorer [ 84 ] and SciMAT [ 85 ]. These tools, first developed a decade ago, are seeing increased and significant use in publication analysis, in particular for environmental scans and surveys. Visualization has become an important tool across understanding research domains as varied as deep learning in autonomous vehicles [ 86 ], understanding how COVID-19 has shaped research [ 87 ], and the links with Augmented Reality in learning [ 88 ]. This work has chosen VOSViewer for use, but does not preclude the use of other tools for future comparative analysis. Through the methods discussed, the aforementioned research questions were analyzed and able to be answered.
3.1. Distribution of Current Research
Data collection occurred by searching for key terms within the Scopus database, and analysis of the results’ bibliographic data. The search phrases utilized are detailed in Table 1 . The search query was applied to the article title, abstract and keywords. These terms were used because they related to answering the aforementioned research questions. They focus on understanding the distribution of research across these fields, whilst also highlighting the degree of intersection into the chosen research area.
Scopus search phrases.
Report Term | Scopus Search Phrase |
---|---|
Complex system | “*Complex system*” OR “*Complexity*” |
Cyber–Physical System/CPS | “*Cyber Physical System*” OR “*Cyber–Physical System*” |
Cyber–Physical–Social System/CPSS | “*Cyber Physical Social System*” OR “*Cyber–Physical Social System*” |
Social Media | “*Social media*” |
Influence | “*Influence*” |
Cyber Security | “*Cybersecurity*” OR “*Cyber security*” |
3.2. Statistical Visualization of Current Research
From the Scopus data sets the degree of research within the chosen fields is quantified and visualized. Figure 2 illustrates the comparative size of each search result. The field of complex systems is the largest and most comprehensive. This implies how this field is endemic and underlies many other research areas. As a subset of CPS, CPSS predictably returned a smaller results size. In its early stage, CPSS is mainly focused on exploring potential applications across various domains, including smart cities, healthcare, transportation, and manufacturing. The CPSS trend focuses on studying how to merge traditional physical systems with cyber intelligence, communication capabilities, and social behavior analysis.

Single search term results.
The intersection between CPS and CPSS involves exploring how technology and human behavior interact and influence each other, considering the security by design of their elements. For example, in a smart city scenario, CPS would include sensor networks and data processing systems to monitor and control infrastructure, while CPSS considers how people will interact with these systems, such as through social media platforms or mobile apps that provide information about the city’s status and encourage citizen engagement. Understanding the intersection between CPS and CPSS is important for designing more efficient and user-centric systems, as well as addressing ethical, legal, and societal implications. It involves studying complex socio-technical systems to ensure that technological advancements are aligned with human needs, values, and social structures.
CPS are systems that combine physical components with computational elements to enable data sensing, processing, and control, with applications ranging from smart homes and energy grids to autonomous vehicles and industrial automation. CPSS, on the other hand, extends CPS by considering not only the technical aspects but also the social interactions, human behavior and cyber security within these systems. Figure 3 presents the intersection of the different fields of research. The most common fields of intersection were complex systems and CPS , followed by complex systems and social media . Conversely, intersections between CPSS and other topics were among the lowest statistics. These statistics align with the general trends identified in Figure 2 , as topics that had a larger quantity of papers were more likely to have a larger intersection of multiple paper topics in Figure 3 . With CPSS being a subset of CPS, the results aligned with this assumption that the subset topic’s intersection with other fields of research would be less than the overarching parents’ topic intersection.

Dual term search results.
Table 2 shows the distribution of papers with an intersection of multiple search topics. Of note, CPSS intersection with multiple other research fields yielded no results in any combination. This indicates that there is potentially a research gap in these areas, which is worth exploring further within the literature.
Multiple term search results.
CPSS | CPS | ||
---|---|---|---|
Three Terms | Complex system + Social Media | 0 | 3 |
Three Terms | Complex system + Cyber Security | 0 | 182 |
Four Terms | Complex system + Social Media + Cyber Security | 0 | 0 |
3.3. Network Analysis of Current Research
The bibliographic data from the Scopus database results were then assessed utilizing network analysis maps in the tool VOSViewer. They were analyzed utilizing the co-occurrence method using keywords of the papers. The counting method specified was ‘full counting’ and no thesaurus file was used. Phrases were prioritized for analysis by lowering the occurrence threshold until the number of occurrences was simultaneously minimized, whilst remaining above 30. For inputs that could not meet the threshold of 30, all terms were used. This meant that most maps had approximately 30 terms. The Scopus search terms for each result set were discounted from the final data set, and any outlying data that did not connect to the main graph was also discounted.
3.4. Conceptual Analysis of Most Influential Research
The conceptual analysis involved a review of the key topics utilizing the Scopus search outputs for the CPSS search term. The intent of this analysis was to determine novel research within the field and highlight the most influential papers. Selection criteria were applied to the results to narrow the focus of the review. The following terms and criteria were excluded from the analysis: survey papers, conference proceedings/journal introductions/opening chapters, references that did not contain abstracts, papers that did not include CPSS in their abstract, references whose full text could not be found, and papers that were not written in English. After this, the top 30 highly cited papers were selected for the development of a CPSS influence concept map.
This process was then repeated with the original CPSS Scopus search results data set. In this iteration, research papers were limited to the years 2021–2023 to assist in identifying the most novel and highly influential recent papers. References were chosen that had 5 or more citations for review, with the final reference count for analysis being 25.
3.5. Analysis of Current Research
The distribution of the research topics was significantly varied. Fields such as complex systems returned over a million results, whereas newer terms such as CPSS returned less than one thousand. CPSS was also highlighted as a significantly small subset against the wider field of CPS, with approximately 500 papers compared to 25,000 in the search results. These results indicated that the field of CPSS is relatively small compared to other established related research areas, and may warrant further expansion and investigation.
When considering the intersection of multiple search terms, as highlighted in Table 2 , there were limited multi-disciplinary papers that addressed the research topics defined in Table 1 . In particular, the queries that searched for the intersection of CPSS with multiple other research topics all produced zero search results, indicating that there is a significant research gap at the overlap of these key areas. As an emerging research area, this indicates that applications and research into CPSS require increased focus and commitment in order to understand the interrelation of complex CPS in the case of social media implications for cyber security.
4. Recent Technologies with CPSS
Recent technologies, including big data, IoT, machine learning and social media platforms have been employed in conjunction with CPSS. These technologies with CPSS, have revolutionised various fields by allowing advanced data analysis and decision-making processes. Big data allow massive volumes of structured and unstructured data that cannot be effectively processed by traditional methods. With recent technological advancements, it is now possible to collect, store, and analyze these vast amounts of data. Machine learning (ML), a subset of artificial intelligence, is a methodology that allows computer systems to automatically learn and improve from experience without being explicitly programmed. By utilizing ML algorithms, they can make predictions, classify data, and discover patterns within big data sets. Social media plays a significant role in the collection and generation of big data. Platforms like Facebook, Twitter, and Instagram provide vast amounts of data generated by users worldwide. These data can be utilized to study human behavior, sentiment analysis, and societal trends.
The integration of these technologies allows monitoring and control of various systems, ranging from smart cities, transportation networks, and healthcare systems, to environmental monitoring. Figure 4 , which is the Complex system and CPS intersection map, exemplifies this trend. Every cluster map generated throughout the analysis, with the exception of the CPS and CPSS intersection map and the CPSS and social media intersection map, includes at least one of the aforementioned topics. This indicates a significant research focus across the literature on these key topics.

Complex system + Cyber–Physical System clusters (2019–2020).
When drilling down into the research topic of CPSS, the largest areas of research focused on big data and the IoT; see Figure 5 . In this model, the oldest research areas included IoT, intelligent systems, and social manufacturing. The newest areas included computational modelling, the Metaverse, and computational experiments.

CPSS research clusters (2018–2021).
When considering the intersection of topics, research that focused on both CPSS and complex systems was clustered across a linear scale. The oldest research topics were located in the center, with newer research extending to either side, as shown in Figure 6 . The oldest research was associated with knowledge automation and parallel control, with newer research associated with computational modeling, smart manufacturing, task analysis and the Metaverse.

CPSS + complex systems time overlay (2019–2022).
5. Most Influential Research Focuses
Within the field of Cyber–Physical–Social Systems, there are several areas of research focus apparent within the literature. Figure 7 highlights the top thirty influential CPSS papers, based on citations, and their topic clusters. Modelling CPSS through frameworks, simulations and data models is a key research area within the most influential CPSS papers. The complexity considerations of CPSS are identified and discussed throughout the literature. There was also a significant research focus on data processing implementations and optimization in areas such as resource allocation and capacity management.

Most influential research clusters in the field of CPSS.
The most influential case studies within the field related to manufacturing and industrial applications, smart cities, social media, and the smart grid. The most influential enabling technologies identified included tensor, edge computing, the IoT, and artificial societies, computational experiments, and parallel execution (ACP).
6. Role of CPSS with Recent Smart Applications
The concept of CPSS was noted in the literature to be linked regularly to several smart applications such as smart cities, smart healthcare, blockchain technologies, etc. The most regularly referenced included industrial applications and smart city implementations. These included manufacturing and Industry 4.0, smart cities, and blockchain tokenization [ 89 , 90 , 91 , 92 ]. Smart city implementations are also referred to in the highest CPSS paper citations [ 15 , 18 , 92 , 93 ]. Other CPSS implementations explored within the recent novel literature include smart wearable devices, data center cooling systems, industrial chart data processing, and electricity savings [ 22 , 94 , 95 , 96 ]. The gaming industry is additionally identified as a CPSS, with research in this space aligning with opportunities to crowd-source task allocation and optimization problems relying on the interaction of people with digital systems [ 97 ]. Future aims of this work include crowd-sensing via real user testing in order to generate incentive strategy evaluations [ 97 ].
Industrial implementations are identified in the literature as novel case studies for CPSS within the wider context of Industry 4.0. Performance monitoring is one area of development, where a CPSS framework is complimented to enable greater fidelity to detection and monitoring processes [ 90 ]. Evolving out of Industry 4.0, Industry 5.0 is an additional paradigm through which the concept of CPSS is discussed, integrating with other enabling technologies such as 6G, tokenization, robotics, tactile internet and collective intelligence [ 65 , 91 ]. These novel ideas also further link to the concept of Society 5.0, where CPSS’s aim becomes to enhance wider system harmony [ 65 , 91 ]. However, Maier’s work is challenged by the semantics of CPSS, sometimes identifying human-in-the-loop centric systems, which in some scenarios may align better with categorization as Cyber–Physical–Human Systems (CPHS) [ 91 ]. This cross-association indicates there is potentially a need for further work in defining the ontologies that separate these two sub-types of CPS, and the factors that conclusively assign a system to one definition over another.
A portion of the research related to industrial applications of CPSS appears to be more closely aligned with a traditional CPS or Industrial CPS (ICPS), instead of the social dimensions and metrics traditionally included within the CPSS construct. In some papers, this manifested in a Cyber–Physical–Human System (CPHS) context, where people’s interactions with the industrial system were considered, but frameworks did not include metrics or sensors relating to complex human social systems, and instead focused on predictable defined human-in-the-loop switch case interactions [ 9 ].
6.1. Data Processing and Resource Allocation
Innovations and novel implementations of CPSS in the recent literature often focused on improving resource allocation or optimizing data mining within large data sets as enabled by sensors and IoT. CPSS is identified as having significant data processing and analysis considerations due to its heterogeneity, growth, and dimensionality [ 98 ].
Collaboration is one research area that aims to improve CPSS data processing in order to support multi-goal optimization solutions [ 98 ]. Furthermore, Wang et al. introduced and tests the performance of algorithms in their role of processing CPSS big data in smart cities, including singular value decomposition (HOSVD), ring-based tree algorithms and tree-based tree algorithms [ 92 ]. This research identifies the need for future computational efficiency studies to improve the algorithmic processing of CPSS data in wide-scale applications [ 92 ].
Machine learning is a further tool used to build solutions to big data for CPSS processing challenges, with algorithms leveraging computing constructs such as cloud computing, edge computing, and distributed training in order to enable systematic processing [ 92 ]. Linear Regression is another machine learning technique that is applied to data analysis for CPSS, with application implications to the cyber security of data processed in untrustworthy environments, such as cloud servers or the blockchain [ 99 ]. Whilst privacy and security concerns were identified as a focus point for experimentation in CPSS data processing, the authors noted that future work in this domain would need to continue to improve data privacy, verifiability of results, and fairness [ 99 ] (p. 3966). Parallel execution is used as one way to validate AI-processed CPSS data, with future work in this space highlighting the need for real-world accurate simulation systems, and validation of outputs against read scene data [ 93 ]. Notably, the recent novel literature regarding CPSS data algorithms does not address newly emerging fields such as federated learning, where collaborative machine learning occurs across multiple decentralized clients utilizing architecture such as IoT [ 100 , 101 ]. The discussions regarding applications of such algorithms are limited, and present a further opportunity for research growth and maturity [ 96 ].
The processing of data to feed recommendation services as part of the CPSS construct is also considered in the literature, with algorithms built to process and analyze user characteristic data in diverse scenarios [ 51 ]. Future work in this area focuses on testing existing solutions with larger and more complex data sets to ensure quality of service in wide-scale applications [ 51 ] (p. 3858). Diverse scenario processing in the context of CPSS is an emerging space. Through the conceptual analysis, there were limited novel studies identified that challenge legacy machine learning algorithms with alternatives such as federated learning.
Data for CPSS processing must also be considered with a system-of-systems mindset, where multiple subsystems with varying data sets must be interconnected and processed. Within the smart city case study, this problem set is exemplified, with sub-systems including power grids, human social systems, and SCADA systems [ 102 ]. Hazard identification for safety is one research goal of these studies, where risk analytics are derived from interdependent network theory. However, future work is required in the fields of system resiliency in the case of cyber attacks in order to provide accurate hazard assessments [ 102 ] (p. 15).
Tensors are identified as one avenue for enabling heterogeneous data learning and optimization for CPSS [ 103 , 104 , 105 ]. Considerations for how to optimize the modelling of CPSS that utilize Intelligent Edge Services (IES) as part of an edge computing construct experience barriers in the form of security-efficiency compromises, energy consumption effects, and cost [ 104 ]. Future work in this optimization space needs to consider the secure optimization of multiple objectives, due to the complexity of CPSS, in order for CPSS-related price execution to become more economically viable [ 104 ] (p. 44). Additionally, the application of leading-edge machine learning technologies, such as federated learning, is a further opportunity for CPSS data processing research, especially in consideration of the joint foundational applicability of IoT to the two fields [ 101 , 106 ].
Algorithms that leverage sensor technology are identified in the literature to demonstrate more accurate classification results compared to legacy models; however, they highlight challenges to the flexibility of such models when training data constraints are introduced [ 105 ]. This is of particular concern when considered within the CPSS construct, where emergence may introduce unpredictable results to algorithm outputs, with the potential introduction of new data sets that do not nearly align with trained parameters.
The social component of human-generated information is also a data processing challenge, with sensors such as social media posts requiring analysis and comprehension in the digital space. The use of models such as Natural Language Processing needs to evolve to meet this need, as data sets may exhibit emergent or unpredictable behavior [ 63 ]. Opportunities within this sphere are posed through solutions such as Zero-Shot algorithms, that can potentially analyze data from unseen classes; however, continued development into the processing of unlabeled data is required to improve performance, especially in relation to semantics and knowledge graphs [ 63 ].
6.2. Cyber Security Implications
Cyber security and privacy concerns relating to data in IoT-enabled CPSS are of note within the literature and are explored through multiple case studies and implementations. Integration of social and IoT technologies into CPSS presents an opportunity to develop frameworks that conceptualize Enterprise Digital Transformation, such as seen in Mendhurwar and Mishra’s framework [ 107 ]. Their model specifically identifies not only the inter-dependencies between social agents and IoT sensors as part of CPSS but highlights the cyber security challenges associated with this digital transformation process [ 107 ]. They further identify that future work in this space may consider case studies that can provide insight into the nuances of specific applications and deliverables within the industry, considering factors such as entity needs and security posture [ 107 ].
Data analysis in industrial applications is also at the leading edge of research regarding CPSS, especially in conjunction with privacy considerations associated with data mining [ 89 ]. Algorithms such as the high-order Bi-Lanczos (HOBI-Lanczos) approach demonstrate an ability to securitize data and protect user privacy. Future work in this space includes exploration of energy implementations and collusion approach considerations [ 89 ]. Gati et al. [ 41 ] explored this concern regarding privacy preservation within CPSS, with their solution leveraging deep learning techniques in order to process private data. They propose a differential privacy framework that is applicable across multiple use cases such as smart agriculture, smart healthcare, and smart transportation [ 41 ]. Privacy protection and secure data considerations are also highlighted within CPSS research [ 96 , 103 ]. Human Activity Recognition (HAR) utilizing machine learning is one avenue for enabling secure processing of data framed through wearable devices [ 96 ]. Future research in this field involves investing in greater efficiency of algorithms, in addition to more personalized modelling whilst maintaining user privacy [ 96 ]. Additional challenges in this space involve the privacy preservation of data and processing, in relation to centralized models, and also the collection and integration of nonindependent and identically distributed (non-IID) data.
CPSS is also identified in the literature as an opportunity for attack vectors, which have the potential to compromise the cyber security of systems and their users. In these contexts, variations of existing attack vectors such as social engineering and malicious code execution are applied within the CPSS context, rather than a new attack class being defined. For example, Yang et al. propose the use of a neural network, called MCNN, to identify digitally manipulated ‘fake’ images in order to reduce the attack surface for anti-forensics tools [ 108 ]. Research such as this highlights the potential for CPSS to have real-world impacts; however, current works tend to focus on specific case studies rather than wider cross-domain conceptual applications. As people and technology integrate further in the Cyber–Physical–Social world, this poses additional opportunities for cyber attacks, as each edge between nodes in the CPSS presents additional potential attack vectors.
7. Research Challenges and Lessons Learned
As discussed above, the majority of novel contributions to the literature surrounding CPSS over the last three years can be categorized into one of three research fields. These areas of concern are broadly considered as case study CPSS implementations, such as smart cities; data processing considerations, such as efficiency algorithms; and cyber security implications, such as user data privacy concerns.
Through this critical survey and analysis of the literature, several significant research gaps and opportunities were identified and synthesized. Research gaps that specifically addressed the research questions included a lack of social metric inclusion in system-of-systems modelling for CPSS, a need for a CPSS ontology that includes consideration of the other intersecting research fields, and the inclusion of complex system dynamics within frameworks, including an understanding of emergent effects within the cyber, physical and social domains of CPSS. Additionally, CPSS research gaps were identified that related to comprehensive holistic case study analysis and cross-silo analysis of enabling emergent technologies including smart contracts and the blockchain, federated learning, sensor design and distribution. Furthermore, the existing research rarely addresses the impact of opaqueness within complex CPSS and how that may affect the accuracy and reliability of machine learning algorithms and decision support systems.
There is a lack of highly cited novel papers that specifically focus on developing frameworks or ontologies related to the social component of CPSS. Many papers that reference CPSS in their keywords or abstract often use this term to exemplify how a system can be considered a CPSS, without necessarily focusing on the nature of CPSS as part of their research. Multiple CPSS papers in the literature name the system in question as a CPSS, but then focus research on multidisciplinary fields such as algorithms for resource allocation or case studies such as smart grids [ 95 ]. Models and frameworks for CPSS as identified in the most recent novel literature also highlighted implementations focused on either parallel execution, or only considered the Cyber–Physical components of the system instead of including the human social metrics. Social data within the context of CPSS are focused upon little within the literature, with the outputs usually measured through methods such as social media posts or interactions with CPS system boundaries, such as interfacing with a smart city application.
There is, therefore, a research opportunity to aid in defining how these social data can be measured, and the subsequent challenges of processing such heterogeneous unlabeled data at scale. There is subsequently a need to identify how human metrics can be effectively modelled within larger CPSS frameworks, which include their social behaviors, attributes, and subsystems. Furthermore, there was evidence in the literature that CPSS was defined in broad and sometimes contradicting ways between different authors, with the comparison of CPHS to CPSS as an example of this. Analysis of the literature further highlighted some related research terms that could contribute to understanding the nature of CPSS. Terms such as persuasive computing, Society 5.0, Internet of Minds, and cybernetics also appeared to constitute part of CPSS as a concept, thus warranting further investigation and linking towards this larger CPSS ontology.
Whilst complexity is defined as an essential component of CPSS as per its originating conception, features of complexity such as emergence are rarely considered within the literature [ 10 ]. When machine learning is considered part of a CPSS application, the feature of emergence is conceptually at odds with the traditional design of training data, which tend to be predictable. Additionally, algorithm design is often considered within a data mining/processing context instead of from the context of human interaction and social media analytics. Finally, there is a lack of research focus associated with understanding multi-domain effects caused by the complexity of data within CPSS, particularly in the social space, especially when these effects are related to emergence. Social systems are inherently complex, albeit self-organizing, and subsequently CPSS research needs to consider the features of complexity within its models and frameworks. As an example, leveraging technologies such as machine learning and artificial intelligence to assist in modelling and data processing for CPSS is an opportunity. However, complications arise when considering the effects of emergence on pre-trained models, and unstructured, unlabeled human metric data sets.
There is a research need for future work that directly addresses these concerns relative to human metrics, an inclusive research ontology, and complexity considerations. This research would have to rely on the assumption that CPSS are inherently different to CPHS and that social systems are included within the CPSS boundary, instead of simply interfacing with it. The development of such a framework would then require testing against an established real-world CPSS that includes real social interactions for validation purposes, such as social media.
The process used to develop this work prioritized impact based on the number of citations individual publications have received. This approach therefore focuses on what has already been deemed impactful within the academic communities. Subsequently, there were some very recent publications that did not fall within the catchment of the analysis, but represent the leading edge of literature published within the last year. Such research still should be considered in future work in the field of CPSS.
Additionally, some of the existing novel literature focuses on fields relating to this paper’s research question. For example, Che et al.’s work into tensor factorization for fake news detection does not explicitly identify the system in question as a CPSS, but still offers valuable insight into CPSS research [ 109 ]. Other recent non-CPSS explicit literature that may contribute to CPSS future research related to this paper’s research focus include studies pertaining to modeling misinformation and bias within social networks and those that address emergence within CPS constructs [ 110 , 111 , 112 , 113 , 114 , 115 ]. Additionally, emergence in the field of cyber security is an identified area of research interest that is worth exploring within the context of CPSS and the IoT.
Ultimately, the field of Cyber–Physical–Social Systems is growing, which has the potential to underpin a multitude of cross-domain applications from healthcare to politics to algorithm optimization to Industry 4.0. Thus, building effective frameworks to understand this field and its cyber security implications is fundamental to ongoing reliance in both the cyber, physical and social spheres of influence.
8. Conclusions
The research field of CPSS is in an evolving state. Definitions, applications, and understandings of the concept are fluid and sometimes contradicting. This is exacerbated by its cross-applicability to multi-disciplinary applications, enabling silos of ontological understanding. However, the research in the field clearly points towards constructs of CPSS being a key enabler for future technological development and implementation into digitally enabled society, and thus warrants continued research and synthesis.
This work has shown that there is are several areas of CPSS that require additional research in order to reach its potential. This work has noted that the community is yet to standardize on common terms and definitions. This is not unexpected with such an emerging area. The development of structured representation mechanisms, such as formal ontologies, are one area of potential future research, that would assist in alleviating some of these issues.
Similarly, the broad nature of CPSS requires both a balance of the holistic in addition to detailed perspectives. This balance is difficult to achieve. The nature of complex systems makes this difficult, and there is more work to be carried out to understand some of the interactions of and between different domains.
Finally, this work has outlined the need for additional cybersecurity research both across both each domain of interest and at the areas that these domains integrate. The social aspect of cybersecurity is well known, but as CPSS evolves, so too will the challenges and opportunities in this area.
Social factors can heavily influence global current affairs, and thus the relationship between social agents and their Cyber–Physical counterparts presents a potentially volatile vector for influence, feedback, and emergence. Fake news, political events like the Arab Spring and Euromaidan, and economic events such as Gamestop all exemplify how Cyber–Physical Systems can have social components that affect outcomes in the real, physical world. Understanding how Cyber–Physical–Social Systems exist and operate is imperative to predict the emergence and the subsequent cyber security implications of these events.
Abbreviations
The following abbreviations are used in this manuscript:
MDPI | Multidisciplinary Digital Publishing Institute |
DOAJ | Directory of open access journals |
TLA | Three letter acronym |
LD | Linear dichroism |
IoT | Internet of Things |
CPS | Cyber–Physical System |
CPSS | Cyber–Physical–Social System |
C2 | Command and Control |
CPHS | Cyber–Physical–Human System |
HOBI-Lanczos | High-Order Bi-Lanczos |
IES | Intelligent Edge Services |
HAR | Human Activity Recognition |
Non-IID | Nonindependent and Identically Distributed |
ICPS | Industrial Cyber–Physical Systems |
ACP | Artificial Societies, Computational Experiments, and Parallel Execution |
Funding Statement
This research is funded by UNSW Canberra.
Author Contributions
Conceptualization, T.S. and B.T.; methodology, T.S. and B.T.; software, T.S.; validation, T.S., B.T. and N.M.; formal analysis, T.S.; investigation, T.S.; resources, T.S. and B.T.; data curation, T.S.; writing—original draft preparation, T.S.; writing—review and editing, T.S., B.T. and N.M.; visualization, T.S., B.T. and N.M.; supervision, B.T. and N.M.; project administration, B.T.; funding acquisition, B.T. All authors have read and agreed to the published version of the manuscript.
Institutional Review Board Statement
Not applicable
Informed Consent Statement
Data availability statement, conflicts of interest.
The authors declare no conflict of interest.
Disclaimer/Publisher’s Note: The statements, opinions and data contained in all publications are solely those of the individual author(s) and contributor(s) and not of MDPI and/or the editor(s). MDPI and/or the editor(s) disclaim responsibility for any injury to people or property resulting from any ideas, methods, instructions or products referred to in the content.
- Discovery Platform
- Innovation Scouting
- Startup Scouting
- Technology Scouting
- Tech Supplier Scouting
- Venture Clienting
- Startup Program
- Trend Intelligence
- Business Intelligence
- All Industries
- Industry 4.0
- Manufacturing
- Case Studies
- Research & Development
- Corporate Strategy
- Corporate Innovation
- Open Innovation
- New Business Development
- Product Development
- Agriculture
- Construction
- Sustainability
- All Startups
- Circularity
- All Innovation
- Business Trends
- Emerging Tech
- Innovation Intelligence
- New Companies
- Scouting Trends
- Startup Programs
- Supplier Scouting
- Tech Scouting
- Top AI Tools
- Trend Tracking
- All Reports [PDF]
- Circular Economy
- Engineering
- Oil & Gas
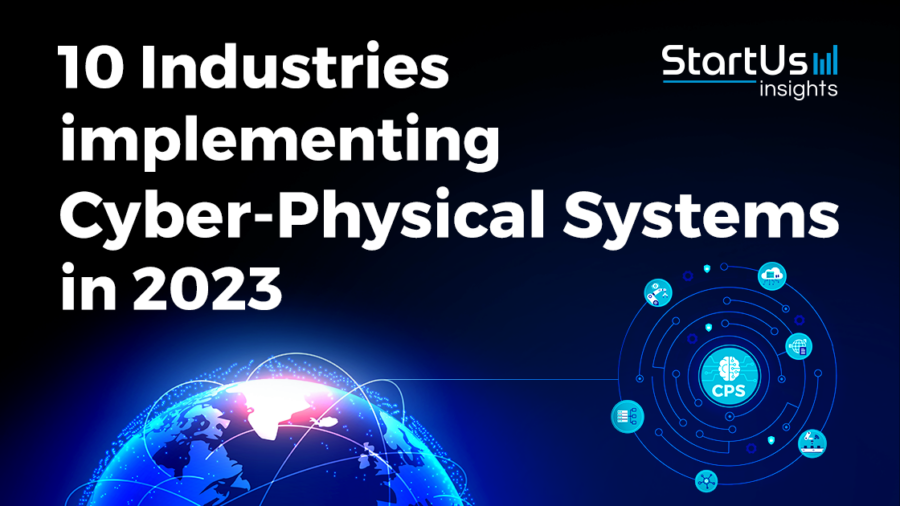
Share this:
- Click to share on Facebook (Opens in new window)
- Click to share on Twitter (Opens in new window)
- Click to share on LinkedIn (Opens in new window)

Top 10 Cyber-Physical Systems Examples in 2023 & 2024
How are businesses integrating various cyber-physical systems to increase productivity? Explore our in-depth analysis on the top examples of cyber-physical systems based on the analysis of 2300+ companies. These applications span autonomous vehicle systems, CPS security solutions, brain interfaces & more!
Cyber-physical systems (CPS) leverage computer-based algorithms to control and monitor mechanisms. They also feature intricate software-hardware interactions. Some examples include smart grids, autonomous vehicles, industrial control systems (ICS), and robotics. This research focuses on the top 10 industries advancing cyber-physical systems in 2023. For this, we analyzed 2313 emerging companies working in the field. Read more to explore the industry-specific applications of CPS and find out how they impact your business.
Tree Map reveals the Cyber-Physical Systems Examples across 10 Industries
Based on the Innovation Map, the Tree Map below illustrates the Applications of Cyber-Physical Systems in 10 industries in 2023. As digitization introduces large numbers of intelligent systems, their protection against cyber threats is very critical. That is why the cybersecurity industry is most impacted by CPS.
The manufacturing, utility, automotive, agriculture, industry 4.0, and aerospace industries integrate smarter assets, including Internet of Things (IoT) devices, to streamline operations. Further, CPS enhances the interfacing between humans and machines in the healthcare, smart city, and agriculture sectors.
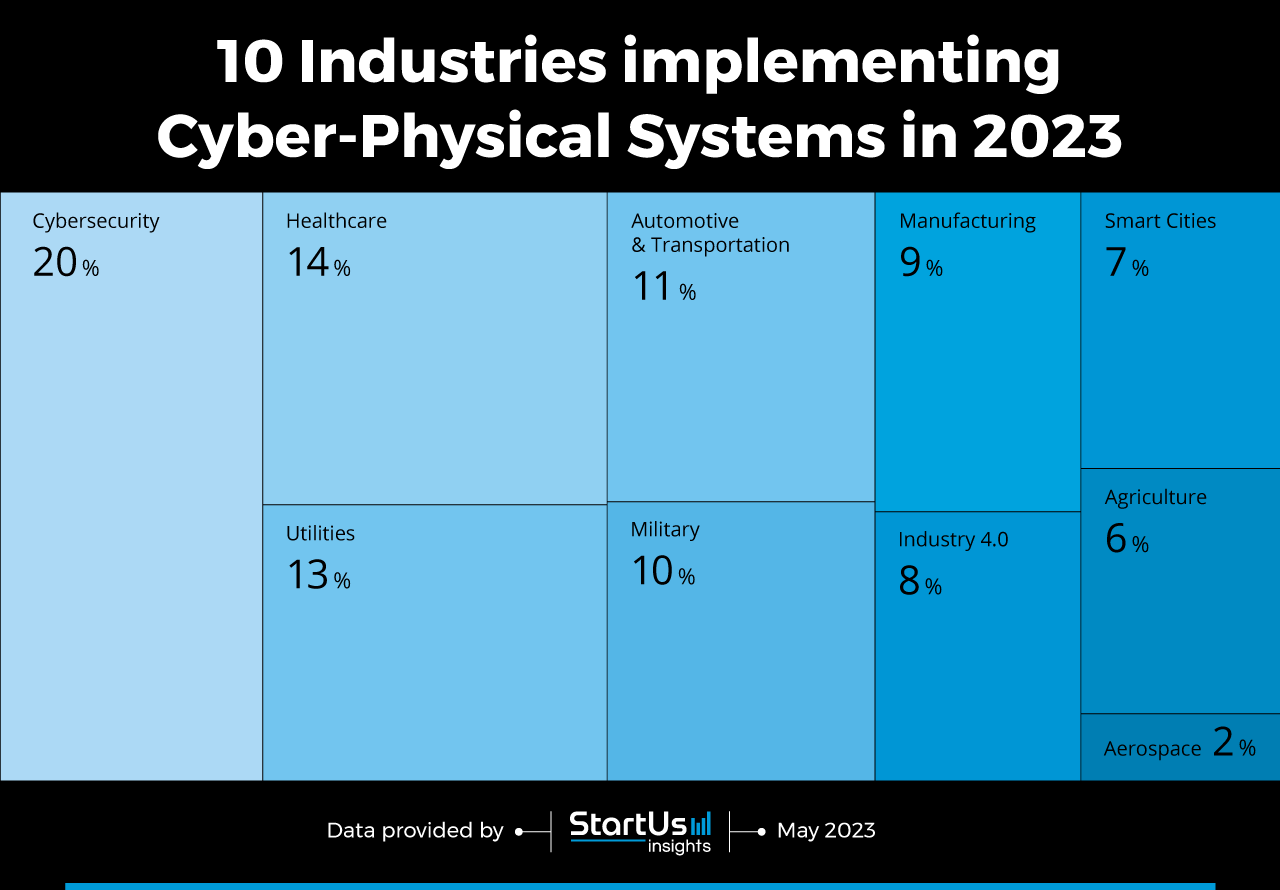
Click to download
Global Startup Heat Map covers 2313 Cyber-Physical System Startups & Scaleups
The Global Startup Heat Map below highlights the global distribution of the 2313 exemplary startups & scaleups that we analyzed for this research. Created through the StartUs Insights Discovery Platform that covers 3 790 000+ startups & scaleups globally, the Heat Map reveals that the US has a high concentration of cyber-physical system companies, followed by Western Europe and India.
Below, you get to meet 10 out of these 2313 promising startups & scaleups as well as the solutions they develop. These emerging CPS companies are hand-picked based on criteria such as founding year, location, funding raised, & more. Depending on your specific needs, your top picks might look entirely different.
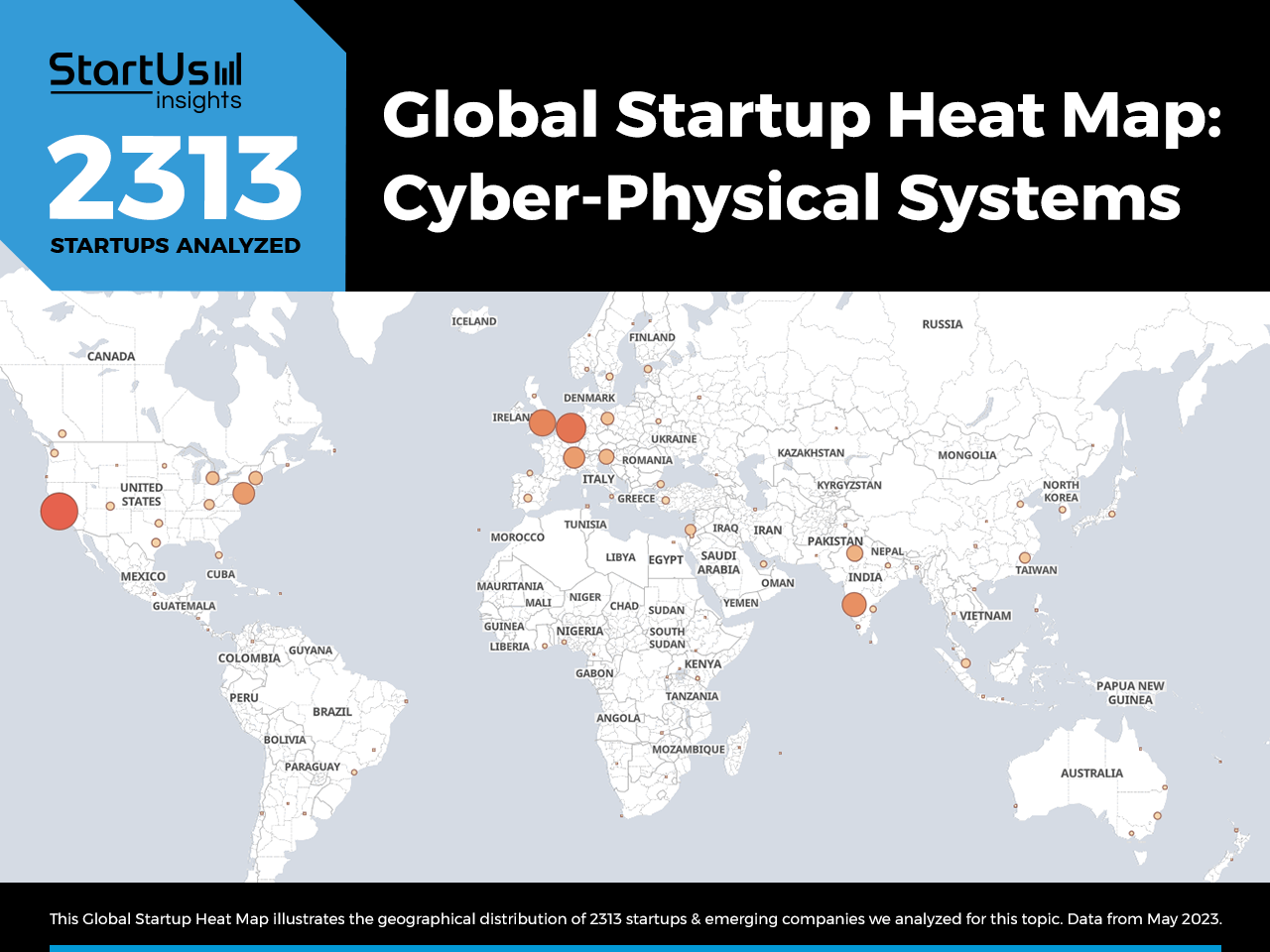
Interested to explore all 2000+ cyber-physical system startups?
Top Cyber-Physical Systems Applications across 10 Industries
1. cybersecurity.
The large-scale integration of cyber-physical systems expands the attack surface for cyber threats. Such malicious actors exploit vulnerabilities and compromise critical systems, leading to infrastructure downtime and sensitive data leaks. To tackle this challenge, the cybersecurity industry is leveraging encrypted communication systems and real-time network and device monitoring.
Further, blockchain drives business decisions and operations with immutable and tamper-resistant data while decentralizing access control. CPS developers are also transitioning to a security-first design approach to ensure data security. Other solutions to tackle cyber attacks against intelligent systems include anomaly detection, AI-assisted protection, and specialized software against ransomware.
C3i Hub enables CPS Intrusion Detection
C3i Hub is an Indian startup that facilitates intrusion detection for cyber-physical systems. The startup’s solution, RobustD , and an integrated controller are deployed in the operational technology (OT) layer. It collects data related to OT devices, calculates anomalies for newly generated measurements, and detects intrusion.
For this, the startup uses machine learning. RobustD thus aids infrastructure management in cities, smart car operations for automotive businesses, and patient tracking in healthcare.
2. Healthcare
Applications of CPS in healthcare operations lead to improved patient monitoring and medication management. Many CPS workflows also automate labor and time-intensive manual tasks while reducing costs. Telehealth solutions are extensions of such intelligent systems that improve access to healthcare. Advances in healthcare CPS include the Internet of Medical Things (IoMT), healthcare AI, machine learning, medical robotics, and more.
For example, healthcare institutions apply AI to analyze massive datasets and improve disease diagnosis as well as deliver personalized treatment recommendations. Additionally, virtual and augmented reality(VR/AR)-powered CPS solutions streamline surgical planning and pain management.
Precision Neuroscience makes a Brain-Computer Interface
Precision Neuroscience is a US-based startup that develops a brain-computer interface. Its flexible electrode arrays are implanted using a micro-slit technique and conform to the brain’s surface to maximize data collection without damage.
In clinical trials, the company’s technology has successfully mapped human brain activity. Using the technology, the startup assists patients with conditions like stroke, dementia, and other neurological conditions.
3. Utilities
Cities now integrate real-time data processing, process automation, and control systems to optimize the operations of utility companies. They improve power generation as well as energy and water distribution and consumption. For example, smart grids collect data across regions to derive insights into power consumption patterns and enable predictive maintenance. This allows energy companies to enhance demand response efforts and avoid blackouts.
Such solutions also support water and sewage infrastructure in making maintenance more streamlined while improving customer satisfaction. Further, implementing intelligent systems, like industrial IoT (IIoT) devices, predictive analytics, and blockchain, aids the integration of distributed energy resources (DERs). This, in turn, reduces the intermittency of renewable resources and accelerates the transition to a low/zero-carbon economy.
Orbis Intelligent Systems offers a Smart Hydrant
Orbis Intelligent Systems is a US-based startup that offers a smart hydrant for utilities. Its multi-sensor configuration detects various pipe and flow conditions and it uploads the data to a portal. The portal, ADARI , allows operators to access these scheduled reports and offers real-time alerts on unauthorized extraction and illegal tampering, reducing leakage and water theft.
The startup also develops a retrofit device, SmartCap , for existing fire hydrants. These solutions enable utilities and municipalities to track water pipeline networks in real time, optimize maintenance planning, and reduce non-revenue water (NRW).
4. Automotive & Transportation
Cyber-physical systems are impacting the automotive and transportation sectors in two key segments – production and post-sales. Automakers integrate IoT, AI, and more at factory floors to increase manufacturing throughput. Other solutions in the sector include advanced driver assistance systems (ADAS), autonomous driving systems, and vehicle-to-everything (V2X) connectivity technologies.
While production-ready solutions cater to the manufacturing segment, the latter elevates the driving experience for vehicle owners. Through such solutions, vehicle manufacturers and mobility service providers ensure passenger and road safety. Further, predictive analytics allows automakers to forecast potential machine and vehicle breakdowns to improve maintenance planning and safety.
Aura Intelligent Systems provides High-Definition (HD) Radar Perception
Aura Intelligent Systems is a US-based startup that delivers HD perception for vehicle radar imaging systems. The startup’s technology combines 5G, sensing, and intelligent software to enable high-resolution 5D information – 3D location, doppler, and signature.
This enables a low-false alarm perception system that enhances the performance of autonomous mobility solutions like drones and ground vehicles. With the startup’s solution, automakers and mobility providers are able to improve vehicle navigation, road safety, and traffic management.
5. Military
Real-time data acquisition, improved communication, and autonomous systems for surveillance and reconnaissance greatly increase the efficiency of military operations. Swarm technology, augmented reality (AR), satellite, edge computing, and unmanned system integrations are key enablers of such solutions. Loitering munitions, also known as kamikaze or suicide drones, and countermeasures against them are gaining interest due to their recent use in the Russo-Ukrainian war.
Through these systems, military organizations improve situational awareness, command and control, personnel training, and more. Militaires further leverage novel encryption techniques and blockchain to make data security and privacy more effective as well as tackle information warfare.
SightX manufactures Edge AI-powered Military Drones
SightX is an Israeli startup that manufactures edge AI-powered military drones. The startup’s autonomous drones leverage electro-optics-based autonomy and connectivity at low power requirements.
Its multi-object acquisition engine also aids target or personnel tracking for mission-critical applications on air, land, and maritime-based platforms. This allows militaries to improve situational awareness and safety on battlefields.
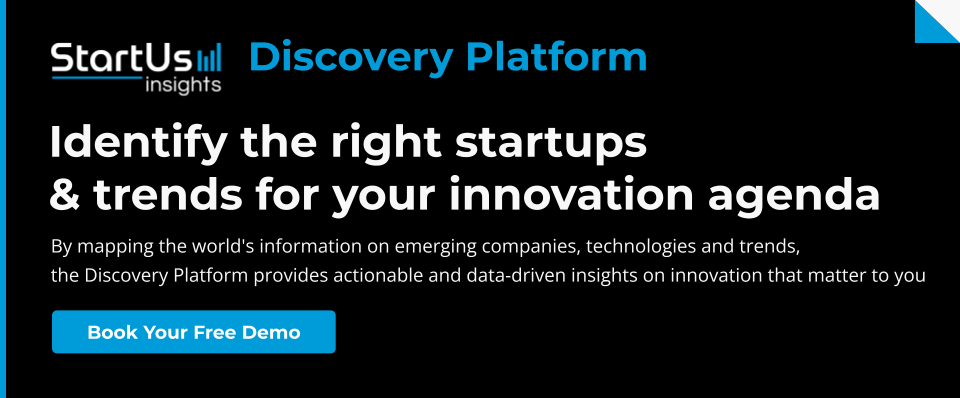
6. Manufacturing
The manufacturing industry utilizes IIoT, digital twins, 3D printers, and cobots, among other cyber-physical systems. They allow businesses to collect production data in real-time, power data processing algorithms, and improve process visualization. Further, 3D printers and robotic systems automate manufacturing workflows to increase throughput and quality control.
Such intelligent systems in manufacturing floors also increase operational flexibility and customization to enable agile workflows. Assisted maintenance is another critical application of CPS in manufacturing. Historic and real-time asset information allows floor managers to monitor equipment health and promptly detect anomalies. This proactive approach reduces unplanned downtime and extends equipment lifespan.
IN40S implements On-Demand Smart Factories
IN40S is a US-based startup that makes cyber-physical infrastructures for the manufacturing sector. The startup’s on-demand smart factory, i-Optimus , combines additive manufacturing, multi-material support, cobots, post-processing equipment, and more. It also features scalable architecture, edge and cloud-powered, AI, and digital warehouse as well as physical security and zero-trust architecture. This allows manufacturers to mitigate in-house hardware and software development while delivering manufacturing at scale.
7. Industry 4.0
Cyber-physical systems are critical factors that enable smart or connected and automated factories. To achieve this, CPS developers leverage low-latent connectivity like 5G, IoT, edge AI, and more. For instance, data-driven process automation platforms and systems allow businesses to streamline their operations while saving cost and time. Digital twins enable companies to track operations in real time while blockchain ensures transparent operations and cybersecurity.
CPS solutions in Industry 4.0 further ensure seamless connectivity across the manufacturing ecosystem and aid human-machine collaboration. For this, businesses leverage cobots and novel human-machine interfaces (HMIs), which enhance productivity and safety. Lastly, CPS enables intelligent supply chains that ensure real-time visibility and traceability while delivering demand forecasts and inventory management.
NQMCyber improves CPS Security
NQMCyber is an Irish startup that creates secure cyber-physical systems. The startup’s high-performance industrial hub features trusted platform modules (TPM), secure boot, and other hardware-based integrity checking. It also features secure storage, multiple network connectivity, AI, IoT, and edge processing to deliver advanced analytics for industries.
8. Smart Cities
Implementing intelligent systems in cities supports four key areas – mobility, infrastructure management, public safety, and energy management. Smart cities utilize IoT sensors, edge devices, AI, connected grids, and more. They enable efficient infrastructure management to track and optimize energy, waste management, and public safety systems. Additionally, intelligent transportation systems aid real-time traffic monitoring, smart traffic signal control, and optimized routing.
This reduces congestion and improves traffic flow. CPS integration further enables demand response programs, facilitates renewable integration, optimizes grids, and more. In the public safety sector, cyber-physical systems increase the performance of surveillance and emergency response systems to monitor critical areas and quickly detect potential threats. These solutions foster citizen engagement and improve the quality of life in cities.
Allas Network aids in Traffic Optimization
Allas Network is a Swedish startup that provides a traffic optimization solution. It combines hardware and software components, Allas Street and Allas Platform . Allas Street features AI-powered cameras, thermal monitoring, and various sensors. Additionally, Allas Platform offers live video feeds and environmental data securely from the distribution sites.
It also preserves citizen privacy with anonymized data, enhances access management, and utilizes an immutable database. The startup’s other scalable hardware platform, Allas Intelligence , uses AI and machine learning. It analyzes data from the Allas Platform to measure air quality and CO2 levels, count pedestrians on crossings, and improve road safety.
9. Agriculture
IoT devices, agricultural drones, blockchain-powered platforms, and smart irrigation systems are the most common cyber-physical systems in agriculture. Connected sensors provide farmers with vital plant and soil health parameters like pH and moisture levels to enable real-time and remote monitoring. Autonomous drones capture and analyze aerial imagery to assess crop health and ensure targeted interventions, also enabling precision agriculture.
Farmers are also transitioning to automated food production systems, such as automated vertical farms and controlled environment agriculture (CEA). This optimizes growing conditions, reduces resource consumption, and enables year-round food production. Moreover, agricultural robots, including autonomous tractors and robotic arms, simplify various farming operations like harvesting and weeding. Lastly, CPS in livestock management aids environmental and animal health monitoring as well as enables automated livestock handling.
Machine Eye Technology facilitates Autonomous Risk Assessment
Machine Eye Technology is a UK-based startup that offers autonomous risk assessment using off-highway vehicles. It leverages edge computer vision and deep learning to continuously assess risks in real time and identify humans.
This solution enables autonomous tractors to navigate in fields with humans and improve worker safety. For example, Agri-EPI Centre utilizes the startup’s perception system to detect people who are not within a safe distance.
10. Aerospace
Advanced flight control systems use sensor data, automation systems, and algorithms to improve flight stability, reduce pilot workload, and improve maneuverability and safety. Further, aerospace manufacturers apply digital twins to simulate and test aircraft designs and monitor aircraft parameters in real-time to enhance maintenance planning. They also leverage additive manufacturing to accelerate rapid prototyping, product/part customization, and reduce lead times.
Other cyber-physical systems in the aerospace industry include IoT devices to improve situational awareness, AI to speed up fault diagnosis, and cybersecurity systems to ensure data integrity and confidentiality. These applications of CPS further enhance operational efficiency, safety, and air traffic management while enabling remote monitoring and diagnostics.
Developair Technologies enhances Aerospace CPS Security
Developair Technologies is a Spanish startup that creates cybersecurity software for cyber-physical systems in the aerospace industry. The startup’s platform offers intelligent assistance across multiple phases of the software development cycle.
It also allows developers to define architecture, features cloud processing, and supports interaction with complementary tools. The startup’s software accelerates software validation and automatically generates tests and oracles based on specific requirements. As a result, it saves costs associated with software specification, implementation, and testing.
Discover All Cyber-Physical System Startups
AI-driven and edge or fog-hosted CPS will move data processing closer to the edge while ensuring high-performance data processing. IoT integration is accelerating across industries, powering more and more intelligent systems. Further, swarm robotics, digital twins, 5G, and explainable AI will improve the performance of cyber-physical systems while novel cybersecurity solutions are critical to keep them online. These technologies, along with other innovations in the field, will make CPS more accessible and interoperable. Get in touch to identify specific cyber-physical system startups & solutions that advance your business!
Your Name Business Email Company
Get our free newsletter on technology and startups.
Protected by reCAPTCHA and the Google Privacy Policy and Terms of Service apply.
Discover our Free Industry 4.0 Report 22 pages
Get free updates on Global Startups, Technologies & Trends!
Join 25k subscribers.
Business Email
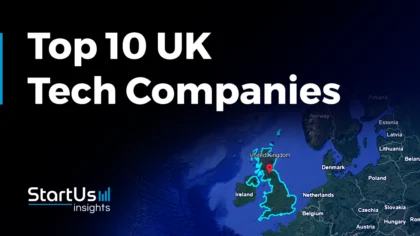
Industry 4.0 22 pages report
Manufacturing 22 pages report, energy 21 pages report, autonomous vehicles.
First & Last Name Business Email Company
Leverage our unparalleled data advantage to quickly and easily find hidden gems among 4.7M+ startups, scaleups. Access the world's most comprehensive innovation intelligence and stay ahead with AI-powered precision.
Get in touch
Your Name Business Email Company How can we support you? (optional)
Explore 30+ Emerging Tech Startups for Free
Get ahead with our interactive Innovation Map, featuring 30+ of the most promising tech startups. Download now to discover top tech companies and accelerate your innovation agenda.

Protected by reCAPTCHA and the Google Privacy Policy and Terms of Service apply.
Advertisement
Cyber-Physical Systems in the Context of Industry 4.0: A Review, Categorization and Outlook
- Open access
- Published: 07 April 2022
Cite this article
You have full access to this open access article
- Sascha Julian Oks ORCID: orcid.org/0000-0001-9035-4248 1 ,
- Max Jalowski ORCID: orcid.org/0000-0002-7676-5662 1 ,
- Michael Lechner 2 ,
- Stefan Mirschberger 3 ,
- Marion Merklein ORCID: orcid.org/0000-0003-0609-7301 2 ,
- Birgit Vogel-Heuser ORCID: orcid.org/0000-0003-2785-8819 4 &
- Kathrin M. Möslein ORCID: orcid.org/0000-0002-5783-9857 1
12k Accesses
18 Citations
1 Altmetric
Explore all metrics
Cyber-physical systems (CPS) offer great potential for the digital transformation of industrial value creation in the context of Industry 4.0. They unify and integrate several technological approaches, including big data analysis and artificial intelligence, enhancing real-time monitoring and control of manufacturing processes. An extensive knowledge base formed by various disciplines, including information systems, engineering, and computer science, already exists for CPS. However, this knowledge has not been holistically captured and structured to date. To address this research gap, this study conducts a large-scale literature review of 2365 papers representing the current state of the research and then develops a novel categorization on industrial CPS with 10 sections, 32 areas, and 246 fields. The categorization is presented in hierarchical graphical form and can also be utilized as a web tool. To conclude, a perspective on future research needs and potentials to enhance Industry 4.0 in both research and practice are offered.
Similar content being viewed by others
Principles for Human-Centered System Design in Industry 4.0 – A Systematic Literature Review
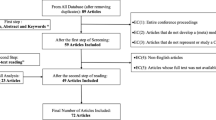
A Review and Analysis of the Characteristics of Cyber-physical Systems in Industry 4.0
The Cyber-Physical Systems Within the industry 4.0 Framework
Explore related subjects.
- Artificial Intelligence
Avoid common mistakes on your manuscript.
1 Introduction
Industrial value creation is undergoing significant changes as part of the digital transformation with Industry 4.0 as the guiding term (Lasi et al., 2014 ), which emerged from a German funding initiative in 2013 with a number of program equivalents worldwide (Li, 2018 ). Cyber-physical systems (CPS), in addition to other technologies and concepts, are of particular relevance for this process (Zhang et al., 2021 ). With their general concept of, “[…] integrations of computation with physical processes. Embedded computers and networks monitor and control the physical processes, usually with feedback loops where physical processes affect computations and vice versa” (Lee, 2006 , p. 1), CPS offer extensive application potentials within the industrial domain (Oks et al., 2017 ). In this, they contribute to the realization of use cases like the real-time monitoring and control of systems and processes, predictive maintenance, and the expansion of human-machine collaboration, among others, and therefore drive the optimization of production, products, and services with increased effectiveness and efficiency (Colombo et al., 2017 ).
However, as CPS are utilized both in industry and many other application domains, they qualify as general purpose technology (Bresnahan, 2010 ). As is to be expected with a technology/a concept that is associated with vast potentials and a wide range of application possibilities, CPS attract research interest from a variety of scientific disciplines and are utilized by numerous communities of practitioners. CPS are therefore constantly being assessed from different perspectives, including the technological (hardware, software, architectures, information systems, etc.), the process-oriented (applications, procedures, operations, etc.), the organizational (value creation, cost-benefit considerations, business models, etc.), the socio-technical (human-computer interaction (HCI), work design, etc.) and others (Geisberger & Broy, 2015 ). As a result, an extensive knowledge base on the subject of CPS and their application in the industrial domain has already been established. However, this knowledge base is very diverse and wide-ranging and therefore complex and difficult to determine.
It is therefore the motivation of this research, in order to exploit the full potential of CPS for the further establishment of Industry 4.0 (Vogel-Heuser & Hess, 2016 ), to disclose and examine the current state of knowledge on CPS and to categorize all CPS related and relevant topics within the industrial application domain, which is highly relevant and of great value to both the research and practice-oriented communities. This paper addresses the aforementioned research demand with the following two objectives: (I) Describe and analyze the state of research on CPS. (II) Develop and graphically present a categorization of CPS related and relevant topics in the context of Industry 4.0. This includes all subjects, technologies, concepts, and procedures that are related or relevant to industrial value creation. Both objectives are addressed in a comprehensive manner, based on a large-scale literature review. Concerning the first objective, the state of research is not thematically restricted to allow the derivation of analogies from other disciplines. This takes into account that CPS are studied and elaborated by a large number of disciplines, particularly in the area of basic research, of which the resulting knowledge cannot be strictly divided according to application domains. An exclusive focus on the area of manufacturing would therefore leave out relevant knowledge. Upon the second objective, the categorization is exclusively focused on CPS related and relevant topics in the context of Industry 4.0. This focus is feasible since for this objective it is no longer a matter of knowledge collection but of the subsequent step of arranging it in a structured, hierarchical, and comprehensive manner. The system of a categorization is therefore suitable, as it allows to present a vast amount of topics in a well-arranged form while also showing their interrelationships.
Thus, this paper provides an overview of research on CPS with pertinence for the Industry 4.0 domain and a categorization of all relevant topics for industrial CPS. It differs from a research survey paper in that it identifies and categorizes the topics but does not describe and analyze them in full depth, as this is done through narrowly defined concept-specific reviews as presented in related work chapter in Section 2.2 .
The paper is structured as follows: After the introduction, the theoretical foundations of CPS in the industrial context are laid and related work is presented in Section 2 . The third Section presents the methodological approach for the literature review and the subsequent analysis and development steps. The resulting state of research and categorization are presented in Section 4 in particular in graphical form. In Section 5 the paper closes with conclusions and an outlook regarding further consolidation and research needs in the field of industrial CPS.
2 Cyber-Physical Systems as an Enabler for Industry 4.0
This Section lays the groundwork for this research; the theoretical foundations of CPS are presented in 2.1 and additional related work is introduced that also seeks to structure industrial CPS in 2.2 .
2.1 Foundations of Cyber-Physical Systems
The term CPS was introduced by E. A. Lee in 2006; since then, the topic has been further analyzed and developed in several scientific and practical disciplines (Geisberger & Broy, 2015 ). In essence, CPS are embedded systems that have both a cyber and a physical sphere between which there is a continuous and iterative information exchange (Alur, 2015 ). In the physical sphere, sensors are used to record environmental conditions, which are then evaluated in the cyber sphere using local computing power. The information obtained from the data stream can be either exchanged with other entities via communication interfaces or used in the physical sphere to affect the environment according to predefined rules of behavior via actuators (Lu et al., 2016 ). CPS can therefore be used for monitoring as well as controlling digital, physical, and especially hybrid processes (Jiang et al., 2018 ). The continuing miniaturization of computer hardware to the point of smart dust, coupled with the ongoing reduction of component costs, enables CPS to be used extensively in a wide range of contexts and conditions (Rajkumar et al., 2010 ). They can also be operated either completely autonomously or in collaboration and interaction with humans (Gil et al., 2019 ).
Three dimensions are to be taken into account in the design, development, and operation of CPS, the technical, the human/social, and the organizational (Oks et al., 2017 ). In the technical dimension, application-specific and requirement-meeting hardware and software have to be orchestrated with an appropriate architecture. Furthermore, the CPS has to be integrated into existing physical and digital infrastructures, and desired interoperability with other systems (Gürdür et al., 2016 ) should be ensured by means of norms and standards (Hehenberger et al., 2016 ). The human/social dimension comprises the integration of humans into or the interaction of humans with a CPS. In this dimension, HCI, safety in use, and the consideration of ethical issues in system design are of major importance (Calinescu et al., 2019 ). The organizational dimension is determined by the insertion of the CPS into the application purpose and context within various institutional structures and frameworks (Oks et al., 2018 ).
In addition, CPS can be divided into three levels that categorize their application in terms of system size and reach. At the micro level, CPS are used in a personal or small-group individual context, usually limited to a local area. At the meso level, CPS applications are organization-wide and can have interregional system dimensions. At the macro level, CPS are deployed, often as volatile systems of systems (Trunzer et al., 2020 ), in application scenarios that encompass entire national economies or are even more far-reaching, and are designed transregionally or globally (Oks et al., 2017 ). Within these levels, CPS are used in various domains in the context of the digital transformation. These include urban development ( Smart City ), healthcare ( Smart Health ), mobility ( Smart Mobility ), building management ( Smart Home ) and, most prevalently, industrial value creation ( Smart Manufacturing ) (Geisberger & Broy, 2015 ). As previous stated, CPS qualify as a general purpose technology because of this wide range of applications across levels and domains (Bresnahan, 2010 ). The distinctive characteristic of technologies of this kind is that they can be used widely and cross-functionally with a high level of utility. Like previous general purpose technologies, such as the steam engine, assembly lines, or computers, CPS, in combination with other technologies of the digital age, are attributed the potential to unleash a surge in productivity that is qualifying to induce an industrial revolution (Liao et al., 2016 ; Rosenberg & Trajtenberg, 2009 ). For this reason, the digital transformation of industrial value creation through CPS is discussed under the guiding term Industry 4.0 (Lu, 2017 ), which anticipates these far-reaching changes. Among other things, the establishment of CPS is considered to have the potential to address market megatrends, such as increasing individualization, dematerialization, and servitization, as well as increase sustainability by both optimizing existing processes and outputs and innovating new ones (Geisberger & Broy, 2015 ).
CPS used in the industrial domain are referred to as industrial CPS (Colombo et al., 2017 ). This term is used inclusively and covers not only CPS used in manufacturing, but also peripheral ones, such as in smart products, which provide relevant data for value creation (Oks et al., 2017 ). Industrial CPS are therefore broader in scope than cyber-physical production systems (CPPS) (Monostori, 2014 ). The schematic functioning of industrial CPS, which is shown in Fig. 1 , can be described as follows: In the physical sphere, state data is collected throughout the production and product life cycle (Tao et al., 2020 ). This includes smart (raw) materials/components in the pre-production stage, CPPS in the production stage, and subsequently, smart products in the product in use stage. The recorded data is then used in the cyber sphere in two ways. First, in real-time for monitoring and control of statuses and processes. Threshold values and algorithms are used to detect (imminent) events to react in such a way that corresponding actuators are triggered in the physical sphere according to pre-defined system logic (Jiang et al., 2018 ). Second, the collected data is processed and aggregated in the form of a digital twin for production plants and (sub-)products in the long term (Biesinger et al., 2019 ). In addition, the vast, continuously growing data sets are analyzed using big data analytics (Marini & Bianchini, 2016 ). The insights gained in this way are then used to optimize the real-time methods of monitoring and control, thereby continuously enhancing the performance of the industrial CPS. Based on this scheme, industrial CPS constitute the basis for a large number of use cases in Industry 4.0, including predictive maintenance (Meesublak & Klinsukont, 2020 ), order and batch size planning (Huang et al., 2021 ), energy management (Ma et al., 2019 ), disaster prevention (Lei et al., 2020 ), and quality control (Colledani et al., 2018 ), among others.
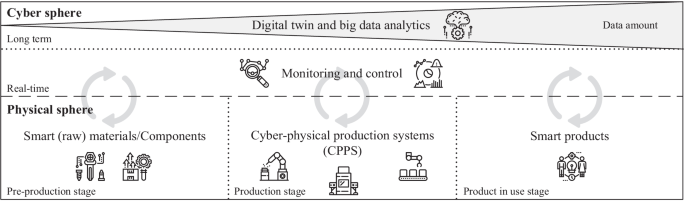
Schematic functioning of industrial CPS
2.2 Related Work
As is to be expected with a widely established general purpose technology, the literature base on CPS is already exceedingly comprehensive. Literature that is relevant for this research as related work in the form of reviews or systematizations on the topic of CPS can be divided into general, topic-specific, and industry-oriented perspectives.
As part of the general examination, Chen ( 2017b ) reviewed and analyzed the theoretical foundations of CPS. In another general review on CPS, Liu et al. ( 2017 ) highlighted the system integration, architectures, and challenges associated with CPS. Using a less theoretical orientation, Hehenberger et al. ( 2016 ) introduced methods and applications for the design, modelling, simulation, and integration of CPS. Adjacent to these topics, there is a systematic review on interoperability and integration in the context of CPS by Gürdür and Asplund ( 2018 ). Besides the contributions mentioned above, which approach the subject of CPS from a broad viewpoint, there are also reviews, such as that by Muccini et al. ( 2016 ), on system self-adaptation, which examine CPS in general but exclusively with respect to one characteristic. In addition to reviews, there are also structuring works on CPS, such as that by Asare et al. ( 2012 ), who designed a CPS Concept Map with 51 items (e.g., applications, requirements, etc.) and their relations based on taxonomy developed during the 2012 NIST CPS Workshop.
Topic-specific research focuses on dedicated applications, technologies, or domains pertaining to CPS. A general overview of possible applications is given in a review by Chen ( 2017a ). In this study, ten application areas are described and analyzed. The survey on CPS security by Humayed et al. ( 2017 ) is an example for reviews focusing on exclusively one application field. Other reviews, like those on blockchain-enabled CPS (Zhao et al., 2021 ) or CPS clouds (Chaâri et al., 2016 ), concentrate on technologies and their integrated operation with CPS. There are also dedicated reviews on CPS utilization in specific domains, such as the one by Haque et al. ( 2014 ) on healthcare.
There is also a wide range of preliminary work in the Industry 4.0 domain. For example, Dafflon et al. ( 2021 ) dealt with the general challenges, approaches, and used techniques of CPS for manufacturing in their literature review. The relevance of CPS to complementary concepts and technologies, such as internet of things (IoT), big data, and cloud computing, in the context of digitalized industrial value creation has been analyzed (Kim, 2017 ). The question of interoperability standards to enable interconnectivity between these technologies and the devices employing them was addressed in the review of Burns et al. ( 2019 ). Furthermore, a systematic mapping study of architectures, technologies, and challenges for CPS in Industry 4.0 was conducted by Hofer ( 2018 ). Further articles focusing on CPS architectures for manufacturing were contributed by Lee et al. ( 2015 ) and Pivoto et al. ( 2021 ), whose reviews drew specific attention to applications involving the industrial internet of things. Other reviews investigated the characteristics of CPS in the context of smart factories (Napoleone et al., 2020 ) and smart manufacturing (Thoben et al., 2017 ). The topic of smart manufacturing, in particular the control of its processes, was also examined in a literature review by Rojas and Rauch ( 2019 ). The design process of CPS for manufacturing was analyzed in the course of a literature review by Lozano and Vijayan ( 2020 ); Hermann et al. ( 2016 ) contributed design principles for Industry 4.0 scenarios. A general state of the art on the topic of Industry 4.0 with an additional outlook on future trends was provided by (Xu et al., 2018 ). In addition to the analytical studies and reviews cited above, there is also research on industrial CPS that present concepts that structure thematic areas in different forms. Against this backdrop, Monostori et al. ( 2016 ) offered 23 keywords, roots, expectations towards research, case studies, and R&D challenges regarding the implementation of CPS in manufacturing. Additionally, an application map for industrial CPS was introduced by Oks et al. ( 2017 ), which indicates specific CPS application fields for both production and smart products. In addition, a taxonomy consisting of nine items for techniques for approaching big data-related issues in CPS by Xu and Duan ( 2019 ), a classification of CPPS applications provided by Cardin ( 2019 ) and a concept map of CPPS research topics by Wu et al. ( 2020 ) should be mentioned in this regard. Along with that, Berger et al. ( 2021 ) provided a terminology, taxonomy, and reference model for entities in CPPS from a self-organizing systems’ perspective. Concluding, there was a trend map for cyber-physical systems research and education in 2030 introduced by Gürdür Broo et al. ( 2021 ), that provides 44 possible influencing factors in 7 categories regarding this topic.
Although the work outlined above is very extensive and contributions came from a wide variety of disciplines, there is yet no comprehensive approach to the topic of CPS in the form of a state of research nor a categorization of CPS related and relevant topics in the context of Industry 4.0 that are presented coherently in a suitable form. This work addresses this research gap through its two objectives.
3 Research Method
In order to address the two research objectives of this study, we chose the following research design: A comprehensive systematic literature review for data collection was conducted. The resulting data set was analyzed and transferred into a state of knowledge on CPS research and a categorization of CPS related and relevant topics in the context of Industry 4.0. Following the suggestions of vom Brocke et al. ( 2015 ) for conducting literature searches in information systems research, we defined the search scope as follows. We chose a sequential process, following the recommendations of Tranfield et al. ( 2003 ), which is described in more detail in subsequent paragraphs. Indexing services and databases were chosen as sources (cf. Fig. 2 ). As described in the motivation for this research, both the state of knowledge on CPS and the categorization of CPS related and relevant topics in the context of Industry 4.0 are intended to provide a comprehensive, general, and holistic overview of the subject area The coverage of our literature search, therefore, is comprehensive as well, in order to include as many relevant publications as possible (cf. vom Brocke et al., 2015 ). In terms of technique, we primarily applied a keyword search; the exact procedure is described below.
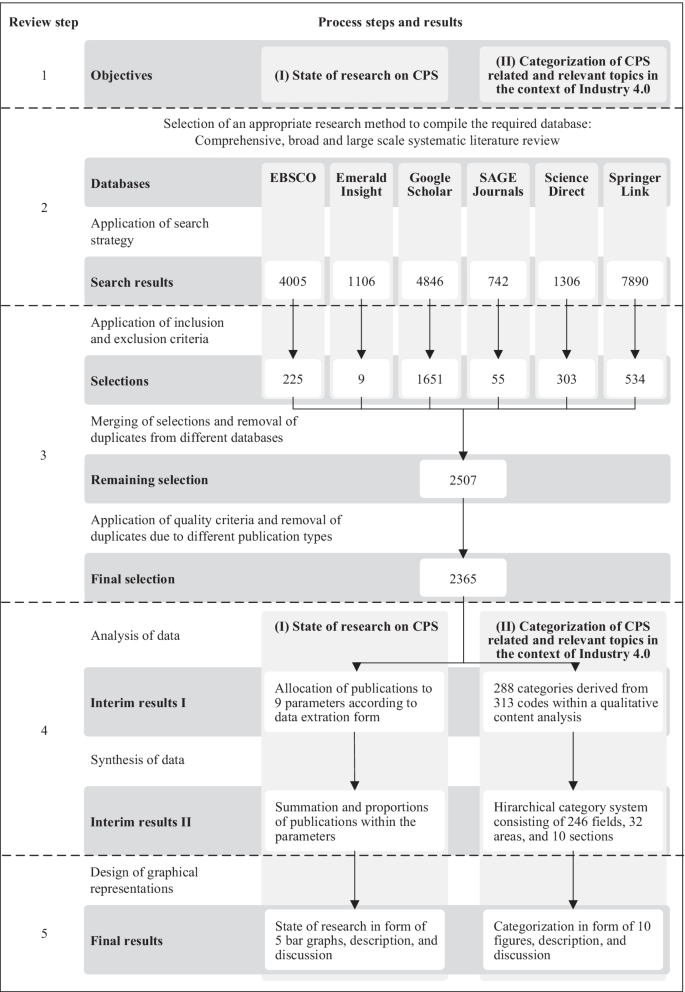
Process of the systematic literature review
A systematic literature review was chosen for data collection and analysis because of its transparent, exhaustive, and heuristic qualities. In a systematic literature review, research contributions on a specific topic are localized, assessed, and interpreted. It differs from a traditional narrative review because of its methodological strategy and the detailed description of each individual process step. Furthermore, it aims at minimizing bias and increasing the reproducibility and transparency of the researchers’ approaches, decisions, and conclusions (Tranfield et al., 2003 ). The concrete procedure follows the recommendations of Denyer and Tranfield ( 2009 ) and Tranfield et al. ( 2003 ); it consists of five steps with sub-steps. All of these were performed manually by the research consortium of this paper, with one exception where the Citavi 6 function to detect and sort out duplicates was used. There were no fewer than five researchers involved in any step of the workflow. Table 1 explains the five main steps of the systematic literature review.
In the first step (1), the two research objectives were defined according to the motivation for this research: The first objective is (I) to describe and analyze the state of research on CPS, and the second is (II) to develop and graphically present a categorization of CPS related and relevant topics in the context of Industry 4.0.
The second step (2) was to identify the relevant literature needed to achieve the defined research objectives, which included screening, selecting, and assessing the search results. For the search, the online databases and library services EBSCO, Emerald Insight, Google Scholar, SAGE Journals, Science Direct, and Springer Link were selected to cover all relevant subject areas. The advanced search function was used in all databases for more comprehensive search options. The keywords were based on the term “cyber-physical systems”, considering different spellings in the existing literature. Both synonyms and plural forms were used to ensure an exhaustive search as well as comprehensive and valid results. The complete list of search terms is available in Appendix Table 3 . The keywords and their synonyms were combined to search strings by using Boolean operators. The keyword “Industry 4.0” was intentionally omitted despite the thematic focus of the categorization within this context. This approach ensures that CPS sources relevant to the Industry 4.0 domain that do not explicitly contain the term Industry 4.0 in their title, keywords or abstract are also collected and considered. E.g., this becomes evident with terms such as smart factory, smart manufacturing, etc., since these tend to be used synonymously for Industry 4.0 and also for each other. Moreover, there are many papers on niche topics that only address a technical problem, phenomenon, etc., but are relevant to CPS in general, regardless of the respective application domain.
In the third step (3), the search results were screened and selected based on definite inclusion and exclusion criteria. The inclusion criteria were the containment of keywords or synonyms and relatedness to the topic. Exclusion criteria included publication languages different from English or German, inadequacy of outlet Footnote 1 , or the use of CPS as an abbreviation with a different meaning. Using the described concepts as search terms, the search returned 2777 publications. The procedure was as follows: The first reduction was realized in the databases EBSCO, Google Scholar and Springer Link, where the selection of the option “Source type is different from “Academic Journal”” and “Title does not contain at least one of the keywords or their synonyms” initially reduced the number of papers to be considered. Thereupon, after prior discussion, approval and sample round, it was decided for each source based on title, keywords and the abstract about the consideration based on the stated exclusion criteria. Six researchers in two-person teams performed this step of the process. After removing duplicates with the software Citavi 6 and merging the results from all databases, 2507 publications remained. In a final reduction by applying quality criteria Footnote 2 , the number of publications decreased to 2365. No exclusions were applied with regard to subject-specific selections and rankings of the research outlets, since all themes as well as new, less established research domains had to be considered to achieve a comprehensive overview. In contrast to other systematic literature reviews, a larger number of publications was explicitly considered for analysis because of the topic of CPS itself. First, CPS emerge from the combination of several hardware and software components, rely on complex architectures and multilayered communication standards, and involve miscellaneous stakeholders (Khaitan & McCalley, 2014 ). Second, the domain of industrial value creation, with its core of production, is interlinked with many other domains, such as logistics and energy supply, which hold many application scenarios for CPS (Oks et al., 2017 ). Third and last, since the research field of CPS is highly topical, findings in specific niches can have a universal validity that is relevant to other research disciplines as well.
In step four (4), the selected contributions were analyzed to extract and synthesize the relevant data, however, in different procedures for the two objectives I and II.
Analysis and synthesis for objective I: First, a data extraction form, which had been adapted to the requirements of the research objective, was applied to outline the present state of the research on CPS. The data extraction form, which is displayed below (cf. Table 2 ), includes both standard information, such as publication type, name of journal, authors, etc., as well as a set of specific parameters, such as dimensions and application domains. In the next step, the sources were analyzed according to the data extraction form. The allocation was performed by the research consortium while ensuring objectivity as is typical for qualitative research by applying inter-coder reliability measures and discussion of results (Mayring, 2015 ). Concluding, the analysis results were processed quantitatively by summation and proportion calculation.
Analysis and synthesis for objective II: For the second objective, to elaborate a holistic categorization of CPS related and relevant topics in the context of Industry 4.0. Due to this large variety, we decided to create a categorization as opposed to a classification. According to Jacob’s ( 2004 ) definition, relevant topics, technologies, concepts, and procedures cannot always be strictly delimited or assigned, and overlapping areas may exist; a classification would require stricter delimitation, hierarchies, and representations (Jacob, 2004 ). Given the heterogeneity within the field of CPS in Industry 4.0, this did not appear to be expedient. A classification in the form of a taxonomy was equally unsuitable for these reasons (Nickerson et al., 2013 ). Ontologies, as a comparable approach, focus more on relationships between different phenomena or constructs (Wand & Weber, 2004 ), however our categorization does not aim to represent encompassing relationships between different items. To compose the categorization, the titles, abstracts, and keywords of the contributions were analyzed for terms of interest regarding CPS in the context of Industry 4.0. These included topics, technologies, concepts, and procedures. Methodically this was conducted by the performance of a structured qualitative content analysis. For this purpose, an inductive code creation approach, following Mayring ( 2015 ), was applied. The titles, abstracts, and keywords of all 2365 papers were included in the analysis; relevant passages or words were marked in Citavi 6. A total of 313 codes were created, often by matching words exactly, but also by marking sentences or paragraphs to include content or context. The qualitative content analysis was conducted by five researchers who regularly discussed the codes to ensure a common and consistent understanding and coding procedure. Results were compared and adjusted until a common consensus was reached. Inter-coder reliability measures were also used for quality assurance opposing potential subjectivity in this qualitative research procedure (Mayring, 2015 ). For the development of the categorization, 288 categories were derived from the 313 codes based on their respective properties. The reduction results from the clustering of similar codes or the omission of codes that were irrelevant or incompatible with the classification system. The 288 categories were arranged into a hierarchy with sub-categories consisting of 246 fields, 32 areas, and 10 sections using Citavi 6. Each field is a specific technology, concept, or procedure. An area is superordinate to this and can be separated, for example, by architecture, value creation process or organizational structure. Sections are overarching subjects into which the areas and fields are classified.
The utilization of software or AI applications was not an option for the development of the categorization either, since it was not a deductive procedure in which all category titles would already have been known, but an inductive one in which the categories first had to be developed from the literature.
In the fifth (5) and final step of the research process, the presentation of the findings is performed.
Presentation and interpretation of findings for objective I: The summed and proportional findings were then converted into bar graphs showing them into proportional, numerical form.
Presentation and interpretation of findings for objective II: The resulting categorization was transferred to a graphical representation for a clearer overview and a more descriptive presentation. In addition, an interactive web tool was created to make the data even more accessible (cf. Appendix Fig. 18 ). The underlying literature for each category is provided and linked, and the fields and areas can be marked and annotated. Furthermore, a search function has been implemented to enable the direct retrieval of terms and properties. The web tool features a selection of languages, including English and German.
Complementing the graphical representations, the findings in form of the state of research and the categorization are extensively described in Section 4 and discussed in Section 5 . The detailed process of the review steps is outlined in Fig. 2 .
In the following, the results of the literature review and analysis are presented in two subchapters. First, in Section 4.1 , a state of research on CPS is given, which is determined based on the characteristics of the analyzed publications. Second, in Section 4.2 , a categorization of CPS related and relevant topics in the context of Industry 4.0 is provided, which organizes them in 10 sections, 32 areas, and 246 fields in detail.
4.1 State of Research on Cyber-Physical Systems
The state of research on cyber-physical systems with regard to the distribution of publications by type is predominantly divided into contributions to edited volumes and conference proceedings (1499) and journal articles (810). The other types, including books, dissertations, and reports, on the other hand, account for a minor proportion of the total, as can be seen in Fig. 3 .
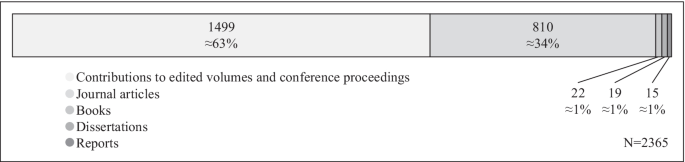
Distribution of publications by type
In terms of the distribution of the publications according to different scientific disciplines, three are the most prominent. These are computer science (856), computer engineering (808), and engineering (625). Business studies (36), mathematics and physics (26), and medicine (15) also deal with the subject matter, though there are a significantly lower number of publications in these disciplines.
Concerning the distribution of publications according to the disciplines specified in Figs. 4 and 5 shows that, in terms of the dimension of CPS introduced by Oks et al. ( 2017 ), the technical is notably the largest, with 2030 contributions. Given the 130 publications in the organizational and 44 in the socio-technical disciplines, it is evident that the topic of CPS has so far been examined primarily from technical and systems design perspectives, while organizational application and systems integration of humans has been of minor interest to date.
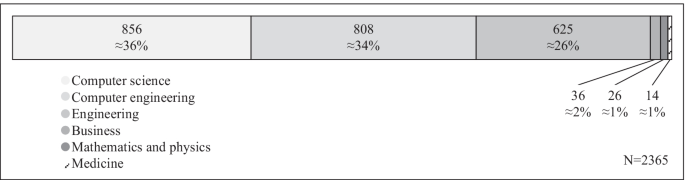
Distribution of publications by discipline
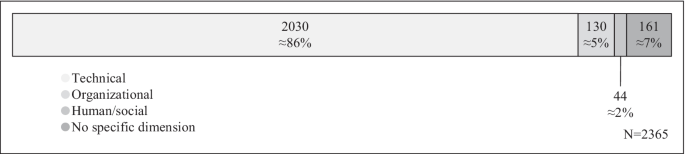
Distribution of publications by CPS dimension
When considering the distribution of publications that can be allocated to a specific application domain (an explicit application is described in relation to singularly one domain), as displayed in Fig. 6 , a greater variety becomes apparent. The four domains that account for more than 10% of all domain-specific publications (593) are mobility (135), manufacturing (109), energy (104), and healthcare (73). With a cumulative total of 313 contributions focusing on manufacturing, energy, logistics, robotics, safety and hazard defense, maintenance, smart products and coal, oil and gas industry, more than half of domain-specific contributions are relevant to industrial utilization.
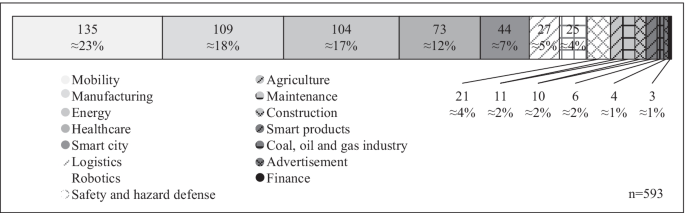
Distribution of publications by application domain
A precise examination of the 109 contributions of the application domain manufacturing shows the various utilization potentials of CPS in this context; specific topics and the distribution of the related literature are illustrated in Fig. 7 .
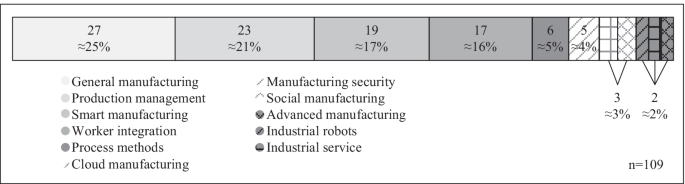
Distribution of publications in the application domain of manufacturing
4.2 Categorization of Cyber-Physical Systems Related and Relevant Topics in the Context of Industry 4.0
The categorization arranges the CPS related and relevant topics in the context of Industry 4.0 in a structured way. To this end, the findings from the literature are categorized into 10 sections. These include the characteristics and the overall context of industrial CPS as well as the potentials/opportunities and challenges/issues associated with their application. The requirements of industrial CPS, concepts and technologies by which they are accompanied, and their functionality as socio-technical systems are presented. Besides, the architecture of industrial CPS is outlined, and its influence on industrial value creation is characterized. Finally, the potentials of industrial CPS with respect to trans-organizational integration and alliance formation are addressed.
To enhance the readability of this sub-section, the categories are marked in italics. Exemplary underlying literature can be found in the Appendix in Table 4 . The table is sorted chronologically by occurrence of the categories in the text and contains sample citations of existing research on the respective topics.
The fundamental characteristics of CPS apply to the industrial application in the same way that they do to other domains, and are divided into general and the self-characteristics as presented in Fig. 8 . General characteristics include connectivity and modularity ; they highlight the comprehensive adaptability of industrial CPS, which can be designed to respond to varying situations and tasks by means of universal interfaces and modular construction. Real-time capability and traceability ensure that system adaptations can be both performed ad hoc and verifiable in this context. The high degree of autonomy of CPS is reflected in the self -characteristics, which describe the abilities of CPS to react autonomously to internal and external influences and control the system state by at least maintaining the system, if not optimizing it by anticipation without external intervention. CPS, therefore, have a high degree of resilience.
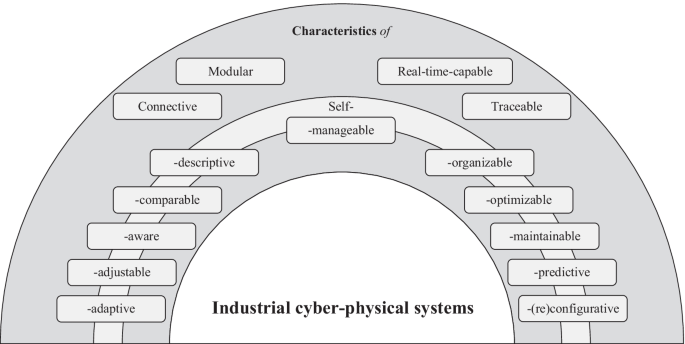
Characteristics of industrial CPS
The overall context in which the systems are situated is what characterizes them specifically as industrial CPS. In the literature, this is widely referred to as Industry 4.0 , as shown in Fig. 9 . Originating from the title of a German governmental funding initiative, Industry 4.0 has become a catchphrase for digitized and interconnected industrial value creation. The firm anchorage of industrial CPS in this context highlights the innovation potential inherent in and relevance of this concept.

Overall context of industrial CPS
The reason for this is apparent due to the potentials/opportunities that industrial CPS offer for value creation processes. From an organizational perspective, they cover both production engineering and management aspects while also providing benefits for the users of products and services. In general, processes can be further automated and autonomized , particularly to the previously discussed characteristics of industrial CPS. Through the continuous monitoring of physical and digital processes and the resulting homogenization, an improved system-wide level of information is achieved, which allows for increases in efficiency for both management activities and process execution. Among other things, this enables batch/lot size one production at costs approaching those of mass production, which means that market demand for product individualization can be anticipated. Due to universal interfaces and increasing location independence, as well as less hierarchical system architectures, industrial CPS can be set up in decentralized structures. Decentralization, in combination with an improved level of information within the overall system, also allows for complex event processing with increasing flexibility . For example, production and logistics processes can be coordinated with a significantly shorter planning horizon facilitated by lead time reductions . The sensor-aided improvement of the level of information regarding the condition of system components allows fault/failure scenarios to be detected earlier or even predictive, which leads to quality improvements for both production facilities and products. The continuous and extensive backflow of status information from smart products reinforces this trend even further. Among other things, the general rapid increase in the availability of data allows for the development of new, data-driven business models. Alongside this, potentials for market penetration strategies arise in the form of product portfolio enlargements and time-to-market reductions. An overview of the potentials/opportunities offered by industrial CPS is provided in Fig. 10 .
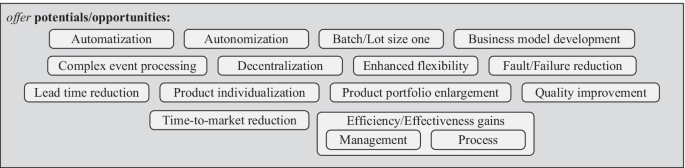
Potentials/opportunities of industrial CPS
In addition to the vast potentials/opportunities, the implementation of industrial CPS also brings challenges/issues with it, including increased system complexity resulting from far-reaching changes in system size and structure. In that way, the number of system components (technological, organizational, inter-organizational) can increase significantly due to the connection and interaction of formerly independent and self-sufficient systems as well as the dissolving of system boundaries towards ad-hoc systems of systems. Additionally, system architectures become more multilayered and overall system diversity increases. Alongside the changes in system architectures, industrial CPS also lead to an increase in complexity in the organizational landscape. Linear value creation processes dissolve towards holistic value networks which become increasingly inter-organizational. Also, further organizational units and stakeholder groups are involved with and affected by industrial CPS than before. This complexity is intensified by time-related factors, as, for example, production management becomes more real-time-critical and product life cycles are shortening. Advancing inter-organizational integration in particular can lead to reduced transparency concerning system structures, synchronization problems, and new challenges for risk and uncertainty management . Due to the integration of numerous system components, the continuous monitoring of conditions, and the thereof resulting data throughput rates and volumes, and the inherent real-time feedback loops between sensors and actuators in industrial CPS, communication problems, such as delays or jitter , pose a severe threat to system functionality. As with many digitization matters, the implementation of industrial CPS occasionally arouses concerns and reservations among employees due to notions that working conditions might change and certain professions might become obsolete. High implementation efforts are an additional challenge/issue. With regard to the acquisition of new production plants or the retrofitting of existing ones to integrate them into industrial CPS and the, in many cases, high capital requirement resulting from this, a conclusive cost-benefit calculation is often rather difficult in advance. Particularly in the case of industrial CPS which have trans-organizational structures or are used to facilitate hybrid value creation networks, juridical matters arise because responsibilities and liability issues in the event of system failures or manufacturing defects that lead to malfunctioning products cannot always be unequivocally clarified.
Two further challenges/issues that are discussed in detail in the literature on industrial CPS are safety and security . The field of safety is divided into hazard defense and state . In hazard defense, strategies are described to prevent system failures through environmental monitoring or, in the case of such failures, to facilitate emergency management . System state control, which attempts to detect fault/failure situations before they become safety issues, is closely related. While safety deals with the operational integrity of systems, i.e., the protection of people and the environment from physical damage, security addresses data and information protection within a system. In the context of industrial CPS, this concerns the defense against threats and vulnerabilities like (cyber-) attacks and the securing of privacy , e.g., via preventing data abuse . Additionally, practical measures are presented for attack detection , information flow control , and access and control message protection ( cryptography , digital signatures , and steganography ). In summary and relation, the challenges/issues associated with industrial CPS are illustrated in Fig. 11 .
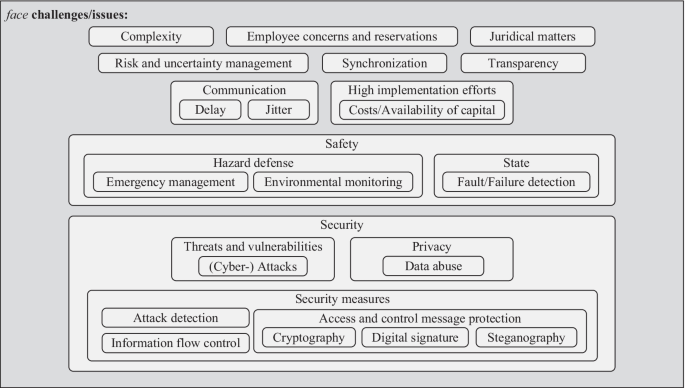
Challenges/issues of industrial CPS
Industrial CPS are subject to various requirements , as listed in Fig. 12 , that are necessary or advantageous for their functionality and operation. These include autonomy , which ensures the functioning of systems within the defined functional objectives, especially if they cannot be operated from outside in either a planned or unplanned capacity. To this end, systems must be designed in order to be context-aware and sensitive so that changes in state and status are not only sensed but can also be considered in the superordinate application context and operate according to predefined algorithms. This ensures a high degree of dependability and reliability with regard to system availability and behavior as well as the value creation processes based on it. This dependable and reliable system availability is particularly necessary because, especially in the context of large-scale interconnected systems, (sub-)system failures can have serious consequences, including the collapse of entire systems of systems. Availability is also of utmost importance whenever safety-relevant processes are monitored and controlled by the system. In the context of maintaining system functionality under adverse conditions and in critical situations, robustness and resilience are also essential for industrial CPS. To a certain extent, the systems should be able to cope with environmental changes; their configuration should be able to robustly sustain these conditions. If the environmental changes are so severe that they cannot be handled by robustness, the systems should be so resilient that they adjust and adapt their configurations accordingly. The system state must be observable , with a high degree of reliability, and the information output on the state and control processes must be trustworthy so that fact-based decisions by administrators are possible at all times. In this context of system monitoring and control, it is also vital to have the most accurate predictability of expected system behavior in different situations so that the controllability of the system is given, despite its complexity and high degree of automation and autonomy.

Requirements of industrial CPS
In order to react to changes and new requirements in industrial CPS-based value creation processes, such as short-term capacity fluctuations or long-term market, production or product-related trends, it is a further requirement of industrial CPS that they are scalable , which can be executed briefly. Furthermore, since, as previously mentioned, value creation activities are becoming increasingly interactive and networked both intra- and inter-organizationally, the interoperability of individual industrial CPS is also of great interest.
All the requirements mentioned above should be met under the premise of sustainability in order to achieve efficiency and effectiveness in economic, ecological, and social dimensions.
In light of the far-reaching and holistic digitization of industrial value creation, a wide range of complementing concepts and technologies are being applied. In this, industrial CPS often serve as a linking element that systematically integrates these concepts and technologies in a goal-oriented and application-specific manner. Big data analyses are one of these concepts. Based on the widespread utilization of sensor technology in production and in products as such, industrial CPS often generate extensive data (5 Vs: volume, velocity, variety, value, veracity) , which can be transferred by algorithm-based analyses such as pattern detection/recognition in smart data for general optimization purposes, as well as data-driven services and business models ( data as a service ). As often distributed and decentralized systems, industrial CPS use cloud , edge and ubiquitous computing to perform data processing and system control detached from the conventional automation pyramid. In many application scenarios of industrial CPS, the use of artificial intelligence (AI) , e.g., as a foundation for the self-characteristics described previously, is suitable. Conventional methods to this end include reasoning or machine learning . As previously indicated, industrial CPS can be connected ad hoc to systems of systems according to context and task. To ensure integrity in the exchange of data and resources, distributed ledger technologies , such as blockchain , offer an adequate solution. Another concept that is compatible with industrial CPS is additive manufacturing . On the one hand, topics as resource efficiency, availability of spare parts, rapid prototyping, etc. can be addressed via this concept. On the other hand, production processes itself can apply technologies such as 3D printing.
Another concept that goes hand in hand with the digitization of industrial processes is work 4.0/future of work , which describes the elaboration of innovative working methods that are either possible or necessary due to technological changes. This may concern the general conditions of work in the industrial sector, which can even allow execution of work independent of time and location and in virtual teams/crowd working . Additionally, the introduction of industrial CPS is often accompanied by extensive changes in job requirements and professional training. Thus, the need for interdisciplinary competencies arises due to increasing system complexity, which is also reflected in a progressive linking and overlapping of disciplines relevant to value creation. Furthermore, the increasing automation associated with industrial CPS in particular leads to a reduction of low-wage-sector and unskilled jobs demand . Role changes become, therefore, necessary, which often require extensive training measures.
The spectrum of concepts and technologies that complement industrial CPS is shown Fig. 13 .
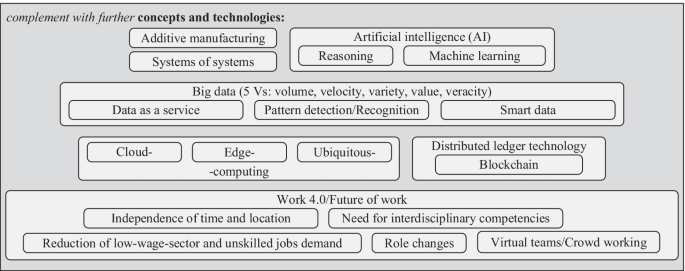
Complementing concepts and technologies to industrial CPS
In addition to the primary technical consideration of industrial CPS, the literature also examines the integration of humans in the form of socio-technical systems . In the field of production-supporting activities, this affects work execution . Due to increasing availability of information and new forms of HCI, information can be provided through various decision support systems , e.g., by means of action guidelines in maintenance. In addition, media discontinuities are being reduced due to increasing document/content digitization . The topic of knowledge in relation to industrial CPS is also covered by the literature. Additionally, due to new methods of system-integrated education and qualification , the integration of implicit knowledge can be achieved, making previously person-bound knowledge increasingly available to a wider circle of personnel (e.g., by the creation of action guidelines for machine repairs and further maintenance activities). The socio-technical systems integration of industrial CPS is presented in Fig. 14 .
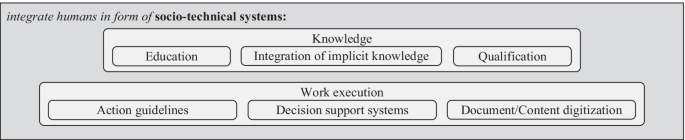
Industrial CPS as socio-technical systems
CPS have a common architecture with individual specifications depending on the application domain. The architecture of industrial CPS, which is described hereafter, serves as the underlying principle and scheme for the definition of concrete system features and configurations from design alternatives, depending on functional and non-functional requirements, and for the selection of suitable system components. Thereby, industrial CPS are allocated to the superordinate domain of information technology (IT) , respectively, information and communication technology (ICT) . From this domain, industrial CPS combine technologies and concepts of the (industrial) internet of things ((I)IoT) or web of things (WoT) , which can be partitioned into a cyber sphere and a physical sphere according to the underlying logic of CPS. Software architecture and the data processing of industrial CPS are situated within the cyber sphere while hardware architecture and human-computer interaction (HCI) exist within the physical sphere. Network architecture serves as a connective link between the two spheres.
In the area software architecture, industrial CPS literature covers the following topics: Adequate operating systems for the respective system components are analyzed, the design of these systems from a programming standpoint with the subfields algorithms and programming languages as well as software agents with further subfields mobile agents and multi-agents . Further topics are sufficient middleware in the form of data distribution services (DDS) and workflow engines . Beyond that, concepts are presented that allow dynamic software updating (DSU) for CPS.
Concerning data in the context of industrial CPS, the following focal points receive particular attention in the literature. First, the data acquisition by sensors is discussed. This data can then be aggregated with existing data or fused with data from external sources. The resulting data sets are analyzed and evaluated by processing . The literature also examines how data traffic , in the form of dissemination , exchange , and transmission , can be performed both within a system but in exchange with other systems. With regard to the qualitative aspects of data, their quality and reliability are considered. Further topics are data recovery and the concept of supervisory control and data acquisition (SCADA) .
The domain of hardware architecture contains the components that physically constitute industrial CPS. These are mainly embedded systems that are extended by sensors that continuously record physical environmental conditions. The resulting data is processed by processors and field programmable gate arrays (FPGA) . The subsequent operation of actuators , which, in turn, affect the physical environment, is carried out by controllers . Identifiers ensure the individual identifiability of each system component. Furthermore, passive components can be integrated into industrial CPS via radio-frequency identification (RFID) technologies, such as near field communication (NFC) . In addition, the field of robotics is receiving a considerable amount of attention in the context of industrial CPS.
In the area of HCI, the integration of humans in industrial CPS is addressed. Against this backdrop, the literature deals, among other things, with the support of humans in the performance of physical work. E.g., cobots or collaborative robotics are used to enable humans and machines to carry out tasks jointly in order to integrate the respective superior skills optimally. Technology can also be worn on the human body as wearables ; these wearables can provide physical support, as seen with (powered) exoskeletons , or can be used to provide information in the form of augmented reality (AR) and virtual reality (VR) devices. In the field of user interfaces of industrial CPS, the literature deals with different forms of human-machine-interfaces (HMI) and graphical user interfaces (GUI) , which can be operated via gesture control or voice control . In the overall context of HCI, unrestrained human-machine collaboration combined with the highest standards of workplace safety is of particular importance.
The network architecture of industrial CPS draws on a variety of established technologies and concepts and adapts them to the specifics inherent in industrial CPS as needed. In general, the network architecture provides the link between the cyber sphere and the physical sphere and enables the transfer of signals and data. The literature on industrial CPS deals extensively with the subject of how network architectures can be designed in these systems and what requirements they have to meet, and a great deal of attention is paid to the networks themselves. Different types of networks and their suitability for a variety of applications due to differences in transmission power, range, and data transfer rates are considered. The first worth mentioning are sensor networks (SN) , which can be divided into mobile actuator/sensor networks (MASN) , wireless sensor networks (WSN) and wireless sensor and actuator networks (WSAN) . These network types are used to link sensors and actuators and to ensure the transfer of measured environmental values and coordinated actuator behavior. Controller area networks (CAN) are used as a serial bus system and are particularly useful in safety-relevant areas. For short-distance applications, wireless personal area networks (WPAN) , such as Bluetooth or wireless personal body networks (WPBN) , offer the advantage that interference with other networks can be reduced and that there is a low power requirement for transmitting units. For large-scale coverage, the pervasive wireless local area networks (WLAN) are used. For the integration of geographically remote system units, wide area networks (WAN) are used in form of long range wide area networks (LoRaWAN) and low power wide area networks (LPWAN) , which offer high energy efficiency. Cellular networks with LTE and 5G standards are also used for interconnecting widely separated system units, especially if those are mobile. Depending on the type of network and application, different protocols are used to determine the communication syntax. In the context of industrial CPS, IP , MAC , message queue telemetry transport (MQTT) , TCP , and TCP/IP are mentioned in the literature. Dynamic spectrum access for the optimization of frequency spectra of connections and routing for the coordination of message streams are also being considered, as they can help to handle increased data volumes in a system-efficient manner. The subject of plant networking is also receiving a large amount of interest; therefore, plug-and-produce and (standardized) interfaces that enable the interoperability of diverse production plants with minimal setup effort are of great importance in the process of industrial CPS development. In this context of machine-to-machine communication (M2M) , the OPC Unified Architecture (OPC UA) provides a platform-independent, service-oriented architecture (SOA) for the exchange of machine data.
Figure 15 provides a holistic visualization of the architecture underlying industrial CPS.
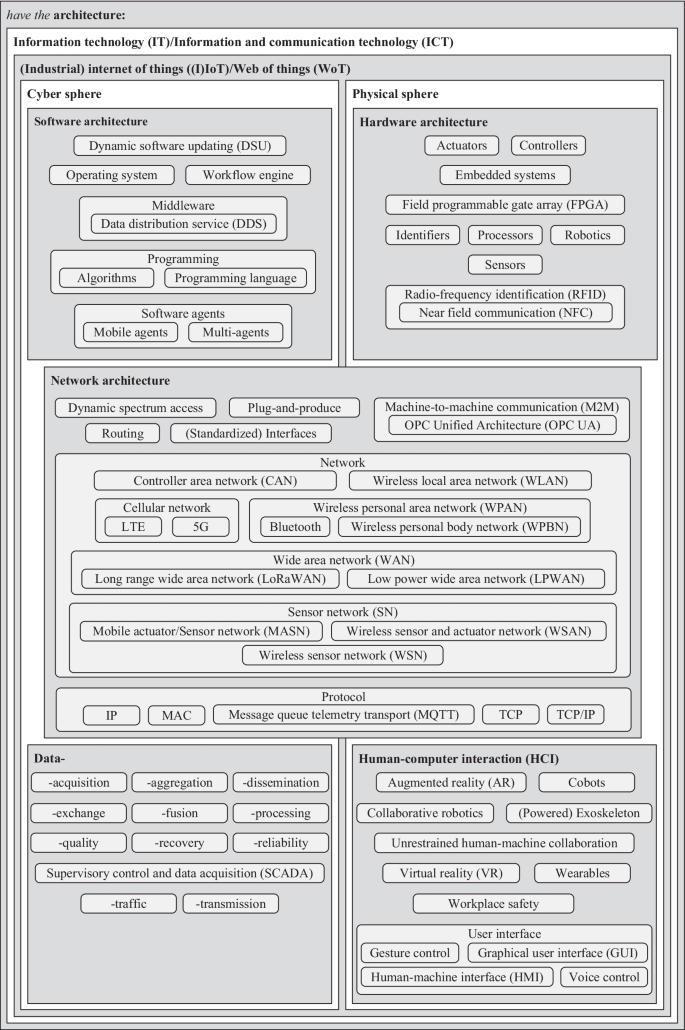
Architecture of industrial CPS
Within the realization of the already described potentials through the application of CPS they transform industrial value creation . This applies to all sequential stages and organizational levels in value chains and value networks; they can be broken down into the pre-production stage , production stage , and product in use stage .
Already in the pre-production stage, the monitoring of raw, auxiliary, and operating materials, as well as of supplier parts and construction groups intended for later production begins. Through the continuous collection and consolidation of data on smart (raw)materials/components , information regarding condition , processing , and transport becomes available in form of digital twins, already in the earliest stages of the value chain and is manipulation-proof passed on across organizational boundaries. This applies both to newly extracted raw materials and to reprocessed and renewed materials and components within the scope of lifecycle management .
In the production stage, the transformation of industrial value creation is discussed in the context of the holistic concepts, digital factory , smart factory , and smart manufacturing . Manufacturing systems that use CPS in their processes are referred to as cyber-physical production systems (CPPS) . In the literature, CPPS are examined from different focal points; specifically, production system development , production execution , and production support can be clustered.
Production system development describes all activities and procedures on the way to a CPS-based production system. In the subarea design , the planning and development of the production processes takes place. Within the design space exploration , the options and alternatives for the future system configuration are discussed and structured. The subsequent IT design process can be carried out with different system level design methodologies . With component-based development, the aim is to design standardized components that can be used several times in different applications of modular systems with the same or related requirements, minimizing the amount of effort required. Contract-based development is particularly important when a large number of modules from various providers are combined into a single system. Hereby, the definition of formal contracts for the use of standardized interfaces ensures compatibility. Model-based design and development is used in particular when the intended system has a high degree of complexity. By using predefined models with advanced functional characteristics, systems can be simulated and tested in detail, even before physical engineering. Due to the previously discussed challenges associated with CPS, such as complexity, method-combining procedures are feasible. If these are participative, co-creative, or open, the term co-design is used.
Simulation is used to determine the behavior and performance, as well as the safety and security, of CPPS before they are constructed and launched. In this process, modeling is used to create a physical or digital representation of the system or its individual parts. Deliberate reductions and omissions lead to an individual abstraction of the original. Depending on the application purpose, models can take the form of formal descriptions, physical objects or computer-based virtualizations. In co-simulation , different simulation tools that use different models, each of which represents subsystems, are interconnected to enable a holistic system simulation. This procedure is particularly suitable for CPPS since components and systems from different (technical) disciplines are combined in this process. Due to the ongoing digitization and increasing automation of production through the establishment of CPS, production control continues to receive a great deal of attention in the literature. For programmable logic controllers (PLC), which are used to control systems, robots, and actuators, hardware-in-the-loop simulation is applied to make them operational before they are directly connected to the hardware to be controlled.
For the subsequent engineering of CPPS, two initial situations can be distinguished: Greenfield , when a completely new production system is designed, and brownfield/retrofit , when an existing production system is upgraded to a CPPS. In the literature, the following activities are described for both cases with the specifics that the respective initial situation entails. In requirements engineering , the first step is to define the characteristics and general parameters that the system should fulfill. One of the factors that affect the requirements for CPPS is product line engineering , which, therefore, should be considered in close connection with production line engineering. Depending on the selection of the hardware to be utilized, software engineering should be adjusted accordingly. For the combination and iterative adaptation of CPPS hardware and software, it is advantageous to prototype them before integrating them into a consistent CPPS.
In the production execution stage, the plants are operated. Manufacturing is an essential part of this. In this area, the literature deals with the effects of implementing industrial CPS on production management with the subfields process control and process management . It is also described how the application of industrial CPS enables advanced manufacturing , which refers to the execution of particularly complex production processes for the manufacturing of equally complex products, both of which are only possible through the use of digital technologies and concepts. Moreover, cloud manufacturing , which describes a less organization- and location-bound value creation through flexible, virtual production networks, benefits from the utilization of industrial CPS. Another topic that receives attention in the context of CPS-based manufacturing is industrial services . This includes service composition , which is concerned with the arrangement and orchestration of service bundles, often from various providers, that are combined to form integrated service systems. One service to be highlighted in this field is maintenance . Due to the many degrees of freedom regarding potential events and their resolution, processes related to maintenance are difficult to optimize. However, based on live sensor data and results of big data analytics, condition-based and predictive maintenance procedures can increasingly be implemented in CPPS with great optimization potential.
Overall, i.e., beyond the maintenance application, industrial CPS, with their sensors and actuators, offer vast potentials for reforming monitoring/control in production. Condition monitoring enables a meaningful and comprehensive status overview to be obtained in real-time for all equipped system components, including both production infrastructure and production parts. Event processing is focused on the continuity and real-time capability through the application of industrial CPS. This enables a reliable event-triggered control , in which events are reacted to mostly automatically with adequate measures when they occur. To prevent adverse events, predictive control uses the ability to recognize trends and patterns in data and take countermeasures before critical values are reached. Also, for the field of fuzzy control , industrial CPS offer implications for the definition of control variables as well as for the already known SCADA.
In addition to the usage of monitoring/control, the exorbitant increase in status information, and data sets generated by industrial CPS, sensor technology can also be exploited for analysis . Testing is carried out, among other activities in this area, all of which can be largely automated by model-based testing with optimized testbed conditions. These test activities can examine hardware and software as well as processes in production plants and production output. Additionally, the literature deals with validation and verification as a means for requirements fulfilment with the subfields model checking and runtime verification . In supplement to this, eigen analysis is explicitly mentioned.
The third and last subject area concerning CPPS is constituted by production support, including the area logistics . Here, the whole context of material handling within an organization but also beyond its borders is examined. Especially for warehouse systems , industrial CPS offer far-reaching application potentials, which allow for optimizations in warehouse volumes and processes through increased transparency. In addition to warehousing, internal logistics also benefit in the form of automated guided vehicles (AGV) , which ensure highly automated, event-based, and system-integrated flows of materials into production. With the establishment of intelligent transportation systems (ITS) , industrial CPS are also applied in logistics between geographically dispersed production sites of an organization or different organizations in a value chain, which results in supply chain optimization , including the delivery of final products to vendors and end-users.
Another area involved in production support is the smart grid integration of plants. The integration of industrial CPS in the power supply of production facilities affects the general energy efficiency of these facilities by better incorporating energy requirements, availability, and costs into production planning and execution. In addition, methods such as energy harvesting from physical processes of industrial CPS and battery management in less grid-dependent production processes offer opportunities to improve energy balances.
In the third stage, the product in use stage, industrial CPS are used to feed back relevant information regarding product performance into the CPPS. In particular, smart products , which, due to their integrated sensor and actuator technology, enable information and data generation similar to that of the production systems that manufacture them, allow monitoring throughout the entire product life cycle (product usage data) . This continuously collected information regarding the condition and usage of the products is a highly valuable source for the evaluation and possible adjustment of product planning and production execution parameters.
By the holistic approach of lifecycle management , recycling or downcycling is applied at the end of product use, in the course of which the data collected over the entire product lifecycle in the form of a digital twin is, at best, reintroduced into the reprocessing or renewal in the new pre-production stage.
Apart from the subjects that can be clearly assigned to the individual stages, there are also those that are relevant across company/organization boundaries throughout the entire value chain . These include the digital twin , which combines the industrial CPS-based information of the entire lifecycles of both production plants and products. The integrated supply chain , which merges inter-organizational logistics processes due to increased transparency from industrial CPS, is another example of an activity that takes place across company/organization boundaries throughout the entire value chain. In this, procedures such as ad-hoc connectivity increase the interoperability of production systems, facilities, and services, which expands the potential realization of industrial CPS. In this context, the increasing establishment of platform ecosystems , which enable the linking of heterogeneous services and hardware to industrial CPS in the form of systems of systems, is particularly noteworthy.
A general overview of how CPS transform industrial value creation is shown in Fig. 16 .
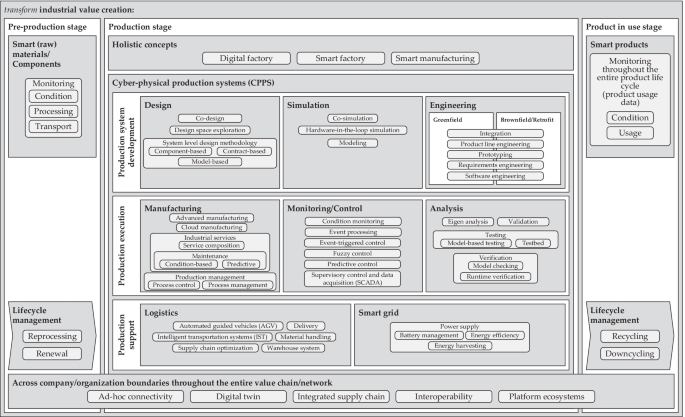
Value creation based on industrial CPS
The application potentials of industrial CPS across company/organizational boundaries offer opportunities for horizontal and vertical integration/operational and strategic alliances . The horizontal integration can either be performed within a company/organization between production sites, departments, manufacturing sectors, etc., which previously operated largely independently, or along the value chain/within the value network across organizational boundaries, both upstream and downstream.
The cooperation between companies/organizations or their organizational units can be performed at the operational or strategic level (vertical integration) . While integration at the operational level is mostly about technical and procedural cooperation, which coordinate the execution of value creation activities, sometimes automated, ad-hoc and for short periods of time, those at the strategic level represent rather long-term alliances between two or more partners, which closely interconnect their industrial CPS and related processes.
The schematics of these integrations and alliances are shown in Fig. 17 .
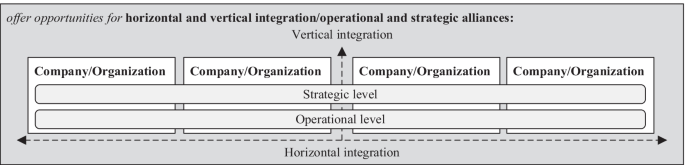
Organizational integration and strategic alliances based on industrial CPS
5 Discussion and Outlook
In the final Section of this study, the results are discussed, contributions to extant research, highlighted and limitations pointed out. Finally, a concluding and prospective outlook is given.
5.1 Contributions
The contribution of this study is twofold. First, it provides insight into the existing literature on CPS by organizing 2365 publications according to discipline, CPS dimension, and application field. Second, the resulting data set was analyzed and transferred into a categorization of CPS related and relevant topics in the context of Industry 4.0. Thereby, this study contributes by complementing the existing topic-specific reviews and categorizations. In addition to the general category formation, the industrial CPS architecture by incorporating technological, data-driven, and socio-technical views as well as the overview of value creation on the basis of this concept, are particularly noteworthy. Thus, our results enhance the CPS concept map of Asare et al. ( 2012 ), whose overview comes the closest to the scope of this work, significantly and set the focus on industrial CPS.
Both contributions thus provide new knowledge to the research on CPS in the context of Industry 4.0. The state of research first provides insights into the distributions of publications by discipline (cf. Figs. 4 and 5 ). Most originate from computer science, computer engineering, and engineering, meaning that the subject area has so far been considered from a highly technical perspective. The business, value creation, and information systems perspectives have therefore been somewhat neglected, which implicates great potential for future research in these areas. It is not surprising that research was initially conducted from a technical perspective, as technological developments for specific problems are developed first and then other application scenarios or generalization potentials are considered. At this point, we are at a frontier of information systems research, which can be more involved here to contextualize the technical developments in a larger context, e.g., business, socio-technical, development with the user/stakeholder, and value creation. This is also reflected in the state of research on CPS dimensions (cf. Fig. 5 ). So far, there have been primarily technical studies and only a few from the organizational and human/social disciplines.
The application domains for CPS are wide ranging (cf. Fig. 6 ). It can therefore be confirmed that CPS are a general purpose technology. Nevertheless, it is noteworthy that many applications are in the domain of smart manufacturing. Here, a distinction can be made between discrete and process manufacturing (Ning et al., 2017 ; Zhang et al., 2020 ). There have been and are several public funding programs and initiatives in these areas due to the feasible potential. This can also be linked to fact that the application of CPS is easier to realize in an organization (on meso level) than in an overarching system.
Furthermore, the manufacturing domain was examined in more detail (cf. Fig. 7 ). There, as well, the application fields for CPS are wide ranging, and there are a large number of applications in industry in general. Related work by Monostori et al. ( 2016 ) also highlights the relevance of CPS in manufacturing. The literature thus suggests that we can assume far-reaching changes that qualify for an industrial revolution.
In addition to the state of research, the categorization of industrial CPS also provides several new insights for research on CPS in the context of Industry 4.0. As compared to existing taxonomies, reviews, and categorizations (cf. Section 2 ), our study is much more comprehensive and provides a detailed categorization and analysis of industrial CPS. We arrange our findings into 10 sections, the key conclusions of which are summarized below.
With regard to the characteristics of industrial CPS, it is apparent that CPS are a further development of systems that are oriented toward autonomous operation and independent action (cf. Fig. 8 ). This aspect is supported by Berger et al. ( 2021 ), who examined CPPS from a self-organizing systems’ perspective. Our results also show that CPS are clearly an enabler for Industry 4.0 . Figure 10 shows that CPS have far-reaching potential that is relevant for industry, consumers, and the common good, in terms of sustainability, for example. There is also a large number of challenges to be overcome, particularly in the areas of safety and security, which is not surprising given the increased openness and interaction of entities and systems (cf. Fig. 11 ). Our results also contribute to an extension of the works of Liu et al. ( 2017 ) and Hofer ( 2018 ). For CPS to function properly, numerous requirements must be fulfilled (cf. Fig. 12 ). This point was also taken up by other authors, e.g., Asare et al. ( 2012 ), who also mentioned a few requirements in their concept map. In addition, CPS are a concept that can be seen as a hub of various complementary concepts and technologies of the digital age. CPS can only unfold their potential through interaction with these concepts and technologies (cf. Fig. 13 ). The relevance of CPS to complementary concepts in the context of digitalized industrial value creation has also been stated by (Kim, 2017 ). As shown in Fig. 14 , CPS integrate humans in the form of socio-technical systems that require a user- and stakeholder-centric consideration. The architecture of CPS can be characterized as highly complex, which is also supported by other authors who described CPS architectures (Hofer, 2018 ; Lee et al., 2015 ; Pivoto et al., 2021 ). The architecture suggested by this study integrates software, hardware, network, data processing, and HCI components (cf. Fig. 15 ). CPS also offer application potential for the entire industrial value creation network (cf. Fig. 16 ). The interconnectivity and general network character of CPS generates potential for operational and strategic alliances with other organizations and entities (cf. Fig. 17 ).
The categorization of CPS related and relevant topics in the context of Industry 4.0 described above offers a variety of possible applications in practice. First, it provides an overview of the existing state of concepts and technologies in the area of industrial CPS. Thus, the categorization also serves as means of analyzing potential by documenting and evaluating existing technologies and systems and performing compatibility checks. In addition, it can be used for strategy development. Thus, organization-specific potentials and limitations regarding digitization and Industry 4.0 can be narrowed down. It can also help develop retrofit or greenfield digitization strategies. Furthermore, the categorization enables CPS design by facilitating the selection of system-relevant technologies and concepts as well as the definition of interfaces and standards. Finally, it can be used in education and training, for example through user-centered formats for knowledge transfer, in the context of system implementations, and digitization activities.
The architecture in Fig. 15 and the value creation based on industrial CPS in Fig. 16 go far beyond existing categorizations and can also be used as a design tool; a fully functional web tool was developed to that end. The web tool enables users to better comprehend industrial CPS and capture their architecture and use across the entire industrial value chain. Specifically, the tool can be applied within established methods or in the form of a canvas in workshops.
5.2 Future Work
The planned continuation of the research work is threefold: First, the state of knowledge and the categorization shall be updated by periodic repetitions of the review. On the one hand, this will allow new research foci, concepts, technologies, etc. to be observed in order to integrate them into the existing findings. On the other hand, trends, changes in thematic emphases, etc. can be identified over time, which allows statements to be made about the development of the research landscape and the implementation and application state of CPS in the context of Industry 4.0.
In addition to the scientific literature, funding projects and best practices from industry related to industrial CPS will be systematically analyzed. These can then underpin the individual topics in the categorization, particularly in the artifact Industry 4.0 Compendium , which is a functional web tool of the categorization (cf. Appendix Fig. 18 ), as a supplement to the scientific literature, and increase the added value for users from practice. Thus, this extension contributes to the managerial contribution. The respective systematic search and analysis process of funding projects and best practices has already started.
Furthermore, the extensive literature dataset provides an opportunity to undertake deductive—including software-assisted—analyses in order to elaborate quantitative measures and weighted links of the identified categories. In this way, the present qualitative findings of the study could be supplemented by quantitative ones, which would facilitate a more comprehensive interpretation.
5.3 Limitations
The limitations of our study are primarily determined by the subject area and the methodology. With industrial CPS, we are exploring a still relatively young and dynamic field of research. As a result, findings are constantly increasing as new developments and studies are being undertaken and published. Thus, the data presented here is only a snapshot representing the state of research and categorization of industrial CPS at one point in time. New findings and developments may have emerged in the meantime that would affect the results of this study.
The systematic literature review is influenced by the selection of literature databases and search engines. We aimed to make a selection that is as comprehensive as possible, including different disciplines and leading publishers. Search strings also influence the results of literature searches; we therefore attempted to search for publications on CPS as broadly and comprehensively as possible by using a wide variety of spellings.
The third limitation of our study results from the exclusive consideration of title, keywords, and abstract for the structured qualitative content analysis. In our opinion, this has no impact on the categorization, but it cannot be guaranteed that our approach did not necessarily exploit the complete amount of information.
5.4 Outlook
The final goal of our study is to provide an outlook on how research on and the use of industrial CPS can be further advanced. As our results show, interdisciplinary approaches are advisable or even necessary for this purpose due to the interwoven and wide-ranging characteristics of the topic. Therefore, the aim of research should be to break down existing silos and collaborate with related disciplines to develop methods and concepts that bring the topic of industrial CPS further into application. Specifically, information systems research has the potential to play a central role. Due to its interdisciplinary approaches, information systems research should act as a link between the disciplines and the entire body of knowledge, especially regarding system design, development, and implementation. This leads to the implication for information systems research to further contribute to the implementation and application of Industry 4.0 by transferring and adapting specific insular knowledge about CPS to value creating applications.
Since the introduction of the concept of CPS, there have been many vision papers and agendas on the subject. Therefore, we suggest an interim assessment and investigation of the current state of knowledge and implementation to determine the further implementation capabilities of scenarios foreseen in agendas and vision papers. Moreover, it is advisable to aim and research towards analogy building in order to enable the transfer of results and knowledge from other disciplines regarding CPS to be applied in industry, but also to make the extensive knowledge from the industrial domain available to other disciplines.
We believe that our categorization of industrial CPS can enhance the knowledge transfer into practice. It can particularly contribute to the design and development of new industrial CPS applications. Through the Industry 4.0 Compendium web tool (cf. Appendix Fig. 18 ), the results of this study have been made available to the research and practice community in an application-oriented manner (Oks & Jalowski, 2020 ); it offers search, selection, and note-taking functions. These features help reduce the plethora and complexity of information, making it more accessible and thus fostering CPS-oriented activities in the context of Industry 4.0.
Publication type outside of a journal article, monograph, contribution to an edited book, contribution to conference or workshop proceedings, dissertation, or university report.
Publications that are a description, preface, or foreword of a workshop or conference, none of the keywords or their synonyms existing in the abstract, keywords or full text of the publication, or the main topic of the publication not being in the context of CPS.
Alur, R. (2015). Principles of cyber-physical systems . The MIT Press
Asare, P., Bakirtzis, G., Bernard, R., Broman, D., Lee, E. A., Prinsloo, G., & Sunder, S. S. (2012). Cyber-Physical Systems - a Concept Map . http://CyberPhysicalSystems.org
Berger, S., Häckel, B., & Häfner, L. (2021). Organizing self-organizing systems: A terminology, taxonomy, and reference model for entities in cyber-physical production systems. Information Systems Frontiers, 23 (2), 391–414. https://doi.org/10.1007/s10796-019-09952-8 .
Article Google Scholar
Biesinger, F., Meike, D., Kraß, B., & Weyrich, M. (2019). A digital twin for production planning based on cyber-physical systems: A case study for a cyber-physical system-based creation of a digital twin. Procedia CIRP, 79, 355–360. https://doi.org/10.1016/j.procir.2019.02.087
Bresnahan, T. (2010). General purpose technologies. In K. J. Arrow & M. D. Intriligator (Eds.), Handbook of the economics of innovation (Vol. 2, pp. 761–791). Elsevier. https://doi.org/10.1016/S0169-7218(10)02002-2
Burns, T., Cosgrove, J., & Doyle, F. (2019). A Review of Interoperability Standards for Industry 4.0. Procedia Manufacturing, 38, 646–653. https://doi.org/10.1016/j.promfg.2020.01.083 .
Calinescu, R., Camara, J., & Paterson, C. (2019). Socio-cyber-physical systems: Models, opportunities, open challenges. In 5th IEEE/ACM International Workshop on Software Engineering for Smart Cyber-Physical Systems (SEsCPS) (pp. 2–6). IEEE. https://doi.org/10.1109/SEsCPS.2019.00008
Cardin, O. (2019). Classification of cyber-physical production systems applications: Proposition of an analysis framework. Computers in Industry, 104, 11–21. https://doi.org/10.1016/j.compind.2018.10.002 .
Chaâri, R., Ellouze, F., Koubâa, A., Qureshi, B., Pereira, N., Youssef, H., & Tovar, E. (2016). Cyber-physical systems clouds: A survey. Computer Networks, 108, 260–278. https://doi.org/10.1016/j.comnet.2016.08.017 .
Chen, H. (2017). Applications of cyber-physical system: a literature review. Journal of Industrial Integration and Management, 02 (03), 1750012–1750028. https://doi.org/10.1142/S2424862217500129 .
Chen, H. (2017). Theoretical foundations for cyber-physical systems: A literature review. Journal of Industrial Integration and Management, 2 (3), 1750013–1750027. https://doi.org/10.1142/S2424862217500130 .
Colledani, M., Coupek, D., Verl, A., Aichele, J., & Yemane, A. (2018). A cyber-physical system for quality-oriented assembly of automotive electric motors. CIRP Journal of Manufacturing Science and Technology, 20, 12–22. https://doi.org/10.1016/j.cirpj.2017.09.001 .
Colombo, A. W., Karnouskos, S., Kaynak, O., Shi, Y., & Yin, S. (2017). Industrial cyberphysical systems: A backbone of the fourth industrial revolution. IEEE Industrial Electronics Magazine, 11 (1), 6–16. https://doi.org/10.1109/MIE.2017.2648857 .
Dafflon, B., Moalla, N., & Ouzrout, Y. (2021). The challenges, approaches, and used techniques of CPS for manufacturing in Industry 4.0: a literature review. The International Journal of Advanced Manufacturing Technology, 113 (7–8), 2395–2412. https://doi.org/10.1007/s00170-020-06572-4 .
Denyer, D., & Tranfield, D. (2009). Producing a systematic review. In D. A. Buchanan, & A. Bryman (Eds.), The SAGE Handbook of Organizational Research Methods (pp. 671–689). SAGE
Geisberger, E., & Broy, M. (2015). Living in a networked world: Integrated research agenda, cyber-physical systems (AgendaCPS) (Acatech Study)
Gil, M., Albert, M., Fons, J., & Pelechano, V. (2019). Designing human-in-the-loop autonomous cyber-physical systems. International Journal of Human-Computer Studies, 130, 21–39. https://doi.org/10.1016/j.ijhcs.2019.04.006 .
Gürdür, D., & Asplund, F. (2018). A systematic review to merge discourses: Interoperability, integration and cyber-physical systems. Journal of Industrial Information Integration, 9, 14–23. https://doi.org/10.1016/j.jii.2017.12.001 .
Gürdür, D., El-Khoury, J., Seceleanu, T., & Lednicki, L. (2016). Making interoperability visible: Data visualization of cyber-physical systems development tool chains. Journal of Industrial Information Integration, 4, 26–34. https://doi.org/10.1016/j.jii.2016.09.002 .
Gürdür Broo, D., Boman, U., & Törngren, M. (2021). Cyber-physical systems research and education in 2030: Scenarios and strategies. Journal of Industrial Information Integration, 21, 100192. https://doi.org/10.1016/j.jii.2020.100192 .
Haque, S. A., Aziz, S. M., & Rahman, M. (2014). Review of cyber-physical system in healthcare. International Journal of Distributed Sensor Networks, 2014, 1–20. https://doi.org/10.1155/2014/217415 .
Hehenberger, P., Vogel-Heuser, B., Bradley, D., Eynard, B., Tomiyama, T., & Achiche, S. (2016). Design, modelling, simulation, and integration of cyber physical systems: Methods and applications. Computers in Industry, 82, 273–289. https://doi.org/10.1016/j.compind.2016.05.006 .
Hermann, M., Pentek, T., & Otto, B. (2016). Design principles for Industrie 4.0 Scenarios. In 49th Hawaii International Conference on System Sciences (HICSS) (pp. 3928–3937). IEEE. https://doi.org/10.1109/HICSS.2016.488
Hofer, F. (2018). Architecture, technologies and challenges for cyber-physical systems in industry 4.0: A systematic mapping study. In M. Oivo, D. Méndez, & A. Mockus (Eds.), Proceedings of the 12th ACM/IEEE International Symposium on Empirical Software Engineering and Measurement (pp. 1–10). ACM. https://doi.org/10.1145/3239235.3239242
Huang, G., Chen, J., & Khojasteh, Y. (2021). A cyber-physical system deployment based on pull strategies for one-of-a-kind production with limited resources. Journal of Intelligent Manufacturing, 32 (2), 579–596. https://doi.org/10.1007/s10845-020-01589-8 .
Humayed, A., Lin, J., Li, F., & Luo, B. (2017). Cyber-physical systems security—A survey. IEEE Internet of Things Journal, 4 (6), 1802–1831. https://doi.org/10.1109/JIOT.2017.2703172 .
Jacob, E. K. (2004). Classification and categorization: A difference that makes a difference. Library Trends, 52 (3), 515–540.
Jiang, Y., Yin, S., & Kaynak, O. (2018). Data-driven monitoring and safety control of industrial cyber-physical Systems: Basics and beyond. IEEE Access, 6, 47374–47384. https://doi.org/10.1109/ACCESS.2018.2866403 .
Khaitan, S. K., & McCalley, J. D. (2014). Design techniques and applications of cyberphysical systems: A survey. IEEE Systems Journal, 9 (2), 1–16. https://doi.org/10.1109/JSYST.2014.2322503 .
Kim, J. H. (2017). A review of cyber-physical system research relevant to the emerging IT trends: Industry 4.0, IoT, Big Data, and cloud computing. Journal of Industrial Integration and Management, 2 (3), 1750011–1750022. https://doi.org/10.1142/S2424862217500117 .
Lasi, H., Fettke, P., Kemper, H. G., Feld, T., & Hoffmann, M. (2014). Industry 4.0. Business & Information Systems Engineering, 6 (4), 239–242. https://doi.org/10.1007/s12599-014-0334-4 .
Lee, E. A. (2006). Cyber-physical systems - are computing foundations adequate? In NSF Workshop On Cyber-Physical Systems: Research Motivation, Techniques and Roadmap , 1–9
Lee, J., Bagheri, B., & Kao, H. A. (2015). A cyber-physical systems architecture for Industry 4.0-based manufacturing systems. Manufacturing Letters, 3, 18–23. https://doi.org/10.1016/j.mfglet.2014.12.001 .
Lei, Y., Rao, Y., Wu, J., & Lin, C. H. (2020). BIM based cyber-physical systems for intelligent disaster prevention. Journal of Industrial Information Integration, 20, 100171. https://doi.org/10.1016/j.jii.2020.100171 .
Li, L. (2018). China’s manufacturing locus in 2025: With a comparison of “Made-in-China 2025” and “Industry 4.0.” Technological Forecasting and Social Change, 135, 66–74. https://doi.org/10.1016/j.techfore.2017.05.028 .
Liao, H., Wang, B., Li, B., & Weyman-Jones, T. (2016). ICT as a general-purpose technology: The productivity of ICT in the United States revisited. Information Economics & Policy, 36, 10–25. https://doi.org/10.1016/j.infoecopol.2016.05.001 .
Liu, Y., Peng, Y., Wang, B., Yao, S., & Liu, Z. (2017). Review on cyber-physical systems. IEEE/CAA Journal of Automatica Sinica, 4 (1), 27–40. https://doi.org/10.1109/JAS.2017.7510349 .
Lozano, C. V., & Vijayan, K. K. (2020). Literature review on cyber physical systems design. Procedia Manufacturing, 45 , 295–300. https://doi.org/10.1016/j.promfg.2020.04.020
Lu, C., Saifullah, A., Li, B., Sha, M., Gonzalez, H., Gunatilaka, D., & Chen, Y. (2016). Real-time wireless sensor-actuator networks for industrial cyber-physical systems. Proceedings of the IEEE , 104 (5), 1013–1024. https://doi.org/10.1109/JPROC.2015.2497161
Lu, Y. (2017). Cyber physical system (CPS)-based Industry 4.0: A survey. Journal of Industrial Integration and Management, 2 (3), 1750014–1750057. https://doi.org/10.1142/S2424862217500142 .
Ma, S., Zhang, Y., Lv, J., Yang, H., & Wu, J. (2019). Energy-cyber-physical system enabled management for energy-intensive manufacturing industries. Journal of Cleaner Production, 226, 892–903. https://doi.org/10.1016/j.jclepro.2019.04.134 .
Marini, A., & Bianchini, D. (2016). Big Data as a service for monitoring cyber-physical production systems. In T. Claus, F. Herrmann, M. Manitz, & O. Rose (Eds.), Proceedings, 30th European conference on modelling and simulation ECMS 2016. European Council for Modelling and Simulation. https://doi.org/10.7148/2016-0579
Mayring, P. (2015). Qualitative content analysis: Theoretical background and procedures. In A. Bikner-Ahsbahs, C. Knipping, & N. Presmeg (Eds.), Advances in Mathematics Education: Approaches to Qualitative Research in Mathematics Education (pp. 365–380). Springer. https://doi.org/10.1007/978-94-017-9181-6_13
Meesublak, K., & Klinsukont, T. (2020). A cyber-physical system approach for predictive maintenance. In IEEE International Conference on Smart Internet of Things (SmartIoT) (pp. 337–341). IEEE. https://doi.org/10.1109/SmartIoT49966.2020.00061
Monostori, L. (2014). Cyber-physical production systems: Roots, Expectations and R&D Challenges. Procedia CIRP, 17, 9–13. https://doi.org/10.1016/j.procir.2014.03.115 .
Monostori, L., Kádár, B., Bauernhansl, T., Kondoh, S., Kumara, S., Reinhart, G., & Ueda, K. (2016). Cyber-physical systems in manufacturing. CIRP Annals - Manufacturing Technology, 65 (2), 621–641. https://doi.org/10.1016/j.cirp.2016.06.005 .
Muccini, H., Sharaf, M., & Weyns, D. (2016). Self-adaptation for cyber-physical systems: A systematic literature review. In 11th international symposium on software engineering for adaptive and self-managing systems (SEAMS) (pp. 75–81). IEEE Computer Society; The Association for Computing Machinery. https://doi.org/10.1145/2897053.2897069
Napoleone, A., Macchi, M., & Pozzetti, A. (2020). A review on the characteristics of cyber-physical systems for the future smart factories. Journal of Manufacturing Systems, 54, 305–335. https://doi.org/10.1016/j.jmsy.2020.01.007 .
Nickerson, R. C., Varshney, U., & Muntermann, J. (2013). A method for taxonomy development and its application in information systems. European Journal of Information Systems, 22 (3), 336–359. https://doi.org/10.1057/ejis.2012.26 .
Ning, Z., Hou, W., Hu, X., & Gong, X. (2017). A cloud-supported cps approach to control decision of process manufacturing: 3D ONoC. In 13th IEEE Conference on Automation Science and Engineering (CASE) (pp. 458–463). IEEE. https://doi.org/10.1109/COASE.2017.8256147
Oks, S. J., Fritzsche, A., & Möslein, K. M. (2017). An application map for industrial cyber-physical systems. In S. Jeschke, C. Brecher, H. Song, & D. B. Rawat (Eds.), Industrial Internet of Things: Cybermanufacturing Systems (pp. 21–46). Springer International Publishing. https://doi.org/10.1007/978-3-319-42559-7_2
Oks, S. J., Fritzsche, A., & Möslein, K. M. (2018). Engineering industrial cyber-physical systems: An application map based method. Procedia CIRP, 72, 456–461. https://doi.org/10.1016/j.procir.2018.03.126 .
Oks, S. J., & Jalowski, M. (2020). QuartRevo Industry 4.0 Compendium. https://quartrevo.de/#compendiumAnchor
Pivoto, D. G., de Almeida, L. F., Righi, D. R., Rodrigues, R., Lugli, J. J., & Alberti, A. M. (2021). Cyber-physical systems architectures for industrial internet of things applications in Industry 4.0: A literature review. Journal of Manufacturing Systems, 58, 176–192. https://doi.org/10.1016/j.jmsy.2020.11.017 .
Rajkumar, R., Lee, I., Sha, L., & Stankovic, J. (2010). Cyber-physical systems: The next computing revolution. In S. Sapatnekar (Ed.), Proceedings of the 47th Design Automation Conference - DAC ‘10 (pp. 731–736). ACM Press. https://doi.org/10.1145/1837274.1837461
Rojas, R. A., & Rauch, E. (2019). From a literature review to a conceptual framework of enablers for smart manufacturing control. The International Journal of Advanced Manufacturing Technology, 104 (1–4), 517–533. https://doi.org/10.1007/s00170-019-03854-4 .
Rosenberg, N., & Trajtenberg, M. (2009). A general-purpose technology at work: The Corliss steam engine in the late-nineteenth-century United States. In N. Rosenberg (Ed.), Studies on Science and the Innovation Process (pp. 97–135). WORLD SCIENTIFIC. https://doi.org/10.1142/9789814273596_0006
Tao, H., Bhuiyan, M. Z. A., Rahman, M. A., Wang, T., Wu, J., Salih, S. Q., & Hayajneh, T. (2020). TrustData: Trustworthy and secured data collection for event detection in industrial cyber-physical system. IEEE Transactions on Industrial Informatics, 16 (5), 3311–3321. https://doi.org/10.1109/TII.2019.2950192 .
Thoben, K. D., Wiesner, S., & Wuest, T. (2017). “Industrie 4.0” and Smart Manufacturing – A review of research issues and application examples. International Journal of Automation Technology, 11 (1), 4–16. https://doi.org/10.20965/ijat.2017.p0004 .
Tranfield, D., Denyer, D., & Smart, P. (2003). Towards a methodology for developing evidence-informed management knowledge by means of systematic review. British Journal of Management, 14 (3), 207–222
Trunzer, E., Wullenweber, A., & Vogel-Heuser, B. (2020). Graphical modeling notation for data collection and analysis architectures in cyber-physical systems of systems. Journal of Industrial Information Integration, 19, 100155–100165. https://doi.org/10.1016/j.jii.2020.100155 .
Vogel-Heuser, B., & Hess, D. (2016). Guest Editorial Industry 4.0–Prerequisites and Visions. IEEE Transactions on Automation Science and Engineering, 13 (2), 411–413. https://doi.org/10.1109/TASE.2016.2523639 .
Vom Brocke, J., Simons, A., Riemer, K., Niehaves, B., Plattfaut, R., & Cleven, A. (2015). Standing on the shoulders of giants: Challenges and recommendations of literature search in information systems research. Communications of the Association for Information Systems , 37 . https://doi.org/10.17705/1CAIS.03709
Wand, Y., & Weber, R. (2004). Reflection: Ontology in information systems. Journal of Database Management, 15 (2), 3–6.
Wu, X., Goepp, V., & Siadat, A. (2020). Concept and engineering development of cyber physical production systems: a systematic literature review. The International Journal of Advanced Manufacturing Technology, 111 (1–2), 243–261. https://doi.org/10.1007/s00170-020-06110-2 .
Xu, L. D., & Duan, L. (2019). Big data for cyber physical systems in industry 4.0: A survey. Enterprise Information Systems, 13 (2), 148–169. https://doi.org/10.1080/17517575.2018.1442934 .
Xu, L. D., Xu, E. L., & Li, L. (2018). Industry 4.0: state of the art and future trends. International Journal of Production Research, 56 (8), 2941–2962. https://doi.org/10.1080/00207543.2018.1444806 .
Zhang, C., Chen, Y., Chen, H., & Chong, D. (2021). Industry 4.0 and its implementation: A review. Information Systems Frontiers . https://doi.org/10.1007/s10796-021-10153-5 .
Zhang, C., Wang, Z., Ding, K., Chan, F. T., & Ji, W. (2020). An energy-aware cyber physical system for energy: Big data analysis and recessive production anomalies detection in discrete manufacturing workshops. International Journal of Production Research, 58 (23), 7059–7077. https://doi.org/10.1080/00207543.2020.1748904 .
Zhao, W., Jiang, C., Gao, H., Yang, S., & Luo, X. (2021). Blockchain-enabled cyber–physical systems: A review. IEEE Internet of Things Journal, 8 (6), 4023–4034. https://doi.org/10.1109/JIOT.2020.3014864 .
Download references
Acknowledgements
The research presented in this paper was partially funded by the German Federal Ministry of Education and Research in the course of the project “ConSensE” (FKZ 02J19B062) as well as by the Friedrich-Alexander-Universität Erlangen-Nürnberg within the Emerging Fields Initiative “Sustainable Smart Industry”.
Open Access funding enabled and organized by Projekt DEAL. The research leading to these results received funding from the German Federal Ministry of Education and Research in the course of the project “ConSensE” under Grant Agreement No FKZ 02J19B062 as well as by the Friedrich-Alexander-Universität Erlangen-Nürnberg within the Emerging Fields Initiative “Sustainable Smart Industry”.
Author information
Authors and affiliations.
Chair of Information Systems, Innovation & Value Creation, Friedrich-Alexander-Universität Erlangen-Nürnberg, Lange Gasse 20, 90403, Nürnberg, Germany
Sascha Julian Oks, Max Jalowski & Kathrin M. Möslein
Institute of Manufacturing Technology, Friedrich-Alexander-Universität Erlangen-Nürnberg, Egerlandstraße 13, 91058, Erlangen, Germany
Michael Lechner & Marion Merklein
Deloitte, Franklinstraße 46-48, 60486, Frankfurt am Main, Germany
Stefan Mirschberger
Institute of Automation and Information Systems, Technische Universität München, Boltzmannstraße 15, 85748, Garching bei München, Germany
Birgit Vogel-Heuser
You can also search for this author in PubMed Google Scholar
Corresponding author
Correspondence to Sascha Julian Oks .
Ethics declarations
Competing interests.
All authors certify that they have no affiliations with or involvement in any organization or entity with any financial interest or non-financial interest in the subject matter or materials discussed in this manuscript. Nor are there any other types of conflicting or competing interests.
Additional information
Publisher’s note.
Springer Nature remains neutral with regard to jurisdictional claims in published maps and institutional affiliations.
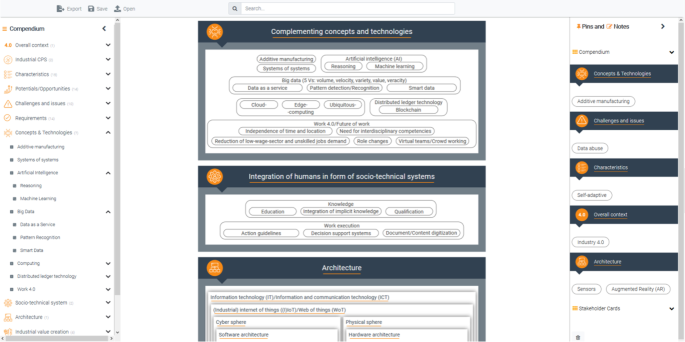
Web tool Industry 4.0 Compendium
Rights and permissions
Open Access This article is licensed under a Creative Commons Attribution 4.0 International License, which permits use, sharing, adaptation, distribution and reproduction in any medium or format, as long as you give appropriate credit to the original author(s) and the source, provide a link to the Creative Commons licence, and indicate if changes were made. The images or other third party material in this article are included in the article's Creative Commons licence, unless indicated otherwise in a credit line to the material. If material is not included in the article's Creative Commons licence and your intended use is not permitted by statutory regulation or exceeds the permitted use, you will need to obtain permission directly from the copyright holder. To view a copy of this licence, visit http://creativecommons.org/licenses/by/4.0/ .
Reprints and permissions
About this article
Oks, S.J., Jalowski, M., Lechner, M. et al. Cyber-Physical Systems in the Context of Industry 4.0: A Review, Categorization and Outlook. Inf Syst Front (2022). https://doi.org/10.1007/s10796-022-10252-x
Download citation
Accepted : 08 January 2022
Published : 07 April 2022
DOI : https://doi.org/10.1007/s10796-022-10252-x
Share this article
Anyone you share the following link with will be able to read this content:
Sorry, a shareable link is not currently available for this article.
Provided by the Springer Nature SharedIt content-sharing initiative
- Cyber-physical systems (CPS)
- Industry 4.0
- State of research
- Categorization
- Find a journal
- Publish with us
- Track your research
- Publications
- News and Events
- Education and Outreach
Software Engineering Institute
Sei digital library, latest publications, embracing ai: unlocking scalability and transformation through generative text, imagery, and synthetic audio, august 28, 2024 • webcast, by tyler brooks , shannon gallagher , dominic a. ross.
In this webcast, Tyler Brooks, Shannon Gallagher, and Dominic Ross aim to demystify AI and illustrate its transformative power in achieving scalability, adapting to changing landscapes, and driving digital innovation.
Counter AI: What Is It and What Can You Do About It?
August 27, 2024 • white paper, by nathan m. vanhoudnos , carol j. smith , matt churilla , shing-hon lau , lauren mcilvenny , greg touhill.
This paper describes counter artificial intelligence (AI) and provides recommendations on what can be done about it.
Using Quality Attribute Scenarios for ML Model Test Case Generation
August 27, 2024 • conference paper, by rachel brower-sinning , grace lewis , sebastián echeverría , ipek ozkaya.
This paper presents an approach based on quality attribute (QA) scenarios to elicit and define system- and model-relevant test cases for ML models.
3 API Security Risks (and How to Protect Against Them)
August 27, 2024 • podcast, by mckinley sconiers-hasan.
McKinley Sconiers-Hasan discusses three API risks and how to address them through the lens of zero trust.
Lessons Learned in Coordinated Disclosure for Artificial Intelligence and Machine Learning Systems
August 20, 2024 • white paper, by allen d. householder , vijay s. sarvepalli , jeff havrilla , matt churilla , lena pons , shing-hon lau , nathan m. vanhoudnos , andrew kompanek , lauren mcilvenny.
In this paper, the authors describe lessons learned from coordinating AI and ML vulnerabilities at the SEI's CERT/CC.
On the Design, Development, and Testing of Modern APIs
July 30, 2024 • white paper, by alejandro gomez , alex vesey.
This white paper discusses the design, desired qualities, development, testing, support, and security of modern application programming interfaces (APIs).
Evaluating Large Language Models for Cybersecurity Tasks: Challenges and Best Practices
July 26, 2024 • podcast, by jeff gennari , samuel j. perl.
Jeff Gennari and Sam Perl discuss applications for LLMs in cybersecurity, potential challenges, and recommendations for evaluating LLMs.
Capability-based Planning for Early-Stage Software Development
July 24, 2024 • podcast, by anandi hira , bill nichols.
This SEI podcast introduces capability-based planning (CBP) and its use and application in software acquisition.
A Model Problem for Assurance Research: An Autonomous Humanitarian Mission Scenario
July 23, 2024 • technical note, by gabriel moreno , anton hristozov , john e. robert , mark h. klein.
This report describes a model problem to support research in large-scale assurance.
Safeguarding Against Recent Vulnerabilities Related to Rust
June 28, 2024 • podcast, by david svoboda.
David Svoboda discusses two vulnerabilities related to Rust, their sources, and how to mitigate them.

IMAGES
COMMENTS
Large Scale Systems and Fuzzy Cognitive Maps: A critical overview of challenges and research opportunities. Peter P. Groumpos, in Annual Reviews in Control, 2014 6.2 Cyber-Physical Systems (CPS). A Cyber-Physical System (CPS) is a system of collaborating computational elements controlling physical entities.Today, a pre-cursor generation of cyber-physical systems can be found in areas as ...
Open issues in medical cyber physical system (MCPS) During our research, we have found a few things that need to be done in the field of Medical Cyber Physical System (MCPS). These issues can be called: 8.1.1. Security and privacy. Security and privacy issues are being talked about in MCPS, which is when medical devices can talk to each other.
ACM Transactions on Cyber-Physical Systems (TCPS) is the premier journal for the publication of high-quality original research papers and survey papers that have scientific and technological understanding of the interactions of information processing, networking and physical processes. TCPS covers the following topics: Computation Abstractions; System Modeling and Languages
Journal overview. Cyber-Physical Systems is an international interdisciplinary journal dedicated to publishing the highest quality research in the rapidly-growing field of cyber-physical systems / Internet-of-Things. Publications cover theory, algorithms, simulations, architectures, implementations, services and applications of state-of-the-art ...
The concept of a cyber-physical system(CPS) was first used by Hellen Gill at National Science Foundation (NSF) in the United States in 2006 to describe systems that linked the physical and digital worlds (Lee et al. 2015).The scientific community defined CPS from a different perspective reviewed by (Chen 2017a).Based on this paper, CPS is defined as a collection of embedded systems, real-time ...
Cyber-physical system (CPS) is an interdisciplinary research field that features systematic integrations of computation, communication, and control of physical processes. It is characterized by the deep complex intertwining process among the embedded cyber components and the dynamic physical components that involve mechanical components, human ...
Cyber-physical systems (CPS) are characterized by integrating cybernetic and physical processes. The theories and applications of CPS face the enormous challenges. The aim of this paper is to provide a latest understanding of this emerging multi-disciplinary methodology. First, the features of CPS are described, and the research progresses are summarized from different components in CPS, such ...
Research challenges. 1. Introduction. Cyber physical systems (CPS) are a combination of closely integrated physical processes, networking and computation. The physical process is monitored and controlled by embedded (cyber) subsystems via networked systems with feedback loops to change their behavior when needed ( Asare et al., 2012 ).
In this article, we combine the system theory as well as its digital translation and the use of quantum decision networks to propose a quantum framework for Industry 4.0 cyber-physical systems ...
A Cyber-Physical System (CPS) is a spatiotemporal multi-dimensional and heterogeneous hybrid autonomous system composed of deep integration of information resources and physical systems. ... In the ADN with a high proportion of distributed generations and electrified loads in the future, it is an important research topic to strengthen the ...
His research spans radio resource management, Internet of Things (IoT) services, and modeling in networked cyber-physical systems. Abd-Elhamid is a senior member of the IEEE, and a recipient of the Natural Sciences and Engineering Research Council (NSERC) Early Career Researcher Award as well as several awards at Alfaisal University for ...
Cyber-Physical Systems (CPS) are integrations of computation with physical processes. [1] In cyber-physical systems, physical and software components are deeply intertwined, able to operate on different spatial and temporal scales, exhibit multiple and distinct behavioral modalities, and interact with each other in ways that change with context. [2] [3] CPS involves transdisciplinary ...
Then, we introduce the recent trend in CPS research with an emphasis on major research topics in CPS. ... computation are tightly merged into a unified framework by carefully considering the complex interactions among all the physical and cyber parts in the system. We believe that this special section will be a meaningful step towards this end.
Cyber-physical system (CPS) is an integration of physical processes with computation and communication. ... mitigation for cyber-physical wireless body area Network System using social networks IEEE Transactions on Emerging Topics in Computing 2013 1 121-132. Crossref. ... A Review of Cyber-Physical System Research Relevant to the Emerging IT ...
This relates or research on Artificial Intelligence (AI) with Cyber Physical Systems (CPS). By Cyber Physical Systems (CPS), we refer to computer-human networks, controlling physical processes, where physical processes affect computations and vice versa. One modern version of Cyber Physical Systems is the Internet of Things (IoT).
Recently, there is a significant growth in the use of the Cyber-Physical System (CPS). New technologies such as Internet of Things (IoT), Industry 4.0, and Analytics have become enablers of CPS implementation. Study of the development and application of CPS in the supply chain context is valuable to operations management and information systems research and practice; especially, a focus on IoT ...
The purpose of this Research Topic is to promote outstanding research concerning human-in-the-loop cyber-physical systems, focusing on state-of-the-art progress, developments, and new trends. Cyber-physical systems (CPS) are connecting our physical world and cyberspace through real-time sensing, situational awareness, and intelligent control.
Cyber-Physical Systems. The term Cyber-Physical System (CPS) is used to describe dynamic systems which combine components characterized by a physical state (e.g., the location, power level, and temperature of a mobile robot) with components (mostly digital devices empowered by software) characterized by an operational state or mode (e.g., on ...
A Cyber-Physical-Social System (CPSS) is an evolving subset of Cyber-Physical Systems (CPS), which involve the interlinking of the cyber, physical, and social domains within a system-of-systems mindset. ... The oldest research topics were located in the center, with newer research extending to either side, as shown in Figure 6. The oldest ...
About the journal. Internet of Things and Cyber-Physical Systems publishes rigorously peer-reviewed, high quality original articles and authoritative reviews that focus on Internet of Things and Cyber-Physical Systems. All articles are published fully Open Access on ScienceDirect. The journal welcomes research …. View full aims & scope.
This research focuses on the top 10 industries advancing cyber-physical systems in 2023. For this, we analyzed 2313 emerging companies working in the field. Read more to explore the industry-specific applications of CPS and find out how they impact your business. Tree Map reveals the Cyber-Physical Systems Examples across 10 Industries
This Section lays the groundwork for this research; the theoretical foundations of CPS are presented in 2.1 and additional related work is introduced that also seeks to structure industrial CPS in 2.2.. 2.1 Foundations of Cyber-Physical Systems. The term CPS was introduced by E. A. Lee in 2006; since then, the topic has been further analyzed and developed in several scientific and practical ...
The SEI Digital Library provides access to more than 6,000 documents from three decades of research into best practices in software engineering. These documents include technical reports, presentations, webcasts, podcasts and other materials searchable by user-supplied keywords and organized by topic, publication type, publication year, and author.
Such that their multi-scale interconnection and interaction is better captured by the notion of Cyber-Physical System of Systems (CPSoS), defining a large and distributed complex system of CPSs. ... To get an overview on the open research topics, the general topics are split into following categories. They only address several general topics as ...
The 25 disruptive technologies to watch on the Gartner, Inc. Hype Cycle for Emerging Technologies, 2024 fall into four key areas: autonomous AI, developer productivity, total experience, and human-centric security and privacy programs. "Generative AI (GenAI) is over the Peak of Inflated Expectations as business focus continues to shift from excitement around foundation models to use cases ...