Have a language expert improve your writing
Run a free plagiarism check in 10 minutes, generate accurate citations for free.
- Knowledge Base
Methodology
- Quasi-Experimental Design | Definition, Types & Examples

Quasi-Experimental Design | Definition, Types & Examples
Published on July 31, 2020 by Lauren Thomas . Revised on January 22, 2024.
Like a true experiment , a quasi-experimental design aims to establish a cause-and-effect relationship between an independent and dependent variable .
However, unlike a true experiment, a quasi-experiment does not rely on random assignment . Instead, subjects are assigned to groups based on non-random criteria.
Quasi-experimental design is a useful tool in situations where true experiments cannot be used for ethical or practical reasons.
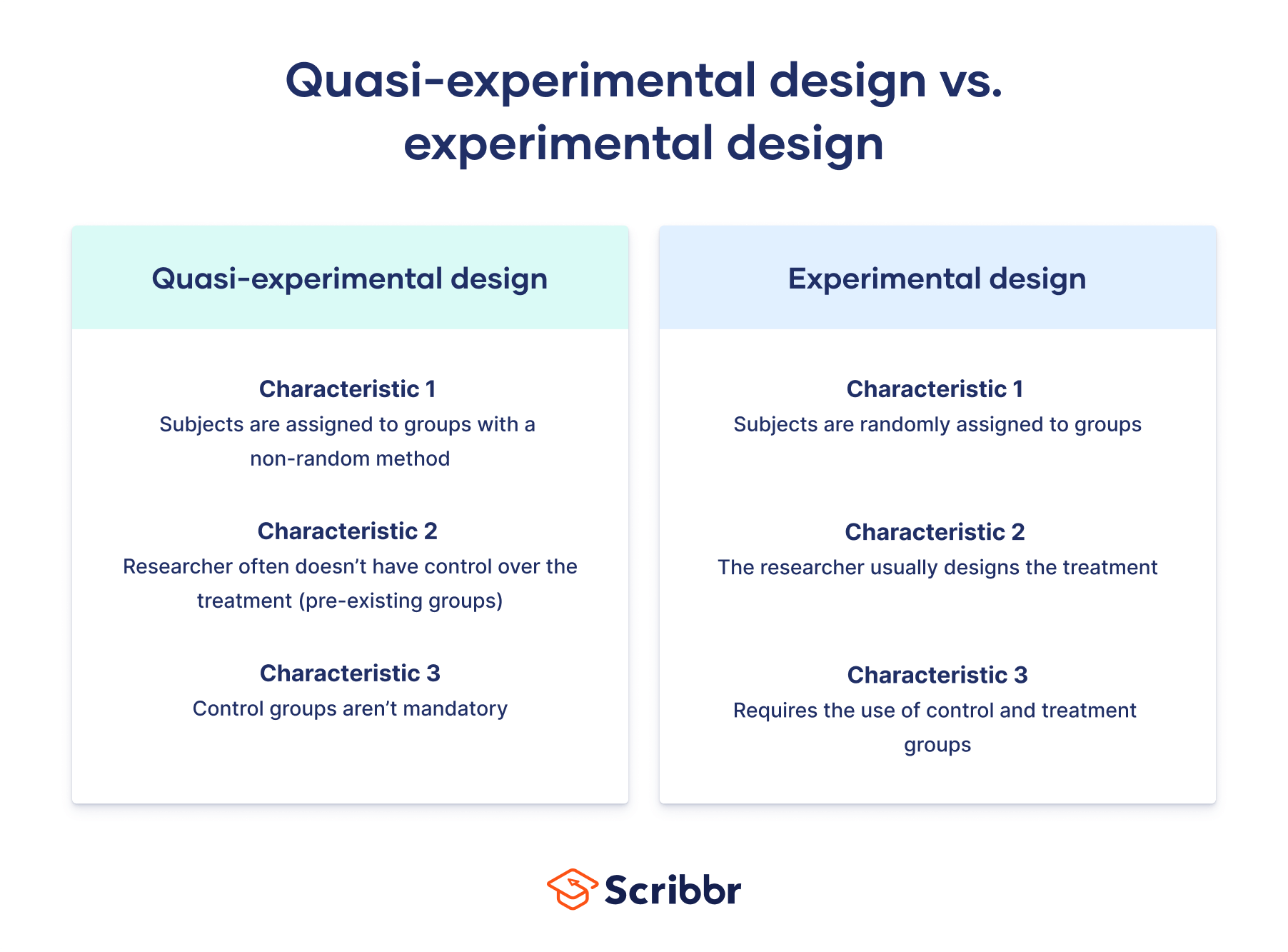
Table of contents
Differences between quasi-experiments and true experiments, types of quasi-experimental designs, when to use quasi-experimental design, advantages and disadvantages, other interesting articles, frequently asked questions about quasi-experimental designs.
There are several common differences between true and quasi-experimental designs.
True experimental design | Quasi-experimental design | |
---|---|---|
Assignment to treatment | The researcher subjects to control and treatment groups. | Some other, method is used to assign subjects to groups. |
Control over treatment | The researcher usually . | The researcher often , but instead studies pre-existing groups that received different treatments after the fact. |
Use of | Requires the use of . | Control groups are not required (although they are commonly used). |
Example of a true experiment vs a quasi-experiment
However, for ethical reasons, the directors of the mental health clinic may not give you permission to randomly assign their patients to treatments. In this case, you cannot run a true experiment.
Instead, you can use a quasi-experimental design.
You can use these pre-existing groups to study the symptom progression of the patients treated with the new therapy versus those receiving the standard course of treatment.
Receive feedback on language, structure, and formatting
Professional editors proofread and edit your paper by focusing on:
- Academic style
- Vague sentences
- Style consistency
See an example
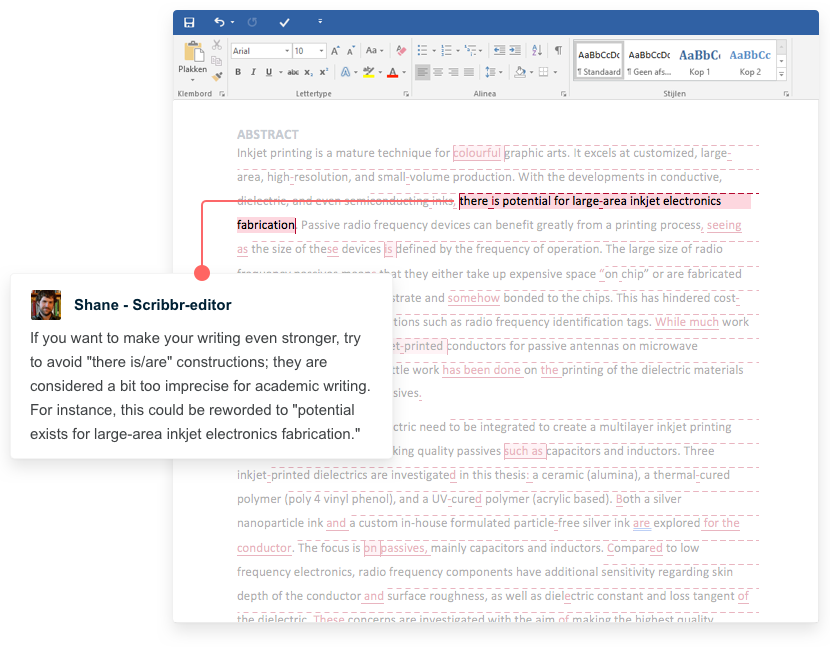
Many types of quasi-experimental designs exist. Here we explain three of the most common types: nonequivalent groups design, regression discontinuity, and natural experiments.
Nonequivalent groups design
In nonequivalent group design, the researcher chooses existing groups that appear similar, but where only one of the groups experiences the treatment.
In a true experiment with random assignment , the control and treatment groups are considered equivalent in every way other than the treatment. But in a quasi-experiment where the groups are not random, they may differ in other ways—they are nonequivalent groups .
When using this kind of design, researchers try to account for any confounding variables by controlling for them in their analysis or by choosing groups that are as similar as possible.
This is the most common type of quasi-experimental design.
Regression discontinuity
Many potential treatments that researchers wish to study are designed around an essentially arbitrary cutoff, where those above the threshold receive the treatment and those below it do not.
Near this threshold, the differences between the two groups are often so minimal as to be nearly nonexistent. Therefore, researchers can use individuals just below the threshold as a control group and those just above as a treatment group.
However, since the exact cutoff score is arbitrary, the students near the threshold—those who just barely pass the exam and those who fail by a very small margin—tend to be very similar, with the small differences in their scores mostly due to random chance. You can therefore conclude that any outcome differences must come from the school they attended.
Natural experiments
In both laboratory and field experiments, researchers normally control which group the subjects are assigned to. In a natural experiment, an external event or situation (“nature”) results in the random or random-like assignment of subjects to the treatment group.
Even though some use random assignments, natural experiments are not considered to be true experiments because they are observational in nature.
Although the researchers have no control over the independent variable , they can exploit this event after the fact to study the effect of the treatment.
However, as they could not afford to cover everyone who they deemed eligible for the program, they instead allocated spots in the program based on a random lottery.
Although true experiments have higher internal validity , you might choose to use a quasi-experimental design for ethical or practical reasons.
Sometimes it would be unethical to provide or withhold a treatment on a random basis, so a true experiment is not feasible. In this case, a quasi-experiment can allow you to study the same causal relationship without the ethical issues.
The Oregon Health Study is a good example. It would be unethical to randomly provide some people with health insurance but purposely prevent others from receiving it solely for the purposes of research.
However, since the Oregon government faced financial constraints and decided to provide health insurance via lottery, studying this event after the fact is a much more ethical approach to studying the same problem.
True experimental design may be infeasible to implement or simply too expensive, particularly for researchers without access to large funding streams.
At other times, too much work is involved in recruiting and properly designing an experimental intervention for an adequate number of subjects to justify a true experiment.
In either case, quasi-experimental designs allow you to study the question by taking advantage of data that has previously been paid for or collected by others (often the government).
Quasi-experimental designs have various pros and cons compared to other types of studies.
- Higher external validity than most true experiments, because they often involve real-world interventions instead of artificial laboratory settings.
- Higher internal validity than other non-experimental types of research, because they allow you to better control for confounding variables than other types of studies do.
- Lower internal validity than true experiments—without randomization, it can be difficult to verify that all confounding variables have been accounted for.
- The use of retrospective data that has already been collected for other purposes can be inaccurate, incomplete or difficult to access.
Here's why students love Scribbr's proofreading services
Discover proofreading & editing
If you want to know more about statistics , methodology , or research bias , make sure to check out some of our other articles with explanations and examples.
- Normal distribution
- Degrees of freedom
- Null hypothesis
- Discourse analysis
- Control groups
- Mixed methods research
- Non-probability sampling
- Quantitative research
- Ecological validity
Research bias
- Rosenthal effect
- Implicit bias
- Cognitive bias
- Selection bias
- Negativity bias
- Status quo bias
A quasi-experiment is a type of research design that attempts to establish a cause-and-effect relationship. The main difference with a true experiment is that the groups are not randomly assigned.
In experimental research, random assignment is a way of placing participants from your sample into different groups using randomization. With this method, every member of the sample has a known or equal chance of being placed in a control group or an experimental group.
Quasi-experimental design is most useful in situations where it would be unethical or impractical to run a true experiment .
Quasi-experiments have lower internal validity than true experiments, but they often have higher external validity as they can use real-world interventions instead of artificial laboratory settings.
Cite this Scribbr article
If you want to cite this source, you can copy and paste the citation or click the “Cite this Scribbr article” button to automatically add the citation to our free Citation Generator.
Thomas, L. (2024, January 22). Quasi-Experimental Design | Definition, Types & Examples. Scribbr. Retrieved September 9, 2024, from https://www.scribbr.com/methodology/quasi-experimental-design/
Is this article helpful?
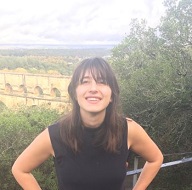
Lauren Thomas
Other students also liked, guide to experimental design | overview, steps, & examples, random assignment in experiments | introduction & examples, control variables | what are they & why do they matter, "i thought ai proofreading was useless but..".
I've been using Scribbr for years now and I know it's a service that won't disappoint. It does a good job spotting mistakes”
- Privacy Policy

Home » Quasi-Experimental Research Design – Types, Methods
Quasi-Experimental Research Design – Types, Methods
Table of Contents

Quasi-Experimental Design
Quasi-experimental design is a research method that seeks to evaluate the causal relationships between variables, but without the full control over the independent variable(s) that is available in a true experimental design.
In a quasi-experimental design, the researcher uses an existing group of participants that is not randomly assigned to the experimental and control groups. Instead, the groups are selected based on pre-existing characteristics or conditions, such as age, gender, or the presence of a certain medical condition.
Types of Quasi-Experimental Design
There are several types of quasi-experimental designs that researchers use to study causal relationships between variables. Here are some of the most common types:
Non-Equivalent Control Group Design
This design involves selecting two groups of participants that are similar in every way except for the independent variable(s) that the researcher is testing. One group receives the treatment or intervention being studied, while the other group does not. The two groups are then compared to see if there are any significant differences in the outcomes.
Interrupted Time-Series Design
This design involves collecting data on the dependent variable(s) over a period of time, both before and after an intervention or event. The researcher can then determine whether there was a significant change in the dependent variable(s) following the intervention or event.
Pretest-Posttest Design
This design involves measuring the dependent variable(s) before and after an intervention or event, but without a control group. This design can be useful for determining whether the intervention or event had an effect, but it does not allow for control over other factors that may have influenced the outcomes.
Regression Discontinuity Design
This design involves selecting participants based on a specific cutoff point on a continuous variable, such as a test score. Participants on either side of the cutoff point are then compared to determine whether the intervention or event had an effect.
Natural Experiments
This design involves studying the effects of an intervention or event that occurs naturally, without the researcher’s intervention. For example, a researcher might study the effects of a new law or policy that affects certain groups of people. This design is useful when true experiments are not feasible or ethical.
Data Analysis Methods
Here are some data analysis methods that are commonly used in quasi-experimental designs:
Descriptive Statistics
This method involves summarizing the data collected during a study using measures such as mean, median, mode, range, and standard deviation. Descriptive statistics can help researchers identify trends or patterns in the data, and can also be useful for identifying outliers or anomalies.
Inferential Statistics
This method involves using statistical tests to determine whether the results of a study are statistically significant. Inferential statistics can help researchers make generalizations about a population based on the sample data collected during the study. Common statistical tests used in quasi-experimental designs include t-tests, ANOVA, and regression analysis.
Propensity Score Matching
This method is used to reduce bias in quasi-experimental designs by matching participants in the intervention group with participants in the control group who have similar characteristics. This can help to reduce the impact of confounding variables that may affect the study’s results.
Difference-in-differences Analysis
This method is used to compare the difference in outcomes between two groups over time. Researchers can use this method to determine whether a particular intervention has had an impact on the target population over time.
Interrupted Time Series Analysis
This method is used to examine the impact of an intervention or treatment over time by comparing data collected before and after the intervention or treatment. This method can help researchers determine whether an intervention had a significant impact on the target population.
Regression Discontinuity Analysis
This method is used to compare the outcomes of participants who fall on either side of a predetermined cutoff point. This method can help researchers determine whether an intervention had a significant impact on the target population.
Steps in Quasi-Experimental Design
Here are the general steps involved in conducting a quasi-experimental design:
- Identify the research question: Determine the research question and the variables that will be investigated.
- Choose the design: Choose the appropriate quasi-experimental design to address the research question. Examples include the pretest-posttest design, non-equivalent control group design, regression discontinuity design, and interrupted time series design.
- Select the participants: Select the participants who will be included in the study. Participants should be selected based on specific criteria relevant to the research question.
- Measure the variables: Measure the variables that are relevant to the research question. This may involve using surveys, questionnaires, tests, or other measures.
- Implement the intervention or treatment: Implement the intervention or treatment to the participants in the intervention group. This may involve training, education, counseling, or other interventions.
- Collect data: Collect data on the dependent variable(s) before and after the intervention. Data collection may also include collecting data on other variables that may impact the dependent variable(s).
- Analyze the data: Analyze the data collected to determine whether the intervention had a significant impact on the dependent variable(s).
- Draw conclusions: Draw conclusions about the relationship between the independent and dependent variables. If the results suggest a causal relationship, then appropriate recommendations may be made based on the findings.
Quasi-Experimental Design Examples
Here are some examples of real-time quasi-experimental designs:
- Evaluating the impact of a new teaching method: In this study, a group of students are taught using a new teaching method, while another group is taught using the traditional method. The test scores of both groups are compared before and after the intervention to determine whether the new teaching method had a significant impact on student performance.
- Assessing the effectiveness of a public health campaign: In this study, a public health campaign is launched to promote healthy eating habits among a targeted population. The behavior of the population is compared before and after the campaign to determine whether the intervention had a significant impact on the target behavior.
- Examining the impact of a new medication: In this study, a group of patients is given a new medication, while another group is given a placebo. The outcomes of both groups are compared to determine whether the new medication had a significant impact on the targeted health condition.
- Evaluating the effectiveness of a job training program : In this study, a group of unemployed individuals is enrolled in a job training program, while another group is not enrolled in any program. The employment rates of both groups are compared before and after the intervention to determine whether the training program had a significant impact on the employment rates of the participants.
- Assessing the impact of a new policy : In this study, a new policy is implemented in a particular area, while another area does not have the new policy. The outcomes of both areas are compared before and after the intervention to determine whether the new policy had a significant impact on the targeted behavior or outcome.
Applications of Quasi-Experimental Design
Here are some applications of quasi-experimental design:
- Educational research: Quasi-experimental designs are used to evaluate the effectiveness of educational interventions, such as new teaching methods, technology-based learning, or educational policies.
- Health research: Quasi-experimental designs are used to evaluate the effectiveness of health interventions, such as new medications, public health campaigns, or health policies.
- Social science research: Quasi-experimental designs are used to investigate the impact of social interventions, such as job training programs, welfare policies, or criminal justice programs.
- Business research: Quasi-experimental designs are used to evaluate the impact of business interventions, such as marketing campaigns, new products, or pricing strategies.
- Environmental research: Quasi-experimental designs are used to evaluate the impact of environmental interventions, such as conservation programs, pollution control policies, or renewable energy initiatives.
When to use Quasi-Experimental Design
Here are some situations where quasi-experimental designs may be appropriate:
- When the research question involves investigating the effectiveness of an intervention, policy, or program : In situations where it is not feasible or ethical to randomly assign participants to intervention and control groups, quasi-experimental designs can be used to evaluate the impact of the intervention on the targeted outcome.
- When the sample size is small: In situations where the sample size is small, it may be difficult to randomly assign participants to intervention and control groups. Quasi-experimental designs can be used to investigate the impact of an intervention without requiring a large sample size.
- When the research question involves investigating a naturally occurring event : In some situations, researchers may be interested in investigating the impact of a naturally occurring event, such as a natural disaster or a major policy change. Quasi-experimental designs can be used to evaluate the impact of the event on the targeted outcome.
- When the research question involves investigating a long-term intervention: In situations where the intervention or program is long-term, it may be difficult to randomly assign participants to intervention and control groups for the entire duration of the intervention. Quasi-experimental designs can be used to evaluate the impact of the intervention over time.
- When the research question involves investigating the impact of a variable that cannot be manipulated : In some situations, it may not be possible or ethical to manipulate a variable of interest. Quasi-experimental designs can be used to investigate the relationship between the variable and the targeted outcome.
Purpose of Quasi-Experimental Design
The purpose of quasi-experimental design is to investigate the causal relationship between two or more variables when it is not feasible or ethical to conduct a randomized controlled trial (RCT). Quasi-experimental designs attempt to emulate the randomized control trial by mimicking the control group and the intervention group as much as possible.
The key purpose of quasi-experimental design is to evaluate the impact of an intervention, policy, or program on a targeted outcome while controlling for potential confounding factors that may affect the outcome. Quasi-experimental designs aim to answer questions such as: Did the intervention cause the change in the outcome? Would the outcome have changed without the intervention? And was the intervention effective in achieving its intended goals?
Quasi-experimental designs are useful in situations where randomized controlled trials are not feasible or ethical. They provide researchers with an alternative method to evaluate the effectiveness of interventions, policies, and programs in real-life settings. Quasi-experimental designs can also help inform policy and practice by providing valuable insights into the causal relationships between variables.
Overall, the purpose of quasi-experimental design is to provide a rigorous method for evaluating the impact of interventions, policies, and programs while controlling for potential confounding factors that may affect the outcome.
Advantages of Quasi-Experimental Design
Quasi-experimental designs have several advantages over other research designs, such as:
- Greater external validity : Quasi-experimental designs are more likely to have greater external validity than laboratory experiments because they are conducted in naturalistic settings. This means that the results are more likely to generalize to real-world situations.
- Ethical considerations: Quasi-experimental designs often involve naturally occurring events, such as natural disasters or policy changes. This means that researchers do not need to manipulate variables, which can raise ethical concerns.
- More practical: Quasi-experimental designs are often more practical than experimental designs because they are less expensive and easier to conduct. They can also be used to evaluate programs or policies that have already been implemented, which can save time and resources.
- No random assignment: Quasi-experimental designs do not require random assignment, which can be difficult or impossible in some cases, such as when studying the effects of a natural disaster. This means that researchers can still make causal inferences, although they must use statistical techniques to control for potential confounding variables.
- Greater generalizability : Quasi-experimental designs are often more generalizable than experimental designs because they include a wider range of participants and conditions. This can make the results more applicable to different populations and settings.
Limitations of Quasi-Experimental Design
There are several limitations associated with quasi-experimental designs, which include:
- Lack of Randomization: Quasi-experimental designs do not involve randomization of participants into groups, which means that the groups being studied may differ in important ways that could affect the outcome of the study. This can lead to problems with internal validity and limit the ability to make causal inferences.
- Selection Bias: Quasi-experimental designs may suffer from selection bias because participants are not randomly assigned to groups. Participants may self-select into groups or be assigned based on pre-existing characteristics, which may introduce bias into the study.
- History and Maturation: Quasi-experimental designs are susceptible to history and maturation effects, where the passage of time or other events may influence the outcome of the study.
- Lack of Control: Quasi-experimental designs may lack control over extraneous variables that could influence the outcome of the study. This can limit the ability to draw causal inferences from the study.
- Limited Generalizability: Quasi-experimental designs may have limited generalizability because the results may only apply to the specific population and context being studied.
About the author
Muhammad Hassan
Researcher, Academic Writer, Web developer
You may also like

One-to-One Interview – Methods and Guide

Descriptive Research Design – Types, Methods and...

Research Methods – Types, Examples and Guide

Correlational Research – Methods, Types and...

Basic Research – Types, Methods and Examples

Case Study – Methods, Examples and Guide

Want to create or adapt books like this? Learn more about how Pressbooks supports open publishing practices.
7.3 Quasi-Experimental Research
Learning objectives.
- Explain what quasi-experimental research is and distinguish it clearly from both experimental and correlational research.
- Describe three different types of quasi-experimental research designs (nonequivalent groups, pretest-posttest, and interrupted time series) and identify examples of each one.
The prefix quasi means “resembling.” Thus quasi-experimental research is research that resembles experimental research but is not true experimental research. Although the independent variable is manipulated, participants are not randomly assigned to conditions or orders of conditions (Cook & Campbell, 1979). Because the independent variable is manipulated before the dependent variable is measured, quasi-experimental research eliminates the directionality problem. But because participants are not randomly assigned—making it likely that there are other differences between conditions—quasi-experimental research does not eliminate the problem of confounding variables. In terms of internal validity, therefore, quasi-experiments are generally somewhere between correlational studies and true experiments.
Quasi-experiments are most likely to be conducted in field settings in which random assignment is difficult or impossible. They are often conducted to evaluate the effectiveness of a treatment—perhaps a type of psychotherapy or an educational intervention. There are many different kinds of quasi-experiments, but we will discuss just a few of the most common ones here.
Nonequivalent Groups Design
Recall that when participants in a between-subjects experiment are randomly assigned to conditions, the resulting groups are likely to be quite similar. In fact, researchers consider them to be equivalent. When participants are not randomly assigned to conditions, however, the resulting groups are likely to be dissimilar in some ways. For this reason, researchers consider them to be nonequivalent. A nonequivalent groups design , then, is a between-subjects design in which participants have not been randomly assigned to conditions.
Imagine, for example, a researcher who wants to evaluate a new method of teaching fractions to third graders. One way would be to conduct a study with a treatment group consisting of one class of third-grade students and a control group consisting of another class of third-grade students. This would be a nonequivalent groups design because the students are not randomly assigned to classes by the researcher, which means there could be important differences between them. For example, the parents of higher achieving or more motivated students might have been more likely to request that their children be assigned to Ms. Williams’s class. Or the principal might have assigned the “troublemakers” to Mr. Jones’s class because he is a stronger disciplinarian. Of course, the teachers’ styles, and even the classroom environments, might be very different and might cause different levels of achievement or motivation among the students. If at the end of the study there was a difference in the two classes’ knowledge of fractions, it might have been caused by the difference between the teaching methods—but it might have been caused by any of these confounding variables.
Of course, researchers using a nonequivalent groups design can take steps to ensure that their groups are as similar as possible. In the present example, the researcher could try to select two classes at the same school, where the students in the two classes have similar scores on a standardized math test and the teachers are the same sex, are close in age, and have similar teaching styles. Taking such steps would increase the internal validity of the study because it would eliminate some of the most important confounding variables. But without true random assignment of the students to conditions, there remains the possibility of other important confounding variables that the researcher was not able to control.
Pretest-Posttest Design
In a pretest-posttest design , the dependent variable is measured once before the treatment is implemented and once after it is implemented. Imagine, for example, a researcher who is interested in the effectiveness of an antidrug education program on elementary school students’ attitudes toward illegal drugs. The researcher could measure the attitudes of students at a particular elementary school during one week, implement the antidrug program during the next week, and finally, measure their attitudes again the following week. The pretest-posttest design is much like a within-subjects experiment in which each participant is tested first under the control condition and then under the treatment condition. It is unlike a within-subjects experiment, however, in that the order of conditions is not counterbalanced because it typically is not possible for a participant to be tested in the treatment condition first and then in an “untreated” control condition.
If the average posttest score is better than the average pretest score, then it makes sense to conclude that the treatment might be responsible for the improvement. Unfortunately, one often cannot conclude this with a high degree of certainty because there may be other explanations for why the posttest scores are better. One category of alternative explanations goes under the name of history . Other things might have happened between the pretest and the posttest. Perhaps an antidrug program aired on television and many of the students watched it, or perhaps a celebrity died of a drug overdose and many of the students heard about it. Another category of alternative explanations goes under the name of maturation . Participants might have changed between the pretest and the posttest in ways that they were going to anyway because they are growing and learning. If it were a yearlong program, participants might become less impulsive or better reasoners and this might be responsible for the change.
Another alternative explanation for a change in the dependent variable in a pretest-posttest design is regression to the mean . This refers to the statistical fact that an individual who scores extremely on a variable on one occasion will tend to score less extremely on the next occasion. For example, a bowler with a long-term average of 150 who suddenly bowls a 220 will almost certainly score lower in the next game. Her score will “regress” toward her mean score of 150. Regression to the mean can be a problem when participants are selected for further study because of their extreme scores. Imagine, for example, that only students who scored especially low on a test of fractions are given a special training program and then retested. Regression to the mean all but guarantees that their scores will be higher even if the training program has no effect. A closely related concept—and an extremely important one in psychological research—is spontaneous remission . This is the tendency for many medical and psychological problems to improve over time without any form of treatment. The common cold is a good example. If one were to measure symptom severity in 100 common cold sufferers today, give them a bowl of chicken soup every day, and then measure their symptom severity again in a week, they would probably be much improved. This does not mean that the chicken soup was responsible for the improvement, however, because they would have been much improved without any treatment at all. The same is true of many psychological problems. A group of severely depressed people today is likely to be less depressed on average in 6 months. In reviewing the results of several studies of treatments for depression, researchers Michael Posternak and Ivan Miller found that participants in waitlist control conditions improved an average of 10 to 15% before they received any treatment at all (Posternak & Miller, 2001). Thus one must generally be very cautious about inferring causality from pretest-posttest designs.
Does Psychotherapy Work?
Early studies on the effectiveness of psychotherapy tended to use pretest-posttest designs. In a classic 1952 article, researcher Hans Eysenck summarized the results of 24 such studies showing that about two thirds of patients improved between the pretest and the posttest (Eysenck, 1952). But Eysenck also compared these results with archival data from state hospital and insurance company records showing that similar patients recovered at about the same rate without receiving psychotherapy. This suggested to Eysenck that the improvement that patients showed in the pretest-posttest studies might be no more than spontaneous remission. Note that Eysenck did not conclude that psychotherapy was ineffective. He merely concluded that there was no evidence that it was, and he wrote of “the necessity of properly planned and executed experimental studies into this important field” (p. 323). You can read the entire article here:
http://psychclassics.yorku.ca/Eysenck/psychotherapy.htm
Fortunately, many other researchers took up Eysenck’s challenge, and by 1980 hundreds of experiments had been conducted in which participants were randomly assigned to treatment and control conditions, and the results were summarized in a classic book by Mary Lee Smith, Gene Glass, and Thomas Miller (Smith, Glass, & Miller, 1980). They found that overall psychotherapy was quite effective, with about 80% of treatment participants improving more than the average control participant. Subsequent research has focused more on the conditions under which different types of psychotherapy are more or less effective.

In a classic 1952 article, researcher Hans Eysenck pointed out the shortcomings of the simple pretest-posttest design for evaluating the effectiveness of psychotherapy.
Wikimedia Commons – CC BY-SA 3.0.
Interrupted Time Series Design
A variant of the pretest-posttest design is the interrupted time-series design . A time series is a set of measurements taken at intervals over a period of time. For example, a manufacturing company might measure its workers’ productivity each week for a year. In an interrupted time series-design, a time series like this is “interrupted” by a treatment. In one classic example, the treatment was the reduction of the work shifts in a factory from 10 hours to 8 hours (Cook & Campbell, 1979). Because productivity increased rather quickly after the shortening of the work shifts, and because it remained elevated for many months afterward, the researcher concluded that the shortening of the shifts caused the increase in productivity. Notice that the interrupted time-series design is like a pretest-posttest design in that it includes measurements of the dependent variable both before and after the treatment. It is unlike the pretest-posttest design, however, in that it includes multiple pretest and posttest measurements.
Figure 7.5 “A Hypothetical Interrupted Time-Series Design” shows data from a hypothetical interrupted time-series study. The dependent variable is the number of student absences per week in a research methods course. The treatment is that the instructor begins publicly taking attendance each day so that students know that the instructor is aware of who is present and who is absent. The top panel of Figure 7.5 “A Hypothetical Interrupted Time-Series Design” shows how the data might look if this treatment worked. There is a consistently high number of absences before the treatment, and there is an immediate and sustained drop in absences after the treatment. The bottom panel of Figure 7.5 “A Hypothetical Interrupted Time-Series Design” shows how the data might look if this treatment did not work. On average, the number of absences after the treatment is about the same as the number before. This figure also illustrates an advantage of the interrupted time-series design over a simpler pretest-posttest design. If there had been only one measurement of absences before the treatment at Week 7 and one afterward at Week 8, then it would have looked as though the treatment were responsible for the reduction. The multiple measurements both before and after the treatment suggest that the reduction between Weeks 7 and 8 is nothing more than normal week-to-week variation.
Figure 7.5 A Hypothetical Interrupted Time-Series Design

The top panel shows data that suggest that the treatment caused a reduction in absences. The bottom panel shows data that suggest that it did not.
Combination Designs
A type of quasi-experimental design that is generally better than either the nonequivalent groups design or the pretest-posttest design is one that combines elements of both. There is a treatment group that is given a pretest, receives a treatment, and then is given a posttest. But at the same time there is a control group that is given a pretest, does not receive the treatment, and then is given a posttest. The question, then, is not simply whether participants who receive the treatment improve but whether they improve more than participants who do not receive the treatment.
Imagine, for example, that students in one school are given a pretest on their attitudes toward drugs, then are exposed to an antidrug program, and finally are given a posttest. Students in a similar school are given the pretest, not exposed to an antidrug program, and finally are given a posttest. Again, if students in the treatment condition become more negative toward drugs, this could be an effect of the treatment, but it could also be a matter of history or maturation. If it really is an effect of the treatment, then students in the treatment condition should become more negative than students in the control condition. But if it is a matter of history (e.g., news of a celebrity drug overdose) or maturation (e.g., improved reasoning), then students in the two conditions would be likely to show similar amounts of change. This type of design does not completely eliminate the possibility of confounding variables, however. Something could occur at one of the schools but not the other (e.g., a student drug overdose), so students at the first school would be affected by it while students at the other school would not.
Finally, if participants in this kind of design are randomly assigned to conditions, it becomes a true experiment rather than a quasi experiment. In fact, it is the kind of experiment that Eysenck called for—and that has now been conducted many times—to demonstrate the effectiveness of psychotherapy.
Key Takeaways
- Quasi-experimental research involves the manipulation of an independent variable without the random assignment of participants to conditions or orders of conditions. Among the important types are nonequivalent groups designs, pretest-posttest, and interrupted time-series designs.
- Quasi-experimental research eliminates the directionality problem because it involves the manipulation of the independent variable. It does not eliminate the problem of confounding variables, however, because it does not involve random assignment to conditions. For these reasons, quasi-experimental research is generally higher in internal validity than correlational studies but lower than true experiments.
- Practice: Imagine that two college professors decide to test the effect of giving daily quizzes on student performance in a statistics course. They decide that Professor A will give quizzes but Professor B will not. They will then compare the performance of students in their two sections on a common final exam. List five other variables that might differ between the two sections that could affect the results.
Discussion: Imagine that a group of obese children is recruited for a study in which their weight is measured, then they participate for 3 months in a program that encourages them to be more active, and finally their weight is measured again. Explain how each of the following might affect the results:
- regression to the mean
- spontaneous remission
Cook, T. D., & Campbell, D. T. (1979). Quasi-experimentation: Design & analysis issues in field settings . Boston, MA: Houghton Mifflin.
Eysenck, H. J. (1952). The effects of psychotherapy: An evaluation. Journal of Consulting Psychology, 16 , 319–324.
Posternak, M. A., & Miller, I. (2001). Untreated short-term course of major depression: A meta-analysis of studies using outcomes from studies using wait-list control groups. Journal of Affective Disorders, 66 , 139–146.
Smith, M. L., Glass, G. V., & Miller, T. I. (1980). The benefits of psychotherapy . Baltimore, MD: Johns Hopkins University Press.
Research Methods in Psychology Copyright © 2016 by University of Minnesota is licensed under a Creative Commons Attribution-NonCommercial-ShareAlike 4.0 International License , except where otherwise noted.
- Skip to secondary menu
- Skip to main content
- Skip to primary sidebar
Statistics By Jim
Making statistics intuitive
Quasi Experimental Design Overview & Examples
By Jim Frost Leave a Comment
What is a Quasi Experimental Design?
A quasi experimental design is a method for identifying causal relationships that does not randomly assign participants to the experimental groups. Instead, researchers use a non-random process. For example, they might use an eligibility cutoff score or preexisting groups to determine who receives the treatment.

Quasi-experimental research is a design that closely resembles experimental research but is different. The term “quasi” means “resembling,” so you can think of it as a cousin to actual experiments. In these studies, researchers can manipulate an independent variable — that is, they change one factor to see what effect it has. However, unlike true experimental research, participants are not randomly assigned to different groups.
Learn more about Experimental Designs: Definition & Types .
When to Use Quasi-Experimental Design
Researchers typically use a quasi-experimental design because they can’t randomize due to practical or ethical concerns. For example:
- Practical Constraints : A school interested in testing a new teaching method can only implement it in preexisting classes and cannot randomly assign students.
- Ethical Concerns : A medical study might not be able to randomly assign participants to a treatment group for an experimental medication when they are already taking a proven drug.
Quasi-experimental designs also come in handy when researchers want to study the effects of naturally occurring events, like policy changes or environmental shifts, where they can’t control who is exposed to the treatment.
Quasi-experimental designs occupy a unique position in the spectrum of research methodologies, sitting between observational studies and true experiments. This middle ground offers a blend of both worlds, addressing some limitations of purely observational studies while navigating the constraints often accompanying true experiments.
A significant advantage of quasi-experimental research over purely observational studies and correlational research is that it addresses the issue of directionality, determining which variable is the cause and which is the effect. In quasi-experiments, an intervention typically occurs during the investigation, and the researchers record outcomes before and after it, increasing the confidence that it causes the observed changes.
However, it’s crucial to recognize its limitations as well. Controlling confounding variables is a larger concern for a quasi-experimental design than a true experiment because it lacks random assignment.
In sum, quasi-experimental designs offer a valuable research approach when random assignment is not feasible, providing a more structured and controlled framework than observational studies while acknowledging and attempting to address potential confounders.
Types of Quasi-Experimental Designs and Examples
Quasi-experimental studies use various methods, depending on the scenario.
Natural Experiments
This design uses naturally occurring events or changes to create the treatment and control groups. Researchers compare outcomes between those whom the event affected and those it did not affect. Analysts use statistical controls to account for confounders that the researchers must also measure.
Natural experiments are related to observational studies, but they allow for a clearer causality inference because the external event or policy change provides both a form of quasi-random group assignment and a definite start date for the intervention.
For example, in a natural experiment utilizing a quasi-experimental design, researchers study the impact of a significant economic policy change on small business growth. The policy is implemented in one state but not in neighboring states. This scenario creates an unplanned experimental setup, where the state with the new policy serves as the treatment group, and the neighboring states act as the control group.
Researchers are primarily interested in small business growth rates but need to record various confounders that can impact growth rates. Hence, they record state economic indicators, investment levels, and employment figures. By recording these metrics across the states, they can include them in the model as covariates and control them statistically. This method allows researchers to estimate differences in small business growth due to the policy itself, separate from the various confounders.
Nonequivalent Groups Design
This method involves matching existing groups that are similar but not identical. Researchers attempt to find groups that are as equivalent as possible, particularly for factors likely to affect the outcome.
For instance, researchers use a nonequivalent groups quasi-experimental design to evaluate the effectiveness of a new teaching method in improving students’ mathematics performance. A school district considering the teaching method is planning the study. Students are already divided into schools, preventing random assignment.
The researchers matched two schools with similar demographics, baseline academic performance, and resources. The school using the traditional methodology is the control, while the other uses the new approach. Researchers are evaluating differences in educational outcomes between the two methods.
They perform a pretest to identify differences between the schools that might affect the outcome and include them as covariates to control for confounding. They also record outcomes before and after the intervention to have a larger context for the changes they observe.
Regression Discontinuity
This process assigns subjects to a treatment or control group based on a predetermined cutoff point (e.g., a test score). The analysis primarily focuses on participants near the cutoff point, as they are likely similar except for the treatment received. By comparing participants just above and below the cutoff, the design controls for confounders that vary smoothly around the cutoff.
For example, in a regression discontinuity quasi-experimental design focusing on a new medical treatment for depression, researchers use depression scores as the cutoff point. Individuals with depression scores just above a certain threshold are assigned to receive the latest treatment, while those just below the threshold do not receive it. This method creates two closely matched groups: one that barely qualifies for treatment and one that barely misses out.
By comparing the mental health outcomes of these two groups over time, researchers can assess the effectiveness of the new treatment. The assumption is that the only significant difference between the groups is whether they received the treatment, thereby isolating its impact on depression outcomes.
Controlling Confounders in a Quasi-Experimental Design
Accounting for confounding variables is a challenging but essential task for a quasi-experimental design.
In a true experiment, the random assignment process equalizes confounders across the groups to nullify their overall effect. It’s the gold standard because it works on all confounders, known and unknown.
Unfortunately, the lack of random assignment can allow differences between the groups to exist before the intervention. These confounding factors might ultimately explain the results rather than the intervention.
Consequently, researchers must use other methods to equalize the groups roughly using matching and cutoff values or statistically adjust for preexisting differences they measure to reduce the impact of confounders.
A key strength of quasi-experiments is their frequent use of “pre-post testing.” This approach involves conducting initial tests before collecting data to check for preexisting differences between groups that could impact the study’s outcome. By identifying these variables early on and including them as covariates, researchers can more effectively control potential confounders in their statistical analysis.
Additionally, researchers frequently track outcomes before and after the intervention to better understand the context for changes they observe.
Statisticians consider these methods to be less effective than randomization. Hence, quasi-experiments fall somewhere in the middle when it comes to internal validity , or how well the study can identify causal relationships versus mere correlation . They’re more conclusive than correlational studies but not as solid as true experiments.
In conclusion, quasi-experimental designs offer researchers a versatile and practical approach when random assignment is not feasible. This methodology bridges the gap between controlled experiments and observational studies, providing a valuable tool for investigating cause-and-effect relationships in real-world settings. Researchers can address ethical and logistical constraints by understanding and leveraging the different types of quasi-experimental designs while still obtaining insightful and meaningful results.
Cook, T. D., & Campbell, D. T. (1979). Quasi-experimentation: Design & analysis issues in field settings . Boston, MA: Houghton Mifflin
Share this:

Reader Interactions
Comments and questions cancel reply.
- Skip to main content
- Skip to primary sidebar
- Skip to footer
- QuestionPro

- Solutions Industries Gaming Automotive Sports and events Education Government Travel & Hospitality Financial Services Healthcare Cannabis Technology Use Case AskWhy Communities Audience Contactless surveys Mobile LivePolls Member Experience GDPR Positive People Science 360 Feedback Surveys
- Resources Blog eBooks Survey Templates Case Studies Training Help center

Home Market Research Research Tools and Apps
Quasi-experimental Research: What It Is, Types & Examples
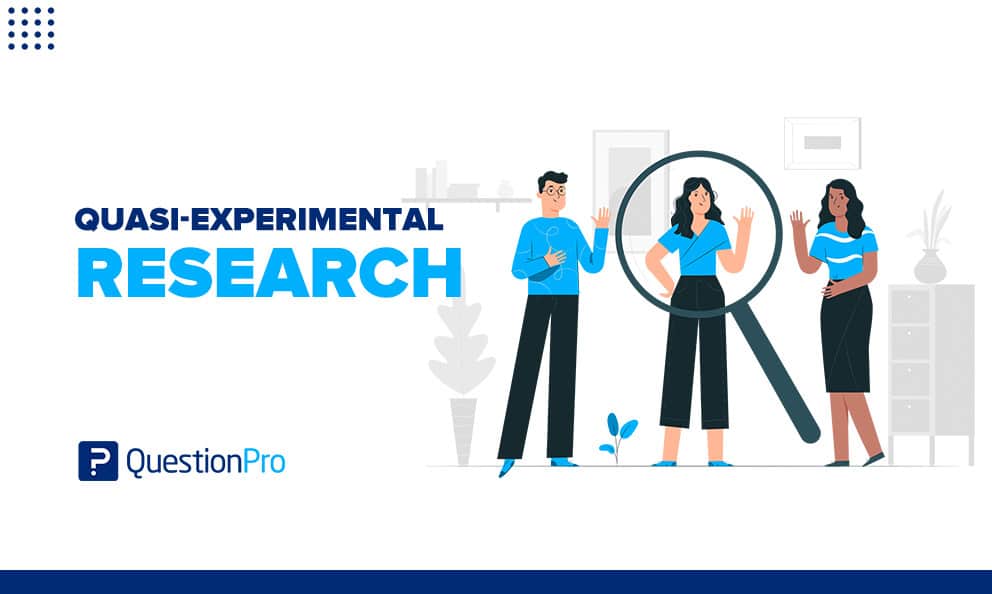
Much like an actual experiment, quasi-experimental research tries to demonstrate a cause-and-effect link between a dependent and an independent variable. A quasi-experiment, on the other hand, does not depend on random assignment, unlike an actual experiment. The subjects are sorted into groups based on non-random variables.
What is Quasi-Experimental Research?
“Resemblance” is the definition of “quasi.” Individuals are not randomly allocated to conditions or orders of conditions, even though the regression analysis is changed. As a result, quasi-experimental research is research that appears to be experimental but is not.
The directionality problem is avoided in quasi-experimental research since the regression analysis is altered before the multiple regression is assessed. However, because individuals are not randomized at random, there are likely to be additional disparities across conditions in quasi-experimental research.
As a result, in terms of internal consistency, quasi-experiments fall somewhere between correlational research and actual experiments.
The key component of a true experiment is randomly allocated groups. This means that each person has an equivalent chance of being assigned to the experimental group or the control group, depending on whether they are manipulated or not.
Simply put, a quasi-experiment is not a real experiment. A quasi-experiment does not feature randomly allocated groups since the main component of a real experiment is randomly assigned groups. Why is it so crucial to have randomly allocated groups, given that they constitute the only distinction between quasi-experimental and actual experimental research ?
Let’s use an example to illustrate our point. Let’s assume we want to discover how new psychological therapy affects depressed patients. In a genuine trial, you’d split half of the psych ward into treatment groups, With half getting the new psychotherapy therapy and the other half receiving standard depression treatment .
And the physicians compare the outcomes of this treatment to the results of standard treatments to see if this treatment is more effective. Doctors, on the other hand, are unlikely to agree with this genuine experiment since they believe it is unethical to treat one group while leaving another untreated.
A quasi-experimental study will be useful in this case. Instead of allocating these patients at random, you uncover pre-existing psychotherapist groups in the hospitals. Clearly, there’ll be counselors who are eager to undertake these trials as well as others who prefer to stick to the old ways.
These pre-existing groups can be used to compare the symptom development of individuals who received the novel therapy with those who received the normal course of treatment, even though the groups weren’t chosen at random.
If any substantial variations between them can be well explained, you may be very assured that any differences are attributable to the treatment but not to other extraneous variables.
As we mentioned before, quasi-experimental research entails manipulating an independent variable by randomly assigning people to conditions or sequences of conditions. Non-equivalent group designs, pretest-posttest designs, and regression discontinuity designs are only a few of the essential types.
What are quasi-experimental research designs?
Quasi-experimental research designs are a type of research design that is similar to experimental designs but doesn’t give full control over the independent variable(s) like true experimental designs do.
In a quasi-experimental design, the researcher changes or watches an independent variable, but the participants are not put into groups at random. Instead, people are put into groups based on things they already have in common, like their age, gender, or how many times they have seen a certain stimulus.
Because the assignments are not random, it is harder to draw conclusions about cause and effect than in a real experiment. However, quasi-experimental designs are still useful when randomization is not possible or ethical.
The true experimental design may be impossible to accomplish or just too expensive, especially for researchers with few resources. Quasi-experimental designs enable you to investigate an issue by utilizing data that has already been paid for or gathered by others (often the government).
Because they allow better control for confounding variables than other forms of studies, they have higher external validity than most genuine experiments and higher internal validity (less than true experiments) than other non-experimental research.
Is quasi-experimental research quantitative or qualitative?
Quasi-experimental research is a quantitative research method. It involves numerical data collection and statistical analysis. Quasi-experimental research compares groups with different circumstances or treatments to find cause-and-effect links.
It draws statistical conclusions from quantitative data. Qualitative data can enhance quasi-experimental research by revealing participants’ experiences and opinions, but quantitative data is the method’s foundation.
Quasi-experimental research types
There are many different sorts of quasi-experimental designs. Three of the most popular varieties are described below: Design of non-equivalent groups, Discontinuity in regression, and Natural experiments.
Design of Non-equivalent Groups
Example: design of non-equivalent groups, discontinuity in regression, example: discontinuity in regression, natural experiments, example: natural experiments.
However, because they couldn’t afford to pay everyone who qualified for the program, they had to use a random lottery to distribute slots.
Experts were able to investigate the program’s impact by utilizing enrolled people as a treatment group and those who were qualified but did not play the jackpot as an experimental group.
How QuestionPro helps in quasi-experimental research?
QuestionPro can be a useful tool in quasi-experimental research because it includes features that can assist you in designing and analyzing your research study. Here are some ways in which QuestionPro can help in quasi-experimental research:
Design surveys
Randomize participants, collect data over time, analyze data, collaborate with your team.
With QuestionPro, you have access to the most mature market research platform and tool that helps you collect and analyze the insights that matter the most. By leveraging InsightsHub, the unified hub for data management, you can leverage the consolidated platform to organize, explore, search, and discover your research data in one organized data repository .
Optimize Your quasi-experimental research with QuestionPro. Get started now!
LEARN MORE FREE TRIAL
MORE LIKE THIS
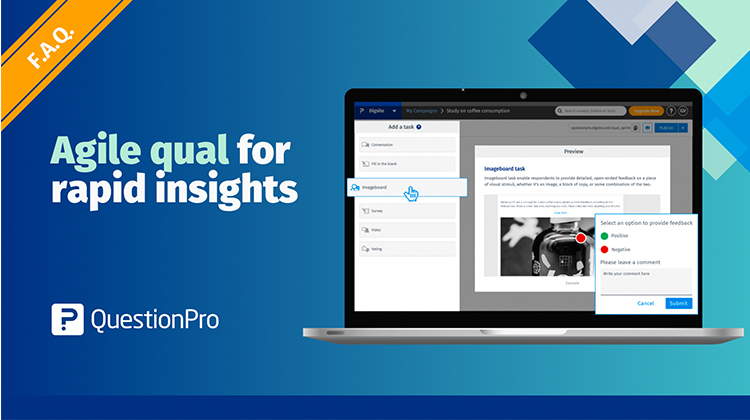
A guide to conducting agile qualitative research for rapid insights with Digsite
Sep 11, 2024
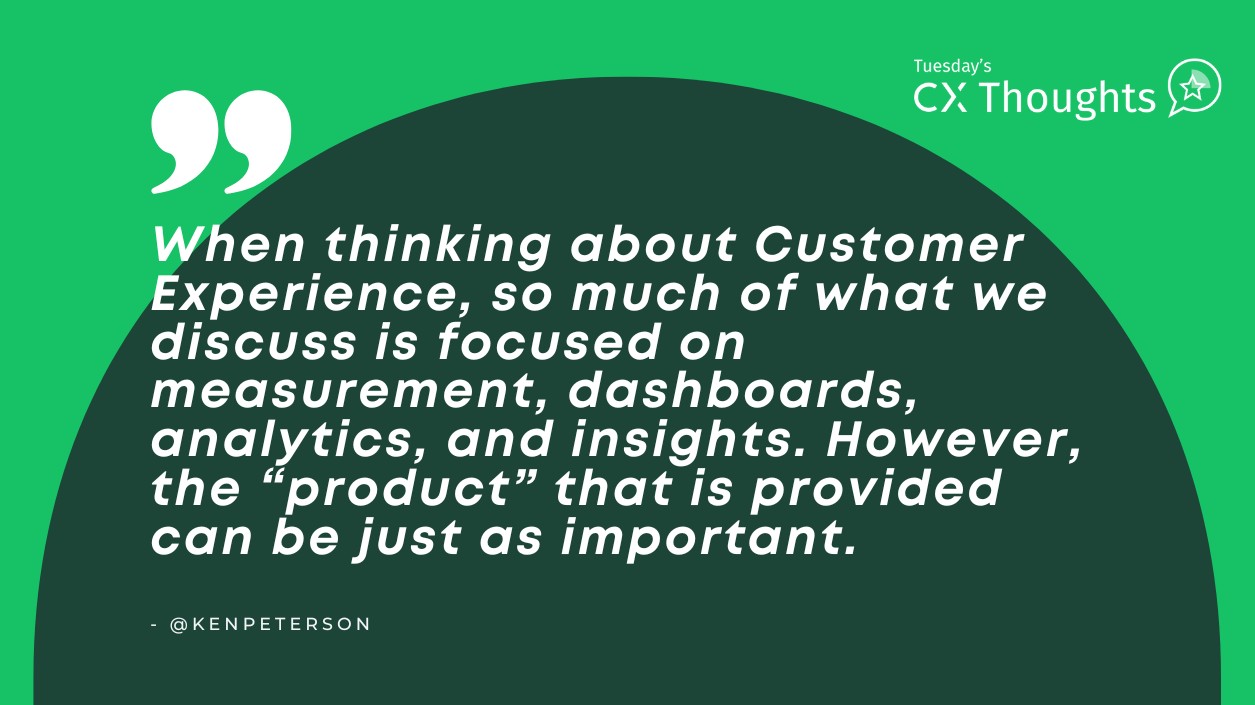
Was The Experience Memorable? — Tuesday CX Thoughts
Sep 10, 2024
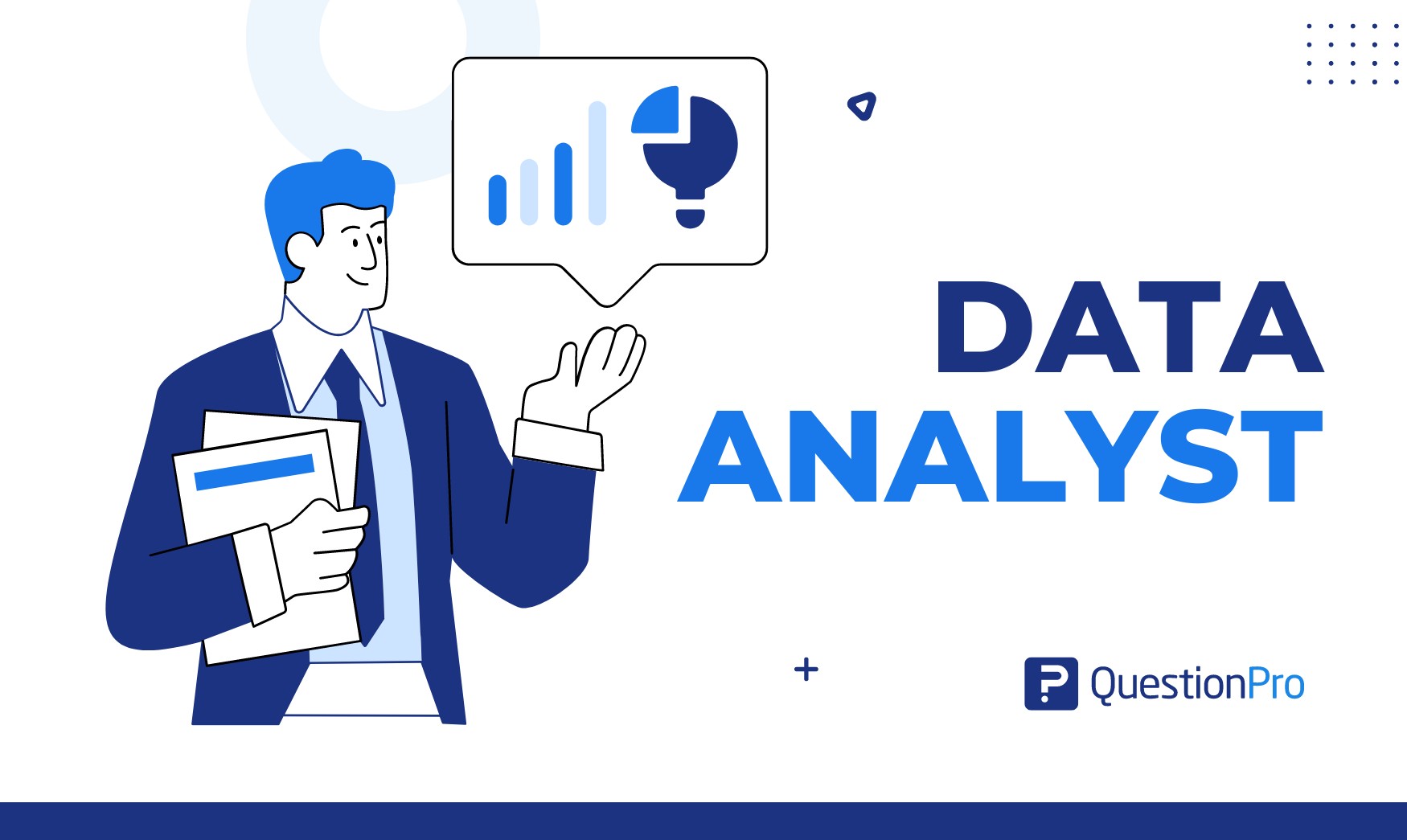
What Does a Data Analyst Do? Skills, Tools & Tips
Sep 9, 2024
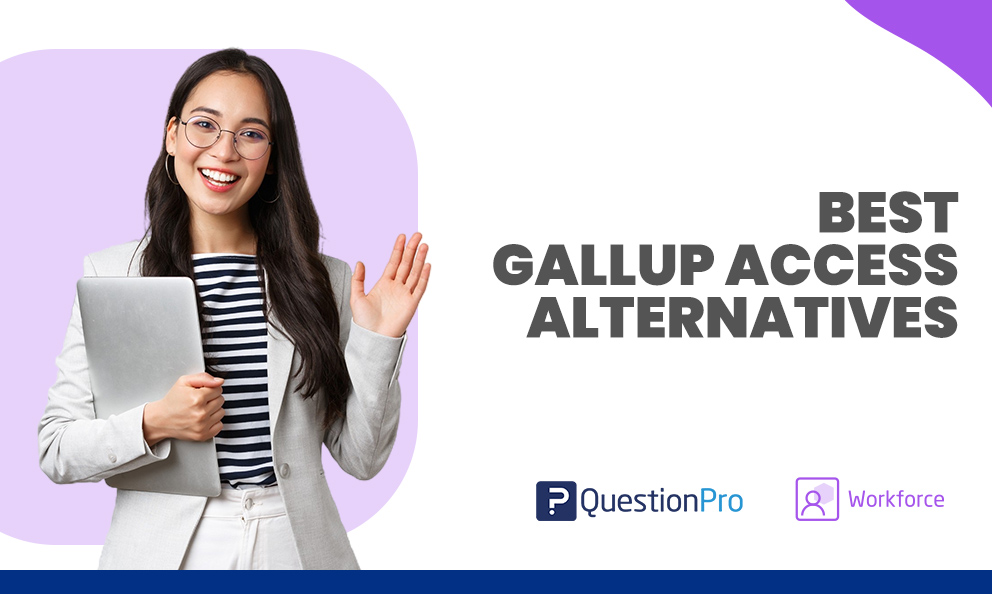
Best Gallup Access Alternatives & Competitors in 2024
Sep 6, 2024
Other categories
- Academic Research
- Artificial Intelligence
- Assessments
- Brand Awareness
- Case Studies
- Communities
- Consumer Insights
- Customer effort score
- Customer Engagement
- Customer Experience
- Customer Loyalty
- Customer Research
- Customer Satisfaction
- Employee Benefits
- Employee Engagement
- Employee Retention
- Friday Five
- General Data Protection Regulation
- Insights Hub
- Life@QuestionPro
- Market Research
- Mobile diaries
- Mobile Surveys
- New Features
- Online Communities
- Question Types
- Questionnaire
- QuestionPro Products
- Release Notes
- Research Tools and Apps
- Revenue at Risk
- Survey Templates
- Training Tips
- Tuesday CX Thoughts (TCXT)
- Uncategorized
- What’s Coming Up
- Workforce Intelligence
Instant insights, infinite possibilities
The use and interpretation of quasi-experimental design
Last updated
6 February 2023
Reviewed by
Miroslav Damyanov
Short on time? Get an AI generated summary of this article instead
- What is a quasi-experimental design?
Commonly used in medical informatics (a field that uses digital information to ensure better patient care), researchers generally use this design to evaluate the effectiveness of a treatment – perhaps a type of antibiotic or psychotherapy, or an educational or policy intervention.
Even though quasi-experimental design has been used for some time, relatively little is known about it. Read on to learn the ins and outs of this research design.
Make research less tedious
Dovetail streamlines research to help you uncover and share actionable insights
- When to use a quasi-experimental design
A quasi-experimental design is used when it's not logistically feasible or ethical to conduct randomized, controlled trials. As its name suggests, a quasi-experimental design is almost a true experiment. However, researchers don't randomly select elements or participants in this type of research.
Researchers prefer to apply quasi-experimental design when there are ethical or practical concerns. Let's look at these two reasons more closely.
Ethical reasons
In some situations, the use of randomly assigned elements can be unethical. For instance, providing public healthcare to one group and withholding it to another in research is unethical. A quasi-experimental design would examine the relationship between these two groups to avoid physical danger.
Practical reasons
Randomized controlled trials may not be the best approach in research. For instance, it's impractical to trawl through large sample sizes of participants without using a particular attribute to guide your data collection .
Recruiting participants and properly designing a data-collection attribute to make the research a true experiment requires a lot of time and effort, and can be expensive if you don’t have a large funding stream.
A quasi-experimental design allows researchers to take advantage of previously collected data and use it in their study.
- Examples of quasi-experimental designs
Quasi-experimental research design is common in medical research, but any researcher can use it for research that raises practical and ethical concerns. Here are a few examples of quasi-experimental designs used by different researchers:
Example 1: Determining the effectiveness of math apps in supplementing math classes
A school wanted to supplement its math classes with a math app. To select the best app, the school decided to conduct demo tests on two apps before selecting the one they will purchase.
Scope of the research
Since every grade had two math teachers, each teacher used one of the two apps for three months. They then gave the students the same math exams and compared the results to determine which app was most effective.
Reasons why this is a quasi-experimental study
This simple study is a quasi-experiment since the school didn't randomly assign its students to the applications. They used a pre-existing class structure to conduct the study since it was impractical to randomly assign the students to each app.
Example 2: Determining the effectiveness of teaching modern leadership techniques in start-up businesses
A hypothetical quasi-experimental study was conducted in an economically developing country in a mid-sized city.
Five start-ups in the textile industry and five in the tech industry participated in the study. The leaders attended a six-week workshop on leadership style, team management, and employee motivation.
After a year, the researchers assessed the performance of each start-up company to determine growth. The results indicated that the tech start-ups were further along in their growth than the textile companies.
The basis of quasi-experimental research is a non-randomized subject-selection process. This study didn't use specific aspects to determine which start-up companies should participate. Therefore, the results may seem straightforward, but several aspects may determine the growth of a specific company, apart from the variables used by the researchers.
Example 3: A study to determine the effects of policy reforms and of luring foreign investment on small businesses in two mid-size cities
In a study to determine the economic impact of government reforms in an economically developing country, the government decided to test whether creating reforms directed at small businesses or luring foreign investments would spur the most economic development.
The government selected two cities with similar population demographics and sizes. In one of the cities, they implemented specific policies that would directly impact small businesses, and in the other, they implemented policies to attract foreign investment.
After five years, they collected end-of-year economic growth data from both cities. They looked at elements like local GDP growth, unemployment rates, and housing sales.
The study used a non-randomized selection process to determine which city would participate in the research. Researchers left out certain variables that would play a crucial role in determining the growth of each city. They used pre-existing groups of people based on research conducted in each city, rather than random groups.
- Advantages of a quasi-experimental design
Some advantages of quasi-experimental designs are:
Researchers can manipulate variables to help them meet their study objectives.
It offers high external validity, making it suitable for real-world applications, specifically in social science experiments.
Integrating this methodology into other research designs is easier, especially in true experimental research. This cuts down on the time needed to determine your outcomes.
- Disadvantages of a quasi-experimental design
Despite the pros that come with a quasi-experimental design, there are several disadvantages associated with it, including the following:
It has a lower internal validity since researchers do not have full control over the comparison and intervention groups or between time periods because of differences in characteristics in people, places, or time involved. It may be challenging to determine whether all variables have been used or whether those used in the research impacted the results.
There is the risk of inaccurate data since the research design borrows information from other studies.
There is the possibility of bias since researchers select baseline elements and eligibility.
- What are the different quasi-experimental study designs?
There are three distinct types of quasi-experimental designs:
Nonequivalent
Regression discontinuity, natural experiment.
This is a hybrid of experimental and quasi-experimental methods and is used to leverage the best qualities of the two. Like the true experiment design, nonequivalent group design uses pre-existing groups believed to be comparable. However, it doesn't use randomization, the lack of which is a crucial element for quasi-experimental design.
Researchers usually ensure that no confounding variables impact them throughout the grouping process. This makes the groupings more comparable.
Example of a nonequivalent group design
A small study was conducted to determine whether after-school programs result in better grades. Researchers randomly selected two groups of students: one to implement the new program, the other not to. They then compared the results of the two groups.
This type of quasi-experimental research design calculates the impact of a specific treatment or intervention. It uses a criterion known as "cutoff" that assigns treatment according to eligibility.
Researchers often assign participants above the cutoff to the treatment group. This puts a negligible distinction between the two groups (treatment group and control group).
Example of regression discontinuity
Students must achieve a minimum score to be enrolled in specific US high schools. Since the cutoff score used to determine eligibility for enrollment is arbitrary, researchers can assume that the disparity between students who only just fail to achieve the cutoff point and those who barely pass is a small margin and is due to the difference in the schools that these students attend.
Researchers can then examine the long-term effects of these two groups of kids to determine the effect of attending certain schools. This information can be applied to increase the chances of students being enrolled in these high schools.
This research design is common in laboratory and field experiments where researchers control target subjects by assigning them to different groups. Researchers randomly assign subjects to a treatment group using nature or an external event or situation.
However, even with random assignment, this research design cannot be called a true experiment since nature aspects are observational. Researchers can also exploit these aspects despite having no control over the independent variables.
Example of the natural experiment approach
An example of a natural experiment is the 2008 Oregon Health Study.
Oregon intended to allow more low-income people to participate in Medicaid.
Since they couldn't afford to cover every person who qualified for the program, the state used a random lottery to allocate program slots.
Researchers assessed the program's effectiveness by assigning the selected subjects to a randomly assigned treatment group, while those that didn't win the lottery were considered the control group.
- Differences between quasi-experiments and true experiments
There are several differences between a quasi-experiment and a true experiment:
Participants in true experiments are randomly assigned to the treatment or control group, while participants in a quasi-experiment are not assigned randomly.
In a quasi-experimental design, the control and treatment groups differ in unknown or unknowable ways, apart from the experimental treatments that are carried out. Therefore, the researcher should try as much as possible to control these differences.
Quasi-experimental designs have several "competing hypotheses," which compete with experimental manipulation to explain the observed results.
Quasi-experiments tend to have lower internal validity (the degree of confidence in the research outcomes) than true experiments, but they may offer higher external validity (whether findings can be extended to other contexts) as they involve real-world interventions instead of controlled interventions in artificial laboratory settings.
Despite the distinct difference between true and quasi-experimental research designs, these two research methodologies share the following aspects:
Both study methods subject participants to some form of treatment or conditions.
Researchers have the freedom to measure some of the outcomes of interest.
Researchers can test whether the differences in the outcomes are associated with the treatment.
- An example comparing a true experiment and quasi-experiment
Imagine you wanted to study the effects of junk food on obese people. Here's how you would do this as a true experiment and a quasi-experiment:
How to carry out a true experiment
In a true experiment, some participants would eat junk foods, while the rest would be in the control group, adhering to a regular diet. At the end of the study, you would record the health and discomfort of each group.
This kind of experiment would raise ethical concerns since the participants assigned to the treatment group are required to eat junk food against their will throughout the experiment. This calls for a quasi-experimental design.
How to carry out a quasi-experiment
In quasi-experimental research, you would start by finding out which participants want to try junk food and which prefer to stick to a regular diet. This allows you to assign these two groups based on subject choice.
In this case, you didn't assign participants to a particular group, so you can confidently use the results from the study.
When is a quasi-experimental design used?
Quasi-experimental designs are used when researchers don’t want to use randomization when evaluating their intervention.
What are the characteristics of quasi-experimental designs?
Some of the characteristics of a quasi-experimental design are:
Researchers don't randomly assign participants into groups, but study their existing characteristics and assign them accordingly.
Researchers study the participants in pre- and post-testing to determine the progress of the groups.
Quasi-experimental design is ethical since it doesn’t involve offering or withholding treatment at random.
Quasi-experimental design encompasses a broad range of non-randomized intervention studies. This design is employed when it is not ethical or logistically feasible to conduct randomized controlled trials. Researchers typically employ it when evaluating policy or educational interventions, or in medical or therapy scenarios.
How do you analyze data in a quasi-experimental design?
You can use two-group tests, time-series analysis, and regression analysis to analyze data in a quasi-experiment design. Each option has specific assumptions, strengths, limitations, and data requirements.
Should you be using a customer insights hub?
Do you want to discover previous research faster?
Do you share your research findings with others?
Do you analyze research data?
Start for free today, add your research, and get to key insights faster
Editor’s picks
Last updated: 18 April 2023
Last updated: 27 February 2023
Last updated: 22 August 2024
Last updated: 5 February 2023
Last updated: 16 August 2024
Last updated: 9 March 2023
Last updated: 30 April 2024
Last updated: 12 December 2023
Last updated: 11 March 2024
Last updated: 4 July 2024
Last updated: 6 March 2024
Last updated: 5 March 2024
Last updated: 13 May 2024
Latest articles
Related topics, .css-je19u9{-webkit-align-items:flex-end;-webkit-box-align:flex-end;-ms-flex-align:flex-end;align-items:flex-end;display:-webkit-box;display:-webkit-flex;display:-ms-flexbox;display:flex;-webkit-flex-direction:row;-ms-flex-direction:row;flex-direction:row;-webkit-box-flex-wrap:wrap;-webkit-flex-wrap:wrap;-ms-flex-wrap:wrap;flex-wrap:wrap;-webkit-box-pack:center;-ms-flex-pack:center;-webkit-justify-content:center;justify-content:center;row-gap:0;text-align:center;max-width:671px;}@media (max-width: 1079px){.css-je19u9{max-width:400px;}.css-je19u9>span{white-space:pre;}}@media (max-width: 799px){.css-je19u9{max-width:400px;}.css-je19u9>span{white-space:pre;}} decide what to .css-1kiodld{max-height:56px;display:-webkit-box;display:-webkit-flex;display:-ms-flexbox;display:flex;-webkit-align-items:center;-webkit-box-align:center;-ms-flex-align:center;align-items:center;}@media (max-width: 1079px){.css-1kiodld{display:none;}} build next, decide what to build next, log in or sign up.
Get started for free
10 September 2024: Due to technical disruption, we are experiencing some delays to publication. We are working to restore services and apologise for the inconvenience. For further updates please visit our website: https://www.cambridge.org/universitypress/about-us/news-and-blogs/cambridge-university-press-publishing-update-following-technical-disruption
We use cookies to distinguish you from other users and to provide you with a better experience on our websites. Close this message to accept cookies or find out how to manage your cookie settings .
Login Alert

- > The Cambridge Handbook of Research Methods and Statistics for the Social and Behavioral Sciences
- > Quasi-Experimental Research
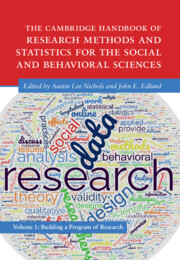
Book contents
- The Cambridge Handbook of Research Methods and Statistics for the Social and Behavioral Sciences
- Cambridge Handbooks in Psychology
- Copyright page
- Contributors
- Part I From Idea to Reality: The Basics of Research
- Part II The Building Blocks of a Study
- Part III Data Collection
- 13 Cross-Sectional Studies
- 14 Quasi-Experimental Research
- 15 Non-equivalent Control Group Pretest–Posttest Design in Social and Behavioral Research
- 16 Experimental Methods
- 17 Longitudinal Research: A World to Explore
- 18 Online Research Methods
- 19 Archival Data
- 20 Qualitative Research Design
- Part IV Statistical Approaches
- Part V Tips for a Successful Research Career
14 - Quasi-Experimental Research
from Part III - Data Collection
Published online by Cambridge University Press: 25 May 2023
In this chapter, we discuss the logic and practice of quasi-experimentation. Specifically, we describe four quasi-experimental designs – one-group pretest–posttest designs, non-equivalent group designs, regression discontinuity designs, and interrupted time-series designs – and their statistical analyses in detail. Both simple quasi-experimental designs and embellishments of these simple designs are presented. Potential threats to internal validity are illustrated along with means of addressing their potentially biasing effects so that these effects can be minimized. In contrast to quasi-experiments, randomized experiments are often thought to be the gold standard when estimating the effects of treatment interventions. However, circumstances frequently arise where quasi-experiments can usefully supplement randomized experiments or when quasi-experiments can fruitfully be used in place of randomized experiments. Researchers need to appreciate the relative strengths and weaknesses of the various quasi-experiments so they can choose among pre-specified designs or craft their own unique quasi-experiments.
Access options
Save book to kindle.
To save this book to your Kindle, first ensure [email protected] is added to your Approved Personal Document E-mail List under your Personal Document Settings on the Manage Your Content and Devices page of your Amazon account. Then enter the ‘name’ part of your Kindle email address below. Find out more about saving to your Kindle .
Note you can select to save to either the @free.kindle.com or @kindle.com variations. ‘@free.kindle.com’ emails are free but can only be saved to your device when it is connected to wi-fi. ‘@kindle.com’ emails can be delivered even when you are not connected to wi-fi, but note that service fees apply.
Find out more about the Kindle Personal Document Service .
- Quasi-Experimental Research
- By Charles S. Reichardt , Daniel Storage , Damon Abraham
- Edited by Austin Lee Nichols , Central European University, Vienna , John Edlund , Rochester Institute of Technology, New York
- Book: The Cambridge Handbook of Research Methods and Statistics for the Social and Behavioral Sciences
- Online publication: 25 May 2023
- Chapter DOI: https://doi.org/10.1017/9781009010054.015
Save book to Dropbox
To save content items to your account, please confirm that you agree to abide by our usage policies. If this is the first time you use this feature, you will be asked to authorise Cambridge Core to connect with your account. Find out more about saving content to Dropbox .
Save book to Google Drive
To save content items to your account, please confirm that you agree to abide by our usage policies. If this is the first time you use this feature, you will be asked to authorise Cambridge Core to connect with your account. Find out more about saving content to Google Drive .
A Modern Guide to Understanding and Conducting Research in Psychology
Chapter 7 quasi-experimental research, learning objectives.
- Explain what quasi-experimental research is and distinguish it clearly from both experimental and correlational research.
- Describe three different types of quasi-experimental research designs (nonequivalent groups, pretest-posttest, and interrupted time series) and identify examples of each one.
The prefix quasi means “resembling.” Thus quasi-experimental research is research that resembles experimental research but is not true experimental research. Although the independent variable is manipulated, participants are not randomly assigned to conditions or orders of conditions ( Cook et al., 1979 ) . Because the independent variable is manipulated before the dependent variable is measured, quasi-experimental research eliminates the directionality problem. But because participants are not randomly assigned—making it likely that there are other differences between conditions—quasi-experimental research does not eliminate the problem of confounding variables. In terms of internal validity, therefore, quasi-experiments are generally somewhere between correlational studies and true experiments.
Quasi-experiments are most likely to be conducted in field settings in which random assignment is difficult or impossible. They are often conducted to evaluate the effectiveness of a treatment—perhaps a type of psychotherapy or an educational intervention. There are many different kinds of quasi-experiments, but we will discuss just a few of the most common ones here, focusing first on nonequivalent groups, pretest-posttest, interrupted time series, and combination designs before turning to single subject designs (including reversal and multiple-baseline designs).
7.1 Nonequivalent Groups Design
Recall that when participants in a between-subjects experiment are randomly assigned to conditions, the resulting groups are likely to be quite similar. In fact, researchers consider them to be equivalent. When participants are not randomly assigned to conditions, however, the resulting groups are likely to be dissimilar in some ways. For this reason, researchers consider them to be nonequivalent. A nonequivalent groups design , then, is a between-subjects design in which participants have not been randomly assigned to conditions.
Imagine, for example, a researcher who wants to evaluate a new method of teaching fractions to third graders. One way would be to conduct a study with a treatment group consisting of one class of third-grade students and a control group consisting of another class of third-grade students. This would be a nonequivalent groups design because the students are not randomly assigned to classes by the researcher, which means there could be important differences between them. For example, the parents of higher achieving or more motivated students might have been more likely to request that their children be assigned to Ms. Williams’s class. Or the principal might have assigned the “troublemakers” to Mr. Jones’s class because he is a stronger disciplinarian. Of course, the teachers’ styles, and even the classroom environments, might be very different and might cause different levels of achievement or motivation among the students. If at the end of the study there was a difference in the two classes’ knowledge of fractions, it might have been caused by the difference between the teaching methods—but it might have been caused by any of these confounding variables.
Of course, researchers using a nonequivalent groups design can take steps to ensure that their groups are as similar as possible. In the present example, the researcher could try to select two classes at the same school, where the students in the two classes have similar scores on a standardized math test and the teachers are the same sex, are close in age, and have similar teaching styles. Taking such steps would increase the internal validity of the study because it would eliminate some of the most important confounding variables. But without true random assignment of the students to conditions, there remains the possibility of other important confounding variables that the researcher was not able to control.
7.2 Pretest-Posttest Design
In a pretest-posttest design , the dependent variable is measured once before the treatment is implemented and once after it is implemented. Imagine, for example, a researcher who is interested in the effectiveness of an STEM education program on elementary school students’ attitudes toward science, technology, engineering and math. The researcher could measure the attitudes of students at a particular elementary school during one week, implement the STEM program during the next week, and finally, measure their attitudes again the following week. The pretest-posttest design is much like a within-subjects experiment in which each participant is tested first under the control condition and then under the treatment condition. It is unlike a within-subjects experiment, however, in that the order of conditions is not counterbalanced because it typically is not possible for a participant to be tested in the treatment condition first and then in an “untreated” control condition.
If the average posttest score is better than the average pretest score, then it makes sense to conclude that the treatment might be responsible for the improvement. Unfortunately, one often cannot conclude this with a high degree of certainty because there may be other explanations for why the posttest scores are better. One category of alternative explanations goes under the name of history . Other things might have happened between the pretest and the posttest. Perhaps an science program aired on television and many of the students watched it, or perhaps a major scientific discover occured and many of the students heard about it. Another category of alternative explanations goes under the name of maturation . Participants might have changed between the pretest and the posttest in ways that they were going to anyway because they are growing and learning. If it were a yearlong program, participants might become more exposed to STEM subjects in class or better reasoners and this might be responsible for the change.
Another alternative explanation for a change in the dependent variable in a pretest-posttest design is regression to the mean . This refers to the statistical fact that an individual who scores extremely on a variable on one occasion will tend to score less extremely on the next occasion. For example, a bowler with a long-term average of 150 who suddenly bowls a 220 will almost certainly score lower in the next game. Her score will “regress” toward her mean score of 150. Regression to the mean can be a problem when participants are selected for further study because of their extreme scores. Imagine, for example, that only students who scored especially low on a test of fractions are given a special training program and then retested. Regression to the mean all but guarantees that their scores will be higher even if the training program has no effect. A closely related concept—and an extremely important one in psychological research—is spontaneous remission . This is the tendency for many medical and psychological problems to improve over time without any form of treatment. The common cold is a good example. If one were to measure symptom severity in 100 common cold sufferers today, give them a bowl of chicken soup every day, and then measure their symptom severity again in a week, they would probably be much improved. This does not mean that the chicken soup was responsible for the improvement, however, because they would have been much improved without any treatment at all. The same is true of many psychological problems. A group of severely depressed people today is likely to be less depressed on average in 6 months. In reviewing the results of several studies of treatments for depression, researchers Michael Posternak and Ivan Miller found that participants in waitlist control conditions improved an average of 10 to 15% before they received any treatment at all ( Posternak & Miller, 2001 ) . Thus one must generally be very cautious about inferring causality from pretest-posttest designs.
Finally, it is possible that the act of taking a pretest can sensitize participants to the measurement process or heighten their awareness of the variable under investigation. This heightened sensitivity, called a testing effect , can subsequently lead to changes in their posttest responses, even in the absence of any external intervention effect.
7.3 Interrupted Time Series Design
A variant of the pretest-posttest design is the interrupted time-series design . A time series is a set of measurements taken at intervals over a period of time. For example, a manufacturing company might measure its workers’ productivity each week for a year. In an interrupted time series-design, a time series like this is “interrupted” by a treatment. In a recent COVID-19 study, the intervention involved the implementation of state-issued mask mandates and restrictions on on-premises restaurant dining. The researchers examined the impact of these measures on COVID-19 cases and deaths ( Guy Jr et al., 2021 ) . Since there was a rapid reduction in daily case and death growth rates following the implementation of mask mandates, and this effect persisted for an extended period, the researchers concluded that the implementation of mask mandates was the cause of the decrease in COVID-19 transmission. This study employed an interrupted time series design, similar to a pretest-posttest design, as it involved measuring the outcomes before and after the intervention. However, unlike the pretest-posttest design, it incorporated multiple measurements before and after the intervention, providing a more comprehensive analysis of the policy impacts.
Figure 7.1 shows data from a hypothetical interrupted time-series study. The dependent variable is the number of student absences per week in a research methods course. The treatment is that the instructor begins publicly taking attendance each day so that students know that the instructor is aware of who is present and who is absent. The top panel of Figure 7.1 shows how the data might look if this treatment worked. There is a consistently high number of absences before the treatment, and there is an immediate and sustained drop in absences after the treatment. The bottom panel of Figure 7.1 shows how the data might look if this treatment did not work. On average, the number of absences after the treatment is about the same as the number before. This figure also illustrates an advantage of the interrupted time-series design over a simpler pretest-posttest design. If there had been only one measurement of absences before the treatment at Week 7 and one afterward at Week 8, then it would have looked as though the treatment were responsible for the reduction. The multiple measurements both before and after the treatment suggest that the reduction between Weeks 7 and 8 is nothing more than normal week-to-week variation.

Figure 7.1: Hypothetical interrupted time-series design. The top panel shows data that suggest that the treatment caused a reduction in absences. The bottom panel shows data that suggest that it did not.
7.4 Combination Designs
A type of quasi-experimental design that is generally better than either the nonequivalent groups design or the pretest-posttest design is one that combines elements of both. There is a treatment group that is given a pretest, receives a treatment, and then is given a posttest. But at the same time there is a control group that is given a pretest, does not receive the treatment, and then is given a posttest. The question, then, is not simply whether participants who receive the treatment improve but whether they improve more than participants who do not receive the treatment.
Imagine, for example, that students in one school are given a pretest on their current level of engagement in pro-environmental behaviors (i.e., recycling, eating less red meat, abstaining for single-use plastics, etc.), then are exposed to an pro-environmental program in which they learn about the effects of human caused climate change on the planet, and finally are given a posttest. Students in a similar school are given the pretest, not exposed to an pro-environmental program, and finally are given a posttest. Again, if students in the treatment condition become more involved in pro-environmental behaviors, this could be an effect of the treatment, but it could also be a matter of history or maturation. If it really is an effect of the treatment, then students in the treatment condition should become engage in more pro-environmental behaviors than students in the control condition. But if it is a matter of history (e.g., news of a forest fire or drought) or maturation (e.g., improved reasoning or sense of responsibility), then students in the two conditions would be likely to show similar amounts of change. This type of design does not completely eliminate the possibility of confounding variables, however. Something could occur at one of the schools but not the other (e.g., a local heat wave with record high temperatures), so students at the first school would be affected by it while students at the other school would not.
Finally, if participants in this kind of design are randomly assigned to conditions, it becomes a true experiment rather than a quasi experiment. In fact, this kind of design has now been conducted many times—to demonstrate the effectiveness of psychotherapy.
KEY TAKEAWAYS
- Quasi-experimental research involves the manipulation of an independent variable without the random assignment of participants to conditions or orders of conditions. Among the important types are nonequivalent groups designs, pretest-posttest, and interrupted time-series designs.
- Quasi-experimental research eliminates the directionality problem because it involves the manipulation of the independent variable. It does not eliminate the problem of confounding variables, however, because it does not involve random assignment to conditions. For these reasons, quasi-experimental research is generally higher in internal validity than correlational studies but lower than true experiments.
- Practice: Imagine that two college professors decide to test the effect of giving daily quizzes on student performance in a statistics course. They decide that Professor A will give quizzes but Professor B will not. They will then compare the performance of students in their two sections on a common final exam. List five other variables that might differ between the two sections that could affect the results.
regression to the mean
Spontaneous remission, 7.5 single-subject research.
- Explain what single-subject research is, including how it differs from other types of psychological research and who uses single-subject research and why.
- Design simple single-subject studies using reversal and multiple-baseline designs.
- Explain how single-subject research designs address the issue of internal validity.
- Interpret the results of simple single-subject studies based on the visual inspection of graphed data.
- Explain some of the points of disagreement between advocates of single-subject research and advocates of group research.
Researcher Vance Hall and his colleagues were faced with the challenge of increasing the extent to which six disruptive elementary school students stayed focused on their schoolwork ( Hall et al., 1968 ) . For each of several days, the researchers carefully recorded whether or not each student was doing schoolwork every 10 seconds during a 30-minute period. Once they had established this baseline, they introduced a treatment. The treatment was that when the student was doing schoolwork, the teacher gave him or her positive attention in the form of a comment like “good work” or a pat on the shoulder. The result was that all of the students dramatically increased their time spent on schoolwork and decreased their disruptive behavior during this treatment phase. For example, a student named Robbie originally spent 25% of his time on schoolwork and the other 75% “snapping rubber bands, playing with toys from his pocket, and talking and laughing with peers” (p. 3). During the treatment phase, however, he spent 71% of his time on schoolwork and only 29% on other activities. Finally, when the researchers had the teacher stop giving positive attention, the students all decreased their studying and increased their disruptive behavior. This was consistent with the claim that it was, in fact, the positive attention that was responsible for the increase in studying. This was one of the first studies to show that attending to positive behavior—and ignoring negative behavior—could be a quick and effective way to deal with problem behavior in an applied setting.

Figure 7.2: Single-subject research has shown that positive attention from a teacher for studying can increase studying and decrease disruptive behavior. Photo by Jerry Wang on Unsplash.
Most of this book is about what can be called group research, which typically involves studying a large number of participants and combining their data to draw general conclusions about human behavior. The study by Hall and his colleagues, in contrast, is an example of single-subject research, which typically involves studying a small number of participants and focusing closely on each individual. In this section, we consider this alternative approach. We begin with an overview of single-subject research, including some assumptions on which it is based, who conducts it, and why they do. We then look at some basic single-subject research designs and how the data from those designs are analyzed. Finally, we consider some of the strengths and weaknesses of single-subject research as compared with group research and see how these two approaches can complement each other.
Overview of Single-Subject Research
What is single-subject research.
Single-subject research is a type of quantitative, quasi-experimental research that involves studying in detail the behavior of each of a small number of participants. Note that the term single-subject does not mean that only one participant is studied; it is more typical for there to be somewhere between two and 10 participants. (This is why single-subject research designs are sometimes called small-n designs, where n is the statistical symbol for the sample size.) Single-subject research can be contrasted with group research , which typically involves studying large numbers of participants and examining their behavior primarily in terms of group means, standard deviations, and so on. The majority of this book is devoted to understanding group research, which is the most common approach in psychology. But single-subject research is an important alternative, and it is the primary approach in some areas of psychology.
Before continuing, it is important to distinguish single-subject research from two other approaches, both of which involve studying in detail a small number of participants. One is qualitative research, which focuses on understanding people’s subjective experience by collecting relatively unstructured data (e.g., detailed interviews) and analyzing those data using narrative rather than quantitative techniques (see. Single-subject research, in contrast, focuses on understanding objective behavior through experimental manipulation and control, collecting highly structured data, and analyzing those data quantitatively.
It is also important to distinguish single-subject research from case studies. A case study is a detailed description of an individual, which can include both qualitative and quantitative analyses. (Case studies that include only qualitative analyses can be considered a type of qualitative research.) The history of psychology is filled with influential cases studies, such as Sigmund Freud’s description of “Anna O.” (see box “The Case of ‘Anna O.’”) and John Watson and Rosalie Rayner’s description of Little Albert ( Watson & Rayner, 1920 ) who learned to fear a white rat—along with other furry objects—when the researchers made a loud noise while he was playing with the rat. Case studies can be useful for suggesting new research questions and for illustrating general principles. They can also help researchers understand rare phenomena, such as the effects of damage to a specific part of the human brain. As a general rule, however, case studies cannot substitute for carefully designed group or single-subject research studies. One reason is that case studies usually do not allow researchers to determine whether specific events are causally related, or even related at all. For example, if a patient is described in a case study as having been sexually abused as a child and then as having developed an eating disorder as a teenager, there is no way to determine whether these two events had anything to do with each other. A second reason is that an individual case can always be unusual in some way and therefore be unrepresentative of people more generally. Thus case studies have serious problems with both internal and external validity.
The Case of “Anna O.”
Sigmund Freud used the case of a young woman he called “Anna O.” to illustrate many principles of his theory of psychoanalysis ( Freud, 1957 ) . (Her real name was Bertha Pappenheim, and she was an early feminist who went on to make important contributions to the field of social work.) Anna had come to Freud’s colleague Josef Breuer around 1880 with a variety of odd physical and psychological symptoms. One of them was that for several weeks she was unable to drink any fluids. According to Freud,
She would take up the glass of water that she longed for, but as soon as it touched her lips she would push it away like someone suffering from hydrophobia.…She lived only on fruit, such as melons, etc., so as to lessen her tormenting thirst (p. 9).
But according to Freud, a breakthrough came one day while Anna was under hypnosis.
[S]he grumbled about her English “lady-companion,” whom she did not care for, and went on to describe, with every sign of disgust, how she had once gone into this lady’s room and how her little dog—horrid creature!—had drunk out of a glass there. The patient had said nothing, as she had wanted to be polite. After giving further energetic expression to the anger she had held back, she asked for something to drink, drank a large quantity of water without any difficulty, and awoke from her hypnosis with the glass at her lips; and thereupon the disturbance vanished, never to return.
Freud’s interpretation was that Anna had repressed the memory of this incident along with the emotion that it triggered and that this was what had caused her inability to drink. Furthermore, her recollection of the incident, along with her expression of the emotion she had repressed, caused the symptom to go away.
As an illustration of Freud’s theory, the case study of Anna O. is quite effective. As evidence for the theory, however, it is essentially worthless. The description provides no way of knowing whether Anna had really repressed the memory of the dog drinking from the glass, whether this repression had caused her inability to drink, or whether recalling this “trauma” relieved the symptom. It is also unclear from this case study how typical or atypical Anna’s experience was.

Figure 7.3: “Anna O.” was the subject of a famous case study used by Freud to illustrate the principles of psychoanalysis. Source: Wikimedia Commons
Assumptions of Single-Subject Research
Again, single-subject research involves studying a small number of participants and focusing intensively on the behavior of each one. But why take this approach instead of the group approach? There are two important assumptions underlying single-subject research, and it will help to consider them now.
First and foremost is the assumption that it is important to focus intensively on the behavior of individual participants. One reason for this is that group research can hide individual differences and generate results that do not represent the behavior of any individual. For example, a treatment that has a positive effect for half the people exposed to it but a negative effect for the other half would, on average, appear to have no effect at all. Single-subject research, however, would likely reveal these individual differences. A second reason to focus intensively on individuals is that sometimes it is the behavior of a particular individual that is primarily of interest. A school psychologist, for example, might be interested in changing the behavior of a particular disruptive student. Although previous published research (both single-subject and group research) is likely to provide some guidance on how to do this, conducting a study on this student would be more direct and probably more effective.
Another assumption of single-subject research is that it is important to study strong and consistent effects that have biological or social importance. Applied researchers, in particular, are interested in treatments that have substantial effects on important behaviors and that can be implemented reliably in the real-world contexts in which they occur. This is sometimes referred to as social validity ( Wolf, 1978 ) . The study by Hall and his colleagues, for example, had good social validity because it showed strong and consistent effects of positive teacher attention on a behavior that is of obvious importance to teachers, parents, and students. Furthermore, the teachers found the treatment easy to implement, even in their often chaotic elementary school classrooms.
Who Uses Single-Subject Research?
Single-subject research has been around as long as the field of psychology itself. In the late 1800s, one of psychology’s founders, Wilhelm Wundt, studied sensation and consciousness by focusing intensively on each of a small number of research participants. Herman Ebbinghaus’s research on memory and Ivan Pavlov’s research on classical conditioning are other early examples, both of which are still described in almost every introductory psychology textbook.
In the middle of the 20th century, B. F. Skinner clarified many of the assumptions underlying single-subject research and refined many of its techniques ( Skinner, 1938 ) . He and other researchers then used it to describe how rewards, punishments, and other external factors affect behavior over time. This work was carried out primarily using nonhuman subjects—mostly rats and pigeons. This approach, which Skinner called the experimental analysis of behavior —remains an important subfield of psychology and continues to rely almost exclusively on single-subject research. For examples of this work, look at any issue of the Journal of the Experimental Analysis of Behavior . By the 1960s, many researchers were interested in using this approach to conduct applied research primarily with humans—a subfield now called applied behavior analysis ( Baer et al., 1968 ) . Applied behavior analysis plays a significant role in contemporary research on developmental disabilities, education, organizational behavior, and health, among many other areas. Examples of this work (including the study by Hall and his colleagues) can be found in the Journal of Applied Behavior Analysis . The single-subject approach can also be used by clinicians who take any theoretical perspective—behavioral, cognitive, psychodynamic, or humanistic—to study processes of therapeutic change with individual clients and to document their clients’ improvement ( Kazdin, 2019 ) .
Single-Subject Research Designs
General features of single-subject designs.
Before looking at any specific single-subject research designs, it will be helpful to consider some features that are common to most of them. Many of these features are illustrated in Figure 7.4 , which shows the results of a generic single-subject study. First, the dependent variable (represented on the y-axis of the graph) is measured repeatedly over time (represented by the x-axis) at regular intervals. Second, the study is divided into distinct phases, and the participant is tested under one condition per phase. The conditions are often designated by capital letters: A, B, C, and so on. Thus Figure 7.4 represents a design in which the participant was tested first in one condition (A), then tested in another condition (B), and finally retested in the original condition (A). (This is called a reversal design and will be discussed in more detail shortly.)

Figure 7.4: Results of a generic single-subject study illustrating several principles of single-subject research.
Another important aspect of single-subject research is that the change from one condition to the next does not usually occur after a fixed amount of time or number of observations. Instead, it depends on the participant’s behavior. Specifically, the researcher waits until the participant’s behavior in one condition becomes fairly consistent from observation to observation before changing conditions. This is sometimes referred to as the steady state strategy ( Sidman, 1960 ) . The idea is that when the dependent variable has reached a steady state, then any change across conditions will be relatively easy to detect. Recall that we encountered this same principle when discussing experimental research more generally. The effect of an independent variable is easier to detect when the “noise” in the data is minimized.
Reversal Designs
The most basic single-subject research design is the reversal design , also called the ABA design . During the first phase, A, a baseline is established for the dependent variable. This is the level of responding before any treatment is introduced, and therefore the baseline phase is a kind of control condition. When steady state responding is reached, phase B begins as the researcher introduces the treatment. Again, the researcher waits until that dependent variable reaches a steady state so that it is clear whether and how much it has changed. Finally, the researcher removes the treatment and again waits until the dependent variable reaches a steady state. This basic reversal design can also be extended with the reintroduction of the treatment (ABAB), another return to baseline (ABABA), and so on. The study by Hall and his colleagues was an ABAB reversal design (Figure 7.5 ).

Figure 7.5: An approximation of the results for Hall and colleagues’ participant Robbie in their ABAB reversal design. The percentage of time he spent studying (the dependent variable) was low during the first baseline phase, increased during the first treatment phase until it leveled off, decreased during the second baseline phase, and again increased during the second treatment phase.
Why is the reversal—the removal of the treatment—considered to be necessary in this type of design? If the dependent variable changes after the treatment is introduced, it is not always clear that the treatment was responsible for the change. It is possible that something else changed at around the same time and that this extraneous variable is responsible for the change in the dependent variable. But if the dependent variable changes with the introduction of the treatment and then changes back with the removal of the treatment, it is much clearer that the treatment (and removal of the treatment) is the cause. In other words, the reversal greatly increases the internal validity of the study.
Multiple-Baseline Designs
There are two potential problems with the reversal design—both of which have to do with the removal of the treatment. One is that if a treatment is working, it may be unethical to remove it. For example, if a treatment seemed to reduce the incidence of self-injury in a developmentally disabled child, it would be unethical to remove that treatment just to show that the incidence of self-injury increases. The second problem is that the dependent variable may not return to baseline when the treatment is removed. For example, when positive attention for studying is removed, a student might continue to study at an increased rate. This could mean that the positive attention had a lasting effect on the student’s studying, which of course would be good, but it could also mean that the positive attention was not really the cause of the increased studying in the first place.
One solution to these problems is to use a multiple-baseline design , which is represented in Figure 7.6 . In one version of the design, a baseline is established for each of several participants, and the treatment is then introduced for each one. In essence, each participant is tested in an AB design. The key to this design is that the treatment is introduced at a different time for each participant. The idea is that if the dependent variable changes when the treatment is introduced for one participant, it might be a coincidence. But if the dependent variable changes when the treatment is introduced for multiple participants—especially when the treatment is introduced at different times for the different participants—then it is less likely to be a coincidence.

Figure 7.6: Results of a generic multiple-baseline study. The multiple baselines can be for different participants, dependent variables, or settings. The treatment is introduced at a different time on each baseline.
As an example, consider a study by Scott Ross and Robert Horner ( Ross et al., 2009 ) . They were interested in how a school-wide bullying prevention program affected the bullying behavior of particular problem students. At each of three different schools, the researchers studied two students who had regularly engaged in bullying. During the baseline phase, they observed the students for 10-minute periods each day during lunch recess and counted the number of aggressive behaviors they exhibited toward their peers. (The researchers used handheld computers to help record the data.) After 2 weeks, they implemented the program at one school. After 2 more weeks, they implemented it at the second school. And after 2 more weeks, they implemented it at the third school. They found that the number of aggressive behaviors exhibited by each student dropped shortly after the program was implemented at his or her school. Notice that if the researchers had only studied one school or if they had introduced the treatment at the same time at all three schools, then it would be unclear whether the reduction in aggressive behaviors was due to the bullying program or something else that happened at about the same time it was introduced (e.g., a holiday, a television program, a change in the weather). But with their multiple-baseline design, this kind of coincidence would have to happen three separate times—an unlikely occurrence—to explain their results.
Data Analysis in Single-Subject Research
In addition to its focus on individual participants, single-subject research differs from group research in the way the data are typically analyzed. As we have seen throughout the book, group research involves combining data across participants. Inferential statistics are used to help decide whether the result for the sample is likely to generalize to the population. Single-subject research, by contrast, relies heavily on a very different approach called visual inspection . This means plotting individual participants’ data as shown throughout this chapter, looking carefully at those data, and making judgments about whether and to what extent the independent variable had an effect on the dependent variable. Inferential statistics are typically not used.
In visually inspecting their data, single-subject researchers take several factors into account. One of them is changes in the level of the dependent variable from condition to condition. If the dependent variable is much higher or much lower in one condition than another, this suggests that the treatment had an effect. A second factor is trend , which refers to gradual increases or decreases in the dependent variable across observations. If the dependent variable begins increasing or decreasing with a change in conditions, then again this suggests that the treatment had an effect. It can be especially telling when a trend changes directions—for example, when an unwanted behavior is increasing during baseline but then begins to decrease with the introduction of the treatment. A third factor is latency , which is the time it takes for the dependent variable to begin changing after a change in conditions. In general, if a change in the dependent variable begins shortly after a change in conditions, this suggests that the treatment was responsible.
In the top panel of Figure 7.7 , there are fairly obvious changes in the level and trend of the dependent variable from condition to condition. Furthermore, the latencies of these changes are short; the change happens immediately. This pattern of results strongly suggests that the treatment was responsible for the changes in the dependent variable. In the bottom panel of Figure 7.7 , however, the changes in level are fairly small. And although there appears to be an increasing trend in the treatment condition, it looks as though it might be a continuation of a trend that had already begun during baseline. This pattern of results strongly suggests that the treatment was not responsible for any changes in the dependent variable—at least not to the extent that single-subject researchers typically hope to see.

Figure 7.7: Visual inspection of the data suggests an effective treatment in the top panel but an ineffective treatment in the bottom panel.
The results of single-subject research can also be analyzed using statistical procedures—and this is becoming more common. There are many different approaches, and single-subject researchers continue to debate which are the most useful. One approach parallels what is typically done in group research. The mean and standard deviation of each participant’s responses under each condition are computed and compared, and inferential statistical tests such as the t test or analysis of variance are applied ( Fisch, 2001 ) . (Note that averaging across participants is less common.) Another approach is to compute the percentage of nonoverlapping data (PND) for each participant ( Scruggs & Mastropieri, 2021 ) . This is the percentage of responses in the treatment condition that are more extreme than the most extreme response in a relevant control condition. In the study of Hall and his colleagues, for example, all measures of Robbie’s study time in the first treatment condition were greater than the highest measure in the first baseline, for a PND of 100%. The greater the percentage of nonoverlapping data, the stronger the treatment effect. Still, formal statistical approaches to data analysis in single-subject research are generally considered a supplement to visual inspection, not a replacement for it.
The Single-Subject Versus Group “Debate”
Single-subject research is similar to group research—especially experimental group research—in many ways. They are both quantitative approaches that try to establish causal relationships by manipulating an independent variable, measuring a dependent variable, and controlling extraneous variables. As we will see, single-subject research and group research are probably best conceptualized as complementary approaches.
Data Analysis
One set of disagreements revolves around the issue of data analysis. Some advocates of group research worry that visual inspection is inadequate for deciding whether and to what extent a treatment has affected a dependent variable. One specific concern is that visual inspection is not sensitive enough to detect weak effects. A second is that visual inspection can be unreliable, with different researchers reaching different conclusions about the same set of data ( Danov & Symons, 2008 ) . A third is that the results of visual inspection—an overall judgment of whether or not a treatment was effective—cannot be clearly and efficiently summarized or compared across studies (unlike the measures of relationship strength typically used in group research).
In general, single-subject researchers share these concerns. However, they also argue that their use of the steady state strategy, combined with their focus on strong and consistent effects, minimizes most of them. If the effect of a treatment is difficult to detect by visual inspection because the effect is weak or the data are noisy, then single-subject researchers look for ways to increase the strength of the effect or reduce the noise in the data by controlling extraneous variables (e.g., by administering the treatment more consistently). If the effect is still difficult to detect, then they are likely to consider it neither strong enough nor consistent enough to be of further interest. Many single-subject researchers also point out that statistical analysis is becoming increasingly common and that many of them are using it as a supplement to visual inspection—especially for the purpose of comparing results across studies ( Scruggs & Mastropieri, 2021 ) .
Turning the tables, some advocates of single-subject research worry about the way that group researchers analyze their data. Specifically, they point out that focusing on group means can be highly misleading. Again, imagine that a treatment has a strong positive effect on half the people exposed to it and an equally strong negative effect on the other half. In a traditional between-subjects experiment, the positive effect on half the participants in the treatment condition would be statistically cancelled out by the negative effect on the other half. The mean for the treatment group would then be the same as the mean for the control group, making it seem as though the treatment had no effect when in fact it had a strong effect on every single participant!
But again, group researchers share this concern. Although they do focus on group statistics, they also emphasize the importance of examining distributions of individual scores. For example, if some participants were positively affected by a treatment and others negatively affected by it, this would produce a bimodal distribution of scores and could be detected by looking at a histogram of the data. The use of within-subjects designs is another strategy that allows group researchers to observe effects at the individual level and even to specify what percentage of individuals exhibit strong, medium, weak, and even negative effects.
External Validity
The second issue about which single-subject and group researchers sometimes disagree has to do with external validity—the ability to generalize the results of a study beyond the people and situation actually studied. In particular, advocates of group research point out the difficulty in knowing whether results for just a few participants are likely to generalize to others in the population. Imagine, for example, that in a single-subject study, a treatment has been shown to reduce self-injury for each of two developmentally disabled children. Even if the effect is strong for these two children, how can one know whether this treatment is likely to work for other developmentally disabled children?
Again, single-subject researchers share this concern. In response, they note that the strong and consistent effects they are typically interested in—even when observed in small samples—are likely to generalize to others in the population. Single-subject researchers also note that they place a strong emphasis on replicating their research results. When they observe an effect with a small sample of participants, they typically try to replicate it with another small sample—perhaps with a slightly different type of participant or under slightly different conditions. Each time they observe similar results, they rightfully become more confident in the generality of those results. Single-subject researchers can also point to the fact that the principles of classical and operant conditioning—most of which were discovered using the single-subject approach—have been successfully generalized across an incredibly wide range of species and situations.
And again turning the tables, single-subject researchers have concerns of their own about the external validity of group research. One extremely important point they make is that studying large groups of participants does not entirely solve the problem of generalizing to other individuals. Imagine, for example, a treatment that has been shown to have a small positive effect on average in a large group study. It is likely that although many participants exhibited a small positive effect, others exhibited a large positive effect, and still others exhibited a small negative effect. When it comes to applying this treatment to another large group , we can be fairly sure that it will have a small effect on average. But when it comes to applying this treatment to another individual , we cannot be sure whether it will have a small, a large, or even a negative effect. Another point that single-subject researchers make is that group researchers also face a similar problem when they study a single situation and then generalize their results to other situations. For example, researchers who conduct a study on the effect of cell phone use on drivers on a closed oval track probably want to apply their results to drivers in many other real-world driving situations. But notice that this requires generalizing from a single situation to a population of situations. Thus the ability to generalize is based on much more than just the sheer number of participants one has studied. It requires a careful consideration of the similarity of the participants and situations studied to the population of participants and situations that one wants to generalize to ( Shadish et al., 2002 ) .
Single-Subject and Group Research as Complementary Methods
As with quantitative and qualitative research, it is probably best to conceptualize single-subject research and group research as complementary methods that have different strengths and weaknesses and that are appropriate for answering different kinds of research questions ( Kazdin, 2019 ) . Single-subject research is particularly good for testing the effectiveness of treatments on individuals when the focus is on strong, consistent, and biologically or socially important effects. It is especially useful when the behavior of particular individuals is of interest. Clinicians who work with only one individual at a time may find that it is their only option for doing systematic quantitative research.
Group research, on the other hand, is good for testing the effectiveness of treatments at the group level. Among the advantages of this approach is that it allows researchers to detect weak effects, which can be of interest for many reasons. For example, finding a weak treatment effect might lead to refinements of the treatment that eventually produce a larger and more meaningful effect. Group research is also good for studying interactions between treatments and participant characteristics. For example, if a treatment is effective for those who are high in motivation to change and ineffective for those who are low in motivation to change, then a group design can detect this much more efficiently than a single-subject design. Group research is also necessary to answer questions that cannot be addressed using the single-subject approach, including questions about independent variables that cannot be manipulated (e.g., number of siblings, extroversion, culture).
- Single-subject research—which involves testing a small number of participants and focusing intensively on the behavior of each individual—is an important alternative to group research in psychology.
- Single-subject studies must be distinguished from case studies, in which an individual case is described in detail. Case studies can be useful for generating new research questions, for studying rare phenomena, and for illustrating general principles. However, they cannot substitute for carefully controlled experimental or correlational studies because they are low in internal and external validity.
- Single-subject research designs typically involve measuring the dependent variable repeatedly over time and changing conditions (e.g., from baseline to treatment) when the dependent variable has reached a steady state. This approach allows the researcher to see whether changes in the independent variable are causing changes in the dependent variable.
- Single-subject researchers typically analyze their data by graphing them and making judgments about whether the independent variable is affecting the dependent variable based on level, trend, and latency.
- Differences between single-subject research and group research sometimes lead to disagreements between single-subject and group researchers. These disagreements center on the issues of data analysis and external validity (especially generalization to other people). Single-subject research and group research are probably best seen as complementary methods, with different strengths and weaknesses, that are appropriate for answering different kinds of research questions.
- Does positive attention from a parent increase a child’s toothbrushing behavior?
- Does self-testing while studying improve a student’s performance on weekly spelling tests?
- Does regular exercise help relieve depression?
- Practice: Create a graph that displays the hypothetical results for the study you designed in Exercise 1. Write a paragraph in which you describe what the results show. Be sure to comment on level, trend, and latency.
- Discussion: Imagine you have conducted a single-subject study showing a positive effect of a treatment on the behavior of a man with social anxiety disorder. Your research has been criticized on the grounds that it cannot be generalized to others. How could you respond to this criticism?
- Discussion: Imagine you have conducted a group study showing a positive effect of a treatment on the behavior of a group of people with social anxiety disorder, but your research has been criticized on the grounds that “average” effects cannot be generalized to individuals. How could you respond to this criticism?
7.6 Glossary
The simplest reversal design, in which there is a baseline condition (A), followed by a treatment condition (B), followed by a return to baseline (A).
applied behavior analysis
A subfield of psychology that uses single-subject research and applies the principles of behavior analysis to real-world problems in areas that include education, developmental disabilities, organizational behavior, and health behavior.
A condition in a single-subject research design in which the dependent variable is measured repeatedly in the absence of any treatment. Most designs begin with a baseline condition, and many return to the baseline condition at least once.
A detailed description of an individual case.
experimental analysis of behavior
A subfield of psychology founded by B. F. Skinner that uses single-subject research—often with nonhuman animals—to study relationships primarily between environmental conditions and objectively observable behaviors.
group research
A type of quantitative research that involves studying a large number of participants and examining their behavior in terms of means, standard deviations, and other group-level statistics.
interrupted time-series design
A research design in which a series of measurements of the dependent variable are taken both before and after a treatment.
item-order effect
The effect of responding to one survey item on responses to a later survey item.
Refers collectively to extraneous developmental changes in participants that can occur between a pretest and posttest or between the first and last measurements in a time series. It can provide an alternative explanation for an observed change in the dependent variable.
multiple-baseline design
A single-subject research design in which multiple baselines are established for different participants, different dependent variables, or different contexts and the treatment is introduced at a different time for each baseline.
naturalistic observation
An approach to data collection in which the behavior of interest is observed in the environment in which it typically occurs.
nonequivalent groups design
A between-subjects research design in which participants are not randomly assigned to conditions, usually because participants are in preexisting groups (e.g., students at different schools).
nonexperimental research
Research that lacks the manipulation of an independent variable or the random assignment of participants to conditions or orders of conditions.
open-ended item
A questionnaire item that asks a question and allows respondents to respond in whatever way they want.
percentage of nonoverlapping data
A statistic sometimes used in single-subject research. The percentage of observations in a treatment condition that are more extreme than the most extreme observation in a relevant baseline condition.
pretest-posttest design
A research design in which the dependent variable is measured (the pretest), a treatment is given, and the dependent variable is measured again (the posttest) to see if there is a change in the dependent variable from pretest to posttest.
quasi-experimental research
Research that involves the manipulation of an independent variable but lacks the random assignment of participants to conditions or orders of conditions. It is generally used in field settings to test the effectiveness of a treatment.
rating scale
An ordered set of response options to a closed-ended questionnaire item.
The statistical fact that an individual who scores extremely on one occasion will tend to score less extremely on the next occasion.
A term often used to refer to a participant in survey research.
reversal design
A single-subject research design that begins with a baseline condition with no treatment, followed by the introduction of a treatment, and after that a return to the baseline condition. It can include additional treatment conditions and returns to baseline.
single-subject research
A type of quantitative research that involves examining in detail the behavior of each of a small number of participants.
single-variable research
Research that focuses on a single variable rather than on a statistical relationship between variables.
social validity
The extent to which a single-subject study focuses on an intervention that has a substantial effect on an important behavior and can be implemented reliably in the real-world contexts (e.g., by teachers in a classroom) in which that behavior occurs.
Improvement in a psychological or medical problem over time without any treatment.
steady state strategy
In single-subject research, allowing behavior to become fairly consistent from one observation to the next before changing conditions. This makes any effect of the treatment easier to detect.
survey research
A quantitative research approach that uses self-report measures and large, carefully selected samples.
testing effect
A bias in participants’ responses in which scores on the posttest are influenced by simple exposure to the pretest
visual inspection
The primary approach to data analysis in single-subject research, which involves graphing the data and making a judgment as to whether and to what extent the independent variable affected the dependent variable.
Research Methodologies Guide
- Action Research
- Bibliometrics
- Case Studies
- Content Analysis
- Digital Scholarship This link opens in a new window
- Documentary
- Ethnography
- Focus Groups
- Grounded Theory
- Life Histories/Autobiographies
- Longitudinal
- Participant Observation
- Qualitative Research (General)

Quasi-Experimental Design
- Usability Studies
Quasi-Experimental Design is a unique research methodology because it is characterized by what is lacks. For example, Abraham & MacDonald (2011) state:
" Quasi-experimental research is similar to experimental research in that there is manipulation of an independent variable. It differs from experimental research because either there is no control group, no random selection, no random assignment, and/or no active manipulation. "
This type of research is often performed in cases where a control group cannot be created or random selection cannot be performed. This is often the case in certain medical and psychological studies.
For more information on quasi-experimental design, review the resources below:
Where to Start
Below are listed a few tools and online guides that can help you start your Quasi-experimental research. These include free online resources and resources available only through ISU Library.
- Quasi-Experimental Research Designs by Bruce A. Thyer This pocket guide describes the logic, design, and conduct of the range of quasi-experimental designs, encompassing pre-experiments, quasi-experiments making use of a control or comparison group, and time-series designs. An introductory chapter describes the valuable role these types of studies have played in social work, from the 1930s to the present. Subsequent chapters delve into each design type's major features, the kinds of questions it is capable of answering, and its strengths and limitations.
- Experimental and Quasi-Experimental Designs for Research by Donald T. Campbell; Julian C. Stanley. Call Number: Q175 C152e Written 1967 but still used heavily today, this book examines research designs for experimental and quasi-experimental research, with examples and judgments about each design's validity.
Online Resources
- Quasi-Experimental Design From the Web Center for Social Research Methods, this is a very good overview of quasi-experimental design.
- Experimental and Quasi-Experimental Research From Colorado State University.
- Quasi-experimental design--Wikipedia, the free encyclopedia Wikipedia can be a useful place to start your research- check the citations at the bottom of the article for more information.
- << Previous: Qualitative Research (General)
- Next: Sampling >>
- Last Updated: Sep 11, 2024 11:05 AM
- URL: https://instr.iastate.libguides.com/researchmethods
Experimental vs Quasi-Experimental Design: Which to Choose?
Here’s a table that summarizes the similarities and differences between an experimental and a quasi-experimental study design:
Experimental Study (a.k.a. Randomized Controlled Trial) | Quasi-Experimental Study | |
---|---|---|
Objective | Evaluate the effect of an intervention or a treatment | Evaluate the effect of an intervention or a treatment |
How participants get assigned to groups? | Random assignment | Non-random assignment (participants get assigned according to their choosing or that of the researcher) |
Is there a control group? | Yes | Not always (although, if present, a control group will provide better evidence for the study results) |
Is there any room for confounding? | No (although check for a detailed discussion on post-randomization confounding in randomized controlled trials) | Yes (however, statistical techniques can be used to study causal relationships in quasi-experiments) |
Level of evidence | A randomized trial is at the highest level in the hierarchy of evidence | A quasi-experiment is one level below the experimental study in the hierarchy of evidence [ ] |
Advantages | Minimizes bias and confounding | – Can be used in situations where an experiment is not ethically or practically feasible – Can work with smaller sample sizes than randomized trials |
Limitations | – High cost (as it generally requires a large sample size) – Ethical limitations – Generalizability issues – Sometimes practically infeasible | Lower ranking in the hierarchy of evidence as losing the power of randomization causes the study to be more susceptible to bias and confounding |
What is a quasi-experimental design?
A quasi-experimental design is a non-randomized study design used to evaluate the effect of an intervention. The intervention can be a training program, a policy change or a medical treatment.
Unlike a true experiment, in a quasi-experimental study the choice of who gets the intervention and who doesn’t is not randomized. Instead, the intervention can be assigned to participants according to their choosing or that of the researcher, or by using any method other than randomness.
Having a control group is not required, but if present, it provides a higher level of evidence for the relationship between the intervention and the outcome.
(for more information, I recommend my other article: Understand Quasi-Experimental Design Through an Example ) .
Examples of quasi-experimental designs include:
- One-Group Posttest Only Design
- Static-Group Comparison Design
- One-Group Pretest-Posttest Design
- Separate-Sample Pretest-Posttest Design
What is an experimental design?
An experimental design is a randomized study design used to evaluate the effect of an intervention. In its simplest form, the participants will be randomly divided into 2 groups:
- A treatment group: where participants receive the new intervention which effect we want to study.
- A control or comparison group: where participants do not receive any intervention at all (or receive some standard intervention).
Randomization ensures that each participant has the same chance of receiving the intervention. Its objective is to equalize the 2 groups, and therefore, any observed difference in the study outcome afterwards will only be attributed to the intervention – i.e. it removes confounding.
(for more information, I recommend my other article: Purpose and Limitations of Random Assignment ).
Examples of experimental designs include:
- Posttest-Only Control Group Design
- Pretest-Posttest Control Group Design
- Solomon Four-Group Design
- Matched Pairs Design
- Randomized Block Design
When to choose an experimental design over a quasi-experimental design?
Although many statistical techniques can be used to deal with confounding in a quasi-experimental study, in practice, randomization is still the best tool we have to study causal relationships.
Another problem with quasi-experiments is the natural progression of the disease or the condition under study — When studying the effect of an intervention over time, one should consider natural changes because these can be mistaken with changes in outcome that are caused by the intervention. Having a well-chosen control group helps dealing with this issue.
So, if losing the element of randomness seems like an unwise step down in the hierarchy of evidence, why would we ever want to do it?
This is what we’re going to discuss next.
When to choose a quasi-experimental design over a true experiment?
The issue with randomness is that it cannot be always achievable.
So here are some cases where using a quasi-experimental design makes more sense than using an experimental one:
- If being in one group is believed to be harmful for the participants , either because the intervention is harmful (ex. randomizing people to smoking), or the intervention has a questionable efficacy, or on the contrary it is believed to be so beneficial that it would be malevolent to put people in the control group (ex. randomizing people to receiving an operation).
- In cases where interventions act on a group of people in a given location , it becomes difficult to adequately randomize subjects (ex. an intervention that reduces pollution in a given area).
- When working with small sample sizes , as randomized controlled trials require a large sample size to account for heterogeneity among subjects (i.e. to evenly distribute confounding variables between the intervention and control groups).
Further reading
- Statistical Software Popularity in 40,582 Research Papers
- Checking the Popularity of 125 Statistical Tests and Models
- Objectives of Epidemiology (With Examples)
- 12 Famous Epidemiologists and Why
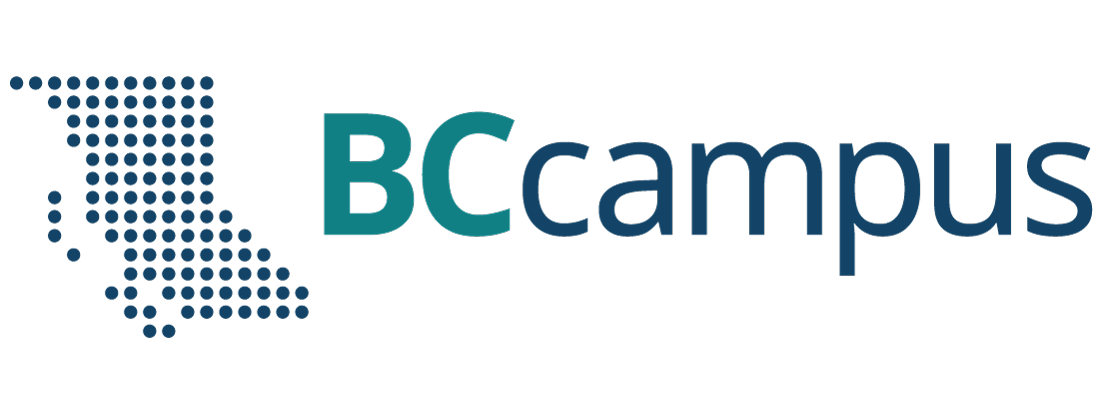
Want to create or adapt books like this? Learn more about how Pressbooks supports open publishing practices.
Chapter 7: Nonexperimental Research
Quasi-Experimental Research
Learning Objectives
- Explain what quasi-experimental research is and distinguish it clearly from both experimental and correlational research.
- Describe three different types of quasi-experimental research designs (nonequivalent groups, pretest-posttest, and interrupted time series) and identify examples of each one.
The prefix quasi means “resembling.” Thus quasi-experimental research is research that resembles experimental research but is not true experimental research. Although the independent variable is manipulated, participants are not randomly assigned to conditions or orders of conditions (Cook & Campbell, 1979). [1] Because the independent variable is manipulated before the dependent variable is measured, quasi-experimental research eliminates the directionality problem. But because participants are not randomly assigned—making it likely that there are other differences between conditions—quasi-experimental research does not eliminate the problem of confounding variables. In terms of internal validity, therefore, quasi-experiments are generally somewhere between correlational studies and true experiments.
Quasi-experiments are most likely to be conducted in field settings in which random assignment is difficult or impossible. They are often conducted to evaluate the effectiveness of a treatment—perhaps a type of psychotherapy or an educational intervention. There are many different kinds of quasi-experiments, but we will discuss just a few of the most common ones here.
Nonequivalent Groups Design
Recall that when participants in a between-subjects experiment are randomly assigned to conditions, the resulting groups are likely to be quite similar. In fact, researchers consider them to be equivalent. When participants are not randomly assigned to conditions, however, the resulting groups are likely to be dissimilar in some ways. For this reason, researchers consider them to be nonequivalent. A nonequivalent groups design , then, is a between-subjects design in which participants have not been randomly assigned to conditions.
Imagine, for example, a researcher who wants to evaluate a new method of teaching fractions to third graders. One way would be to conduct a study with a treatment group consisting of one class of third-grade students and a control group consisting of another class of third-grade students. This design would be a nonequivalent groups design because the students are not randomly assigned to classes by the researcher, which means there could be important differences between them. For example, the parents of higher achieving or more motivated students might have been more likely to request that their children be assigned to Ms. Williams’s class. Or the principal might have assigned the “troublemakers” to Mr. Jones’s class because he is a stronger disciplinarian. Of course, the teachers’ styles, and even the classroom environments, might be very different and might cause different levels of achievement or motivation among the students. If at the end of the study there was a difference in the two classes’ knowledge of fractions, it might have been caused by the difference between the teaching methods—but it might have been caused by any of these confounding variables.
Of course, researchers using a nonequivalent groups design can take steps to ensure that their groups are as similar as possible. In the present example, the researcher could try to select two classes at the same school, where the students in the two classes have similar scores on a standardized math test and the teachers are the same sex, are close in age, and have similar teaching styles. Taking such steps would increase the internal validity of the study because it would eliminate some of the most important confounding variables. But without true random assignment of the students to conditions, there remains the possibility of other important confounding variables that the researcher was not able to control.
Pretest-Posttest Design
In a pretest-posttest design , the dependent variable is measured once before the treatment is implemented and once after it is implemented. Imagine, for example, a researcher who is interested in the effectiveness of an antidrug education program on elementary school students’ attitudes toward illegal drugs. The researcher could measure the attitudes of students at a particular elementary school during one week, implement the antidrug program during the next week, and finally, measure their attitudes again the following week. The pretest-posttest design is much like a within-subjects experiment in which each participant is tested first under the control condition and then under the treatment condition. It is unlike a within-subjects experiment, however, in that the order of conditions is not counterbalanced because it typically is not possible for a participant to be tested in the treatment condition first and then in an “untreated” control condition.
If the average posttest score is better than the average pretest score, then it makes sense to conclude that the treatment might be responsible for the improvement. Unfortunately, one often cannot conclude this with a high degree of certainty because there may be other explanations for why the posttest scores are better. One category of alternative explanations goes under the name of history . Other things might have happened between the pretest and the posttest. Perhaps an antidrug program aired on television and many of the students watched it, or perhaps a celebrity died of a drug overdose and many of the students heard about it. Another category of alternative explanations goes under the name of maturation . Participants might have changed between the pretest and the posttest in ways that they were going to anyway because they are growing and learning. If it were a yearlong program, participants might become less impulsive or better reasoners and this might be responsible for the change.
Another alternative explanation for a change in the dependent variable in a pretest-posttest design is regression to the mean . This refers to the statistical fact that an individual who scores extremely on a variable on one occasion will tend to score less extremely on the next occasion. For example, a bowler with a long-term average of 150 who suddenly bowls a 220 will almost certainly score lower in the next game. Her score will “regress” toward her mean score of 150. Regression to the mean can be a problem when participants are selected for further study because of their extreme scores. Imagine, for example, that only students who scored especially low on a test of fractions are given a special training program and then retested. Regression to the mean all but guarantees that their scores will be higher even if the training program has no effect. A closely related concept—and an extremely important one in psychological research—is spontaneous remission . This is the tendency for many medical and psychological problems to improve over time without any form of treatment. The common cold is a good example. If one were to measure symptom severity in 100 common cold sufferers today, give them a bowl of chicken soup every day, and then measure their symptom severity again in a week, they would probably be much improved. This does not mean that the chicken soup was responsible for the improvement, however, because they would have been much improved without any treatment at all. The same is true of many psychological problems. A group of severely depressed people today is likely to be less depressed on average in 6 months. In reviewing the results of several studies of treatments for depression, researchers Michael Posternak and Ivan Miller found that participants in waitlist control conditions improved an average of 10 to 15% before they received any treatment at all (Posternak & Miller, 2001) [2] . Thus one must generally be very cautious about inferring causality from pretest-posttest designs.
Does Psychotherapy Work?
Early studies on the effectiveness of psychotherapy tended to use pretest-posttest designs. In a classic 1952 article, researcher Hans Eysenck summarized the results of 24 such studies showing that about two thirds of patients improved between the pretest and the posttest (Eysenck, 1952) [3] . But Eysenck also compared these results with archival data from state hospital and insurance company records showing that similar patients recovered at about the same rate without receiving psychotherapy. This parallel suggested to Eysenck that the improvement that patients showed in the pretest-posttest studies might be no more than spontaneous remission. Note that Eysenck did not conclude that psychotherapy was ineffective. He merely concluded that there was no evidence that it was, and he wrote of “the necessity of properly planned and executed experimental studies into this important field” (p. 323). You can read the entire article here: Classics in the History of Psychology .
Fortunately, many other researchers took up Eysenck’s challenge, and by 1980 hundreds of experiments had been conducted in which participants were randomly assigned to treatment and control conditions, and the results were summarized in a classic book by Mary Lee Smith, Gene Glass, and Thomas Miller (Smith, Glass, & Miller, 1980) [4] . They found that overall psychotherapy was quite effective, with about 80% of treatment participants improving more than the average control participant. Subsequent research has focused more on the conditions under which different types of psychotherapy are more or less effective.
Interrupted Time Series Design
A variant of the pretest-posttest design is the interrupted time-series design . A time series is a set of measurements taken at intervals over a period of time. For example, a manufacturing company might measure its workers’ productivity each week for a year. In an interrupted time series-design, a time series like this one is “interrupted” by a treatment. In one classic example, the treatment was the reduction of the work shifts in a factory from 10 hours to 8 hours (Cook & Campbell, 1979) [5] . Because productivity increased rather quickly after the shortening of the work shifts, and because it remained elevated for many months afterward, the researcher concluded that the shortening of the shifts caused the increase in productivity. Notice that the interrupted time-series design is like a pretest-posttest design in that it includes measurements of the dependent variable both before and after the treatment. It is unlike the pretest-posttest design, however, in that it includes multiple pretest and posttest measurements.
Figure 7.3 shows data from a hypothetical interrupted time-series study. The dependent variable is the number of student absences per week in a research methods course. The treatment is that the instructor begins publicly taking attendance each day so that students know that the instructor is aware of who is present and who is absent. The top panel of Figure 7.3 shows how the data might look if this treatment worked. There is a consistently high number of absences before the treatment, and there is an immediate and sustained drop in absences after the treatment. The bottom panel of Figure 7.3 shows how the data might look if this treatment did not work. On average, the number of absences after the treatment is about the same as the number before. This figure also illustrates an advantage of the interrupted time-series design over a simpler pretest-posttest design. If there had been only one measurement of absences before the treatment at Week 7 and one afterward at Week 8, then it would have looked as though the treatment were responsible for the reduction. The multiple measurements both before and after the treatment suggest that the reduction between Weeks 7 and 8 is nothing more than normal week-to-week variation.
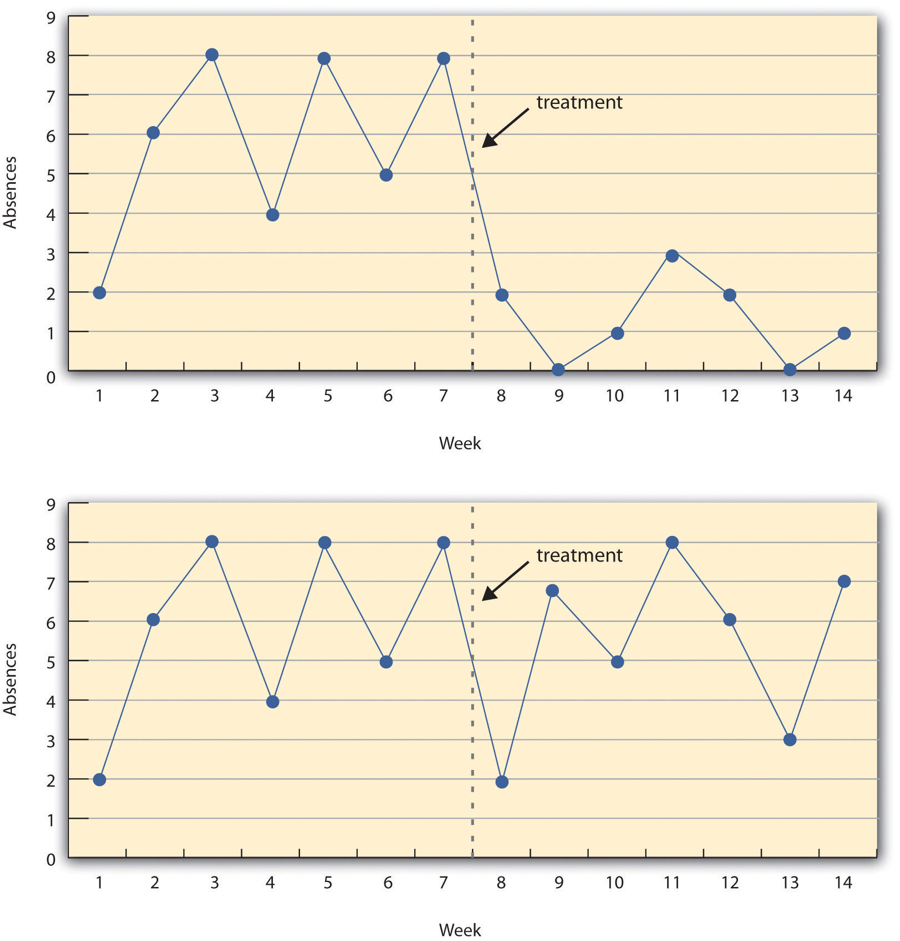
Combination Designs
A type of quasi-experimental design that is generally better than either the nonequivalent groups design or the pretest-posttest design is one that combines elements of both. There is a treatment group that is given a pretest, receives a treatment, and then is given a posttest. But at the same time there is a control group that is given a pretest, does not receive the treatment, and then is given a posttest. The question, then, is not simply whether participants who receive the treatment improve but whether they improve more than participants who do not receive the treatment.
Imagine, for example, that students in one school are given a pretest on their attitudes toward drugs, then are exposed to an antidrug program, and finally are given a posttest. Students in a similar school are given the pretest, not exposed to an antidrug program, and finally are given a posttest. Again, if students in the treatment condition become more negative toward drugs, this change in attitude could be an effect of the treatment, but it could also be a matter of history or maturation. If it really is an effect of the treatment, then students in the treatment condition should become more negative than students in the control condition. But if it is a matter of history (e.g., news of a celebrity drug overdose) or maturation (e.g., improved reasoning), then students in the two conditions would be likely to show similar amounts of change. This type of design does not completely eliminate the possibility of confounding variables, however. Something could occur at one of the schools but not the other (e.g., a student drug overdose), so students at the first school would be affected by it while students at the other school would not.
Finally, if participants in this kind of design are randomly assigned to conditions, it becomes a true experiment rather than a quasi experiment. In fact, it is the kind of experiment that Eysenck called for—and that has now been conducted many times—to demonstrate the effectiveness of psychotherapy.
Key Takeaways
- Quasi-experimental research involves the manipulation of an independent variable without the random assignment of participants to conditions or orders of conditions. Among the important types are nonequivalent groups designs, pretest-posttest, and interrupted time-series designs.
- Quasi-experimental research eliminates the directionality problem because it involves the manipulation of the independent variable. It does not eliminate the problem of confounding variables, however, because it does not involve random assignment to conditions. For these reasons, quasi-experimental research is generally higher in internal validity than correlational studies but lower than true experiments.
- Practice: Imagine that two professors decide to test the effect of giving daily quizzes on student performance in a statistics course. They decide that Professor A will give quizzes but Professor B will not. They will then compare the performance of students in their two sections on a common final exam. List five other variables that might differ between the two sections that could affect the results.
- regression to the mean
- spontaneous remission
Image Descriptions
Figure 7.3 image description: Two line graphs charting the number of absences per week over 14 weeks. The first 7 weeks are without treatment and the last 7 weeks are with treatment. In the first line graph, there are between 4 to 8 absences each week. After the treatment, the absences drop to 0 to 3 each week, which suggests the treatment worked. In the second line graph, there is no noticeable change in the number of absences per week after the treatment, which suggests the treatment did not work. [Return to Figure 7.3]
- Cook, T. D., & Campbell, D. T. (1979). Quasi-experimentation: Design & analysis issues in field settings . Boston, MA: Houghton Mifflin. ↵
- Posternak, M. A., & Miller, I. (2001). Untreated short-term course of major depression: A meta-analysis of studies using outcomes from studies using wait-list control groups. Journal of Affective Disorders, 66 , 139–146. ↵
- Eysenck, H. J. (1952). The effects of psychotherapy: An evaluation. Journal of Consulting Psychology, 16 , 319–324. ↵
- Smith, M. L., Glass, G. V., & Miller, T. I. (1980). The benefits of psychotherapy . Baltimore, MD: Johns Hopkins University Press. ↵
A between-subjects design in which participants have not been randomly assigned to conditions.
The dependent variable is measured once before the treatment is implemented and once after it is implemented.
A category of alternative explanations for differences between scores such as events that happened between the pretest and posttest, unrelated to the study.
An alternative explanation that refers to how the participants might have changed between the pretest and posttest in ways that they were going to anyway because they are growing and learning.
The statistical fact that an individual who scores extremely on a variable on one occasion will tend to score less extremely on the next occasion.
The tendency for many medical and psychological problems to improve over time without any form of treatment.
A set of measurements taken at intervals over a period of time that are interrupted by a treatment.
Research Methods in Psychology - 2nd Canadian Edition Copyright © 2015 by Paul C. Price, Rajiv Jhangiani, & I-Chant A. Chiang is licensed under a Creative Commons Attribution-NonCommercial-ShareAlike 4.0 International License , except where otherwise noted.
Share This Book
- Quasi-Experimental Research: Types, Examples & Application

Let’s say you want to study the effects of a new drug on lowering blood pressure. You could randomly assign half of the participants to receive the drug and the other half to receive a placebo. However, this isn’t fair to the patients in the placebo group.
So, instead of experimenting to find out the effect of the new drug, you use a quasi-experiment to evaluate the drug. The purpose of quasi-experimental research is to establish a causal relationship between an independent variable and a dependent variable.
This guide will discuss the different types of quasi-experimental research, their practical applications, and the best practices for conducting successful quasi-experimental research.
Understanding Quasi-Experimental Research

Quasi-experimental research is a way of finding out if there’s a cause-and-effect relationship between variables when true experiments are not possible because of practical or ethical constraints.
For example, you want to know if a new medicine is effective for migraines. Instead of giving the medication to some people and not to others, you use quasi-experimental research to compare who takes the medication and people who haven’t.
What’s The Difference Between True Experiments and Quasi-Experiments
Quasi-experimental research doesn’t always have the same level of internal validity as real experiments. Pre-selected samples can be biased and not represent the real population.
In a true experiment, the participants are assigned randomly to the experimental or control groups. This ensures that groups are as homogeneous as possible, except for the treatment they receive.
Quasi-experiments don’t randomly assign participants to groups, so the differences within the groups could affect the groups.
Read – Experimental Research Designs: Types, Examples & Methods
- Types of Quasi-Experimental Designs
Pretest-Posttest Design
This design captures changes by measuring participants before and after an intervention. The pretest measures the dependent variable before the intervention, while the posttest measures it after the intervention.
The difference between the two measurements is the change that occurred due to the intervention.
However, it is important to be aware of the potential threats to the internal validity of the pretest-postest design. One threat is selection bias , which happens when the group is not homogenous.
Another is maturation, which occurs when participants change naturally over time. You can mitigate these threats using a control group, randomization, and blinding techniques.

Posttest-Only Design with Nonequivalent Groups
The posttest-only design with nonequivalent groups is similar to the pretest-posttest design, but it does not include a pretest. You see the effect of the intervention by comparing groups to see if there is a difference in their scores.
The difference in scores determines the impact of the intervention. This design is less powerful than the pretest-posttest design because it does not account for potential differences between the groups at baseline.
However, the posttest design is still a valuable tool for research, especially when you can’t collect pretest data. You can mitigate its limitations by using matching participants on important factors, statistical analysis, and sensitivity analysis
- Regression Discontinuity Design
The regression discontinuity design uses naturally occurring cutoff points to assign participants to treatment and control groups. It’s widely adopted in education and social policy research.
For example, a talent recruiter might use the cutoff score on a standardized test to move candidates to the next phase of the application.
Interrupted Time Series Design
The Interrupted Time Series design is a type of study that uses time series data to figure out how interventions or events affect the population. In this study, you measure the dependent variable multiple times over time, before and after the intervention.
The interrupted time series design is most commonly used to study the impact of policies or programs. For example, you are studying the impact of a new law on traffic accidents.
You could collect data on the number of traffic accidents each month for a year before the law was passed and a year after the law was passed. If the number of traffic accidents decreases after the law was passed, then you could conclude that the law had a positive impact on traffic safety.
Rigor in Quasi-Experimental Research
Matching techniques.
Matching techniques are a way to create balanced treatment and control groups in quasi-experimental research. This is done by matching participants on important characteristics, such as age, gender, or socioeconomic status.
Propensity score matching is one of the most popular matching methods. It works by using a statistical model to figure out how likely it is that each person in the study would have been in the treatment group if they were selected randomly. Then, people are randomly assigned according to their propensity scores, making sure that the treatment group and the control group are as close to the same as possible.
- Creates balanced treatment and control groups, which reduces bias in the results.
- Versatile- you can use them in various research settings, especially where randomization is not possible.
- Relatively easy to implement.
- Computationally complex.
- Sensitive to the choice of the matching algorithm.
- Does not perfectly balance the treatment and control groups on all relevant characteristics.
Instrumental Variables

An instrumental variable (IV) in quasi-experimental research is a variable that’s related to the independent variable, but not to the error term. It’s a variable that can be used to measure how the independent variable affects the dependent variable.
Let’s say you want to investigate how a new drug reduces the risk of heart attack. You can use the number of days a person has taken aspirin as the instrumental variable.
Aspirin is associated with an independent variable (new drug), however, it is not associated with a dependent variable (risk of a heart attack). This is because people who take aspirin are more likely to take other medications, such as statins, which also lower the risk of heart attack.
- Addresses endogeneity issues.
- Versatile-you can use it in different research settings
- It is relatively hard to find an instrumental variable that meets all of the criteria.
- May not be perfectly correlated with the independent variable.
- Tends to be affected by the dependent variable.
Difference-in-Differences Analysis

Difference-in-differences analysis is a statistical technique that can be used to compare changes in treatment and control groups over time. It is typically used in quasi-experimental research to estimate the causal effect of an intervention.
You have to first define two groups when using the difference-in-differences analysis: the treatment group and the control group. A treatment group is a group that receives an intervention, while a control group doesn’t receive an intervention.
Next, collect data on the dependent variable for both groups before and after the intervention. The difference-in-differences estimate is then calculated by comparing the change in the dependent variable for the treatment group to the change in the dependent variable for the control group.
For example, in a study by David Card and Alan Krueger , they compared the effect of increasing the minimum wage in a particular region to the employment rate. They found that the minimum wage increase in New Jersey did not lead to job losses.
- Addresses selection bias.
- Control for time-invariant confounders.
- Requires a rigorous research design.
- Sensitive to measurement errors.
- Difficult to interpret when multiple interventions or events are happening during the study period.
Challenges and Best Practices
- Validity Threat
- Selection bias : This occurs when the groups being compared are not equivalent. This can happen if participants are self-selected into the groups, or if the groups are not randomly assigned.
- History effects : These are events that happen during the study period that could affect the dependent variable. For example, if there is a natural disaster during the study period, it could affect the results.
- Maturation : This refers to the natural changes that occur over time. For example, students may naturally improve their test scores over time, regardless of whether or not they receive an intervention.
- Testing effects : These are the effects of taking a test on subsequent test scores. For example, if students take a pretest before an intervention, they may learn from the test and do better on the posttest, even if the intervention had no effect.
- Instrumentation : This refers to changes in the way the dependent variable is measured. For example, if the test used to measure student achievement is changed during the study period, it could affect the results.
Strategies for Minimizing Validity Threats
- Matching participants on important characteristics such as age, education level, and more to reduce selection bias.
- Use control groups to avoid history effects, maturation effects, and testing effects.
- Use multiple methods to measure dependent variables to reduce instrumentation effects.
- Conduct a pilot study to identify and address potential validity threats
Read Also – Internal Validity in Research: Definition, Threats, Examples
Sample Size and Power
Sample size is the number of participants in a study, while power is the probability of detecting a meaningful effect if it exists.
You have to carefully consider sample size and power when designing a quasi-experiment. This is because groups may not be 100% homogeneous at the start of the study, which can limit the strength of the design.
Using power analysis, you can figure out the sample size you need to see a significant effect. Power analysis looks at the magnitude of the effect, and the standard deviation of the dependent variable, and determines the alpha level.
Generalizability
A major downside of the quasi-experimental design is that it’s usually not generalizable or applicable to other environments. It is typically done in natural environments, so you can’t control factors that could influence the results.
Carefully consider the context of the study before you generalize a quasi-experimental. Also, try replicating the study in other settings to see if the results are consistent.
Real-World Applications
- Healthcare Interventions:
A study by the Universiti Kebangsaan, Malaysia used a quasi-experimental design to assess the effectiveness of a new program for preventing childhood obesity. The study found that the program was effective in reducing the risk of obesity, but it was also expensive.
- Education Policy Changes
A study by Raj Chetty and his colleagues found that students who attended charter schools in California were more likely to attend college than students who did not attend charter schools. However, this study arguably promoted academically underqualified students being admitted to colleges.
- Social and Economic Interventions
A study by the RAND Corporation used a quasi-experimental design to assess the effects of a job training program on employment and earnings.
The study found that job training programs were effective in increasing employment and earnings, but they also found that the impact varied depending on the characteristics of the participants and the design of the program.
Ethical Considerations
- Informed consent : Provide full information about the purpose of the study, the procedures, the risks and benefits of participating, and their right to withdraw from the study at any time.
- Confidentiality : Do not collect any personal information that is irrelevant to the study and keep participant information confidential.
- Risks to participants : Quasi-experimental research may pose some risks to participants, such as the risk of harm or discomfort. Minimize these risks as much as possible and only perform the research if the benefits outweigh the risks.
- Benefits to participants : Quasi-experimental research should offer some potential benefits to participants, such as access to new treatments or interventions. Researchers should carefully consider the potential benefits and risks of participating in the study before recruiting participants
- Balance of research goals and participant welfare : Do not conduct research that is likely to harm participants, even if the research has the potential to benefit society.
Read: What are Ethical Practices in Market Research?
Quasi-experimental research is a valuable tool for understanding the causal effects of interventions. It is particularly useful when you can’t conduct actual experiments because of ethical or practical constraints.
However, it is important to be aware of the limitations of this type of research. Carefully design the study and consider the limitations to ensure that the findings are accurate and reliable.
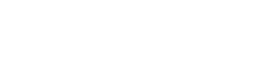
Connect to Formplus, Get Started Now - It's Free!
- Quasi-Experimental Research
- Quasi-Experiments
- True Experiments
- Moradeke Owa
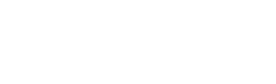
You may also like:
Market Research: Types, Methods & Survey Examples
A complete guide on market research; definitions, survey examples, templates, importance and tips.

What is Pure or Basic Research? + [Examples & Method]
Simple guide on pure or basic research, its methods, characteristics, advantages, and examples in science, medicine, education and psychology
Recall Bias: Definition, Types, Examples & Mitigation
This article will discuss the impact of recall bias in studies and the best ways to avoid them during research.
Exploratory Research: What are its Method & Examples?
Overview on exploratory research, examples and methodology. Shows guides on how to conduct exploratory research with online surveys
Formplus - For Seamless Data Collection
Collect data the right way with a versatile data collection tool. try formplus and transform your work productivity today..
Experimental and Quasi-Experimental Research
Guide Title: Experimental and Quasi-Experimental Research Guide ID: 64
You approach a stainless-steel wall, separated vertically along its middle where two halves meet. After looking to the left, you see two buttons on the wall to the right. You press the top button and it lights up. A soft tone sounds and the two halves of the wall slide apart to reveal a small room. You step into the room. Looking to the left, then to the right, you see a panel of more buttons. You know that you seek a room marked with the numbers 1-0-1-2, so you press the button marked "10." The halves slide shut and enclose you within the cubicle, which jolts upward. Soon, the soft tone sounds again. The door opens again. On the far wall, a sign silently proclaims, "10th floor."
You have engaged in a series of experiments. A ride in an elevator may not seem like an experiment, but it, and each step taken towards its ultimate outcome, are common examples of a search for a causal relationship-which is what experimentation is all about.
You started with the hypothesis that this is in fact an elevator. You proved that you were correct. You then hypothesized that the button to summon the elevator was on the left, which was incorrect, so then you hypothesized it was on the right, and you were correct. You hypothesized that pressing the button marked with the up arrow would not only bring an elevator to you, but that it would be an elevator heading in the up direction. You were right.
As this guide explains, the deliberate process of testing hypotheses and reaching conclusions is an extension of commonplace testing of cause and effect relationships.
Basic Concepts of Experimental and Quasi-Experimental Research
Discovering causal relationships is the key to experimental research. In abstract terms, this means the relationship between a certain action, X, which alone creates the effect Y. For example, turning the volume knob on your stereo clockwise causes the sound to get louder. In addition, you could observe that turning the knob clockwise alone, and nothing else, caused the sound level to increase. You could further conclude that a causal relationship exists between turning the knob clockwise and an increase in volume; not simply because one caused the other, but because you are certain that nothing else caused the effect.
Independent and Dependent Variables
Beyond discovering causal relationships, experimental research further seeks out how much cause will produce how much effect; in technical terms, how the independent variable will affect the dependent variable. You know that turning the knob clockwise will produce a louder noise, but by varying how much you turn it, you see how much sound is produced. On the other hand, you might find that although you turn the knob a great deal, sound doesn't increase dramatically. Or, you might find that turning the knob just a little adds more sound than expected. The amount that you turned the knob is the independent variable, the variable that the researcher controls, and the amount of sound that resulted from turning it is the dependent variable, the change that is caused by the independent variable.
Experimental research also looks into the effects of removing something. For example, if you remove a loud noise from the room, will the person next to you be able to hear you? Or how much noise needs to be removed before that person can hear you?
Treatment and Hypothesis
The term treatment refers to either removing or adding a stimulus in order to measure an effect (such as turning the knob a little or a lot, or reducing the noise level a little or a lot). Experimental researchers want to know how varying levels of treatment will affect what they are studying. As such, researchers often have an idea, or hypothesis, about what effect will occur when they cause something. Few experiments are performed where there is no idea of what will happen. From past experiences in life or from the knowledge we possess in our specific field of study, we know how some actions cause other reactions. Experiments confirm or reconfirm this fact.
Experimentation becomes more complex when the causal relationships they seek aren't as clear as in the stereo knob-turning examples. Questions like "Will olestra cause cancer?" or "Will this new fertilizer help this plant grow better?" present more to consider. For example, any number of things could affect the growth rate of a plant-the temperature, how much water or sun it receives, or how much carbon dioxide is in the air. These variables can affect an experiment's results. An experimenter who wants to show that adding a certain fertilizer will help a plant grow better must ensure that it is the fertilizer, and nothing else, affecting the growth patterns of the plant. To do this, as many of these variables as possible must be controlled.
Matching and Randomization
In the example used in this guide (you'll find the example below), we discuss an experiment that focuses on three groups of plants -- one that is treated with a fertilizer named MegaGro, another group treated with a fertilizer named Plant!, and yet another that is not treated with fetilizer (this latter group serves as a "control" group). In this example, even though the designers of the experiment have tried to remove all extraneous variables, results may appear merely coincidental. Since the goal of the experiment is to prove a causal relationship in which a single variable is responsible for the effect produced, the experiment would produce stronger proof if the results were replicated in larger treatment and control groups.
Selecting groups entails assigning subjects in the groups of an experiment in such a way that treatment and control groups are comparable in all respects except the application of the treatment. Groups can be created in two ways: matching and randomization. In the MegaGro experiment discussed below, the plants might be matched according to characteristics such as age, weight and whether they are blooming. This involves distributing these plants so that each plant in one group exactly matches characteristics of plants in the other groups. Matching may be problematic, though, because it "can promote a false sense of security by leading [the experimenter] to believe that [the] experimental and control groups were really equated at the outset, when in fact they were not equated on a host of variables" (Jones, 291). In other words, you may have flowers for your MegaGro experiment that you matched and distributed among groups, but other variables are unaccounted for. It would be difficult to have equal groupings.
Randomization, then, is preferred to matching. This method is based on the statistical principle of normal distribution. Theoretically, any arbitrarily selected group of adequate size will reflect normal distribution. Differences between groups will average out and become more comparable. The principle of normal distribution states that in a population most individuals will fall within the middle range of values for a given characteristic, with increasingly fewer toward either extreme (graphically represented as the ubiquitous "bell curve").
Differences between Quasi-Experimental and Experimental Research
Thus far, we have explained that for experimental research we need:
- a hypothesis for a causal relationship;
- a control group and a treatment group;
- to eliminate confounding variables that might mess up the experiment and prevent displaying the causal relationship; and
- to have larger groups with a carefully sorted constituency; preferably randomized, in order to keep accidental differences from fouling things up.
But what if we don't have all of those? Do we still have an experiment? Not a true experiment in the strictest scientific sense of the term, but we can have a quasi-experiment, an attempt to uncover a causal relationship, even though the researcher cannot control all the factors that might affect the outcome.
A quasi-experimenter treats a given situation as an experiment even though it is not wholly by design. The independent variable may not be manipulated by the researcher, treatment and control groups may not be randomized or matched, or there may be no control group. The researcher is limited in what he or she can say conclusively.
The significant element of both experiments and quasi-experiments is the measure of the dependent variable, which it allows for comparison. Some data is quite straightforward, but other measures, such as level of self-confidence in writing ability, increase in creativity or in reading comprehension are inescapably subjective. In such cases, quasi-experimentation often involves a number of strategies to compare subjectivity, such as rating data, testing, surveying, and content analysis.
Rating essentially is developing a rating scale to evaluate data. In testing, experimenters and quasi-experimenters use ANOVA (Analysis of Variance) and ANCOVA (Analysis of Co-Variance) tests to measure differences between control and experimental groups, as well as different correlations between groups.
Since we're mentioning the subject of statistics, note that experimental or quasi-experimental research cannot state beyond a shadow of a doubt that a single cause will always produce any one effect. They can do no more than show a probability that one thing causes another. The probability that a result is the due to random chance is an important measure of statistical analysis and in experimental research.
Example: Causality
Let's say you want to determine that your new fertilizer, MegaGro, will increase the growth rate of plants. You begin by getting a plant to go with your fertilizer. Since the experiment is concerned with proving that MegaGro works, you need another plant, using no fertilizer at all on it, to compare how much change your fertilized plant displays. This is what is known as a control group.
Set up with a control group, which will receive no treatment, and an experimental group, which will get MegaGro, you must then address those variables that could invalidate your experiment. This can be an extensive and exhaustive process. You must ensure that you use the same plant; that both groups are put in the same kind of soil; that they receive equal amounts of water and sun; that they receive the same amount of exposure to carbon-dioxide-exhaling researchers, and so on. In short, any other variable that might affect the growth of those plants, other than the fertilizer, must be the same for both plants. Otherwise, you can't prove absolutely that MegaGro is the only explanation for the increased growth of one of those plants.
Such an experiment can be done on more than two groups. You may not only want to show that MegaGro is an effective fertilizer, but that it is better than its competitor brand of fertilizer, Plant! All you need to do, then, is have one experimental group receiving MegaGro, one receiving Plant! and the other (the control group) receiving no fertilizer. Those are the only variables that can be different between the three groups; all other variables must be the same for the experiment to be valid.
Controlling variables allows the researcher to identify conditions that may affect the experiment's outcome. This may lead to alternative explanations that the researcher is willing to entertain in order to isolate only variables judged significant. In the MegaGro experiment, you may be concerned with how fertile the soil is, but not with the plants'; relative position in the window, as you don't think that the amount of shade they get will affect their growth rate. But what if it did? You would have to go about eliminating variables in order to determine which is the key factor. What if one receives more shade than the other and the MegaGro plant, which received more shade, died? This might prompt you to formulate a plausible alternative explanation, which is a way of accounting for a result that differs from what you expected. You would then want to redo the study with equal amounts of sunlight.
Methods: Five Steps
Experimental research can be roughly divided into five phases:
Identifying a research problem
The process starts by clearly identifying the problem you want to study and considering what possible methods will affect a solution. Then you choose the method you want to test, and formulate a hypothesis to predict the outcome of the test.
For example, you may want to improve student essays, but you don't believe that teacher feedback is enough. You hypothesize that some possible methods for writing improvement include peer workshopping, or reading more example essays. Favoring the former, your experiment would try to determine if peer workshopping improves writing in high school seniors. You state your hypothesis: peer workshopping prior to turning in a final draft will improve the quality of the student's essay.
Planning an experimental research study
The next step is to devise an experiment to test your hypothesis. In doing so, you must consider several factors. For example, how generalizable do you want your end results to be? Do you want to generalize about the entire population of high school seniors everywhere, or just the particular population of seniors at your specific school? This will determine how simple or complex the experiment will be. The amount of time funding you have will also determine the size of your experiment.
Continuing the example from step one, you may want a small study at one school involving three teachers, each teaching two sections of the same course. The treatment in this experiment is peer workshopping. Each of the three teachers will assign the same essay assignment to both classes; the treatment group will participate in peer workshopping, while the control group will receive only teacher comments on their drafts.
Conducting the experiment
At the start of an experiment, the control and treatment groups must be selected. Whereas the "hard" sciences have the luxury of attempting to create truly equal groups, educators often find themselves forced to conduct their experiments based on self-selected groups, rather than on randomization. As was highlighted in the Basic Concepts section, this makes the study a quasi-experiment, since the researchers cannot control all of the variables.
For the peer workshopping experiment, let's say that it involves six classes and three teachers with a sample of students randomly selected from all the classes. Each teacher will have a class for a control group and a class for a treatment group. The essay assignment is given and the teachers are briefed not to change any of their teaching methods other than the use of peer workshopping. You may see here that this is an effort to control a possible variable: teaching style variance.
Analyzing the data
The fourth step is to collect and analyze the data. This is not solely a step where you collect the papers, read them, and say your methods were a success. You must show how successful. You must devise a scale by which you will evaluate the data you receive, therefore you must decide what indicators will be, and will not be, important.
Continuing our example, the teachers' grades are first recorded, then the essays are evaluated for a change in sentence complexity, syntactical and grammatical errors, and overall length. Any statistical analysis is done at this time if you choose to do any. Notice here that the researcher has made judgments on what signals improved writing. It is not simply a matter of improved teacher grades, but a matter of what the researcher believes constitutes improved use of the language.
Writing the paper/presentation describing the findings
Once you have completed the experiment, you will want to share findings by publishing academic paper (or presentations). These papers usually have the following format, but it is not necessary to follow it strictly. Sections can be combined or not included, depending on the structure of the experiment, and the journal to which you submit your paper.
- Abstract : Summarize the project: its aims, participants, basic methodology, results, and a brief interpretation.
- Introduction : Set the context of the experiment.
- Review of Literature : Provide a review of the literature in the specific area of study to show what work has been done. Should lead directly to the author's purpose for the study.
- Statement of Purpose : Present the problem to be studied.
- Participants : Describe in detail participants involved in the study; e.g., how many, etc. Provide as much information as possible.
- Materials and Procedures : Clearly describe materials and procedures. Provide enough information so that the experiment can be replicated, but not so much information that it becomes unreadable. Include how participants were chosen, the tasks assigned them, how they were conducted, how data were evaluated, etc.
- Results : Present the data in an organized fashion. If it is quantifiable, it is analyzed through statistical means. Avoid interpretation at this time.
- Discussion : After presenting the results, interpret what has happened in the experiment. Base the discussion only on the data collected and as objective an interpretation as possible. Hypothesizing is possible here.
- Limitations : Discuss factors that affect the results. Here, you can speculate how much generalization, or more likely, transferability, is possible based on results. This section is important for quasi-experimentation, since a quasi-experiment cannot control all of the variables that might affect the outcome of a study. You would discuss what variables you could not control.
- Conclusion : Synthesize all of the above sections.
- References : Document works cited in the correct format for the field.
Experimental and Quasi-Experimental Research: Issues and Commentary
Several issues are addressed in this section, including the use of experimental and quasi-experimental research in educational settings, the relevance of the methods to English studies, and ethical concerns regarding the methods.
Using Experimental and Quasi-Experimental Research in Educational Settings
Charting causal relationships in human settings.
Any time a human population is involved, prediction of casual relationships becomes cloudy and, some say, impossible. Many reasons exist for this; for example,
- researchers in classrooms add a disturbing presence, causing students to act abnormally, consciously or unconsciously;
- subjects try to please the researcher, just because of an apparent interest in them (known as the Hawthorne Effect); or, perhaps
- the teacher as researcher is restricted by bias and time pressures.
But such confounding variables don't stop researchers from trying to identify causal relationships in education. Educators naturally experiment anyway, comparing groups, assessing the attributes of each, and making predictions based on an evaluation of alternatives. They look to research to support their intuitive practices, experimenting whenever they try to decide which instruction method will best encourage student improvement.
Combining Theory, Research, and Practice
The goal of educational research lies in combining theory, research, and practice. Educational researchers attempt to establish models of teaching practice, learning styles, curriculum development, and countless other educational issues. The aim is to "try to improve our understanding of education and to strive to find ways to have understanding contribute to the improvement of practice," one writer asserts (Floden 1996, p. 197).
In quasi-experimentation, researchers try to develop models by involving teachers as researchers, employing observational research techniques. Although results of this kind of research are context-dependent and difficult to generalize, they can act as a starting point for further study. The "educational researcher . . . provides guidelines and interpretive material intended to liberate the teacher's intelligence so that whatever artistry in teaching the teacher can achieve will be employed" (Eisner 1992, p. 8).
Bias and Rigor
Critics contend that the educational researcher is inherently biased, sample selection is arbitrary, and replication is impossible. The key to combating such criticism has to do with rigor. Rigor is established through close, proper attention to randomizing groups, time spent on a study, and questioning techniques. This allows more effective application of standards of quantitative research to qualitative research.
Often, teachers cannot wait to for piles of experimentation data to be analyzed before using the teaching methods (Lauer and Asher 1988). They ultimately must assess whether the results of a study in a distant classroom are applicable in their own classrooms. And they must continuously test the effectiveness of their methods by using experimental and qualitative research simultaneously. In addition to statistics (quantitative), researchers may perform case studies or observational research (qualitative) in conjunction with, or prior to, experimentation.
Relevance to English Studies
Situations in english studies that might encourage use of experimental methods.
Whenever a researcher would like to see if a causal relationship exists between groups, experimental and quasi-experimental research can be a viable research tool. Researchers in English Studies might use experimentation when they believe a relationship exists between two variables, and they want to show that these two variables have a significant correlation (or causal relationship).
A benefit of experimentation is the ability to control variables, such as the amount of treatment, when it is given, to whom and so forth. Controlling variables allows researchers to gain insight into the relationships they believe exist. For example, a researcher has an idea that writing under pseudonyms encourages student participation in newsgroups. Researchers can control which students write under pseudonyms and which do not, then measure the outcomes. Researchers can then analyze results and determine if this particular variable alone causes increased participation.
Transferability-Applying Results
Experimentation and quasi-experimentation allow for generating transferable results and accepting those results as being dependent upon experimental rigor. It is an effective alternative to generalizability, which is difficult to rely upon in educational research. English scholars, reading results of experiments with a critical eye, ultimately decide if results will be implemented and how. They may even extend that existing research by replicating experiments in the interest of generating new results and benefiting from multiple perspectives. These results will strengthen the study or discredit findings.
Concerns English Scholars Express about Experiments
Researchers should carefully consider if a particular method is feasible in humanities studies, and whether it will yield the desired information. Some researchers recommend addressing pertinent issues combining several research methods, such as survey, interview, ethnography, case study, content analysis, and experimentation (Lauer and Asher, 1988).
Advantages and Disadvantages of Experimental Research: Discussion
In educational research, experimentation is a way to gain insight into methods of instruction. Although teaching is context specific, results can provide a starting point for further study. Often, a teacher/researcher will have a "gut" feeling about an issue which can be explored through experimentation and looking at causal relationships. Through research intuition can shape practice .
A preconception exists that information obtained through scientific method is free of human inconsistencies. But, since scientific method is a matter of human construction, it is subject to human error . The researcher's personal bias may intrude upon the experiment , as well. For example, certain preconceptions may dictate the course of the research and affect the behavior of the subjects. The issue may be compounded when, although many researchers are aware of the affect that their personal bias exerts on their own research, they are pressured to produce research that is accepted in their field of study as "legitimate" experimental research.
The researcher does bring bias to experimentation, but bias does not limit an ability to be reflective . An ethical researcher thinks critically about results and reports those results after careful reflection. Concerns over bias can be leveled against any research method.
Often, the sample may not be representative of a population, because the researcher does not have an opportunity to ensure a representative sample. For example, subjects could be limited to one location, limited in number, studied under constrained conditions and for too short a time.
Despite such inconsistencies in educational research, the researcher has control over the variables , increasing the possibility of more precisely determining individual effects of each variable. Also, determining interaction between variables is more possible.
Even so, artificial results may result . It can be argued that variables are manipulated so the experiment measures what researchers want to examine; therefore, the results are merely contrived products and have no bearing in material reality. Artificial results are difficult to apply in practical situations, making generalizing from the results of a controlled study questionable. Experimental research essentially first decontextualizes a single question from a "real world" scenario, studies it under controlled conditions, and then tries to recontextualize the results back on the "real world" scenario. Results may be difficult to replicate .
Perhaps, groups in an experiment may not be comparable . Quasi-experimentation in educational research is widespread because not only are many researchers also teachers, but many subjects are also students. With the classroom as laboratory, it is difficult to implement randomizing or matching strategies. Often, students self-select into certain sections of a course on the basis of their own agendas and scheduling needs. Thus when, as often happens, one class is treated and the other used for a control, the groups may not actually be comparable. As one might imagine, people who register for a class which meets three times a week at eleven o'clock in the morning (young, no full-time job, night people) differ significantly from those who register for one on Monday evenings from seven to ten p.m. (older, full-time job, possibly more highly motivated). Each situation presents different variables and your group might be completely different from that in the study. Long-term studies are expensive and hard to reproduce. And although often the same hypotheses are tested by different researchers, various factors complicate attempts to compare or synthesize them. It is nearly impossible to be as rigorous as the natural sciences model dictates.
Even when randomization of students is possible, problems arise. First, depending on the class size and the number of classes, the sample may be too small for the extraneous variables to cancel out. Second, the study population is not strictly a sample, because the population of students registered for a given class at a particular university is obviously not representative of the population of all students at large. For example, students at a suburban private liberal-arts college are typically young, white, and upper-middle class. In contrast, students at an urban community college tend to be older, poorer, and members of a racial minority. The differences can be construed as confounding variables: the first group may have fewer demands on its time, have less self-discipline, and benefit from superior secondary education. The second may have more demands, including a job and/or children, have more self-discipline, but an inferior secondary education. Selecting a population of subjects which is representative of the average of all post-secondary students is also a flawed solution, because the outcome of a treatment involving this group is not necessarily transferable to either the students at a community college or the students at the private college, nor are they universally generalizable.
When a human population is involved, experimental research becomes concerned if behavior can be predicted or studied with validity. Human response can be difficult to measure . Human behavior is dependent on individual responses. Rationalizing behavior through experimentation does not account for the process of thought, making outcomes of that process fallible (Eisenberg, 1996).
Nevertheless, we perform experiments daily anyway . When we brush our teeth every morning, we are experimenting to see if this behavior will result in fewer cavities. We are relying on previous experimentation and we are transferring the experimentation to our daily lives.
Moreover, experimentation can be combined with other research methods to ensure rigor . Other qualitative methods such as case study, ethnography, observational research and interviews can function as preconditions for experimentation or conducted simultaneously to add validity to a study.
We have few alternatives to experimentation. Mere anecdotal research , for example is unscientific, unreplicatable, and easily manipulated. Should we rely on Ed walking into a faculty meeting and telling the story of Sally? Sally screamed, "I love writing!" ten times before she wrote her essay and produced a quality paper. Therefore, all the other faculty members should hear this anecdote and know that all other students should employ this similar technique.
On final disadvantage: frequently, political pressure drives experimentation and forces unreliable results. Specific funding and support may drive the outcomes of experimentation and cause the results to be skewed. The reader of these results may not be aware of these biases and should approach experimentation with a critical eye.
Advantages and Disadvantages of Experimental Research: Quick Reference List
Experimental and quasi-experimental research can be summarized in terms of their advantages and disadvantages. This section combines and elaborates upon many points mentioned previously in this guide.
|
|
gain insight into methods of instruction | subject to human error |
intuitive practice shaped by research | personal bias of researcher may intrude |
teachers have bias but can be reflective | sample may not be representative |
researcher can have control over variables | can produce artificial results |
humans perform experiments anyway | results may only apply to one situation and may be difficult to replicate |
can be combined with other research methods for rigor | groups may not be comparable |
use to determine what is best for population | human response can be difficult to measure |
provides for greater transferability than anecdotal research | political pressure may skew results |
Ethical Concerns
Experimental research may be manipulated on both ends of the spectrum: by researcher and by reader. Researchers who report on experimental research, faced with naive readers of experimental research, encounter ethical concerns. While they are creating an experiment, certain objectives and intended uses of the results might drive and skew it. Looking for specific results, they may ask questions and look at data that support only desired conclusions. Conflicting research findings are ignored as a result. Similarly, researchers, seeking support for a particular plan, look only at findings which support that goal, dismissing conflicting research.
Editors and journals do not publish only trouble-free material. As readers of experiments members of the press might report selected and isolated parts of a study to the public, essentially transferring that data to the general population which may not have been intended by the researcher. Take, for example, oat bran. A few years ago, the press reported how oat bran reduces high blood pressure by reducing cholesterol. But that bit of information was taken out of context. The actual study found that when people ate more oat bran, they reduced their intake of saturated fats high in cholesterol. People started eating oat bran muffins by the ton, assuming a causal relationship when in actuality a number of confounding variables might influence the causal link.
Ultimately, ethical use and reportage of experimentation should be addressed by researchers, reporters and readers alike.
Reporters of experimental research often seek to recognize their audience's level of knowledge and try not to mislead readers. And readers must rely on the author's skill and integrity to point out errors and limitations. The relationship between researcher and reader may not sound like a problem, but after spending months or years on a project to produce no significant results, it may be tempting to manipulate the data to show significant results in order to jockey for grants and tenure.
Meanwhile, the reader may uncritically accept results that receive validity by being published in a journal. However, research that lacks credibility often is not published; consequentially, researchers who fail to publish run the risk of being denied grants, promotions, jobs, and tenure. While few researchers are anything but earnest in their attempts to conduct well-designed experiments and present the results in good faith, rhetorical considerations often dictate a certain minimization of methodological flaws.
Concerns arise if researchers do not report all, or otherwise alter, results. This phenomenon is counterbalanced, however, in that professionals are also rewarded for publishing critiques of others' work. Because the author of an experimental study is in essence making an argument for the existence of a causal relationship, he or she must be concerned not only with its integrity, but also with its presentation. Achieving persuasiveness in any kind of writing involves several elements: choosing a topic of interest, providing convincing evidence for one's argument, using tone and voice to project credibility, and organizing the material in a way that meets expectations for a logical sequence. Of course, what is regarded as pertinent, accepted as evidence, required for credibility, and understood as logical varies according to context. If the experimental researcher hopes to make an impact on the community of professionals in their field, she must attend to the standards and orthodoxy's of that audience.
Related Links
Contrasts: Traditional and computer-supported writing classrooms. This Web presents a discussion of the Transitions Study, a year-long exploration of teachers and students in computer-supported and traditional writing classrooms. Includes description of study, rationale for conducting the study, results and implications of the study.
http://kairos.technorhetoric.net/2.2/features/reflections/page1.htm
Annotated Bibliography
A cozy world of trivial pursuits? (1996, June 28) The Times Educational Supplement . 4174, pp. 14-15.
A critique discounting the current methods Great Britain employs to fund and disseminate educational research. The belief is that research is performed for fellow researchers not the teaching public and implications for day to day practice are never addressed.
Anderson, J. A. (1979, Nov. 10-13). Research as argument: the experimental form. Paper presented at the annual meeting of the Speech Communication Association, San Antonio, TX.
In this paper, the scientist who uses the experimental form does so in order to explain that which is verified through prediction.
Anderson, Linda M. (1979). Classroom-based experimental studies of teaching effectiveness in elementary schools . (Technical Report UTR&D-R- 4102). Austin: Research and Development Center for Teacher Education, University of Texas.
Three recent large-scale experimental studies have built on a database established through several correlational studies of teaching effectiveness in elementary school.
Asher, J. W. (1976). Educational research and evaluation methods . Boston: Little, Brown.
Abstract unavailable by press time.
Babbie, Earl R. (1979). The Practice of Social Research . Belmont, CA: Wadsworth.
A textbook containing discussions of several research methodologies used in social science research.
Bangert-Drowns, R.L. (1993). The word processor as instructional tool: a meta-analysis of word processing in writing instruction. Review of Educational Research, 63 (1), 69-93.
Beach, R. (1993). The effects of between-draft teacher evaluation versus student self-evaluation on high school students' revising of rough drafts. Research in the Teaching of English, 13 , 111-119.
The question of whether teacher evaluation or guided self-evaluation of rough drafts results in increased revision was addressed in Beach's study. Differences in the effects of teacher evaluations, guided self-evaluation (using prepared guidelines,) and no evaluation of rough drafts were examined. The final drafts of students (10th, 11th, and 12th graders) were compared with their rough drafts and rated by judges according to degree of change.
Beishuizen, J. & Moonen, J. (1992). Research in technology enriched schools: a case for cooperation between teachers and researchers . (ERIC Technical Report ED351006).
This paper describes the research strategies employed in the Dutch Technology Enriched Schools project to encourage extensive and intensive use of computers in a small number of secondary schools, and to study the effects of computer use on the classroom, the curriculum, and school administration and management.
Borg, W. P. (1989). Educational Research: an Introduction . (5th ed.). New York: Longman.
An overview of educational research methodology, including literature review and discussion of approaches to research, experimental design, statistical analysis, ethics, and rhetorical presentation of research findings.
Campbell, D. T., & Stanley, J. C. (1963). Experimental and quasi-experimental designs for research . Boston: Houghton Mifflin.
A classic overview of research designs.
Campbell, D.T. (1988). Methodology and epistemology for social science: selected papers . ed. E. S. Overman. Chicago: University of Chicago Press.
This is an overview of Campbell's 40-year career and his work. It covers in seven parts measurement, experimental design, applied social experimentation, interpretive social science, epistemology and sociology of science. Includes an extensive bibliography.
Caporaso, J. A., & Roos, Jr., L. L. (Eds.). Quasi-experimental approaches: Testing theory and evaluating policy. Evanston, WA: Northwestern University Press.
A collection of articles concerned with explicating the underlying assumptions of quasi-experimentation and relating these to true experimentation. With an emphasis on design. Includes a glossary of terms.
Collier, R. Writing and the word processor: How wary of the gift-giver should we be? Unpublished manuscript.
Unpublished typescript. Charts the developments to date in computers and composition and speculates about the future within the framework of Willie Sypher's model of the evolution of creative discovery.
Cook, T.D. & Campbell, D.T. (1979). Quasi-experimentation: design and analysis issues for field settings . Boston: Houghton Mifflin Co.
The authors write that this book "presents some quasi-experimental designs and design features that can be used in many social research settings. The designs serve to probe causal hypotheses about a wide variety of substantive issues in both basic and applied research."
Cutler, A. (1970). An experimental method for semantic field study. Linguistic Communication, 2 , N. pag.
This paper emphasizes the need for empirical research and objective discovery procedures in semantics, and illustrates a method by which these goals may be obtained.
Daniels, L. B. (1996, Summer). Eisenberg's Heisenberg: The indeterminancies of rationality. Curriculum Inquiry, 26 , 181-92.
Places Eisenberg's theories in relation to the death of foundationalism by showing that he distorts rational studies into a form of relativism. He looks at Eisenberg's ideas on indeterminacy, methods and evidence, what he is against and what we should think of what he says.
Danziger, K. (1990). Constructing the subject: Historical origins of psychological research. Cambridge: Cambridge University Press.
Danzinger stresses the importance of being aware of the framework in which research operates and of the essentially social nature of scientific activity.
Diener, E., et al. (1972, December). Leakage of experimental information to potential future subjects by debriefed subjects. Journal of Experimental Research in Personality , 264-67.
Research regarding research: an investigation of the effects on the outcome of an experiment in which information about the experiment had been leaked to subjects. The study concludes that such leakage is not a significant problem.
Dudley-Marling, C., & Rhodes, L. K. (1989). Reflecting on a close encounter with experimental research. Canadian Journal of English Language Arts. 12 , 24-28.
Researchers, Dudley-Marling and Rhodes, address some problems they met in their experimental approach to a study of reading comprehension. This article discusses the limitations of experimental research, and presents an alternative to experimental or quantitative research.
Edgington, E. S. (1985). Random assignment and experimental research. Educational Administration Quarterly, 21 , N. pag.
Edgington explores ways on which random assignment can be a part of field studies. The author discusses both non-experimental and experimental research and the need for using random assignment.
Eisenberg, J. (1996, Summer). Response to critiques by R. Floden, J. Zeuli, and L. Daniels. Curriculum Inquiry, 26 , 199-201.
A response to critiques of his argument that rational educational research methods are at best suspect and at worst futile. He believes indeterminacy controls this method and worries that chaotic research is failing students.
Eisner, E. (1992, July). Are all causal claims positivistic? A reply to Francis Schrag. Educational Researcher, 21 (5), 8-9.
Eisner responds to Schrag who claimed that critics like Eisner cannot escape a positivistic paradigm whatever attempts they make to do so. Eisner argues that Schrag essentially misses the point for trying to argue for the paradigm solely on the basis of cause and effect without including the rest of positivistic philosophy. This weakens his argument against multiple modal methods, which Eisner argues provides opportunities to apply the appropriate research design where it is most applicable.
Floden, R.E. (1996, Summer). Educational research: limited, but worthwhile and maybe a bargain. (response to J.A. Eisenberg). Curriculum Inquiry, 26 , 193-7.
Responds to John Eisenberg critique of educational research by asserting the connection between improvement of practice and research results. He places high value of teacher discrepancy and knowledge that research informs practice.
Fortune, J. C., & Hutson, B. A. (1994, March/April). Selecting models for measuring change when true experimental conditions do not exist. Journal of Educational Research, 197-206.
This article reviews methods for minimizing the effects of nonideal experimental conditions by optimally organizing models for the measurement of change.
Fox, R. F. (1980). Treatment of writing apprehension and tts effects on composition. Research in the Teaching of English, 14 , 39-49.
The main purpose of Fox's study was to investigate the effects of two methods of teaching writing on writing apprehension among entry level composition students, A conventional teaching procedure was used with a control group, while a workshop method was employed with the treatment group.
Gadamer, H-G. (1976). Philosophical hermeneutics . (D. E. Linge, Trans.). Berkeley, CA: University of California Press.
A collection of essays with the common themes of the mediation of experience through language, the impossibility of objectivity, and the importance of context in interpretation.
Gaise, S. J. (1981). Experimental vs. non-experimental research on classroom second language learning. Bilingual Education Paper Series, 5 , N. pag.
Aims on classroom-centered research on second language learning and teaching are considered and contrasted with the experimental approach.
Giordano, G. (1983). Commentary: Is experimental research snowing us? Journal of Reading, 27 , 5-7.
Do educational research findings actually benefit teachers and students? Giordano states his opinion that research may be helpful to teaching, but is not essential and often is unnecessary.
Goldenson, D. R. (1978, March). An alternative view about the role of the secondary school in political socialization: A field-experimental study of theory and research in social education. Theory and Research in Social Education , 44-72.
This study concludes that when political discussion among experimental groups of secondary school students is led by a teacher, the degree to which the students' views were impacted is proportional to the credibility of the teacher.
Grossman, J., and J. P. Tierney. (1993, October). The fallibility of comparison groups. Evaluation Review , 556-71.
Grossman and Tierney present evidence to suggest that comparison groups are not the same as nontreatment groups.
Harnisch, D. L. (1992). Human judgment and the logic of evidence: A critical examination of research methods in special education transition literature. In D. L. Harnisch et al. (Eds.), Selected readings in transition.
This chapter describes several common types of research studies in special education transition literature and the threats to their validity.
Hawisher, G. E. (1989). Research and recommendations for computers and composition. In G. Hawisher and C. Selfe. (Eds.), Critical Perspectives on Computers and Composition Instruction . (pp. 44-69). New York: Teacher's College Press.
An overview of research in computers and composition to date. Includes a synthesis grid of experimental research.
Hillocks, G. Jr. (1982). The interaction of instruction, teacher comment, and revision in teaching the composing process. Research in the Teaching of English, 16 , 261-278.
Hillock conducted a study using three treatments: observational or data collecting activities prior to writing, use of revisions or absence of same, and either brief or lengthy teacher comments to identify effective methods of teaching composition to seventh and eighth graders.
Jenkinson, J. C. (1989). Research design in the experimental study of intellectual disability. International Journal of Disability, Development, and Education, 69-84.
This article catalogues the difficulties of conducting experimental research where the subjects are intellectually disables and suggests alternative research strategies.
Jones, R. A. (1985). Research Methods in the Social and Behavioral Sciences. Sunderland, MA: Sinauer Associates, Inc..
A textbook designed to provide an overview of research strategies in the social sciences, including survey, content analysis, ethnographic approaches, and experimentation. The author emphasizes the importance of applying strategies appropriately and in variety.
Kamil, M. L., Langer, J. A., & Shanahan, T. (1985). Understanding research in reading and writing . Newton, Massachusetts: Allyn and Bacon.
Examines a wide variety of problems in reading and writing, with a broad range of techniques, from different perspectives.
Kennedy, J. L. (1985). An Introduction to the Design and Analysis of Experiments in Behavioral Research . Lanham, MD: University Press of America.
An introductory textbook of psychological and educational research.
Keppel, G. (1991). Design and analysis: a researcher's handbook . Englewood Cliffs, NJ: Prentice Hall.
This updates Keppel's earlier book subtitled "a student's handbook." Focuses on extensive information about analytical research and gives a basic picture of research in psychology. Covers a range of statistical topics. Includes a subject and name index, as well as a glossary.
Knowles, G., Elija, R., & Broadwater, K. (1996, Spring/Summer). Teacher research: enhancing the preparation of teachers? Teaching Education, 8 , 123-31.
Researchers looked at one teacher candidate who participated in a class which designed their own research project correlating to a question they would like answered in the teaching world. The goal of the study was to see if preservice teachers developed reflective practice by researching appropriate classroom contexts.
Lace, J., & De Corte, E. (1986, April 16-20). Research on media in western Europe: A myth of sisyphus? Paper presented at the annual meeting of the American Educational Research Association. San Francisco.
Identifies main trends in media research in western Europe, with emphasis on three successive stages since 1960: tools technology, systems technology, and reflective technology.
Latta, A. (1996, Spring/Summer). Teacher as researcher: selected resources. Teaching Education, 8 , 155-60.
An annotated bibliography on educational research including milestones of thought, practical applications, successful outcomes, seminal works, and immediate practical applications.
Lauer. J.M. & Asher, J. W. (1988). Composition research: Empirical designs . New York: Oxford University Press.
Approaching experimentation from a humanist's perspective to it, authors focus on eight major research designs: Case studies, ethnographies, sampling and surveys, quantitative descriptive studies, measurement, true experiments, quasi-experiments, meta-analyses, and program evaluations. It takes on the challenge of bridging language of social science with that of the humanist. Includes name and subject indexes, as well as a glossary and a glossary of symbols.
Mishler, E. G. (1979). Meaning in context: Is there any other kind? Harvard Educational Review, 49 , 1-19.
Contextual importance has been largely ignored by traditional research approaches in social/behavioral sciences and in their application to the education field. Developmental and social psychologists have increasingly noted the inadequacies of this approach. Drawing examples for phenomenology, sociolinguistics, and ethnomethodology, the author proposes alternative approaches for studying meaning in context.
Mitroff, I., & Bonoma, T. V. (1978, May). Psychological assumptions, experimentations, and real world problems: A critique and an alternate approach to evaluation. Evaluation Quarterly , 235-60.
The authors advance the notion of dialectic as a means to clarify and examine the underlying assumptions of experimental research methodology, both in highly controlled situations and in social evaluation.
Muller, E. W. (1985). Application of experimental and quasi-experimental research designs to educational software evaluation. Educational Technology, 25 , 27-31.
Muller proposes a set of guidelines for the use of experimental and quasi-experimental methods of research in evaluating educational software. By obtaining empirical evidence of student performance, it is possible to evaluate if programs are making the desired learning effect.
Murray, S., et al. (1979, April 8-12). Technical issues as threats to internal validity of experimental and quasi-experimental designs . San Francisco: University of California.
The article reviews three evaluation models and analyzes the flaws common to them. Remedies are suggested.
Muter, P., & Maurutto, P. (1991). Reading and skimming from computer screens and books: The paperless office revisited? Behavior and Information Technology, 10 (4), 257-66.
The researchers test for reading and skimming effectiveness, defined as accuracy combined with speed, for written text compared to text on a computer monitor. They conclude that, given optimal on-line conditions, both are equally effective.
O'Donnell, A., Et al. (1992). The impact of cooperative writing. In J. R. Hayes, et al. (Eds.). Reading empirical research studies: The rhetoric of research . (pp. 371-84). Hillsdale, NJ: Lawrence Erlbaum Associates.
A model of experimental design. The authors investigate the efficacy of cooperative writing strategies, as well as the transferability of skills learned to other, individual writing situations.
Palmer, D. (1988). Looking at philosophy . Mountain View, CA: Mayfield Publishing.
An introductory text with incisive but understandable discussions of the major movements and thinkers in philosophy from the Pre-Socratics through Sartre. With illustrations by the author. Includes a glossary.
Phelps-Gunn, T., & Phelps-Terasaki, D. (1982). Written language instruction: Theory and remediation . London: Aspen Systems Corporation.
The lack of research in written expression is addressed and an application on the Total Writing Process Model is presented.
Poetter, T. (1996, Spring/Summer). From resistance to excitement: becoming qualitative researchers and reflective practitioners. Teaching Education , 8109-19.
An education professor reveals his own problematic research when he attempted to institute a educational research component to a teacher preparation program. He encountered dissent from students and cooperating professionals and ultimately was rewarded with excitement towards research and a recognized correlation to practice.
Purves, A. C. (1992). Reflections on research and assessment in written composition. Research in the Teaching of English, 26 .
Three issues concerning research and assessment is writing are discussed: 1) School writing is a matter of products not process, 2) school writing is an ill-defined domain, 3) the quality of school writing is what observers report they see. Purves discusses these issues while looking at data collected in a ten-year study of achievement in written composition in fourteen countries.
Rathus, S. A. (1987). Psychology . (3rd ed.). Poughkeepsie, NY: Holt, Rinehart, and Winston.
An introductory psychology textbook. Includes overviews of the major movements in psychology, discussions of prominent examples of experimental research, and a basic explanation of relevant physiological factors. With chapter summaries.
Reiser, R. A. (1982). Improving the research skills of instructional designers. Educational Technology, 22 , 19-21.
In his paper, Reiser starts by stating the importance of research in advancing the field of education, and points out that graduate students in instructional design lack the proper skills to conduct research. The paper then goes on to outline the practicum in the Instructional Systems Program at Florida State University which includes: 1) Planning and conducting an experimental research study; 2) writing the manuscript describing the study; 3) giving an oral presentation in which they describe their research findings.
Report on education research . (Journal). Washington, DC: Capitol Publication, Education News Services Division.
This is an independent bi-weekly newsletter on research in education and learning. It has been publishing since Sept. 1969.
Rossell, C. H. (1986). Why is bilingual education research so bad?: Critique of the Walsh and Carballo study of Massachusetts bilingual education programs . Boston: Center for Applied Social Science, Boston University. (ERIC Working Paper 86-5).
The Walsh and Carballo evaluation of the effectiveness of transitional bilingual education programs in five Massachusetts communities has five flaws and the five flaws are discussed in detail.
Rubin, D. L., & Greene, K. (1992). Gender-typical style in written language. Research in the Teaching of English, 26.
This study was designed to find out whether the writing styles of men and women differ. Rubin and Green discuss the pre-suppositions that women are better writers than men.
Sawin, E. (1992). Reaction: Experimental research in the context of other methods. School of Education Review, 4 , 18-21.
Sawin responds to Gage's article on methodologies and issues in educational research. He agrees with most of the article but suggests the concept of scientific should not be regarded in absolute terms and recommends more emphasis on scientific method. He also questions the value of experiments over other types of research.
Schoonmaker, W. E. (1984). Improving classroom instruction: A model for experimental research. The Technology Teacher, 44, 24-25.
The model outlined in this article tries to bridge the gap between classroom practice and laboratory research, using what Schoonmaker calls active research. Research is conducted in the classroom with the students and is used to determine which two methods of classroom instruction chosen by the teacher is more effective.
Schrag, F. (1992). In defense of positivist research paradigms. Educational Researcher, 21, (5), 5-8.
The controversial defense of the use of positivistic research methods to evaluate educational strategies; the author takes on Eisner, Erickson, and Popkewitz.
Smith, J. (1997). The stories educational researchers tell about themselves. Educational Researcher, 33 (3), 4-11.
Recapitulates main features of an on-going debate between advocates for using vocabularies of traditional language arts and whole language in educational research. An "impasse" exists were advocates "do not share a theoretical disposition concerning both language instruction and the nature of research," Smith writes (p. 6). He includes a very comprehensive history of the debate of traditional research methodology and qualitative methods and vocabularies. Definitely worth a read by graduates.
Smith, N. L. (1980). The feasibility and desirability of experimental methods in evaluation. Evaluation and Program Planning: An International Journal , 251-55.
Smith identifies the conditions under which experimental research is most desirable. Includes a review of current thinking and controversies.
Stewart, N. R., & Johnson, R. G. (1986, March 16-20). An evaluation of experimental methodology in counseling and counselor education research. Paper presented at the annual meeting of the American Educational Research Association, San Francisco.
The purpose of this study was to evaluate the quality of experimental research in counseling and counselor education published from 1976 through 1984.
Spector, P. E. (1990). Research Designs. Newbury Park, California: Sage Publications.
In this book, Spector introduces the basic principles of experimental and nonexperimental design in the social sciences.
Tait, P. E. (1984). Do-it-yourself evaluation of experimental research. Journal of Visual Impairment and Blindness, 78 , 356-363 .
Tait's goal is to provide the reader who is unfamiliar with experimental research or statistics with the basic skills necessary for the evaluation of research studies.
Walsh, S. M. (1990). The current conflict between case study and experimental research: A breakthrough study derives benefits from both . (ERIC Document Number ED339721).
This paper describes a study that was not experimentally designed, but its major findings were generalizable to the overall population of writers in college freshman composition classes. The study was not a case study, but it provided insights into the attitudes and feelings of small clusters of student writers.
Waters, G. R. (1976). Experimental designs in communication research. Journal of Business Communication, 14 .
The paper presents a series of discussions on the general elements of experimental design and the scientific process and relates these elements to the field of communication.
Welch, W. W. (March 1969). The selection of a national random sample of teachers for experimental curriculum evaluation. Scholastic Science and Math , 210-216.
Members of the evaluation section of Harvard project physics describe what is said to be the first attempt to select a national random sample of teachers, and list 6 steps to do so. Cost and comparison with a volunteer group are also discussed.
Winer, B.J. (1971). Statistical principles in experimental design , (2nd ed.). New York: McGraw-Hill.
Combines theory and application discussions to give readers a better understanding of the logic behind statistical aspects of experimental design. Introduces the broad topic of design, then goes into considerable detail. Not for light reading. Bring your aspirin if you like statistics. Bring morphine is you're a humanist.
Winn, B. (1986, January 16-21). Emerging trends in educational technology research. Paper presented at the Annual Convention of the Association for Educational Communication Technology.
This examination of the topic of research in educational technology addresses four major areas: (1) why research is conducted in this area and the characteristics of that research; (2) the types of research questions that should or should not be addressed; (3) the most appropriate methodologies for finding answers to research questions; and (4) the characteristics of a research report that make it good and ultimately suitable for publication.
Citation Information
Luann Barnes, Jennifer Hauser, Luana Heikes, Anthony J. Hernandez, Paul Tim Richard, Katherine Ross, Guo Hua Yang, and Mike Palmquist. (1994-2024). Experimental and Quasi-Experimental Research. The WAC Clearinghouse. Colorado State University. Available at https://wac.colostate.edu/repository/writing/guides/.
Copyright Information
Copyright © 1994-2024 Colorado State University and/or this site's authors, developers, and contributors . Some material displayed on this site is used with permission.

An official website of the United States government
The .gov means it’s official. Federal government websites often end in .gov or .mil. Before sharing sensitive information, make sure you’re on a federal government site.
The site is secure. The https:// ensures that you are connecting to the official website and that any information you provide is encrypted and transmitted securely.
- Publications
- Account settings
Preview improvements coming to the PMC website in October 2024. Learn More or Try it out now .
- Advanced Search
- Journal List
- HHS Author Manuscripts

Experimental and Quasi-Experimental Designs in Implementation Research
Christopher j. miller.
a VA Boston Healthcare System, Center for Healthcare Organization and Implementation Research (CHOIR), United States Department of Veterans Affairs, Boston, MA, USA
b Department of Psychiatry, Harvard Medical School, Boston, MA, USA
Shawna N. Smith
c Department of Psychiatry, University of Michigan Medical School, Ann Arbor, MI, USA
d Survey Research Center, Institute for Social Research, University of Michigan, Ann Arbor, MI, USA
Marianne Pugatch
Implementation science is focused on maximizing the adoption, appropriate use, and sustainability of effective clinical practices in real world clinical settings. Many implementation science questions can be feasibly answered by fully experimental designs, typically in the form of randomized controlled trials (RCTs). Implementation-focused RCTs, however, usually differ from traditional efficacy- or effectiveness-oriented RCTs on key parameters. Other implementation science questions are more suited to quasi-experimental designs, which are intended to estimate the effect of an intervention in the absence of randomization. These designs include pre-post designs with a non-equivalent control group, interrupted time series (ITS), and stepped wedges, the last of which require all participants to receive the intervention, but in a staggered fashion. In this article we review the use of experimental designs in implementation science, including recent methodological advances for implementation studies. We also review the use of quasi-experimental designs in implementation science, and discuss the strengths and weaknesses of these approaches. This article is therefore meant to be a practical guide for researchers who are interested in selecting the most appropriate study design to answer relevant implementation science questions, and thereby increase the rate at which effective clinical practices are adopted, spread, and sustained.
1. Background
The first documented clinical trial was conducted in 1747 by James Lind, a royal navy physician, who tested the hypothesis that citrus fruit could cure scurvy. Since then, based on foundational work by Fisher and others (1935), the randomized controlled trial (RCT) has emerged as the gold standard for testing the efficacy of treatment versus a control condition for individual patients. Randomization of patients is seen as a crucial to reducing the impact of measured or unmeasured confounding variables, in turn allowing researchers to draw conclusions regarding causality in clinical trials.
As described elsewhere in this special issue, implementation science is ultimately focused on maximizing the adoption, appropriate use, and sustainability of effective clinical practices in real world clinical settings. As such, some implementation science questions may be addressed by experimental designs. For our purposes here, we use the term “experimental” to refer to designs that feature two essential ingredients: first, manipulation of an independent variable; and second, random assignment of subjects. This corresponds to the definition of randomized experiments originally championed by Fisher (1925) . From this perspective, experimental designs usually take the form of RCTs—but implementation- oriented RCTs typically differ in important ways from traditional efficacy- or effectiveness-oriented RCTs. Other implementation science questions require different methodologies entirely: specifically, several forms of quasi-experimental designs may be used for implementation research in situations where an RCT would be inappropriate. These designs are intended to estimate the effect of an intervention despite a lack of randomization. Quasi-experimental designs include pre-post designs with a nonequivalent control group, interrupted time series (ITS), and stepped wedge designs. Stepped wedges are studies in which all participants receive the intervention, but in a staggered fashion. It is important to note that quasi-experimental designs are not unique to implementation science. As we will discuss below, however, each of them has strengths that make them particularly useful in certain implementation science contexts.
Our goal for this manuscript is two-fold. First, we will summarize the use of experimental designs in implementation science. This will include discussion of ways that implementation-focused RCTs may differ from efficacy- or effectiveness-oriented RCTs. Second, we will summarize the use of quasi-experimental designs in implementation research. This will include discussion of the strengths and weaknesses of these types of approaches in answering implementation research questions. For both experimental and quasi-experimental designs, we will discuss a recent implementation study as an illustrative example of one approach.
1. Experimental Designs in Implementation Science
RCTs in implementation science share the same basic structure as efficacy- or effectiveness-oriented RCTs, but typically feature important distinctions. In this section we will start by reviewing key factors that separate implementation RCTs from more traditional efficacy- or effectiveness-oriented RCTs. We will then discuss optimization trials, which are a type of experimental design that is especially useful for certain implementation science questions. We will then briefly turn our attention to single subject experimental designs (SSEDs) and on-off-on (ABA) designs.
The first common difference that sets apart implementation RCTs from more traditional clinical trials is the primary research question they aim to address. For most implementation trials, the primary research question is not the extent to which a particular treatment or evidence-based practice is more effective than a comparison condition, but instead the extent to which a given implementation strategy is more effective than a comparison condition. For more detail on this pivotal issue, see Drs. Bauer and Kirchner in this special issue.
Second, as a corollary of this point, implementation RCTs typically feature different outcome measures than efficacy or effectiveness RCTs, with an emphasis on the extent to which a health intervention was successfully implemented rather than an evaluation of the health effects of that intervention ( Proctor et al., 2011 ). For example, typical implementation outcomes might include the number of patients who receive the intervention, or the number of providers who administer the intervention as intended. A variety of evaluation-oriented implementation frameworks may guide the choices of such measures (e.g. RE-AIM; Gaglio et al., 2013 ; Glasgow et al., 1999 ). Hybrid implementation-effectiveness studies attend to both effectiveness and implementation outcomes ( Curran et al., 2012 ); these designs are also covered in more detail elsewhere in this issue (Landes, this issue).
Third, given their focus, implementation RCTs are frequently cluster-randomized (i.e. with sites or clinics as the unit of randomization, and patients nested within those sites or clinics). For example, consider a hypothetical RCT that aims to evaluate the implementation of a training program for cognitive behavioral therapy (CBT) in community clinics. Randomizing at the patient level for such a trial would be inappropriate due to the risk of contamination, as providers trained in CBT might reasonably be expected to incorporate CBT principles into their treatment even to patients assigned to the control condition. Randomizing at the provider level would also risk contamination, as providers trained in CBT might discuss this treatment approach with their colleagues. Thus, many implementation trials are cluster randomized at the site or clinic level. While such clustering minimizes the risk of contamination, it can unfortunately create commensurate problems with confounding, especially for trials with very few sites to randomize. Stratification may be used to at least partially address confounding issues in cluster- randomized and more traditional trials alike, by ensuring that intervention and control groups are broadly similar on certain key variables. Furthermore, such allocation schemes typically require analytic models that account for this clustering and the resulting correlations among error structures (e.g., generalized estimating equations [GEE] or mixed-effects models; Schildcrout et al., 2018 ).
1.1. Optimization trials
Key research questions in implementation science often involve determining which implementation strategies to provide, to whom, and when, to achieve optimal implementation success. As such, trials designed to evaluate comparative effectiveness, or to optimize provision of different types or intensities of implementation strategies, may be more appealing than traditional effectiveness trials. The methods described in this section are not unique to implementation science, but their application in the context of implementation trials may be particularly useful for informing implementation strategies.
While two-arm RCTs can be used to evaluate comparative effectiveness, trials focused on optimizing implementation support may use alternative experimental designs ( Collins et al., 2005 ; Collins et al., 2007 ). For example, in certain clinical contexts, multi-component “bundles” of implementation strategies may be warranted (e.g. a bundle consisting of clinician training, technical assistance, and audit/feedback to encourage clinicians to use a new evidence-based practice). In these situations, implementation researchers might consider using factorial or fractional-factorial designs. In the context of implementation science, these designs randomize participants (e.g. sites or providers) to different combinations of implementation strategies, and can be used to evaluate the effectiveness of each strategy individually to inform an optimal combination (e.g. Coulton et al., 2009 ; Pellegrini et al., 2014 ; Wyrick, et al., 2014 ). Such designs can be particularly useful in informing multi-component implementation strategies that are not redundant or overly burdensome ( Collins et al., 2014a ; Collins et al., 2009 ; Collins et al., 2007 ).
Researchers interested in optimizing sequences of implementation strategies that adapt to ongoing needs over time may be interested in a variant of factorial designs known as the sequential, multiple-assignment randomized trial (SMART; Almirall et al., 2012 ; Collins et al., 2014b ; Kilbourne et al., 2014b ; Lei et al., 2012 ; Nahum-Shani et al., 2012 ; NeCamp et al., 2017 ). SMARTs are multistage randomized trials in which some or all participants are randomized more than once, often based on ongoing information (e.g., treatment response). In implementation research, SMARTs can inform optimal sequences of implementation strategies to maximize downstream clinical outcomes. Thus, such designs are well-suited to answering questions about what implementation strategies should be used, in what order, to achieve the best outcomes in a given context.
One example of an implementation SMART is the Adaptive Implementation of Effective Program Trial (ADEPT; Kilbourne et al., 2014a ). ADEPT was a clustered SMART ( NeCamp et al., 2017 ) designed to inform an adaptive sequence of implementation strategies for implementing an evidence-based collaborative chronic care model, Life Goals ( Kilbourne et al., 2014c ; Kilbourne et al., 2012a ), into community-based practices. Life Goals, the clinical intervention being implemented, has proven effective at improving physical and mental health outcomes for patients with unipolar and bipolar depression by encouraging providers to instruct patients in self-management, and improving clinical information systems and care management across physical and mental health providers ( Bauer et al., 2006 ; Kilbourne et al., 2012a ; Kilbourne et al., 2008 ; Simon et al., 2006 ). However, in spite of its established clinical effectiveness, community-based clinics experienced a number of barriers in trying to implement the Life Goals model, and there were questions about how best to efficiently and effectively augment implementation strategies for clinics that struggled with implementation.
The ADEPT study was thus designed to determine the best sequence of implementation strategies to offer sites interested in implementing Life Goals. The ADEPT study involved use of three different implementation strategies. First, all sites received implementation support based on Replicating Effective Programs (REP), which offered an implementation manual, brief training, and low- level technical support ( Kilbourne et al., 2007 ; Kilbourne et al., 2012b ; Neumann and Sogolow, 2000 ). REP implementation support had been previously found to be low-cost and readily scalable, but also insufficient for uptake for many community-based settings ( Kilbourne et al., 2015 ). For sites that failed to implement Life Goals under REP, two additional implementation strategies were considered as augmentations to REP: External Facilitation (EF; Kilbourne et al., 2014b ; Stetler et al., 2006 ), consisting of phone-based mentoring in strategic skills from a study team member; and Internal Facilitation (IF; Kirchner et al., 2014 ), which supported protected time for a site employee to address barriers to program adoption.
The ADEPT study was designed to evaluate the best way to augment support for these sites that were not able to implement Life Goals under REP, specifically querying whether it was better to augment REP with EF only or the more intensive EF/IF, and whether augmentations should be provided all at once, or staged. Intervention assignments are mapped in Figure 1 . Seventy-nine community-based clinics across Michigan and Colorado were provided with initial implementation support under REP. After six months, implementation of the clinical intervention, Life Goals, was evaluated at all sites. Sites that had failed to reach an adequate level of delivery (defined as those sites enrolling fewer than ten patients in Life Goals, or those at which fewer than 50% of enrolled patients had received at least three Life Goals sessions) were considered non-responsive to REP and randomized to receive additional support through either EF or combined EF/IF. After six further months, Life Goals implementation at these sites was again evaluated. Sites surpassing the implementation response benchmark had their EF or EF/IF support discontinued. EF/IF sites that remained non-responsive continued to receive EF/IF for an additional six months. EF sites that remained non-responsive were randomized a second time to either continue with EF or further augment with IF. This design thus allowed for comparison of three different adaptive implementation interventions for sites that were initially non-responsive to REP to determine the best adaptive sequence of implementation support for sites that were initially non-responsive under REP:

SMART design from ADEPT trial.
- Provide EF for 6 months; continue EF for a further six months for sites that remain nonresponsive; discontinue EF for sites that are responsive;
- Provide EF/IF for 6 months; continue EF/IF for a further six months for sites that remain non-responsive; discontinue EF/IF for sites that are responsive; and
- Provide EF for 6 months; step up to EF/IF for a further six months for sites that remain non-responsive; discontinue EF for sites that are responsive.
While analyses of this study are still ongoing, including the comparison of these three adaptive sequences of implementation strategies, results have shown that patients at sites that were randomized to receive EF as the initial augmentation to REP saw more improvement in clinical outcomes (SF-12 mental health quality of life and PHQ-9 depression scores) after 12 months than patients at sites that were randomized to receive the more intensive EF/IF augmentation.
1.2. Single Subject Experimental Designs and On-Off-On (ABA) Designs
We also note that there are a variety of Single Subject Experimental Designs (SSEDs; Byiers et al., 2012 ), including withdrawal designs and alternating treatment designs, that can be used in testing evidence-based practices. Similarly, an implementation strategy may be used to encourage the use of a specific treatment at a particular site, followed by that strategy’s withdrawal and subsequent reinstatement, with data collection throughout the process (on-off-on or ABA design). A weakness of these approaches in the context of implementation science, however, is that they usually require reversibility of the intervention (i.e. that the withdrawal of implementation support truly allows the healthcare system to revert to its pre-implementation state). When this is not the case—for example, if a hypothetical study is focused on training to encourage use of an evidence-based psychotherapy—then these designs may be less useful.
2. Quasi-Experimental Designs in Implementation Science
In some implementation science contexts, policy-makers or administrators may not be willing to have a subset of participating patients or sites randomized to a control condition, especially for high-profile or high-urgency clinical issues. Quasi-experimental designs allow implementation scientists to conduct rigorous studies in these contexts, albeit with certain limitations. We briefly review the characteristics of these designs here; other recent review articles are available for the interested reader (e.g. Handley et al., 2018 ).
2.1. Pre-Post with Non-Equivalent Control Group
The pre-post with non-equivalent control group uses a control group in the absence of randomization. Ideally, the control group is chosen to be as similar to the intervention group as possible (e.g. by matching on factors such as clinic type, patient population, geographic region, etc.). Theoretically, both groups are exposed to the same trends in the environment, making it plausible to decipher if the intervention had an effect. Measurement of both treatment and control conditions classically occurs pre- and post-intervention, with differential improvement between the groups attributed to the intervention. This design is popular due to its practicality, especially if data collection points can be kept to a minimum. It may be especially useful for capitalizing on naturally occurring experiments such as may occur in the context of certain policy initiatives or rollouts—specifically, rollouts in which it is plausible that a control group can be identified. For example, Kirchner and colleagues (2014) used this type of design to evaluate the integration of mental health services into primary care clinics at seven US Department of Veterans Affairs (VA) medical centers and seven matched controls.
One overarching drawback of this design is that it is especially vulnerable to threats to internal validity ( Shadish, 2002 ), because pre-existing differences between the treatment and control group could erroneously be attributed to the intervention. While unmeasured differences between treatment and control groups are always a possibility in healthcare research, such differences are especially likely to occur in the context of these designs due to the lack of randomization. Similarly, this design is particularly sensitive to secular trends that may differentially affect the treatment and control groups ( Cousins et al., 2014 ; Pape et al., 2013 ), as well as regression to the mean confounding study results ( Morton and Torgerson, 2003 ). For example, if a study site is selected for the experimental condition precisely because it is underperforming in some way, then regression to the mean would suggest that the site will show improvement regardless of any intervention; in the context of a pre-post with non-equivalent control group study, however, this improvement would erroneously be attributed to the intervention itself (Type I error).
There are, however, various ways that implementation scientists can mitigate these weaknesses. First, as mentioned briefly above, it is important to select a control group that is as similar as possible to the intervention site(s), which can include matching at both the health care network and clinic level (e.g. Kirchner et al., 2014 ). Second, propensity score weighting (e.g. Morgan, 2018 ) can statistically mitigate internal validity concerns, although this approach may be of limited utility when comparing secular trends between different study cohorts ( Dimick and Ryan, 2014 ). More broadly, qualitative methods (e.g. periodic interviews with staff at intervention and control sites) can help uncover key contextual factors that may be affecting study results above and beyond the intervention itself.
2.2. Interrupted Time Series
Interrupted time series (ITS; Shadish, 2002 ; Taljaard et al., 2014 ; Wagner et al., 2002 ) designs represent one of the most robust categories of quasi-experimental designs. Rather than relying on a non-equivalent control group, ITS designs rely on repeated data collections from intervention sites to determine whether a particular intervention is associated with improvement on a given metric relative to the pre-intervention secular trend. They are particularly useful in cases where a comparable control group cannot be identified—for example, following widespread implementation of policy mandates, quality improvement initiatives, or dissemination campaigns ( Eccles et al., 2003 ). In ITS designs, data are collected at multiple time points both before and after an intervention (e.g., policy change, implementation effort), and analyses explore whether the intervention was associated with the outcome beyond any pre-existing secular trend. More formally, ITS evaluations focus on identifying whether there is discontinuity in the trend (change in slope or level) after the intervention relative to before the intervention, using segmented regression to model pre- and post-intervention trends ( Gebski et al., 2012 ; Penfold and Zhang, 2013 ; Taljaard et al., 2014 ; Wagner et al., 2002 ). A number of recent implementation studies have used ITS designs, including an evaluation of implementation of a comprehensive smoke-free policy in a large UK mental health organization to reduce physical assaults ( Robson et al., 2017 ); the impact of a national policy limiting alcohol availability on suicide mortality in Slovenia ( Pridemore and Snowden, 2009 ); and the effect of delivery of a tailored intervention for primary care providers to increase psychological referrals for women with mild to moderate postnatal depression ( Hanbury et al., 2013 ).
ITS designs are appealing in implementation work for several reasons. Relative to uncontrolled pre-post analyses, ITS analyses reduce the chances that intervention effects are confounded by secular trends ( Bernal et al., 2017 ; Eccles et al., 2003 ). Time-varying confounders, such as seasonality, can also be adjusted for, provided adequate data ( Bernal et al., 2017 ). Indeed, recent work has confirmed that ITS designs can yield effect estimates similar to those derived from cluster-randomized RCTs ( Fretheim et al., 2013 ; Fretheim et al., 2015 ). Relative to an RCT, ITS designs can also allow for a more comprehensive assessment of the longitudinal effects of an intervention (positive or negative), as effects can be traced over all included time points ( Bernal et al., 2017 ; Penfold and Zhang, 2013 ).
ITS designs also present a number of challenges. First, the segmented regression approach requires clear delineation between pre- and post-intervention periods; interventions with indeterminate implementation periods are likely not good candidates for ITS. While ITS designs that include multiple ‘interruptions’ (e.g. introductions of new treatment components) are possible, they will require collection of enough time points between interruptions to ensure that each intervention’s effects can be ascertained individually ( Bernal et al., 2017 ). Second, collecting data from sufficient time points across all sites of interest, especially for the pre-intervention period, can be challenging ( Eccles et al., 2003 ): a common recommendation is at least eight time points both pre- and post-intervention ( Penfold and Zhang, 2013 ). This may be onerous, particularly if the data are not routinely collected by the health system(s) under study. Third, ITS cannot protect against confounding effects from other interventions that begin contemporaneously and may impact similar outcomes ( Eccles et al., 2003 ).
2.3. Stepped Wedge Designs
Stepped wedge trials are another type of quasi-experimental design. In a stepped wedge, all participants receive the intervention, but are assigned to the timing of the intervention in a staggered fashion ( Betran et al., 2018 ; Brown and Lilford, 2006 ; Hussey and Hughes, 2007 ), typically at the site or cluster level. Stepped wedge designs have their analytic roots in balanced incomplete block designs, in which all pairs of treatments occur an equal number of times within each block ( Hanani, 1961 ). Traditionally, all sites in stepped wedge trials have outcome measures assessed at all time points, thus allowing sites that receive the intervention later in the trial to essentially serve as controls for early intervention sites. A recent special issue of the journal Trials includes more detail on these designs ( Davey et al., 2015 ), which may be ideal for situations in which it is important for all participating patients or sites to receive the intervention during the trial. Stepped wedge trials may also be useful when resources are scarce enough that intervening at all sites at once (or even half of the sites as in a standard treatment-versus-control RCT) would not be feasible. If desired, the administration of the intervention to sites in waves allows for lessons learned in early sites to be applied to later sites (via formative evaluation; see Elwy et al., this issue).
The Behavioral Health Interdisciplinary Program (BHIP) Enhancement Project is a recent example of a stepped-wedge implementation trial ( Bauer et al., 2016 ; Bauer et al., 2019 ). This study involved using blended facilitation (including internal and external facilitators; Kirchner et al., 2014 ) to implement care practices consistent with the collaborative chronic care model (CCM; Bodenheimer et al., 2002a , b ; Wagner et al., 1996 ) in nine outpatient mental health teams in VA medical centers. Figure 2 illustrates the implementation and stepdown periods for that trial, with black dots representing primary data collection points.

BHIP Enhancement Project stepped wedge (adapted form Bauer et al., 2019).
The BHIP Enhancement Project was conducted as a stepped wedge for several reasons. First, the stepped wedge design allowed the trial to reach nine sites despite limited implementation resources (i.e. intervening at all nine sites simultaneously would not have been feasible given study funding). Second, the stepped wedge design aided in recruitment and retention, as all participating sites were certain to receive implementation support during the trial: at worst, sites that were randomized to later- phase implementation had to endure waiting periods totaling about eight months before implementation began. This was seen as a major strength of the design by its operational partner, the VA Office of Mental Health and Suicide Prevention. To keep sites engaged during the waiting period, the BHIP Enhancement Project offered a guiding workbook and monthly technical support conference calls.
Three additional features of the BHIP Enhancement Project deserve special attention. First, data collection for late-implementing sites did not begin until immediately before the onset of implementation support (see Figure 2 ). While this reduced statistical power, it also significantly reduced data collection burden on the study team. Second, onset of implementation support was staggered such that wave 2 began at the end of month 4 rather than month 6. This had two benefits: first, this compressed the overall amount of time required for implementation during the trial. Second, it meant that the study team only had to collect data from one site at a time, with data collection periods coming every 2–4 months. More traditional stepped wedge approaches typically have data collection across sites temporally aligned (e.g. Betran et al., 2018 ). Third, the BHIP Enhancement Project used a balancing algorithm ( Lew et al., 2019 ) to assign sites to waves, retaining some of the benefits of randomization while ensuring balance on key site characteristics (e.g. size, geographic region).
Despite their utility, stepped wedges have some important limitations. First, because they feature delayed implementation at some sites, stepped wedges typically take longer than similarly-sized parallel group RCTs. This increases the chances that secular trends, policy changes, or other external forces impact study results. Second, as with RCTs, imbalanced site assignment can confound results. This may occur deliberately in some cases—for example, if sites that develop their implementation plans first are assigned to earlier waves. Even if sites are randomized, however, early and late wave sites may still differ on important characteristics such as size, rurality, and case mix. The resulting confounding between site assignment and time can threaten the internal validity of the study—although, as above, balancing algorithms can reduce this risk. Third, the use of formative evaluation (Elwy, this issue), while useful for maximizing the utility of implementation efforts in a stepped wedge, can mean that late-wave sites receive different implementation strategies than early-wave sites. Similarly, formative evaluation may inform midstream adaptations to the clinical innovation being implemented. In either case, these changes may again threaten internal validity. Overall, then, stepped wedges represent useful tools for evaluating the impact of health interventions that (as with all designs) are subject to certain weaknesses and limitations.
3. Conclusions and Future Directions
Implementation science is focused on maximizing the extent to which effective healthcare practices are adopted, used, and sustained by clinicians, hospitals, and systems. Answering questions in these domains frequently requires different research methods than those employed in traditional efficacy- or effectiveness-oriented randomized clinical trials (RCTs). Implementation-oriented RCTs typically feature cluster or site-level randomization, and emphasize implementation outcomes (e.g. the number of patients receiving the new treatment as intended) rather than traditional clinical outcomes. Hybrid implementation-effectiveness designs incorporate both types of outcomes; more details on these approaches can be found elsewhere in this special issue (Landes, this issue). Other methodological innovations, such as factorial designs or sequential, multiple-assignment randomized trials (SMARTs), can address questions about multi-component or adaptive interventions, still under the umbrella of experimental designs. These types of trials may be especially important for demystifying the “black box” of implementation—that is, determining what components of an implementation strategy are most strongly associated with implementation success. In contrast, pre-post designs with non-equivalent control groups, interrupted time series (ITS), and stepped wedge designs are all examples of quasiexperimental designs that may serve implementation researchers when experimental designs would be inappropriate. A major theme cutting across each of these designs is that there are relative strengths and weaknesses associated with any study design decision. Determining what design to use ultimately will need to be informed by the primary research question to be answered, while simultaneously balancing the need for internal validity, external validity, feasibility, and ethics.
New innovations in study design are constantly being developed and refined. Several such innovations are covered in other articles within this special issue (e.g. Kim et al., this issue). One future direction relevant to the study designs presented in this article is the potential for adaptive trial designs, which allow information gleaned during the trial to inform the adaptation of components like treatment allocation, sample size, or study recruitment in the later phases of the same trial ( Pallmann et al., 2018 ). These designs are becoming increasingly popular in clinical treatment ( Bhatt and Mehta, 2016 ) but could also hold promise for implementation scientists, especially as interest grows in rapid-cycle testing of implementation strategies or efforts. Adaptive designs could potentially be incorporated into both SMART designs and stepped wedge studies, as well as traditional RCTs to further advance implementation science ( Cheung et al., 2015 ). Ideally, these and other innovations will provide researchers with increasingly robust and useful methodologies for answering timely implementation science questions.
- Many implementation science questions can be addressed by fully experimental designs (e.g. randomized controlled trials [RCTs]).
- Implementation trials differ in important ways, however, from more traditional efficacy- or effectiveness-oriented RCTs.
- Adaptive designs represent a recent innovation to determine optimal implementation strategies within a fully experimental framework.
- Quasi-experimental designs can be used to answer implementation science questions in the absence of randomization.
- The choice of study designs in implementation science requires careful consideration of scientific, pragmatic, and ethical issues.
Acknowledgments
This work was supported by Department of Veterans Affairs grants QUE 15–289 (PI: Bauer) and CIN 13403 and National Institutes of Health grant RO1 MH 099898 (PI: Kilbourne).
Publisher's Disclaimer: This is a PDF file of an unedited manuscript that has been accepted for publication. As a service to our customers we are providing this early version of the manuscript. The manuscript will undergo copyediting, typesetting, and review of the resulting proof before it is published in its final citable form. Please note that during the production process errors may be discovered which could affect the content, and all legal disclaimers that apply to the journal pertain.
- Almirall D, Compton SN, Gunlicks-Stoessel M, Duan N, Murphy SA, 2012. Designing a pilot sequential multiple assignment randomized trial for developing an adaptive treatment strategy . Stat Med 31 ( 17 ), 1887–1902. [ PMC free article ] [ PubMed ] [ Google Scholar ]
- Bauer MS, McBride L, Williford WO, Glick H, Kinosian B, Altshuler L, Beresford T, Kilbourne AM, Sajatovic M, Cooperative Studies Program 430 Study, T., 2006. Collaborative care for bipolar disorder: Part II. Impact on clinical outcome, function, and costs . Psychiatr Serv 57 ( 7 ), 937–945. [ PubMed ] [ Google Scholar ]
- Bauer MS, Miller C, Kim B, Lew R, Weaver K, Coldwell C, Henderson K, Holmes S, Seibert MN, Stolzmann K, Elwy AR, Kirchner J, 2016. Partnering with health system operations leadership to develop a controlled implementation trial . Implement Sci 11 , 22. [ PMC free article ] [ PubMed ] [ Google Scholar ]
- Bauer MS, Miller CJ, Kim B, Lew R, Stolzmann K, Sullivan J, Riendeau R, Pitcock J, Williamson A, Connolly S, Elwy AR, Weaver K, 2019. Effectiveness of Implementing a Collaborative Chronic Care Model for Clinician Teams on Patient Outcomes and Health Status in Mental Health: A Randomized Clinical Trial . JAMA Netw Open 2 ( 3 ), e190230. [ PMC free article ] [ PubMed ] [ Google Scholar ]
- Bernal JL, Cummins S, Gasparrini A, 2017. Interrupted time series regression for the evaluation of public health interventions: a tutorial . Int J Epidemiol 46 ( 1 ), 348–355. [ PMC free article ] [ PubMed ] [ Google Scholar ]
- Betran AP, Bergel E, Griffin S, Melo A, Nguyen MH, Carbonell A, Mondlane S, Merialdi M, Temmerman M, Gulmezoglu AM, 2018. Provision of medical supply kits to improve quality of antenatal care in Mozambique: a stepped-wedge cluster randomised trial . Lancet Glob Health 6 ( 1 ), e57–e65. [ PMC free article ] [ PubMed ] [ Google Scholar ]
- Bhatt DL, Mehta C, 2016. Adaptive Designs for Clinical Trials . N Engl J Med 375 ( 1 ), 65–74. [ PubMed ] [ Google Scholar ]
- Bodenheimer T, Wagner EH, Grumbach K, 2002a. Improving primary care for patients with chronic illness . JAMA 288 ( 14 ), 1775–1779. [ PubMed ] [ Google Scholar ]
- Bodenheimer T, Wagner EH, Grumbach K, 2002b. Improving primary care for patients with chronic illness: the chronic care model, Part 2 . JAMA 288 ( 15 ), 1909–1914. [ PubMed ] [ Google Scholar ]
- Brown CA, Lilford RJ, 2006. The stepped wedge trial design: a systematic review . BMC medical research methodology 6 ( 1 ), 54. [ PMC free article ] [ PubMed ] [ Google Scholar ]
- Byiers BJ, Reichle J, Symons FJ, 2012. Single-subject experimental design for evidence-based practice . Am J Speech Lang Pathol 21 ( 4 ), 397–414. [ PMC free article ] [ PubMed ] [ Google Scholar ]
- Cheung YK, Chakraborty B, Davidson KW, 2015. Sequential multiple assignment randomized trial (SMART) with adaptive randomization for quality improvement in depression treatment program . Biometrics 71 ( 2 ), 450–459. [ PMC free article ] [ PubMed ] [ Google Scholar ]
- Collins LM, Dziak JJ, Kugler KC, Trail JB, 2014a. Factorial experiments: efficient tools for evaluation of intervention components . Am J Prev Med 47 ( 4 ), 498–504. [ PMC free article ] [ PubMed ] [ Google Scholar ]
- Collins LM, Dziak JJ, Li R, 2009. Design of experiments with multiple independent variables: a resource management perspective on complete and reduced factorial designs . Psychol Methods 14 ( 3 ), 202–224. [ PMC free article ] [ PubMed ] [ Google Scholar ]
- Collins LM, Murphy SA, Bierman KL, 2004. A conceptual framework for adaptive preventive interventions . Prev Sci 5 ( 3 ), 185–196. [ PMC free article ] [ PubMed ] [ Google Scholar ]
- Collins LM, Murphy SA, Nair VN, Strecher VJ, 2005. A strategy for optimizing and evaluating behavioral interventions . Ann Behav Med 30 ( 1 ), 65–73. [ PubMed ] [ Google Scholar ]
- Collins LM, Murphy SA, Strecher V, 2007. The multiphase optimization strategy (MOST) and the sequential multiple assignment randomized trial (SMART): new methods for more potent eHealth interventions . Am J Prev Med 32 ( 5 Suppl ), S112–118. [ PMC free article ] [ PubMed ] [ Google Scholar ]
- Collins LM, Nahum-Shani I, Almirall D, 2014b. Optimization of behavioral dynamic treatment regimens based on the sequential, multiple assignment, randomized trial (SMART) . Clin Trials 11 ( 4 ), 426–434. [ PMC free article ] [ PubMed ] [ Google Scholar ]
- Coulton S, Perryman K, Bland M, Cassidy P, Crawford M, Deluca P, Drummond C, Gilvarry E, Godfrey C, Heather N, Kaner E, Myles J, Newbury-Birch D, Oyefeso A, Parrott S, Phillips T, Shenker D, Shepherd J, 2009. Screening and brief interventions for hazardous alcohol use in accident and emergency departments: a randomised controlled trial protocol . BMC Health Serv Res 9 , 114. [ PMC free article ] [ PubMed ] [ Google Scholar ]
- Cousins K, Connor JL, Kypri K, 2014. Effects of the Campus Watch intervention on alcohol consumption and related harm in a university population . Drug Alcohol Depend 143 , 120–126. [ PubMed ] [ Google Scholar ]
- Curran GM, Bauer M, Mittman B, Pyne JM, Stetler C, 2012. Effectiveness-implementation hybrid designs: combining elements of clinical effectiveness and implementation research to enhance public health impact . Med Care 50 ( 3 ), 217–226. [ PMC free article ] [ PubMed ] [ Google Scholar ]
- Davey C, Hargreaves J, Thompson JA, Copas AJ, Beard E, Lewis JJ, Fielding KL, 2015. Analysis and reporting of stepped wedge randomised controlled trials: synthesis and critical appraisal of published studies, 2010 to 2014 . Trials 16 ( 1 ), 358. [ PMC free article ] [ PubMed ] [ Google Scholar ]
- Dimick JB, Ryan AM, 2014. Methods for evaluating changes in health care policy: the difference-in- differences approach . JAMA 312 ( 22 ), 2401–2402. [ PubMed ] [ Google Scholar ]
- Eccles M, Grimshaw J, Campbell M, Ramsay C, 2003. Research designs for studies evaluating the effectiveness of change and improvement strategies . Qual Saf Health Care 12 ( 1 ), 47–52. [ PMC free article ] [ PubMed ] [ Google Scholar ]
- Fisher RA, 1925, July Theory of statistical estimation In Mathematical Proceedings of the Cambridge Philosophical Society (Vol. 22, No. 5, pp. 700–725). Cambridge University Press. [ Google Scholar ]
- Fisher RA, 1935. The design of experiments . Oliver and Boyd, Edinburgh. [ Google Scholar ]
- Fretheim A, Soumerai SB, Zhang F, Oxman AD, Ross-Degnan D, 2013. Interrupted time-series analysis yielded an effect estimate concordant with the cluster-randomized controlled trial result . Journal of Clinical Epidemiology 66 ( 8 ), 883–887. [ PubMed ] [ Google Scholar ]
- Fretheim A, Zhang F, Ross-Degnan D, Oxman AD, Cheyne H, Foy R, Goodacre S, Herrin J, Kerse N, McKinlay RJ, Wright A, Soumerai SB, 2015. A reanalysis of cluster randomized trials showed interrupted time-series studies were valuable in health system evaluation . J Clin Epidemiol 68 ( 3 ), 324–333. [ PubMed ] [ Google Scholar ]
- Gaglio B, Shoup JA, Glasgow RE, 2013. The RE-AIM framework: a systematic review of use over time . Am J Public Health 103 ( 6 ), e38–46. [ PMC free article ] [ PubMed ] [ Google Scholar ]
- Gebski V, Ellingson K, Edwards J, Jernigan J, Kleinbaum D, 2012. Modelling interrupted time series to evaluate prevention and control of infection in healthcare . Epidemiol Infect 140 ( 12 ), 2131–2141. [ PMC free article ] [ PubMed ] [ Google Scholar ]
- Glasgow RE, Vogt TM, Boles SM, 1999. Evaluating the public health impact of health promotion interventions: the RE-AIM framework . Am J Public Health 89 ( 9 ), 1322–1327. [ PMC free article ] [ PubMed ] [ Google Scholar ]
- Hanani H, 1961. The existence and construction of balanced incomplete block designs . The Annals of Mathematical Statistics 32 ( 2 ), 361–386. [ Google Scholar ]
- Hanbury A, Farley K, Thompson C, Wilson PM, Chambers D, Holmes H, 2013. Immediate versus sustained effects: interrupted time series analysis of a tailored intervention . Implement Sci 8 , 130. [ PMC free article ] [ PubMed ] [ Google Scholar ]
- Handley MA, Lyles CR, McCulloch C, Cattamanchi A, 2018. Selecting and Improving Quasi-Experimental Designs in Effectiveness and Implementation Research . Annu Rev Public Health 39 , 5–25. [ PMC free article ] [ PubMed ] [ Google Scholar ]
- Hussey MA, Hughes JP, 2007. Design and analysis of stepped wedge cluster randomized trials . Contemp Clin Trials 28 ( 2 ), 182–191. [ PubMed ] [ Google Scholar ]
- Kilbourne AM, Almirall D, Eisenberg D, Waxmonsky J, Goodrich DE, Fortney JC, Kirchner JE, Solberg LI, Main D, Bauer MS, Kyle J, Murphy SA, Nord KM, Thomas MR, 2014a. Protocol: Adaptive Implementation of Effective Programs Trial (ADEPT): cluster randomized SMART trial comparing a standard versus enhanced implementation strategy to improve outcomes of a mood disorders program . Implement Sci 9 , 132. [ PMC free article ] [ PubMed ] [ Google Scholar ]
- Kilbourne AM, Almirall D, Goodrich DE, Lai Z, Abraham KM, Nord KM, Bowersox NW, 2014b. Enhancing outreach for persons with serious mental illness: 12-month results from a cluster randomized trial of an adaptive implementation strategy . Implement Sci 9 , 163. [ PMC free article ] [ PubMed ] [ Google Scholar ]
- Kilbourne AM, Bramlet M, Barbaresso MM, Nord KM, Goodrich DE, Lai Z, Post EP, Almirall D, Verchinina L, Duffy SA, Bauer MS, 2014c. SMI life goals: description of a randomized trial of a collaborative care model to improve outcomes for persons with serious mental illness . Contemp Clin Trials 39 ( 1 ), 74–85. [ PMC free article ] [ PubMed ] [ Google Scholar ]
- Kilbourne AM, Goodrich DE, Lai Z, Clogston J, Waxmonsky J, Bauer MS, 2012a. Life Goals Collaborative Care for patients with bipolar disorder and cardiovascular disease risk . Psychiatr Serv 63 ( 12 ), 1234–1238. [ PMC free article ] [ PubMed ] [ Google Scholar ]
- Kilbourne AM, Goodrich DE, Nord KM, Van Poppelen C, Kyle J, Bauer MS, Waxmonsky JA, Lai Z, Kim HM, Eisenberg D, Thomas MR, 2015. Long-Term Clinical Outcomes from a Randomized Controlled Trial of Two Implementation Strategies to Promote Collaborative Care Attendance in Community Practices . Adm Policy Ment Health 42 ( 5 ), 642–653. [ PMC free article ] [ PubMed ] [ Google Scholar ]
- Kilbourne AM, Neumann MS, Pincus HA, Bauer MS, Stall R, 2007. Implementing evidence-based interventions in health care: application of the replicating effective programs framework . Implement Sci 2 , 42. [ PMC free article ] [ PubMed ] [ Google Scholar ]
- Kilbourne AM, Neumann MS, Waxmonsky J, Bauer MS, Kim HM, Pincus HA, Thomas M, 2012b. Public-academic partnerships: evidence-based implementation: the role of sustained community-based practice and research partnerships . Psychiatr Serv 63 ( 3 ), 205–207. [ PMC free article ] [ PubMed ] [ Google Scholar ]
- Kilbourne AM, Post EP, Nossek A, Drill L, Cooley S, Bauer MS, 2008. Improving medical and psychiatric outcomes among individuals with bipolar disorder: a randomized controlled trial . Psychiatr Serv 59 ( 7 ), 760–768. [ PubMed ] [ Google Scholar ]
- Kirchner JE, Ritchie MJ, Pitcock JA, Parker LE, Curran GM, Fortney JC, 2014. Outcomes of a partnered facilitation strategy to implement primary care-mental health . J Gen Intern Med 29 Suppl 4 , 904–912. [ PMC free article ] [ PubMed ] [ Google Scholar ]
- Lei H, Nahum-Shani I, Lynch K, Oslin D, Murphy SA, 2012. A “SMART” design for building individualized treatment sequences . Annu Rev Clin Psychol 8 , 21–48. [ PMC free article ] [ PubMed ] [ Google Scholar ]
- Lew RA, Miller CJ, Kim B, Wu H, Stolzmann K, Bauer MS, 2019. A robust method to reduce imbalance for site-level randomized controlled implementation trial designs . Implementation Sci , 14 , 46. [ PMC free article ] [ PubMed ] [ Google Scholar ]
- Morgan CJ, 2018. Reducing bias using propensity score matching . J Nucl Cardiol 25 ( 2 ), 404–406. [ PubMed ] [ Google Scholar ]
- Morton V, Torgerson DJ, 2003. Effect of regression to the mean on decision making in health care . BMJ 326 ( 7398 ), 1083–1084. [ PMC free article ] [ PubMed ] [ Google Scholar ]
- Nahum-Shani I, Qian M, Almirall D, Pelham WE, Gnagy B, Fabiano GA, Waxmonsky JG, Yu J, Murphy SA, 2012. Experimental design and primary data analysis methods for comparing adaptive interventions . Psychol Methods 17 ( 4 ), 457–477. [ PMC free article ] [ PubMed ] [ Google Scholar ]
- NeCamp T, Kilbourne A, Almirall D, 2017. Comparing cluster-level dynamic treatment regimens using sequential, multiple assignment, randomized trials: Regression estimation and sample size considerations . Stat Methods Med Res 26 ( 4 ), 1572–1589. [ PMC free article ] [ PubMed ] [ Google Scholar ]
- Neumann MS, Sogolow ED, 2000. Replicating effective programs: HIV/AIDS prevention technology transfer . AIDS Educ Prev 12 ( 5 Suppl ), 35–48. [ PubMed ] [ Google Scholar ]
- Pallmann P, Bedding AW, Choodari-Oskooei B, Dimairo M, Flight L, Hampson LV, Holmes J, Mander AP, Odondi L.o., Sydes MR, Villar SS, Wason JMS, Weir CJ, Wheeler GM, Yap C, Jaki T, 2018. Adaptive designs in clinical trials: why use them, and how to run and report them . BMC medicine 16 ( 1 ), 29–29. [ PMC free article ] [ PubMed ] [ Google Scholar ]
- Pape UJ, Millett C, Lee JT, Car J, Majeed A, 2013. Disentangling secular trends and policy impacts in health studies: use of interrupted time series analysis . J R Soc Med 106 ( 4 ), 124–129. [ PMC free article ] [ PubMed ] [ Google Scholar ]
- Pellegrini CA, Hoffman SA, Collins LM, Spring B, 2014. Optimization of remotely delivered intensive lifestyle treatment for obesity using the Multiphase Optimization Strategy: Opt-IN study protocol . Contemp Clin Trials 38 ( 2 ), 251–259. [ PMC free article ] [ PubMed ] [ Google Scholar ]
- Penfold RB, Zhang F, 2013. Use of Interrupted Time Series Analysis in Evaluating Health Care Quality Improvements . Academic Pediatrics 13 ( 6, Supplement ), S38–S44. [ PubMed ] [ Google Scholar ]
- Pridemore WA, Snowden AJ, 2009. Reduction in suicide mortality following a new national alcohol policy in Slovenia: an interrupted time-series analysis . Am J Public Health 99 ( 5 ), 915–920. [ PMC free article ] [ PubMed ] [ Google Scholar ]
- Proctor E, Silmere H, Raghavan R, Hovmand P, Aarons G, Bunger A, Griffey R, Hensley M, 2011. Outcomes for implementation research: conceptual distinctions, measurement challenges, and research agenda . Adm Policy Ment Health 38 ( 2 ), 65–76. [ PMC free article ] [ PubMed ] [ Google Scholar ]
- Robson D, Spaducci G, McNeill A, Stewart D, Craig TJK, Yates M, Szatkowski L, 2017. Effect of implementation of a smoke-free policy on physical violence in a psychiatric inpatient setting: an interrupted time series analysis . Lancet Psychiatry 4 ( 7 ), 540–546. [ PubMed ] [ Google Scholar ]
- Schildcrout JS, Schisterman EF, Mercaldo ND, Rathouz PJ, Heagerty PJ, 2018. Extending the Case-Control Design to Longitudinal Data: Stratified Sampling Based on Repeated Binary Outcomes . Epidemiology 29 ( 1 ), 67–75. [ PMC free article ] [ PubMed ] [ Google Scholar ]
- Shadish WR, Cook Thomas D., Campbell Donald T, 2002. Experimental and quasi-experimental designs for generalized causal inference . Houghton Miffflin Company, Boston, MA. [ Google Scholar ]
- Simon GE, Ludman EJ, Bauer MS, Unutzer J, Operskalski B, 2006. Long-term effectiveness and cost of a systematic care program for bipolar disorder . Arch Gen Psychiatry 63 ( 5 ), 500–508. [ PubMed ] [ Google Scholar ]
- Stetler CB, Legro MW, Rycroft-Malone J, Bowman C, Curran G, Guihan M, Hagedorn H, Pineros S, Wallace CM, 2006. Role of “external facilitation” in implementation of research findings: a qualitative evaluation of facilitation experiences in the Veterans Health Administration . Implement Sci 1 , 23. [ PMC free article ] [ PubMed ] [ Google Scholar ]
- Taljaard M, McKenzie JE, Ramsay CR, Grimshaw JM, 2014. The use of segmented regression in analysing interrupted time series studies: an example in pre-hospital ambulance care . Implement Sci 9 , 77. [ PMC free article ] [ PubMed ] [ Google Scholar ]
- Wagner AK, Soumerai SB, Zhang F, Ross-Degnan D, 2002. Segmented regression analysis of interrupted time series studies in medication use research . J Clin Pharm Ther 27 ( 4 ), 299–309. [ PubMed ] [ Google Scholar ]
- Wagner EH, Austin BT, Von Korff M, 1996. Organizing care for patients with chronic illness . Milbank Q 74 ( 4 ), 511–544. [ PubMed ] [ Google Scholar ]
- Wyrick DL, Rulison KL, Fearnow-Kenney M, Milroy JJ, Collins LM, 2014. Moving beyond the treatment package approach to developing behavioral interventions: addressing questions that arose during an application of the Multiphase Optimization Strategy (MOST) . Transl Behav Med 4 ( 3 ), 252–259. [ PMC free article ] [ PubMed ] [ Google Scholar ]
- Search Menu
Sign in through your institution
- Browse content in Arts and Humanities
- Browse content in Archaeology
- Anglo-Saxon and Medieval Archaeology
- Archaeological Methodology and Techniques
- Archaeology by Region
- Archaeology of Religion
- Archaeology of Trade and Exchange
- Biblical Archaeology
- Contemporary and Public Archaeology
- Environmental Archaeology
- Historical Archaeology
- History and Theory of Archaeology
- Industrial Archaeology
- Landscape Archaeology
- Mortuary Archaeology
- Prehistoric Archaeology
- Underwater Archaeology
- Zooarchaeology
- Browse content in Architecture
- Architectural Structure and Design
- History of Architecture
- Residential and Domestic Buildings
- Theory of Architecture
- Browse content in Art
- Art Subjects and Themes
- History of Art
- Industrial and Commercial Art
- Theory of Art
- Biographical Studies
- Byzantine Studies
- Browse content in Classical Studies
- Classical History
- Classical Philosophy
- Classical Mythology
- Classical Numismatics
- Classical Literature
- Classical Reception
- Classical Art and Architecture
- Classical Oratory and Rhetoric
- Greek and Roman Epigraphy
- Greek and Roman Law
- Greek and Roman Papyrology
- Greek and Roman Archaeology
- Late Antiquity
- Religion in the Ancient World
- Social History
- Digital Humanities
- Browse content in History
- Colonialism and Imperialism
- Diplomatic History
- Environmental History
- Genealogy, Heraldry, Names, and Honours
- Genocide and Ethnic Cleansing
- Historical Geography
- History by Period
- History of Emotions
- History of Agriculture
- History of Education
- History of Gender and Sexuality
- Industrial History
- Intellectual History
- International History
- Labour History
- Legal and Constitutional History
- Local and Family History
- Maritime History
- Military History
- National Liberation and Post-Colonialism
- Oral History
- Political History
- Public History
- Regional and National History
- Revolutions and Rebellions
- Slavery and Abolition of Slavery
- Social and Cultural History
- Theory, Methods, and Historiography
- Urban History
- World History
- Browse content in Language Teaching and Learning
- Language Learning (Specific Skills)
- Language Teaching Theory and Methods
- Browse content in Linguistics
- Applied Linguistics
- Cognitive Linguistics
- Computational Linguistics
- Forensic Linguistics
- Grammar, Syntax and Morphology
- Historical and Diachronic Linguistics
- History of English
- Language Acquisition
- Language Evolution
- Language Reference
- Language Variation
- Language Families
- Lexicography
- Linguistic Anthropology
- Linguistic Theories
- Linguistic Typology
- Phonetics and Phonology
- Psycholinguistics
- Sociolinguistics
- Translation and Interpretation
- Writing Systems
- Browse content in Literature
- Bibliography
- Children's Literature Studies
- Literary Studies (Asian)
- Literary Studies (European)
- Literary Studies (Eco-criticism)
- Literary Studies (Romanticism)
- Literary Studies (American)
- Literary Studies (Modernism)
- Literary Studies - World
- Literary Studies (1500 to 1800)
- Literary Studies (19th Century)
- Literary Studies (20th Century onwards)
- Literary Studies (African American Literature)
- Literary Studies (British and Irish)
- Literary Studies (Early and Medieval)
- Literary Studies (Fiction, Novelists, and Prose Writers)
- Literary Studies (Gender Studies)
- Literary Studies (Graphic Novels)
- Literary Studies (History of the Book)
- Literary Studies (Plays and Playwrights)
- Literary Studies (Poetry and Poets)
- Literary Studies (Postcolonial Literature)
- Literary Studies (Queer Studies)
- Literary Studies (Science Fiction)
- Literary Studies (Travel Literature)
- Literary Studies (War Literature)
- Literary Studies (Women's Writing)
- Literary Theory and Cultural Studies
- Mythology and Folklore
- Shakespeare Studies and Criticism
- Browse content in Media Studies
- Browse content in Music
- Applied Music
- Dance and Music
- Ethics in Music
- Ethnomusicology
- Gender and Sexuality in Music
- Medicine and Music
- Music Cultures
- Music and Religion
- Music and Media
- Music and Culture
- Music Education and Pedagogy
- Music Theory and Analysis
- Musical Scores, Lyrics, and Libretti
- Musical Structures, Styles, and Techniques
- Musicology and Music History
- Performance Practice and Studies
- Race and Ethnicity in Music
- Sound Studies
- Browse content in Performing Arts
- Browse content in Philosophy
- Aesthetics and Philosophy of Art
- Epistemology
- Feminist Philosophy
- History of Western Philosophy
- Metaphysics
- Moral Philosophy
- Non-Western Philosophy
- Philosophy of Science
- Philosophy of Language
- Philosophy of Mind
- Philosophy of Perception
- Philosophy of Action
- Philosophy of Law
- Philosophy of Religion
- Philosophy of Mathematics and Logic
- Practical Ethics
- Social and Political Philosophy
- Browse content in Religion
- Biblical Studies
- Christianity
- East Asian Religions
- History of Religion
- Judaism and Jewish Studies
- Qumran Studies
- Religion and Education
- Religion and Health
- Religion and Politics
- Religion and Science
- Religion and Law
- Religion and Art, Literature, and Music
- Religious Studies
- Browse content in Society and Culture
- Cookery, Food, and Drink
- Cultural Studies
- Customs and Traditions
- Ethical Issues and Debates
- Hobbies, Games, Arts and Crafts
- Natural world, Country Life, and Pets
- Popular Beliefs and Controversial Knowledge
- Sports and Outdoor Recreation
- Technology and Society
- Travel and Holiday
- Visual Culture
- Browse content in Law
- Arbitration
- Browse content in Company and Commercial Law
- Commercial Law
- Company Law
- Browse content in Comparative Law
- Systems of Law
- Competition Law
- Browse content in Constitutional and Administrative Law
- Government Powers
- Judicial Review
- Local Government Law
- Military and Defence Law
- Parliamentary and Legislative Practice
- Construction Law
- Contract Law
- Browse content in Criminal Law
- Criminal Procedure
- Criminal Evidence Law
- Sentencing and Punishment
- Employment and Labour Law
- Environment and Energy Law
- Browse content in Financial Law
- Banking Law
- Insolvency Law
- History of Law
- Human Rights and Immigration
- Intellectual Property Law
- Browse content in International Law
- Private International Law and Conflict of Laws
- Public International Law
- IT and Communications Law
- Jurisprudence and Philosophy of Law
- Law and Politics
- Law and Society
- Browse content in Legal System and Practice
- Courts and Procedure
- Legal Skills and Practice
- Legal System - Costs and Funding
- Primary Sources of Law
- Regulation of Legal Profession
- Medical and Healthcare Law
- Browse content in Policing
- Criminal Investigation and Detection
- Police and Security Services
- Police Procedure and Law
- Police Regional Planning
- Browse content in Property Law
- Personal Property Law
- Restitution
- Study and Revision
- Terrorism and National Security Law
- Browse content in Trusts Law
- Wills and Probate or Succession
- Browse content in Medicine and Health
- Browse content in Allied Health Professions
- Arts Therapies
- Clinical Science
- Dietetics and Nutrition
- Occupational Therapy
- Operating Department Practice
- Physiotherapy
- Radiography
- Speech and Language Therapy
- Browse content in Anaesthetics
- General Anaesthesia
- Browse content in Clinical Medicine
- Acute Medicine
- Cardiovascular Medicine
- Clinical Genetics
- Clinical Pharmacology and Therapeutics
- Dermatology
- Endocrinology and Diabetes
- Gastroenterology
- Genito-urinary Medicine
- Geriatric Medicine
- Infectious Diseases
- Medical Toxicology
- Medical Oncology
- Pain Medicine
- Palliative Medicine
- Rehabilitation Medicine
- Respiratory Medicine and Pulmonology
- Rheumatology
- Sleep Medicine
- Sports and Exercise Medicine
- Clinical Neuroscience
- Community Medical Services
- Critical Care
- Emergency Medicine
- Forensic Medicine
- Haematology
- History of Medicine
- Browse content in Medical Dentistry
- Oral and Maxillofacial Surgery
- Paediatric Dentistry
- Restorative Dentistry and Orthodontics
- Surgical Dentistry
- Browse content in Medical Skills
- Clinical Skills
- Communication Skills
- Nursing Skills
- Surgical Skills
- Medical Ethics
- Medical Statistics and Methodology
- Browse content in Neurology
- Clinical Neurophysiology
- Neuropathology
- Nursing Studies
- Browse content in Obstetrics and Gynaecology
- Gynaecology
- Occupational Medicine
- Ophthalmology
- Otolaryngology (ENT)
- Browse content in Paediatrics
- Neonatology
- Browse content in Pathology
- Chemical Pathology
- Clinical Cytogenetics and Molecular Genetics
- Histopathology
- Medical Microbiology and Virology
- Patient Education and Information
- Browse content in Pharmacology
- Psychopharmacology
- Browse content in Popular Health
- Caring for Others
- Complementary and Alternative Medicine
- Self-help and Personal Development
- Browse content in Preclinical Medicine
- Cell Biology
- Molecular Biology and Genetics
- Reproduction, Growth and Development
- Primary Care
- Professional Development in Medicine
- Browse content in Psychiatry
- Addiction Medicine
- Child and Adolescent Psychiatry
- Forensic Psychiatry
- Learning Disabilities
- Old Age Psychiatry
- Psychotherapy
- Browse content in Public Health and Epidemiology
- Epidemiology
- Public Health
- Browse content in Radiology
- Clinical Radiology
- Interventional Radiology
- Nuclear Medicine
- Radiation Oncology
- Reproductive Medicine
- Browse content in Surgery
- Cardiothoracic Surgery
- Gastro-intestinal and Colorectal Surgery
- General Surgery
- Neurosurgery
- Paediatric Surgery
- Peri-operative Care
- Plastic and Reconstructive Surgery
- Surgical Oncology
- Transplant Surgery
- Trauma and Orthopaedic Surgery
- Vascular Surgery
- Browse content in Science and Mathematics
- Browse content in Biological Sciences
- Aquatic Biology
- Biochemistry
- Bioinformatics and Computational Biology
- Developmental Biology
- Ecology and Conservation
- Evolutionary Biology
- Genetics and Genomics
- Microbiology
- Molecular and Cell Biology
- Natural History
- Plant Sciences and Forestry
- Research Methods in Life Sciences
- Structural Biology
- Systems Biology
- Zoology and Animal Sciences
- Browse content in Chemistry
- Analytical Chemistry
- Computational Chemistry
- Crystallography
- Environmental Chemistry
- Industrial Chemistry
- Inorganic Chemistry
- Materials Chemistry
- Medicinal Chemistry
- Mineralogy and Gems
- Organic Chemistry
- Physical Chemistry
- Polymer Chemistry
- Study and Communication Skills in Chemistry
- Theoretical Chemistry
- Browse content in Computer Science
- Artificial Intelligence
- Computer Architecture and Logic Design
- Game Studies
- Human-Computer Interaction
- Mathematical Theory of Computation
- Programming Languages
- Software Engineering
- Systems Analysis and Design
- Virtual Reality
- Browse content in Computing
- Business Applications
- Computer Security
- Computer Games
- Computer Networking and Communications
- Digital Lifestyle
- Graphical and Digital Media Applications
- Operating Systems
- Browse content in Earth Sciences and Geography
- Atmospheric Sciences
- Environmental Geography
- Geology and the Lithosphere
- Maps and Map-making
- Meteorology and Climatology
- Oceanography and Hydrology
- Palaeontology
- Physical Geography and Topography
- Regional Geography
- Soil Science
- Urban Geography
- Browse content in Engineering and Technology
- Agriculture and Farming
- Biological Engineering
- Civil Engineering, Surveying, and Building
- Electronics and Communications Engineering
- Energy Technology
- Engineering (General)
- Environmental Science, Engineering, and Technology
- History of Engineering and Technology
- Mechanical Engineering and Materials
- Technology of Industrial Chemistry
- Transport Technology and Trades
- Browse content in Environmental Science
- Applied Ecology (Environmental Science)
- Conservation of the Environment (Environmental Science)
- Environmental Sustainability
- Environmentalist Thought and Ideology (Environmental Science)
- Management of Land and Natural Resources (Environmental Science)
- Natural Disasters (Environmental Science)
- Nuclear Issues (Environmental Science)
- Pollution and Threats to the Environment (Environmental Science)
- Social Impact of Environmental Issues (Environmental Science)
- History of Science and Technology
- Browse content in Materials Science
- Ceramics and Glasses
- Composite Materials
- Metals, Alloying, and Corrosion
- Nanotechnology
- Browse content in Mathematics
- Applied Mathematics
- Biomathematics and Statistics
- History of Mathematics
- Mathematical Education
- Mathematical Finance
- Mathematical Analysis
- Numerical and Computational Mathematics
- Probability and Statistics
- Pure Mathematics
- Browse content in Neuroscience
- Cognition and Behavioural Neuroscience
- Development of the Nervous System
- Disorders of the Nervous System
- History of Neuroscience
- Invertebrate Neurobiology
- Molecular and Cellular Systems
- Neuroendocrinology and Autonomic Nervous System
- Neuroscientific Techniques
- Sensory and Motor Systems
- Browse content in Physics
- Astronomy and Astrophysics
- Atomic, Molecular, and Optical Physics
- Biological and Medical Physics
- Classical Mechanics
- Computational Physics
- Condensed Matter Physics
- Electromagnetism, Optics, and Acoustics
- History of Physics
- Mathematical and Statistical Physics
- Measurement Science
- Nuclear Physics
- Particles and Fields
- Plasma Physics
- Quantum Physics
- Relativity and Gravitation
- Semiconductor and Mesoscopic Physics
- Browse content in Psychology
- Affective Sciences
- Clinical Psychology
- Cognitive Psychology
- Cognitive Neuroscience
- Criminal and Forensic Psychology
- Developmental Psychology
- Educational Psychology
- Evolutionary Psychology
- Health Psychology
- History and Systems in Psychology
- Music Psychology
- Neuropsychology
- Organizational Psychology
- Psychological Assessment and Testing
- Psychology of Human-Technology Interaction
- Psychology Professional Development and Training
- Research Methods in Psychology
- Social Psychology
- Browse content in Social Sciences
- Browse content in Anthropology
- Anthropology of Religion
- Human Evolution
- Medical Anthropology
- Physical Anthropology
- Regional Anthropology
- Social and Cultural Anthropology
- Theory and Practice of Anthropology
- Browse content in Business and Management
- Business Strategy
- Business Ethics
- Business History
- Business and Government
- Business and Technology
- Business and the Environment
- Comparative Management
- Corporate Governance
- Corporate Social Responsibility
- Entrepreneurship
- Health Management
- Human Resource Management
- Industrial and Employment Relations
- Industry Studies
- Information and Communication Technologies
- International Business
- Knowledge Management
- Management and Management Techniques
- Operations Management
- Organizational Theory and Behaviour
- Pensions and Pension Management
- Public and Nonprofit Management
- Social Issues in Business and Management
- Strategic Management
- Supply Chain Management
- Browse content in Criminology and Criminal Justice
- Criminal Justice
- Criminology
- Forms of Crime
- International and Comparative Criminology
- Youth Violence and Juvenile Justice
- Development Studies
- Browse content in Economics
- Agricultural, Environmental, and Natural Resource Economics
- Asian Economics
- Behavioural Finance
- Behavioural Economics and Neuroeconomics
- Econometrics and Mathematical Economics
- Economic Systems
- Economic History
- Economic Methodology
- Economic Development and Growth
- Financial Markets
- Financial Institutions and Services
- General Economics and Teaching
- Health, Education, and Welfare
- History of Economic Thought
- International Economics
- Labour and Demographic Economics
- Law and Economics
- Macroeconomics and Monetary Economics
- Microeconomics
- Public Economics
- Urban, Rural, and Regional Economics
- Welfare Economics
- Browse content in Education
- Adult Education and Continuous Learning
- Care and Counselling of Students
- Early Childhood and Elementary Education
- Educational Equipment and Technology
- Educational Strategies and Policy
- Higher and Further Education
- Organization and Management of Education
- Philosophy and Theory of Education
- Schools Studies
- Secondary Education
- Teaching of a Specific Subject
- Teaching of Specific Groups and Special Educational Needs
- Teaching Skills and Techniques
- Browse content in Environment
- Applied Ecology (Social Science)
- Climate Change
- Conservation of the Environment (Social Science)
- Environmentalist Thought and Ideology (Social Science)
- Management of Land and Natural Resources (Social Science)
- Natural Disasters (Environment)
- Pollution and Threats to the Environment (Social Science)
- Social Impact of Environmental Issues (Social Science)
- Sustainability
- Browse content in Human Geography
- Cultural Geography
- Economic Geography
- Political Geography
- Browse content in Interdisciplinary Studies
- Communication Studies
- Museums, Libraries, and Information Sciences
- Browse content in Politics
- African Politics
- Asian Politics
- Chinese Politics
- Comparative Politics
- Conflict Politics
- Elections and Electoral Studies
- Environmental Politics
- Ethnic Politics
- European Union
- Foreign Policy
- Gender and Politics
- Human Rights and Politics
- Indian Politics
- International Relations
- International Organization (Politics)
- Irish Politics
- Latin American Politics
- Middle Eastern Politics
- Political Methodology
- Political Communication
- Political Philosophy
- Political Sociology
- Political Behaviour
- Political Economy
- Political Institutions
- Political Theory
- Politics and Law
- Politics of Development
- Public Administration
- Public Policy
- Qualitative Political Methodology
- Quantitative Political Methodology
- Regional Political Studies
- Russian Politics
- Security Studies
- State and Local Government
- UK Politics
- US Politics
- Browse content in Regional and Area Studies
- African Studies
- Asian Studies
- East Asian Studies
- Japanese Studies
- Latin American Studies
- Middle Eastern Studies
- Native American Studies
- Scottish Studies
- Browse content in Research and Information
- Research Methods
- Browse content in Social Work
- Addictions and Substance Misuse
- Adoption and Fostering
- Care of the Elderly
- Child and Adolescent Social Work
- Couple and Family Social Work
- Direct Practice and Clinical Social Work
- Emergency Services
- Human Behaviour and the Social Environment
- International and Global Issues in Social Work
- Mental and Behavioural Health
- Social Justice and Human Rights
- Social Policy and Advocacy
- Social Work and Crime and Justice
- Social Work Macro Practice
- Social Work Practice Settings
- Social Work Research and Evidence-based Practice
- Welfare and Benefit Systems
- Browse content in Sociology
- Childhood Studies
- Community Development
- Comparative and Historical Sociology
- Disability Studies
- Economic Sociology
- Gender and Sexuality
- Gerontology and Ageing
- Health, Illness, and Medicine
- Marriage and the Family
- Migration Studies
- Occupations, Professions, and Work
- Organizations
- Population and Demography
- Race and Ethnicity
- Social Theory
- Social Movements and Social Change
- Social Research and Statistics
- Social Stratification, Inequality, and Mobility
- Sociology of Religion
- Sociology of Education
- Sport and Leisure
- Urban and Rural Studies
- Browse content in Warfare and Defence
- Defence Strategy, Planning, and Research
- Land Forces and Warfare
- Military Administration
- Military Life and Institutions
- Naval Forces and Warfare
- Other Warfare and Defence Issues
- Peace Studies and Conflict Resolution
- Weapons and Equipment

Quasi-Experimental Research Designs

- Cite Icon Cite
- Permissions Icon Permissions
Quasi-experimental research designs are the most widely used research approach employed to evaluate the outcomes of social work programs and policies. This new volume describes the logic, design, and conduct of the range of such designs, encompassing pre-experiments, quasi-experiments making use of a control or comparison group, and time-series designs. An introductory chapter describes the valuable role these types of studies have played in social work, going back to the 1930s, and continuing to the present. Subsequent chapters describe the major features of individual quasi-experimental designs, the types of questions they are capable of answering, and their strengths and limitations. Each discussion of these designs presented in the abstract is subsequently illustrated with descriptions of real examples of their use as published in the social work literature and related fields. By linking the discussion of quasi-experimental designs in the abstract to actual applications to evaluate the outcomes of social services, the usefulness and vitality of these research methods comes alive to the reader. While this volume could be used as a research textbook, it will also have great value to practitioners seeking a greater conceptual understanding of the quasi-experimental studies they frequently read about in the social work literature. Human service professionals planning to undertake a program evaluation of their own agency's services will find this book of immense help in understanding the steps and actions needed to adopt a quasi-experimental strategy. It is usually the case that ethical and pragmatic considerations preclude the use of randomly assigning social work clients to experimental and comparative treatment conditions, and in such instances, the practicality of employing a quasi-experimental method becomes an excellent alternative.
Personal account
- Sign in with email/username & password
- Get email alerts
- Save searches
- Purchase content
- Activate your purchase/trial code
- Add your ORCID iD
Institutional access
Sign in with a library card.
- Sign in with username/password
- Recommend to your librarian
- Institutional account management
- Get help with access
Access to content on Oxford Academic is often provided through institutional subscriptions and purchases. If you are a member of an institution with an active account, you may be able to access content in one of the following ways:
IP based access
Typically, access is provided across an institutional network to a range of IP addresses. This authentication occurs automatically, and it is not possible to sign out of an IP authenticated account.
Choose this option to get remote access when outside your institution. Shibboleth/Open Athens technology is used to provide single sign-on between your institution’s website and Oxford Academic.
- Click Sign in through your institution.
- Select your institution from the list provided, which will take you to your institution's website to sign in.
- When on the institution site, please use the credentials provided by your institution. Do not use an Oxford Academic personal account.
- Following successful sign in, you will be returned to Oxford Academic.
If your institution is not listed or you cannot sign in to your institution’s website, please contact your librarian or administrator.
Enter your library card number to sign in. If you cannot sign in, please contact your librarian.
Society Members
Society member access to a journal is achieved in one of the following ways:
Sign in through society site
Many societies offer single sign-on between the society website and Oxford Academic. If you see ‘Sign in through society site’ in the sign in pane within a journal:
- Click Sign in through society site.
- When on the society site, please use the credentials provided by that society. Do not use an Oxford Academic personal account.
If you do not have a society account or have forgotten your username or password, please contact your society.
Sign in using a personal account
Some societies use Oxford Academic personal accounts to provide access to their members. See below.
A personal account can be used to get email alerts, save searches, purchase content, and activate subscriptions.
Some societies use Oxford Academic personal accounts to provide access to their members.
Viewing your signed in accounts
Click the account icon in the top right to:
- View your signed in personal account and access account management features.
- View the institutional accounts that are providing access.
Signed in but can't access content
Oxford Academic is home to a wide variety of products. The institutional subscription may not cover the content that you are trying to access. If you believe you should have access to that content, please contact your librarian.
For librarians and administrators, your personal account also provides access to institutional account management. Here you will find options to view and activate subscriptions, manage institutional settings and access options, access usage statistics, and more.
Our books are available by subscription or purchase to libraries and institutions.
Month: | Total Views: |
---|---|
October 2022 | 1 |
October 2022 | 4 |
October 2022 | 3 |
October 2022 | 15 |
October 2022 | 2 |
October 2022 | 1 |
October 2022 | 1 |
October 2022 | 15 |
October 2022 | 9 |
October 2022 | 2 |
November 2022 | 28 |
November 2022 | 5 |
November 2022 | 15 |
November 2022 | 9 |
November 2022 | 1 |
November 2022 | 21 |
December 2022 | 1 |
December 2022 | 16 |
December 2022 | 5 |
December 2022 | 7 |
January 2023 | 1 |
January 2023 | 12 |
January 2023 | 15 |
January 2023 | 7 |
February 2023 | 1 |
February 2023 | 7 |
February 2023 | 1 |
February 2023 | 6 |
February 2023 | 18 |
February 2023 | 9 |
February 2023 | 9 |
March 2023 | 2 |
March 2023 | 11 |
March 2023 | 19 |
March 2023 | 1 |
March 2023 | 15 |
March 2023 | 7 |
March 2023 | 13 |
April 2023 | 7 |
April 2023 | 15 |
April 2023 | 4 |
April 2023 | 7 |
April 2023 | 17 |
April 2023 | 39 |
April 2023 | 34 |
April 2023 | 5 |
April 2023 | 14 |
April 2023 | 5 |
May 2023 | 7 |
May 2023 | 13 |
May 2023 | 31 |
May 2023 | 18 |
May 2023 | 15 |
June 2023 | 2 |
June 2023 | 7 |
June 2023 | 13 |
June 2023 | 19 |
June 2023 | 11 |
July 2023 | 1 |
July 2023 | 8 |
July 2023 | 2 |
July 2023 | 2 |
July 2023 | 14 |
July 2023 | 17 |
July 2023 | 1 |
July 2023 | 2 |
July 2023 | 24 |
July 2023 | 9 |
August 2023 | 3 |
August 2023 | 14 |
August 2023 | 9 |
August 2023 | 5 |
August 2023 | 14 |
August 2023 | 19 |
August 2023 | 22 |
August 2023 | 3 |
August 2023 | 7 |
August 2023 | 23 |
September 2023 | 3 |
September 2023 | 15 |
September 2023 | 13 |
September 2023 | 30 |
September 2023 | 15 |
October 2023 | 12 |
October 2023 | 16 |
October 2023 | 12 |
October 2023 | 39 |
October 2023 | 9 |
November 2023 | 10 |
November 2023 | 1 |
November 2023 | 16 |
November 2023 | 31 |
November 2023 | 15 |
November 2023 | 26 |
November 2023 | 1 |
December 2023 | 19 |
December 2023 | 2 |
December 2023 | 2 |
December 2023 | 2 |
December 2023 | 18 |
December 2023 | 3 |
December 2023 | 12 |
December 2023 | 25 |
December 2023 | 2 |
December 2023 | 18 |
January 2024 | 1 |
January 2024 | 7 |
January 2024 | 25 |
January 2024 | 8 |
January 2024 | 7 |
February 2024 | 7 |
February 2024 | 2 |
February 2024 | 25 |
February 2024 | 27 |
February 2024 | 34 |
February 2024 | 20 |
February 2024 | 2 |
March 2024 | 1 |
March 2024 | 12 |
March 2024 | 2 |
March 2024 | 24 |
March 2024 | 2 |
March 2024 | 2 |
March 2024 | 1 |
March 2024 | 25 |
March 2024 | 11 |
March 2024 | 18 |
April 2024 | 2 |
April 2024 | 11 |
April 2024 | 2 |
April 2024 | 17 |
April 2024 | 1 |
April 2024 | 15 |
April 2024 | 31 |
April 2024 | 17 |
May 2024 | 7 |
May 2024 | 1 |
May 2024 | 12 |
May 2024 | 19 |
May 2024 | 1 |
May 2024 | 21 |
May 2024 | 12 |
June 2024 | 3 |
June 2024 | 24 |
June 2024 | 1 |
June 2024 | 15 |
June 2024 | 49 |
June 2024 | 1 |
June 2024 | 15 |
June 2024 | 14 |
July 2024 | 12 |
July 2024 | 1 |
July 2024 | 2 |
July 2024 | 17 |
July 2024 | 1 |
July 2024 | 20 |
July 2024 | 19 |
July 2024 | 21 |
July 2024 | 2 |
August 2024 | 3 |
August 2024 | 6 |
August 2024 | 10 |
August 2024 | 12 |
August 2024 | 7 |
September 2024 | 3 |
September 2024 | 1 |
September 2024 | 11 |
September 2024 | 3 |
September 2024 | 6 |
- About Oxford Academic
- Publish journals with us
- University press partners
- What we publish
- New features
- Open access
- Rights and permissions
- Accessibility
- Advertising
- Media enquiries
- Oxford University Press
- Oxford Languages
- University of Oxford
Oxford University Press is a department of the University of Oxford. It furthers the University's objective of excellence in research, scholarship, and education by publishing worldwide
- Copyright © 2024 Oxford University Press
- Cookie settings
- Cookie policy
- Privacy policy
- Legal notice
This Feature Is Available To Subscribers Only
Sign In or Create an Account
This PDF is available to Subscribers Only
For full access to this pdf, sign in to an existing account, or purchase an annual subscription.
Click through the PLOS taxonomy to find articles in your field.
For more information about PLOS Subject Areas, click here .
Loading metrics
Open Access
Peer-reviewed
Research Article
Police violence reduces trust in the police among Black residents
Contributed equally to this work with: Jonathan Ben-Menachem, Gerard Torrats-Espinosa
Roles Conceptualization, Methodology, Writing – original draft, Writing – review & editing
* E-mail: [email protected] (JBM); [email protected] (GTE)
Affiliation Department of Sociology, Columbia University, New York, New York, United States of America

Roles Conceptualization, Data curation, Formal analysis, Methodology, Project administration, Supervision, Visualization, Writing – original draft, Writing – review & editing
- Jonathan Ben-Menachem,
- Gerard Torrats-Espinosa
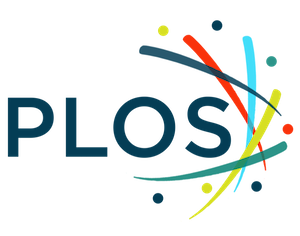
- Published: September 11, 2024
- https://doi.org/10.1371/journal.pone.0308487
- Reader Comments
Recent high-profile incidents involving the shooting or killing of unarmed Black men have intensified the debate about how police violence affects trust in the criminal justice system, particularly among communities of color. In this article, we propose a quasi-experimental design that leverages the timing of the shooting of Jacob Blake by the Kenosha Police Department relative to when a large survey was fielded in the city of Chicago. We demonstrate that individuals interviewed 4 weeks before and 4 weeks after the shooting are comparable across a large set of observed characteristics, thus approximating an experimental setting. We find that Blake’s shooting caused substantial reductions in Black respondents’ trust in the police, concentrated among younger residents and criminalized residents. These results suggest that police violence against racial minorities may lead to lower civic engagement and cooperation with law enforcement in those communities, exacerbating issues of public safety and community well-being. The pronounced distrust among younger Black residents suggests a generational rift that could risk further entrenching systemic biases and inequalities within the criminal justice system. Additionally, the higher levels of distrust among criminalized respondents could have implications for research detailing this population’s decreased willingness to engage with public institutions more broadly.
Citation: Ben-Menachem J, Torrats-Espinosa G (2024) Police violence reduces trust in the police among Black residents. PLoS ONE 19(9): e0308487. https://doi.org/10.1371/journal.pone.0308487
Editor: W. David Allen, University of Alabama in Huntsville, UNITED STATES OF AMERICA
Received: April 4, 2024; Accepted: July 22, 2024; Published: September 11, 2024
Copyright: © 2024 Ben-Menachem, Torrats-Espinosa. This is an open access article distributed under the terms of the Creative Commons Attribution License , which permits unrestricted use, distribution, and reproduction in any medium, provided the original author and source are credited.
Data Availability: The data and code used in our analyses is available here: https://doi.org/10.7910/DVN/CO404V .
Funding: The author(s) received no specific funding for this work.
Competing interests: The authors have declared that no competing interests exist.
Introduction
In recent years, the escalation of high-profile incidents of police violence, particularly against members of the Black community, has brought police violence to the forefront of domestic policy debates. From 2015 to 2020, there were 4,740 civilians fatally shot by police in the U.S., with a disproportionately high 26.7% being Black, while Hispanic fatalities stood at 18.8%, and white fatalities accounted for 51% of these cases [ 1 ]. This over-representation of minorities in fatal police encounters signifies a distressing trend that has made police violence a leading cause of death among young minority men [ 2 ].
The publicized killings of individuals such as Eric Garner, Philando Castile, George Floyd, and Jacob Blake have not only captured national attention but have also sparked a reevaluation of the role of law enforcement in society. Such incidents, often documented on video and spread across social media and news outlets, provide a raw glimpse into the interactions between police officers and Black individuals, potentially influencing public perceptions and trust in the police as an institution. These highly publicized events of police violence can change police-community dynamics. Individuals who have not been victims of police violence themselves may lose trust in law enforcement if they perceive the police as a discriminatory institution that systematically targets their racial group. This cycle of mistrust can result in a downward spiral where a heightened sense of alienation fuels more violence, particularly in the communities where police violence is high. Prior research finds that individuals reporting low trust in police are more likely to acquire a firearm for self-protection, either from nearby residents or from the police themselves [ 3 , 4 ].
The relationship between law enforcement and the communities they serve has been a focal point of sociological research for decades [ 5 – 7 ]. Central to this discourse is the issue of trust in the criminal justice system, particularly among communities of color. Legal cynicism, or the belief in the incompetence and illegitimacy of the criminal justice system, is prevalent in many minority communities [ 8 ]. This cynicism, exacerbated by incidents of police violence, can lead to a reluctance to cooperate with law enforcement agencies.
Prior research has examined the extent to which police violence and misconduct perpetuate legal cynicism using interviews and correlational survey designs, finding that police contact can alienate residents who are subjected to it [ 9 – 11 ]. A growing number of studies have relied on 911 calls to document how city- and neighborhood-level patterns of crime reporting decline following police violence [ 7 , 12 ]. Despite their important contributions, efforts to identify causal effects of police violence events have limitations. 911 calls may not be a reliable proxy to capture underlying trust in law enforcement. Furthermore, such studies often rely on data at the neighborhood or area level. The use of aggregate data poses challenges for causal inference and prevents the assessment of heterogeneous effects across different demographic groups.
The present study investigates how highly publicized events of police violence change trust in law enforcement, examining effect heterogeneity across race and ethnicity, age, and contact with the criminal legal system. These features of our research design could have implications for behaviors beyond cooperation with law enforcement such as system avoidance [ 13 ] or political participation [ 14 ].
To estimate the causal effect of police violence on trust in law enforcement, we leverage individual-level survey data in a quasi-experimental design that exploits the timing of the shooting of Jacob Blake (on August 23, 2020) relative to the fielding of the 2020 Healthy Chicago Survey (HCS), a survey of Chicago residents that the Chicago Department of Public Health ran between June 27, 2020 and December 9, 2020. Due to sample size limitations that prevent us to test effects by age, gender, and prior arrest record for other racial groups, our study primarily focuses on Black and White individuals. That being said, we do show results of an analysis of all Hispanic respondents in the S1 File . We show that individuals interviewed 4 weeks before and 4 weeks after the shooting took place are statistically identical. This enables a research design in which the group surveyed 4 weeks before the events serves as a plausible counterfactual for the group surveyed 4 weeks after.
We find large declines in trust in law enforcement among Black residents in Chicago after the shooting of Jacob Blake. During the two weeks that followed the shooting, trust in law enforcement declined by an average of 15 percentage points, a 31-percent decline from its baseline before the shooting. For Hispanic and white residents, trust in law enforcement remained unchanged. Among Black young adults (ages 18–44), law enforcement trust declined by up to 32 percentage points, with this effect lasting for three weeks. Among Black individuals who reported prior contact with the criminal legal system (e.g., having been arrested, booked, or charged at some point in the past), law enforcement trust declined by up to 36 percentage points in the two weeks following the shooting.
Our focus on individuals with prior exposure to policing and incarceration has theoretical and methodological importance; data limitations have made it difficult for researchers to identify the causal effect of police violence on institutional trust for this group. Generating causal evidence on the impact of police violence on trust is particularly important given the growing evidence showing that aggressive policing practices and police violence disproportionately affect people of color, low-income individuals, and other marginalized groups [ 15 – 17 ]. Accordingly, for sociologists of punishment, our findings constitute new evidence regarding the influence of police violence on perceptions of police among the most-policed communities. For political sociologists and political scientists, we contribute to a fast-growing literature showing how the behavior of “street-level bureaucrats” (rather than e.g. Presidential candidates) can shape citizens’ orientation toward government institutions [ 14 ].
Literature and theory
American trust in police has been historically theorized via police legitimacy, or a person’s linked perception of law enforcement and their own obligation to obey the law [ 11 ]. Legitimacy theory is complemented by the concept of legal cynicism, a cultural frame in criminalized communities holding that law enforcement agencies are illegitimate, inadequate, or otherwise harmful [ 8 , 18 ]. Bell further develops the concept of legal estrangement, wherein the experience of living in heavily policed communities reinforces a broader sense of marginalization at the hands of the law [ 19 ].
Empirical work measuring these interrelated concepts historically involved interviews or surveys digging into the mechanisms that drive e.g. Black Americans’ comparatively lower trust in police [ 5 , 20 ]. Many such studies examine the connection between legitimacy and gun violence [ 3 , 21 ]. A related body of work incorporates administrative data to assess whether events that negatively affect perceptions of police can also reduce residents’ willingness to report crimes or cooperate with law enforcement. This measure is consistent with the logic of police legitimacy, i.e., people who distrust the police are less likely to perceive reporting a crime to police as a viable solution. Desmond and coauthors found that calls for service in Milwaukee were reduced following the police beating of Frank Jude [ 7 ]. While this finding has been revised [ 22 , 23 ], recent research found a similar negative effect following the murder of George Floyd [ 12 ].
This literature overlaps with work on social organization and collective efficacy, or a group’s ability to achieve collectively desired goals [ 24 , 25 ]. Work in this spatial-ecological tradition has examined the connection between differential rates of interpersonal violence and interrelated measures of police legitimacy and willingness to enact informal social controls. Police violence may hinder collective efficacy in part by eroding trust in law enforcement and government institutions, particularly in communities where police violence is more prevalent.
Two recent studies provide strong evidence regarding the causal effects of police violence on trust. White and coauthors leverage a quasi-experimental design to assess the effect of the police killing of Freddie Gray on Baltimore residents’ perceptions of police legitimacy, but did not observe changes in perceptions of police. The authors suggest that this could follow from the lack of video footage depicting police brutality [ 26 ]. Additionally, their sample was not intended to be representative of Baltimore residents, but instead clustered in “hot spots.” Reny and Newman use a regression discontinuity design with nationally representative survey data to gauge the effect of George Floyd protests on perceptions of police, finding that protests diminished police favorability reports among politically liberal and “low-prejudice” respondents [ 27 ]. Although this study focuses on the effects of police brutality protests rather than police violence itself, these phenomena are tightly linked.
Apart from studies examining trust in police, recent work has improved the scholarly understanding of behaviors which follow from criminal legal contact (including police violence). Scholars argue that routine encounters with law enforcement constitute significant learning experiences with respect to government and one’s relationship with it [ 14 , 28 ]. Political science studies detailing these dynamics generally contribute to prior theories of political socialization and focus on outcomes including voting or other political behaviors like volunteering for political candidates. Such work finds that many forms of criminal legal contact reduce voter turnout, often with pronounced effects for Black or Latinx residents [ 29 – 31 ]. This work focuses on personal and proximal contact–being personally arrested or learning about the arrest of a friend or family member. Yet criminal legal contact may also affect perceptions of police through less direct channels. To that end, Morris and Shoub theorize “community contact”: “diffuse contact an individual has with the police via community incidents, word of mouth, and/or the media” [ 32 ]. The observed community-level effect of police violence supports a key assumption of our own analysis–that police violence can politically socialize Americans who are not personally subjected to it.
The preceding literature motivates our first hypothesis (H1): Jacob Blake’s shooting negatively affected perceptions of police for both white and Black respondents. We expect to see an effect among Black respondents in large part because Jacob Blake is Black; Morris and Shoub find stronger effects of police violence against Black victims on other Black residents’ political behavior [ 32 ]. Yet other recent work found more strongly diminished trust in police for white residents compared to Black residents [ 27 , 33 ]. These apparently contradictory findings suggest that this theoretical space is still developing.
Sociologists have argued that criminal legal contact can shape individuals’ orientations towards a wide variety of public institutions, including but not limited to law enforcement. In her analysis of “system avoidance,” Brayne found that criminalized respondents were less likely to interact with institutions that might be perceived as surveilling due to a fear of re-arrest (e.g. hospitals, schools, banks), and the negative effect intensified alongside criminal legal contact (police stops, arrests, conviction, and incarceration) [ 13 ]. These findings were further substantiated by Remster and Kramer [ 34 ], and they track with the increasing severity of withdrawal observed in the political participation literature cited above (e.g. [ 29 ]). The system avoidance literature informs our second hypothesis (H2): We expect the effect of Jacob Blake’s shooting to be more pronounced for residents who have had contact with the criminal justice system in the past (e.g., having been arrested, booked, jailed, or incarcerated) .
Prior research leads us to believe that observed effects are likely to vary by age and gender. Exploring effect heterogeneity by age is important given that young adults and early middle-aged individuals play a key role in political activism and social movements [ 35 ]–and people who recently attended protests were much younger than the general population [ 36 ]. The presence of a strong narrative of racial injustice can compel members of oft-criminalized communities to participate in non-voting political behaviors such as signing petitions or volunteering for candidates [ 37 , 38 ]. This theory is compatible with legal cynicism or legal estrangement to the extent that individual grievances are newly construed as signs of group-level inequality and accords with prior accounts of criminal legal contact catalyzing heightened political consciousness [ 17 ]. Protests are one site where such narratives could be cultivated and propagated. To the extent that protests may politically socialize participants [ 27 , 32 ] we can propose our third hypothesis (H3): The negative effect of Jacob Blake’s shooting on perceptions of police was more pronounced for younger Chicagoans .
Evidence on the gendered experience of police encounters and police violence suggests that men, particularly those from minority groups, may be more likely to experience racial profiling, excessive use of force, or discriminatory treatment [ 15 , 17 , 39 ]. Such experiences can create a higher likelihood of mistrust and negative perceptions of the police. Yet women are also subjected to similarly alienating forms of police misconduct; for instance, young Black women’s views of police are colored by sexual harassment and sexual violence, and Black mothers consistently report fears that their children will experience police violence [ 9 , 18 , 40 ]. Accordingly, we formulate our fourth hypothesis (H4): We expect trust in law enforcement to be similarly affected across Black men and women .
To sum up, police legitimacy theory proposes that unjust interactions with police or high-profile police violence events experienced vicariously lead to reduced trust in police, thus leading to reduced legal compliance. The present study differs from past literature in that we estimate individual-level average causal effects and stratify our analyses along a wide range of individual characteristics.
The events in Kenosha in August of 2020
The summer of 2020 in the United States was marked by racial tensions and social upheaval. The killing of George Floyd, a Black man, by a Minneapolis police officer on May 25, 2020 sparked nationwide protests. Floyd’s death became a symbol of entrenched systemic racism and police brutality. These racial tensions were further complicated by the COVID-19 pandemic, which disproportionately affected communities of color, exposing deep-seated disparities in healthcare, economic opportunities, and education.
It was against this backdrop that the shooting of Jacob Blake took place in Kenosha, Wisconsin. On August 23, 2020, officers responded to a domestic disturbance call involving Blake, a 29-year-old African-American who was unarmed. As Blake walked away from the officers, he was grabbed by his shirt and shot multiple times in the back by Officer Rusten Sheskey. Blake survived, but was left paralyzed from the waist down. The incident was captured on video and went viral, igniting widespread outrage and protests both in Kenosha and across the nation for 8 days following the shooting. The city declared a state of emergency, and the National Guard was deployed.
Amidst these protests, Kyle Rittenhouse, a white 17-year-old from Antioch, Illinois, crossed state lines armed with an AR-15-style rifle and arrived at Kenosha on August 24. Rittenhouse claimed he went to Kenosha to protect local businesses and provide medical aid, but on the night of August 25, 2020, during ongoing protests, Rittenhouse was involved in a series of confrontations that culminated in the shooting of three individuals.
This adds a layer of complexity to our study as it becomes difficult to disentangle the effects driven by the shooting of Jacob Blake from those driven by the Rittenhouse events. It could be that observed changes in trust in the week that followed Blake’s shooting resulted from the frustration of seeing police officers do nothing against an armed White man who shot three protesters. We will address these concerns in our empirical strategy by assessing the extent to which changes in trust in law enforcement were already visible in the two days before Rittenhouse shot three protesters.
Identifying the causal effect of police violence on trust in the police is complicated by the fact that exposure to these events is not random. Black Americans are simultaneously more likely to be victims of lethal and aggressive police violence [ 15 ] and to show lower levels of trust in the police [ 41 ]. Although previous studies relying on survey data have shown that individuals with prior contact with the police report lower levels of trust in government [ 42 ], establishing a causal relationship between the experience of police violence and trust in law enforcement can be challenging. Correlational evidence may not accurately capture the sequence of events; an individual who reports low trust following a police violence event may have lost trust long before that event, suggesting reverse causality. Similarly, self-reported data often lack control over confounding variables such as racial identity and the experience of police violence that may influence both the experience of police violence and trust in the police. Without accounting for these variables, it is challenging to establish a direct causal relationship between police violence and trust.
In this study, we overcome these challenges by using a quasi-experimental design in which we leverage the timing of a police violence event relative to a wave of survey data collection. This approach is commonly called “unexpected event during survey design,” or UESD [ 43 , 44 ]. Under the assumptions that underlie this research design, individuals surveyed before the shooting took place serve as plausible counterfactuals for individuals surveyed after the shooting. We assess the validity of this assumption in the next section.
To set up the UESD to answer our research questions, we link data from a survey that was fielded in Chicago around the time when the shooting of Jacob Blake took place (August 23, 2020). Our identification strategy relies on the exogenous timing of the shooting relative to when the survey was fielded. This allows us to compare the answers to questions about trust in the police across two sets of survey participants: those interviewed four weeks before the shooting took place and those interviewed four weeks after. We show that these two sets of respondents are statistically identical in terms of a large set of observed attributes.
Our analysis draws on Healthy Chicago Survey (HCS) data collected by the Chicago Department of Public Health between June 27 and December 9, 2020. The survey has been used to identify health concerns for each community in Chicago and to understand environmental, neighborhood, and social factors associated with health. It has been conducted annually since 2014, but the 2020 wave was the first iteration including questions about trust in police. In 2020, the survey’s format also shifted from a random-digit dial telephone survey to a self-administered, mixed-mode design wherein informants sampled by address could complete the survey online or by sending in a pencil-and-paper form.
The sampling frame in the HCS survey ensured that the data were representative of the Chicago population. RTI International, the organization in charge of designing and implementing the 2020 HCS survey, geocoded all Chicago postal addresses (N = 1,201,979) and nested them from the 77 community areas that divide the city of Chicago. From that universe of addresses, RTI targeted a minimum of 35 survey completes within each of the 77 community areas and a total of 4,500 survey completes overall. We refer readers to the 2020 Healthy Chicago Survey Methodology Report for additional details on the design and implementation of the survey [ 45 ]. The HCS sample includes 4,474 Chicago residents who are at least 18 years old. Due to its large sample size and city-wide coverage, a number of studies have used the HCS survey to study health outcomes in Chicago ([ 46 , 47 ]).
We focus on the subset of 584 Black and 939 white respondents interviewed during the eight weeks that surrounded the shooting. During that same period, only 389 Hispanic Chicagoans were surveyed, leaving us statistically under-powered to test all hypotheses for this group. The duration of this window is informed by prior studies using similar windows [ 48 ]. We focus on Black and white respondents due to statistical power limitations that prevent us from carrying out subgroup analyses for respondents of other racial and ethnic backgrounds. The constraints of the UESD design may raise concerns about the representativeness of our analytical sample. We evaluate these concerns by assessing differences across the pool of Black and white HCS survey respondents that made it to our analytical sample and Black and white HCS survey respondents that were excluded because they happened to be interviewed outside the four weeks before and after the shooting.
Ethics approval (i.e., IRB) for this study was not necessary because we are analyzing non-identifiable, publicly available survey data. All of the analyses are on secondary data, and there is no linkage of individual-level survey data to any other datasets.
The first column in Table 1 reports descriptive statistics for the subsample of Black and white respondents included in our UESD analyses. The second column shows descriptive statistics for Black and white respondents whom we excluded due to their survey response date being prior to the four weeks before or posterior to the four weeks after the shooting. We report proportions in the different categories that define race (Black and white); gender (male, female, and other gender identities); age (aged 18–29, 30–44, 45–64, and 65 and above); educational attainment (less than high school, high school diploma, and bachelor’s degree or more); home ownership status; employment status; having lived in the neighborhood for more than 5 years; having ever been arrested, booked, or jailed; and having any children. We find that our UESD analytical sample of 1,523 survey participants is almost identical to the 1,425 survey respondents that we excluded from the UESD analyses. One pattern that stands out from Table 1 is that the HCS data are not fully representative of the Chicago population. This is apparent by looking at the gender composition of the included and excluded subsamples, which are both a third male and two-thirds female. While RTI International randomly selected addresses to be contacted in each of the 77 community areas, they did not impose any restrictions on which adult member of the household would respond to the questionnaire. It is important to note, however, that demographic composition discrepancies do not pose any threats to the internal validity of our findings. 62% of respondents in our analytical sample are white and 38% are Black; 37% are male, 62% are female, and 1% identify with another gender; 16% have ages between 18 and 29, 26% have ages between 30 and 44, 31% have ages between 45 and 64, and 27% have ages 65 and above. When compared to the race, gender, and age composition of the rest of the HCS data, we don’t observe substantial differences except for the somewhat larger share of individuals aged 65 and above in our sample. Moving to educational attainment, we see that 3% of respondents in our sample did not complete high school, 13% ended their education with a high school degree only, 24% have completed some years of college education, and 58% have a college degree or more. These proportions are almost identical in the subsample of excluded HCS survey respondents. The last set of covariates show that 45% of our respondents are homeowners, 56% were employed at the time of the survey, 59% of them had lived in the neighborhood for at least 5 years, and 12% had been arrested, booked, or charged at some point in the past.
- PPT PowerPoint slide
- PNG larger image
- TIFF original image
https://doi.org/10.1371/journal.pone.0308487.t001
The outcome measures in our analysis of HCS data reflect respondents’ trust in local police. We construct the dependent variable from respondents’ answers to these this question: “To what extent do you trust your law enforcement agency?” The possible responses to this question include “a great extent, somewhat, a little, not at all.” We code these variables as binary, taking on value 1 for those that responded “a great extent” or “somewhat” and 0 for the rest. Fig 1 shows the distribution of responses to the questions that we use as outcomes regarding trust in police. We find that 49 percent of Black respondents express great or some trust in the police, with this percentage increasing to 69 for whites. In S1 Table in S1 File , we show regression-adjusted differences in trust across Black and White respondents.
Data are from the 2020 Healthy Chicago Survey. The sample includes 584 Black respondents and 939 white respondents interviewed in the four weeks prior and four weeks after the shooting of Jacob Blake. The survey question underlying the graphs is “To what extent do you trust your law enforcement agency?” .
https://doi.org/10.1371/journal.pone.0308487.g001
Fig 2 begins to examine changes in our outcome of interest in a temporal way. It presents the city-wide share of survey participants that reported trust in law enforcement in the days before and after Jacob Blake’s shooting, with separate panels for different racial groups: Black respondents (Panel a), Hispanic respondents (Panel b), and White respondents (Panel c). The horizontal axis of each graph represents the days from Jacob Blake’s shooting, with the day of the shooting denoted by a vertical red line at day 0. The vertical axis measures the share of respondents that trust the police, ranging from 0 to 1. Each panel’s data is represented by individual dots for each day, with a smoothed line to indicate the overall trend over time. In Panel (a), trust among Black respondents shows a noticeable decline beginning shortly before the shooting and reaching its nadir in the days immediately following the incident. The pattern indicates a sharp drop in trust in the day after the shooting, which then fluctuates but stays below the pre-incident levels for the observed period. In Panel (b), Hispanic respondents’ trust appears to fluctuate widely before and after the shooting, with a less pronounced dip in trust immediately after the shooting. Panel (c) shows the trust levels among White respondents, which does not exhibit a significant change at the time of the shooting but does show some fluctuation in the days following. Overall, the level of trust among white respondents remains relatively stable compared to Black respondents.
https://doi.org/10.1371/journal.pone.0308487.g002
S2 Table in S1 File shows the proportion of respondents who showed some or a great deal of trust in the police for the different subgroups for whom we estimate the impact of the shooting (i.e., race, race by age, race by criminal justice contact, and race by gender). The baseline levels of trust among the group of respondents surveyed in the four weeks before the shooting will be useful to interpret the relative size of the causal effects that our models estimate. We also show the number of respondents in each subgroup. For the analyses of Black respondents, our data include 430 respondents in the four weeks prior and 154 respondents in the four weeks after. The analyses of whites include 730 respondents in the four weeks prior and 209 respondents in the four weeks after. The sample shrinks substantially as we further stratify by other attributes. For example, for the analyses of respondents with prior contact with the police, we have 75 Black respondents in the four weeks prior and 26 in the four weeks after. For white respondents with prior contact with the police, we are left with 49 in the four weeks prior and 27 in the four weeks after. One clear pattern in S2 Table in S1 File is that there are fewer observations in the group that responded four weeks after the shooting. Across all subgroups analyses, individuals in post-shooting group are approximately evenly distributed across the four weeks that followed the event. This is a feature of the data rather than a bug. Looking at S1 Fig in S1 File , it is clear that the number of daily survey responses was generally declining over time. This downward trend in survey responses is “smooth” and does not show any discontinuity around the date of Blake’s shooting, thus strengthening the validity of our UESD design. What is relevant is that the composition of the before and after groups is generally balanced across covariates, something that we have shown in Table 2 . The modeling approach that we describe below is designed to deal with these features of the data and their related sample size concerns.
https://doi.org/10.1371/journal.pone.0308487.t002
Quasi-experimental design
We propose a UESD design that allows us to compare responses from HCS survey participants interviewed four weeks before and after the police violence events occurred. We construct a control group by pooling survey responses submitted in the 4-week period preceding the shooting. This larger control group is compared against four treatment groups, each corresponding to a one-week period in the four weeks following the shooting (i.e. August 24 to August 31 is the first treatment group). The analytical sample includes 584 Black respondents and 939 white respondents. S2 Table in S1 File shows the sample size for the treatment and control groups in the different subgroup analyses that we run.
The UESD design exploits survey response timing, which makes it conceptually similar to the regression discontinuity and interrupted time series designs. Two assumptions allowing identification of causal effects using UESD are excludability, or that the timing of the interview only affects the outcome through the treatment; and ignorability, or that the timing of each interview is independent from the potential outcomes [ 43 ].
In order to assess whether the ignorability assumption is satisfied, we conduct a covariate balance test and show that the set of survey respondents interviewed four weeks before the shooting is statistically equivalent to the set of survey respondents interviewed four weeks afterward, resembling the balance that one would expect if exposure to police violence had been randomly assigned. The empirical argument that informs our UESD design is that the timing of Jacob Blake’s shooting is exogenous with respect to the timing of when the HCS surveys were completed. This assumption could be violated if survey participants were contacted in a manner that prioritized respondents living in certain community areas over others. Having a larger share of Black respondents in the group interviewed after the shooting could confound any observed differences in trust after the shooting given that Black residents are generally less likely to trust law enforcement. Similarly, our ability to identify the causal effect of police violence on trust would be compromised if the occurrence of the shooting was what encouraged some survey participants to respond to the survey. Because the survey was self-administered, participants had the freedom to respond to the survey within a specified time frame. The selected survey participants received an invitation letter containing instructions to access the web survey and personalized login credentials. A week later, a reminder postcard was mailed to all addresses encouraging participation online. Two weeks later, non-respondents received a full paper questionnaire packet.
The balance assessment in Table 2 shows proportions for the group of respondents from four weeks before and after Blake’s shooting (first and second columns). To evaluate any imbalances, we perform t-tests for the differences in proportions across the two groups, reporting the size of the difference (third column) and the p-value from the t-test for the difference in proportions (fourth column). For most attributes, the balance assessment test reveals no systematic differences across the pool of respondents—differences in proportions are generally small and statistically non-significant at the 5% level. However, the pool interviewed after the shooting skews Black and has fewer college-educated respondents. It also includes a larger share of respondents with prior contact with the criminal justice system. Looking at patterns of survey response across community areas, we don’t observe any systematic differences in the order in which surveys from different areas were submitted. However, if community areas had been surveyed in sequential order, we could have ended with some demographic groups being more represented in the before or after groups, potentially skewing the results and introducing bias into our analysis. This could be particularly significant if some community areas have residents that are wealthier or have a higher proportion of a given racial group, which we know is the case in Chicago and most American cities.
So the differences observed in Table 2 appear to result from Black, lower-SES respondents feeling more compelled to complete the survey after the shooting. We mitigate concerns about possible compositional changes by running and reporting models with and without controls. Similarly, because we estimate models by race and criminal justice contact status (and the combination of the two), the slight imbalance seen in Table 2 becomes less problematic. Although we cannot be certain that our treatment and control groups do not differ across unobserved attributes, seeing no differences in the comprehensive list of covariates shown in Table 2 give us confidence that the ignorability assumption is not violated.
With respect to the excludability assumption, S1 Fig in S1 File shows that the frequency of HCS survey completion was not significantly altered by Jacob Blake’s shooting. A large discontinuity in responses to the survey following the shooting could suggest selection or sorting on the basis of when the survey was conducted. Fortunately, we observe no such discontinuity. Similarly, it would be cause for concern if the unexpected event appeared to affect responses to theoretically unrelated questions. Our falsification test found no evidence of this (see S6 Fig in S1 File ).
We report the main findings in Figs 3 and 4 and supplementary ones in S3 to S6 Figs in S1 File . As stated above, we lack sufficient power to run subgroup analyses by age, gender and criminal justice contact status among Hispanics, Asians, and respondents of other racial groups. Nonetheless, we report estimated impacts for all Hispanics in S3 Fig in S1 File . Each pair of point estimates in the coefficient plots shows the change in the probability of expressing trust for those surveyed one, two, three, and four weeks after Jacob Blake’s shooting, relative to the pool of survey respondents interviewed in the four weeks prior. Within each pair of estimates, we show results with and without the set of controls listed in Table 2 .
Data are from the 2020 HCS survey. The survey question used to create the measure of trust in law enforcement is “To what extent do you trust your law enforcement agency?” We code answers to this question as binary, taking on value 1 for those that responded “a great extent” or “somewhat” and 0 for the rest. Each pair of point estimates shows the change in the probability of expressing trust for those surveyed one, two, three, and four weeks after Jacob Blake’s shooting, relative to the pool of survey respondents interviewed in the four weeks prior. Within each pair of estimates, we show results with and without the set of controls listed in Table 2 . Confidence intervals are at the 95% level with standard errors robust to heteroskedasticity.
https://doi.org/10.1371/journal.pone.0308487.g003
https://doi.org/10.1371/journal.pone.0308487.g004
Our results provide varying degrees of support for our hypotheses. The clearest evidence is that in support of H1, H2 and H3: the shooting of Jacob Blake led to a decline in trust in law enforcement among the Black community driven by younger cohorts and by individuals who had previously been arrested or incarcerated. Notably, the baseline level of reported trust was lower among these subgroups of Black respondents—49 percent of all Black respondents in the control group said that they trusted police before Jacob Blake’s shooting, whereas only 32 percent of Black respondents aged 18–44 and 36 percent of Black respondents with prior arrests reported that they trusted police.
Focusing on H1, Figs 3a and 4a show results when all Black and white respondents are evaluated as a group. We find a 13 to 16 percentage point decline in trust in police among all Black respondents who completed surveys in the first and second weeks after Jacob Blake was shot. These declines are relative to the control group’s average of 49 percentage points, as shown in S2 Table in S1 File . For whites, we do not observe any substantial changes in police trust after Blake’s shooting. H1 is the only hypothesis that we have sufficient power to test among Hispanics. Results in S3 Fig in S1 File show no effect for this set of respondents.
To test H2, Figs 3b and 4b limit the analysis to respondents who reported previously being arrested, jailed, or imprisoned. We find a significant decline in reported trust in law enforcement among Black respondents: 27 and 42 percentage points in the first and second weeks, relative to a baseline of 36 percentage points. In S4 Fig in S1 File , we report smaller declines in trust in law enforcement among Black respondents who have never been arrested, jailed, or imprisoned. These patterns are consistent with H2. We don’t find any changes in trust among white respondents in this subgroup.
With respect to H3, Figs 3c and 4c show a substantial decline in trust in police among Black respondents aged 18–44. We observe declines ranging from 12 to 18 percent across the first three weeks (from a baseline of 32 percentage points). When we compare these estimates to those among Black respondents that are older than 44 (S4 Fig in S1 File ), we observe somewhat smaller declines in trust that lasted only for two weeks. While the coefficients in the two weeks following the shooting are not statistically different across the two age groups, the effect for the younger cohort increases substantially in the third week whereas the effect for the older cohort disappears. We interpret this as evidence in support of H3. As before, we find no effect among white respondents in these two age groups.
With respect to gender, we find suggestive evidence in support of H4 in Figs 3d and 4d . Black men are the group that experienced more substantial declines in trust in law enforcement in the week after Blake’s shooting: a 24-percentage-point drop from a baseline of 43 percentage points. This effect, however, is only significant at the 10 percent level. As shown in S4 Fig in S1 File , Black women exhibit smaller, statistically non-significant effects in the second week following the shooting.
Separating the Kyle Rittenhouse events
The results presented thus far indicate that Black respondents report lower trust in law enforcement in response to police violence events. One valid concern about our empirical approach is that the effect of Blake’s shooting could be influenced by the effect of the events in the days that followed. Blake was shot in Kenosha on August 23, 2020, and on the night of August 25, Kyle Rittenhouse shot three protesters in Kenosha, with both events receiving extensive news coverage at the national level. It is plausible to think that Black survey respondents lost trust in law enforcement not only because a cop shot another unarmed Black man, but because Kyle Rittenhouse (a white man) was able to attend a protest armed with an AR-15-style rifle, shoot three individuals, and return back to his hometown without being stopped or arrested by the police (he turned himself in on August 26). With a large enough sample, we could run an analysis where we model changes in trust in one-day intervals after the shooting, allowing us to observe daily changes in trust from August 23 to August 25. If the effect is driven by Blake’s shooting, we should see a decline in trust in the days when Kyle Rittenhouse had not yet arrived in Kenosha and shot anyone.
Seeing trust declines in the two days after Blake’s shooting would support our theory that police violence increases feelings of mistrust among the Black population. This is what we find in S5 Fig in S1 File . The coefficient plot shows changes in trust in two-day intervals during the six days that followed Blake’s shooting. The control group remains the set of survey participants who responded to the survey in the four weeks prior to Blake’s shooting. These models include the same set of controls that we use in models that correspond to Figs 3 and 4 . We estimate these models for the two groups for which we found the clearest effects in the models discussed above—all Black respondents and Black respondents with prior contact with the police or the criminal justice system. The coefficients that help us separate the Blake effect from the Rittenhouse effect are those estimating changes in the Aug 24–25 window. Since Rittenhouse began his shooting spree at 11:48pm on August 25, survey responses submitted on August 25 had not yet been influenced by the Rittenhouse events. We find that the trend seen in Fig 3 was set in the immediate aftermath of Blake’s shooting and before Rittenhouse shot anyone. On August 24–25, we see a 26-percentage-point decline in trust in law enforcement among all Black respondents and a 52-percentage-point decline in trust in law enforcement among Black respondents who reported prior contact with the criminal justice system. Both of these estimates are statistically significant at the 5% level. Seeing that levels of trust dropped within 48 hours of Blake’s shooting increases our confidence that the Rittenhouse events are not driving our core findings.
Alternative explanations and falsification test results
Blake’s shooting and the resultant protests unfolded during the most intense days of the COVID-19 pandemic. A change in the frequency and nature of police-civilian interactions in the local context could have influenced perceptions of trust. Evidence from Houston shows that reactive police activities (e.g., deployments of special units) significantly decreased during the pandemic, but proactive patrols significantly increased [ 50 ]. To assess the possibility that the decline in trust that our models identify is a result of Chicagoans’ perceptions of city-wide changes in the frequency of police activity, we look at discontinuities in arrest patterns in the weeks before and after Blake’s shooting. S2 Fig in S1 File shows the number of arrests in Chicago in the weeks before and after Jacob Blake’s killing, broken down by racial categories: Black arrests (Panel a), Hispanic arrests (Panel b), and white arrests (Panel c). The horizontal axis on each graph spans the weeks surrounding Jacob Blake’s shooting, with the event marked by a vertical red line at week 0. The vertical axis represents the number of arrests per week for each group. We don’t see any noticeable discontinuity in the number of each type of arrest in the weeks surrounding Jacob Blake’s shooting.
Additionally, it could be the case that local COVID-19 policies had some effect on perceptions of police (i.e., if police were enforcing stay-at-home orders). A search of Chicago COVID-19 policies and local news articles during the survey period provides little evidence suggesting that COVID-19 policies confound our analysis. The state of Illinois phased out stay-at-home orders in late May, before the 2020 HCS survey was fielded. Although an additional stay-at-home advisory was issued towards the end of the HCS survey period (effective November 16, 2020), this was less stringent than a stay-at-home order and also falls outside the temporal window for the analytical sample used in this study.
In S6 Fig in S1 File , we assess whether Jacob Blake’s shooting changed an outcome that should not have been affected by the shooting. This test is recommended to generate suggestive evidence to support the excludability assumption [ 43 ]. Unfortunately, the HCS data don’t include many questions related to behavioral outcomes that could be used for this test. Most of the questions ask respondents to report on health-related issues retroactively over long periods of time (e.g., having ever been diagnosed with hypertension), rendering them unsuitable for the falsification test. The only question that speaks to a behavioral outcome that could have changed over a short period is one on soda consumption habits. HCS participants were asked “During the past 30 days, how many regular soda or pop or other sweetened drinks like sweetened iced tea, sports drinks, fruit punch or other fruit-flavored drinks have you had per day?” We use this question to test whether the shooting changed the probability of consuming more than one soda drink per day. We find no evidence of any changes in soda consumption in the aftermath of the shooting.
While prior research has thoroughly probed the descriptive relationship between criminalization and perceptions of police, few prior studies credibly identify individual-level causal effects. We examine whether these effects differ by age, gender, racial identity, or prior exposure to criminalization. While some recent work has examined effect heterogeneity according to e.g. partisan identification and media consumption habits [ 27 ], our study builds on gaps in existing literature by testing effects among various demographic subgroups and gauging their durability over time.
We find substantial, yet short-lived declines in perceptions of law enforcement among Black Chicago residents following the shooting of Jacob Blake. These effects were particularly pronounced among younger cohorts and respondents who reported prior contact with the criminal legal system. Unlike prior work, we do not find particularly strong evidence of a stronger effect for Black men compared to Black women. We also find that the decline in trust that we report in our core findings began in the immediate aftermath of Blake’s shooting—before Rittenhouse arrived in Kenosha and shot three protesters.
We believe that three of our four hypotheses pass a ‘hard test’: we saw declines in trust in police among Black Chicagoans whose quasi-experimental counterparts already reported very low levels of trust before Jacob Blake’s shooting. These respondents had likely been exposed to the police murder of George Floyd a few months earlier, which was a similarly salient continuing national news story. If we think about the Black Chicagoans in our study as subjects of many prior, analogous “treatments,” the fact that we observed any effect at all is notable.
We acknowledge that some features of this police violence incident (the Kyle Rittenhouse events and potential confounding related to the the COVID-19 pandemic) may initially give readers reason to doubt the viability of our identification strategy. We endeavoured to address these concerns through a series of robustness tests which ultimately increased our confidence in the analysis and findings.
Another potential threat to our identification strategy is noncompliance, i.e., some survey respondents may not have been aware of the police violence event [ 43 ]. If this were the case, our estimates could be interpreted as “intent-to-treat” (ITT) rather than the average treatment effect (ATE). That being said, Blake’s shooting was highly salient both in Chicago and nationwide and was covered by all major newspapers, TV, and radio channels for several days.
One limitation of the UESD design (in general) is that unique events may limit the generalizability of findings. Unfortunately, police violence is a relatively frequent event in America: according to the nonprofit research organization Mapping Police Violence, at least 1,176 people were killed by police in 2022. Granted, most of these killings do not reach the same level of public awareness as e.g. George Floyd, but police violence is still a regular phenomenon, and media coverage of police violence has increased over time [ 27 ].
We are also unable to probe certain causal mechanisms driving observed effects. For example, Reny and Newman [ 27 ] tests self-reported media consumption and geographical proximity to George Floyd protests. It could be the case that our observed effects were driven in part by news outlets’ amplification of Jacob Blake’s shooting or viral social media posts [ 51 , 52 ]. Although it is true that news coverage of police violence has increased following the rise of the Black Lives Matter movement [ 53 ], we find implausible that our observed effects primarily result from selective media amplification of police violence incidents. Research on social media usage shows that individuals who do not intentionally use social media for news but encounter it incidentally on platforms like Facebook, YouTube, and Twitter tend to engage with a wider array of online news sources compared to those who do not use social media at all [ 54 ]. This incidental exposure effect is notably stronger among younger users and those with a low interest in the news. This suggests that, independently of how traditional news outlets covered Jacob Blake’s shooting, the occurrence of such an event likely reached a broad segment of the population.
Furthermore, past research suggests that individuals who have previously been arrested (one of the groups for which we observe the strongest effects) are less likely to have their views influenced by crime news coverage [ 55 ]. Additionally, even if fewer news stories about the shooting were published, it seems possible that viral social media posts would have ensured that the survey respondents were informed about the shooting. Further research is required to better formulate a complete account of legal cynicism via police violence. Our design also cannot differentiate between the effect of police violence itself and the effect of ensuing protests. Yet there would be no police brutality protests without police brutality.
Finally, survey responses may be an imperfect proxy for actual beliefs and behaviors, and particularly with respect to law enforcement (as highlighted by [ 7 ]). This is particularly true when measuring interactions with the police, as surveys can be less reliable in capturing genuine experiences and sentiments [ 56 ]. While some might express a reluctance to cooperate with the police in surveys, their actions might indicate otherwise. This divergence between stated attitudes and actual behaviors underscores the potential pitfalls of relying on survey data alone. Yet for the specific question examined in this paper—whether police violence affects trust in police (rather than e.g. willingness to report a crime)—these potential shortcomings are likely less salient.
Moving away from the limitations of our study, the fact that we observed substantial effects only among Black respondents is somewhat puzzling because recent, similar studies have found different results. In the wake of George Floyd’s murder, Reny and Newman found that changes in white Americans’ attitudes towards police were larger than changes among Black Americans [ 27 ]. Anoll and coauthors found that white survey respondents’ attitudes towards law enforcement were much more strongly associated with recent criminal legal contact compared to Black men [ 33 ]. Yet we observed a much stronger effect for Black respondents–even when we limited the sample to only those who reported prior police contact. Speculating beyond the survey data, it could be the case that white supremacist organizing in defense of Kyle Rittenhouse following Jacob Blake’s shooting by police influenced the average effect we observed for white respondents. The chronology of police violence events in 2020 may also be explanatory–perhaps changes in white attitudes towards police had already reached a ‘ceiling’ following George Floyd protests in the spring and summer.
The idea that our target population was “pre-treated” via exposure to previous police violence incidents (e.g. George Floyd) may also help to explain why most of the effects we observe here appear to be short-lived. To the extent that acquiring new information about a police violence incident constitutes ‘learning’ about one’s own relationship to police or government more broadly, Black residents have ‘less to learn’ due to their disproportionate historical exposure to policing and incarceration (at the personal, proximal, and community levels). Some recent work has found similar temporal bounds for related treatment effects, i.e., Black residents who were ticketed closer to the date of an election were less likely to vote compared to voters who were ticketed further away from the election date [ 31 ]. It could have been the case that Black respondents experienced a powerful short-term reaction (i.e., “anticipatory stress of police brutality,” per Alang and coauthors [ 57 ]) when hearing about a new police violence incident, and the salience faded as the incident was incorporated into pre-existing knowledge and narratives of group-level exposure to criminalization.
We also note with interest that we observed more pronounced negative effects among Black men than Black women, which may be seen as contrary to the predictions of prior scholarship. It may be the case that Black men responded more strongly to Jacob Blake’s shooting since the specific context of the incident corresponded more directly to the types of police violence or misconduct most commonly experienced by men rather than women; the vast majority of American police shooting victims are men.
To the extent that legal cynicism might mediate police violence and e.g. political participation or system avoidance, the direction of any subsequent causal effect is not a given. For example, while personal exposure to criminalization tends to decrease the likelihood that an individual will vote [ 30 , 31 ], community-level indirect exposure can increase voter turnout [ 32 ]. Although Brantingham and coauthors found stable crime reporting trends in the wake of George Floyd protests [ 58 ], this may reflect an awareness that police were the only available option that residents could turn to in response to e.g. interpersonal violence. Further research is required to determine how legal cynicism affects participation in civic life across a variety of contexts and for different groups of Americans. These effects could also plausibly shift over time, as Americans are exposed to new “injustice narratives” and nascent protest movements.
Taken together, our findings enhance our understanding of the ways in which racial identity and the lived experience of criminalization affect perceptions of police. In addition to prior literature suggesting that declining trust in police could lead to e.g. lower rates of crime reporting, our study suggests that police violence drives legal cynicism among Black youth, plausibly shaping a much wider range of activities of interest.
Supporting information
S1 file. appendix containing additional figures and tables..
https://doi.org/10.1371/journal.pone.0308487.s001
- View Article
- Google Scholar
- PubMed/NCBI
- 6. Sampson RJ. Neighborhood Inequality, Violence, and the Social Infrastructure of the American City. In: Tate WF IV, editor. Research on Schools, Neighborhoods, and Communities: Toward Civic Responsibility. Published for the American Educational Research Association by Rowman and Littlefield, Inc.; 2012. p. 11–28.
- 11. Fagan J, Tyler T, Meares TL. Street Stops and Police Legitimacy in New York. In Jacqueline E. Ross and Thierry Delpeuch (eds.), Comparing the Democratic Governance of Police Intelligence: New Models of Participation and Expertise in the United States and Europe 203 (2016). URL: https://papers.ssrn.com/abstract=2795175 .
- 12. Ang D, Bencsik P, Bruhn J, Derenoncourt E. Police Violence Reduces Civilian Cooperation and Engagement with Law Enforcement. Working Paper. 2021.
- 17. Rios VM. Punished: Policing the Lives of Black and Latino Boys. NYU Press; 2011. Available from: https://nyupress.org/9780814776384/punished .
- 20. Tyler TR, Huo YJ. Trust in the Law: Encouraging Public Cooperation with the Police and Courts Through. Russell Sage Foundation; 2002. Available from: https://www.jstor.org/stable/10.7758/9781610445429 .
- 21. Papachristos AV, Meares TL, Fagan J. Why Do Criminals Obey the Law? The Influence of Legitimacy and Social Networks on Active Gun Offenders. 2009. https://papers.ssrn.com/abstract=1326631 .
- 29. Lerman AE, Weaver VM. Arresting citizenship: the democratic consequences of American crime control. Chicago studies in American politics. Chicago; London: The University of Chicago Press; 2014.
- 35. Leighley JE, Nagler J. Who votes now?: Demographics, issues, inequality, and turnout in the United States. Princeton University Press; 2013.
- 37. Walker HL. Mobilized by injustice: criminal justice contact, political participation, and race. NewYork: Oxford University Press 2020; 2020.
- 39. Fagan JA, Geller A, Davies G, West V. Street Stops and Broken Windows Revisited: The Demography and Logic of Proactive Policing in a Safe and Changing City. In: Rice SK, White MD, editors. Race, Ethnicity, and Policing. New York University Press; 2010. p. 309–348.
- 45. RTI International. 2020 Healthy Chicago Survey (HCS): Methodology Report; 2020. https://www.chicago.gov/content/dam/city/depts/cdph/statistics_and_reports/2020_HCS_Methodology_Report.pdf .
Quasi-Experimenteller Aufbau: Definition, Arten, Beispiele
Appinio Research · 11.09.2024 · 37min Lesezeit
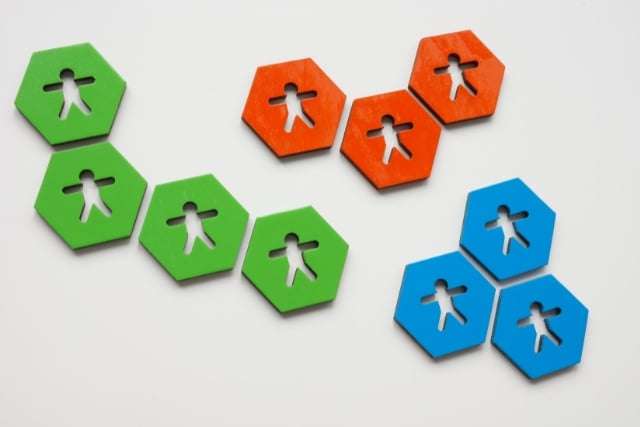
Haben Sie sich schon einmal gefragt, wie Forscher Ursache-Wirkungs-Beziehungen in der realen Welt aufdecken, wo kontrollierte Experimente oft nicht möglich sind? Die quasi-experimentelle Planung ist der Schlüssel dazu. In diesem Leitfaden werden wir die Feinheiten des quasi-experimentellen Designs enträtseln und seine Definition, seinen Zweck und seine Anwendungen in verschiedenen Bereichen beleuchten. Egal, ob Sie studieren, berufstätig sind oder einfach nur neugierig auf die Methoden hinter aussagekräftigen Forschungsergebnissen sind, begleiten Sie uns, wenn wir in die Welt der quasi-experimentellen Planung eintauchen, um komplexe Konzepte zu vereinfachen und uns auf eine Reise des Wissens und der Entdeckung zu begeben.
Was ist ein quasi-experimentelles Design?
Ein quasi-experimentelles Design ist eine Forschungsmethode, mit der die Auswirkungen unabhängiger Variablen auf abhängige Variablen untersucht werden, wenn eine vollständige experimentelle Kontrolle nicht möglich oder ethisch nicht vertretbar ist. Sie liegt zwischen kontrollierten Experimenten, bei denen die Variablen streng kontrolliert werden, und reinen Beobachtungsstudien, bei denen die Forscher kaum Kontrolle über die Variablen haben. Das quasi-experimentelle Design ahmt einige Aspekte der experimentellen Forschung nach, verfügt aber nicht über eine Randomisierung.
Der Hauptzweck des quasi-experimentellen Designs besteht darin, Ursache-Wirkungs-Beziehungen zwischen Variablen in realen Umgebungen zu untersuchen. Forscher nutzen diesen Ansatz, um Forschungsfragen zu beantworten, Hypothesen zu testen und die Auswirkungen von Maßnahmen oder Behandlungen zu untersuchen, wenn sie keine traditionellen experimentellen Methoden anwenden können. Quasi-experimentelle Studien zielen darauf ab, die interne Validität zu maximieren und aussagekräftige Schlüsse zu ziehen, wobei praktische Einschränkungen und ethische Erwägungen berücksichtigt werden.
Quasi-experimentelles vs. experimentelles Design
Es ist wichtig, die Unterschiede zwischen quasi-experimentellem und experimentellem Design zu verstehen, um die einzigartigen Merkmale jedes Ansatzes zu verstehen:
- Randomisierung: Bei der experimentellen Planung ist die zufällige Zuordnung der Teilnehmer zu den Gruppen ein entscheidendes Merkmal. Bei der quasi-experimentellen Planung hingegen wird aufgrund praktischer Einschränkungen oder ethischer Erwägungen auf eine Randomisierung verzichtet.
- Kontrollgruppen : Experimentelle Studien umfassen in der Regel Kontrollgruppen, die keiner Behandlung oder einem Placebo unterzogen werden. Das quasi-experimentelle Design kann Vergleichsgruppen haben, hat aber nicht das gleiche Maß an Kontrolle.
- Manipulation der IV: Die experimentelle Planung beinhaltet die absichtliche Manipulation der unabhängigen Variablen. Bei quasi-experimentellen Versuchsplänen geht es oft um natürlich vorkommende unabhängige Variablen.
- Kausalschluss: Die experimentelle Planung ermöglicht aufgrund der Randomisierung und Kontrolle stärkere Kausalschlüsse. Quasi-experimentelles Design erlaubt kausale Schlüsse, allerdings mit einigen Einschränkungen.
Wann sollte man ein quasi-experimentelles Design verwenden?
Ein quasi-experimentelles Design ist in verschiedenen Situationen besonders wertvoll:
- Ethische Einschränkungen: Wenn die Manipulation der unabhängigen Variable ethisch nicht vertretbar oder unpraktisch ist, bietet ein quasi-experimentelles Design eine Alternative zur Untersuchung natürlich vorkommender Variablen.
- Reale Umgebungen: Wenn Forscher Phänomene in realen Kontexten untersuchen wollen, ermöglicht ihnen das quasi-experimentelle Design, dies ohne künstliche Laborumgebungen zu tun.
- Begrenzte Ressourcen: In Fällen, in denen die Ressourcen begrenzt sind und die Durchführung eines kontrollierten Experiments zu kostspielig ist, kann ein quasi-experimentelles Design wertvolle Erkenntnisse liefern.
- Politik- und Programmbewertung: Quasi-experimentelles Design wird häufig zur Bewertung der Wirksamkeit von Maßnahmen, Interventionen oder Programmen verwendet, die den Teilnehmern nicht zufällig zugewiesen werden können.
Bedeutung des quasi-experimentellen Designs in der Forschung
Quasi-experimentelles Design spielt in der Forschung aus mehreren Gründen eine wichtige Rolle:
- Komplexität der realen Welt: Sie ermöglicht es den Forschern, komplexe Probleme der realen Welt anzugehen, bei denen kontrollierte Experimente nicht durchführbar sind. Damit wird die Lücke zwischen kontrollierten Experimenten und reinen Beobachtungsstudien geschlossen.
- Ethische Forschung: Sie bietet einen ehrlichen Ansatz, wenn die Manipulation von Variablen oder die Zuweisung von Behandlungen den Teilnehmern schaden oder ethische Standards verletzen könnte.
- Auswirkungen auf Politik und Praxis: Quasi-experimentelle Studien führen zu Erkenntnissen, die direkt in die Politikgestaltung einfließen und praktische Lösungen in Bereichen wie Bildung, Gesundheitswesen und Sozialwissenschaften bieten.
- Verbesserte externe Validität: Ergebnisse aus quasi-experimenteller Forschung haben oft eine hohe externe Validität, wodurch sie besser auf breitere Bevölkerungsgruppen und Kontexte anwendbar sind.
Indem sie die Herausforderungen und Chancen des quasi-experimentellen Designs annehmen, können Forscher wertvolle Erkenntnisse zu ihrem jeweiligen Fachgebiet beitragen und positive Veränderungen in der realen Welt vorantreiben.
Schlüsselkonzepte im quasi-experimentellen Design
Beim quasi-experimentellen Design ist es wichtig, die grundlegenden Konzepte zu verstehen, die dieser Forschungsmethodik zugrunde liegen. Lassen Sie uns diese Schlüsselkonzepte im Detail untersuchen.
Unabhängige Variable
Die unabhängige Variable (IV) ist der Faktor, den Sie in Ihrer Forschung untersuchen oder manipulieren möchten. Im Gegensatz zu kontrollierten Experimenten, bei denen Sie die IV direkt beeinflussen können, geht es bei quasi-experimentellen Versuchsplänen oft um natürlich vorkommende Variablen. Wenn Sie zum Beispiel die Auswirkungen einer neuen Lehrmethode auf die Schülerleistungen untersuchen, ist die Lehrmethode Ihre unabhängige Variable.
Abhängige Variable
Die abhängige Variable (DV) ist das Ergebnis oder die Reaktion, die Sie messen, um die Auswirkungen von Veränderungen der unabhängigen Variable zu bewerten. Um beim Beispiel der Lehrmethode zu bleiben, wäre die abhängige Variable die akademische Leistung der Schüler, die in der Regel anhand von Testergebnissen, Noten oder anderen relevanten Messgrößen gemessen wird.
Kontrollgruppen vs. Vergleichsgruppen
Auch wenn bei quasi-experimentellen Studien der Luxus fehlt, die Teilnehmer nach dem Zufallsprinzip einer Kontroll- und einer Versuchsgruppe zuzuordnen, können Sie dennoch Vergleichsgruppen bilden, um aussagekräftige Schlüsse zu ziehen. Kontrollgruppen bestehen aus Personen, die die Behandlung nicht erhalten, während Vergleichsgruppen verschiedenen Stufen oder Varianten der Behandlung ausgesetzt sind. Diese Gruppen helfen den Forschern, die Wirkung der unabhängigen Variable zu messen.
Pre-Test und Post-Test Maßnahmen
Bei quasi-experimentellen Studien ist es üblich, Daten sowohl vor als auch nach der Einführung der unabhängigen Variable zu erheben. Die anfänglichen Daten (Pre-Test) dienen als Ausgangsbasis, so dass Sie die Veränderungen im Laufe der Zeit (Post-Test) messen können. Auf diese Weise lassen sich die Auswirkungen der unabhängigen Variable genauer beurteilen. Wenn Sie zum Beispiel die Wirksamkeit eines neuen Medikaments untersuchen, messen Sie den Gesundheitszustand der Patienten vor der Verabreichung des Medikaments (Pre-Test) und danach (Post-Test).
Bedrohungen der internen Validität
Die interne Validität ist entscheidend für den Nachweis einer Ursache-Wirkungs-Beziehung zwischen den unabhängigen und abhängigen Variablen. Bei einem quasi-experimentellen Design kann die interne Validität jedoch durch mehrere Faktoren beeinträchtigt werden. Zu diesen Bedrohungen gehören:
- Selektionsverzerrung : Wenn sich nicht randomisierte Gruppen systematisch in einer Weise unterscheiden, die das Ergebnis der Studie beeinflusst.
- Historische Effekte: Externe Ereignisse oder Veränderungen im Laufe der Zeit, die die Ergebnisse beeinflussen.
- Reifungseffekte: Natürliche Veränderungen oder Entwicklungen, die bei den Teilnehmern während der Studie auftreten.
- Regression zum Mittelwert: Die Tendenz der extremen Werte einer Variablen, sich bei einer erneuten Prüfung dem Mittelwert anzunähern.
- Attrition und Mortalität: Der Verlust von Teilnehmern im Laufe der Zeit, der die Ergebnisse möglicherweise verzerrt.
- Testeffekte: Der bloße Akt des Testens oder Beurteilens von Teilnehmern kann sich auf deren spätere Leistung auswirken.
Das Verständnis dieser Risiken ist für die Planung und Durchführung von quasi-experimentellen Studien, die gültige und zuverlässige Ergebnisse liefern, unerlässlich.
Randomisierung und Nicht-Randomisierung
In traditionellen experimentellen Studien ist die Randomisierung ein wirksames Mittel, um sicherzustellen, dass die Gruppen zu Beginn der Studie gleichwertig sind. Bei quasi-experimentellen Versuchsplänen wird jedoch aufgrund der Art der Untersuchung häufig auf eine Randomisierung verzichtet. Das bedeutet, dass die Teilnehmer nicht nach dem Zufallsprinzip den Behandlungs- und Kontrollgruppen zugewiesen werden. Stattdessen müssen die Forscher verschiedene Techniken anwenden, um Verzerrungen zu minimieren und sicherzustellen, dass die Gruppen so ähnlich wie möglich sind.
Wenn Sie beispielsweise eine Studie über die Auswirkungen einer neuen Lehrmethode in einem realen Klassenzimmer durchführen, können Sie die Schüler nicht nach dem Zufallsprinzip den Behandlungs- und Kontrollgruppen zuordnen. Stattdessen können Sie statistische Methoden verwenden, um die Schüler anhand relevanter Merkmale wie früherer akademischer Leistungen oder des sozioökonomischen Status zu vergleichen. Dieser Abgleich hilft bei der Kontrolle potenzieller Störvariablen und erhöht die Validität Ihrer Studie.
Arten von quasi-experimentellen Designs
Bei quasi-experimentellen Versuchsplänen verwenden Forscher verschiedene Ansätze, um kausale Zusammenhänge zu untersuchen und die Auswirkungen unabhängiger Variablen zu untersuchen, wenn eine vollständige experimentelle Kontrolle schwierig ist. Im Folgenden werden diese Arten von quasi-experimentellen Versuchsplänen erläutert.
Ein-Gruppen-Posttest-Only-Versuch
Der Ein-Gruppen-Posttest-Only-Versuch ist eine der einfachsten Formen des quasi-experimentellen Versuchsplans. Bei diesem Design wird eine einzige Gruppe der unabhängigen Variable ausgesetzt, und die Daten werden erst nach der Intervention erhoben. Im Gegensatz zu kontrollierten Experimenten gibt es keine Vergleichsgruppe. Dieses Design ist nützlich, wenn die Forscher keinen Vortest durchführen können oder wenn dies logistisch schwierig ist.
Beispiel : Angenommen, Sie möchten die Wirksamkeit eines neuen Zeitmanagement-Seminars bewerten. Sie bieten das Seminar einer Gruppe von Mitarbeitern an und messen unmittelbar danach deren Produktivität, um festzustellen, ob es eine erkennbare Wirkung gibt.
Ein-Gruppen-Pretest-Posttest-Design
Ähnlich wie beim Nur-Posttest-Design für eine Gruppe umfasst dieser Ansatz zusätzlich zum Posttest eine Pretest-Messung. Die Forscher erheben Daten sowohl vor als auch nach der Intervention. Durch den Vergleich der Pretest- und Posttest-Ergebnisse innerhalb derselben Gruppe erhalten Sie ein besseres Verständnis für die Veränderungen, die durch die unabhängige Variable auftreten.
Beispiel : Wenn Sie die Auswirkungen eines Stressbewältigungsprogramms auf das Stressniveau der Teilnehmer untersuchen, würden Sie deren Stressniveau vor dem Programm (Vortest) und nach Abschluss des Programms (Nachtest) messen, um etwaige Veränderungen zu bewerten.
Nicht-äquivalentes Gruppendesign
Das Design der nicht-äquivalenten Gruppen umfasst mehrere Gruppen, die jedoch nicht zufällig zugewiesen werden. Stattdessen müssen die Forscher die relevanten Variablen sorgfältig abgleichen oder kontrollieren, um Verzerrungen zu minimieren. Dieses Design ist besonders nützlich, wenn eine zufällige Zuweisung nicht möglich oder ethisch nicht vertretbar ist.
Beispiel : Stellen Sie sich vor, Sie untersuchen die Wirksamkeit von zwei Lehrmethoden an zwei verschiedenen Schulen. Sie können die Schüler nicht nach dem Zufallsprinzip den Schulen zuweisen, aber Sie können sie anhand von Faktoren wie Alter, frühere akademische Leistungen und sozioökonomischer Status sorgfältig zuordnen, um gleichwertige Gruppen zu bilden.
Zeitreihen-Design
Das Zeitreihendesign ist ein Ansatz, bei dem Daten zu mehreren Zeitpunkten vor und nach der Intervention erfasst werden. Dieses Design ermöglicht es den Forschern, Trends und Muster im Laufe der Zeit zu analysieren, was wertvolle Einblicke in die anhaltenden Auswirkungen der unabhängigen Variable liefert.
Beispiel : Wenn Sie die Auswirkungen einer neuen Marketingkampagne auf die Produktverkäufe untersuchen, würden Sie die Verkaufsdaten in regelmäßigen Abständen (z. B. monatlich) vor und nach dem Start der Kampagne erfassen, um langfristige Trends zu beobachten.
Regressions-Diskontinuitäts-Design
Das Regressions-Diskontinuitäts-Design wird eingesetzt, wenn die Teilnehmer auf der Grundlage eines bestimmten Grenzwerts oder Schwellenwerts verschiedenen Gruppen zugeordnet werden. Dieses Design wird häufig in der Bildungs- und Politikforschung verwendet, um die Auswirkungen von Interventionen in der Nähe eines Grenzwertes zu bewerten.
Beispiel : Angenommen, Sie bewerten die Auswirkungen eines Stipendienprogramms auf die akademischen Leistungen der Schüler. Studierende, die knapp über oder unter einem bestimmten GPA-Schwellenwert liegen, werden dem Programm unterschiedlich zugewiesen. Dieses Design hilft bei der Bewertung der Wirksamkeit des Programms am Grenzwert.
Propensity Score Matching
Propensity Score Matching ist eine Technik, mit der in nicht-randomisierten Studien vergleichbare Behandlungs- und Kontrollgruppen gebildet werden. Die Forscher berechnen Propensity Scores auf der Grundlage der Merkmale der Teilnehmer und gleichen die Personen in der Behandlungsgruppe mit denen in der Kontrollgruppe ab, die ähnliche Werte aufweisen.
Beispiel : Wenn Sie die Auswirkungen eines neuen Medikaments auf die Ergebnisse von Patienten untersuchen, würden Sie Propensity Scores verwenden, um Patienten, die das Medikament erhalten haben, mit denen abzugleichen, die es nicht erhalten haben, aber ähnliche Gesundheitsprofile aufweisen.
Unterbrochenes Zeitreihendesign
Beim unterbrochenen Zeitreihendesign werden Daten zu mehreren Zeitpunkten vor und nach der Einführung einer Intervention erhoben. Bei diesem Design findet die Intervention jedoch zu einem bestimmten Zeitpunkt statt, so dass die Forscher ihre unmittelbare Wirkung bewerten können.
Beispiel : Angenommen, Sie analysieren die Auswirkungen eines neuen Verkehrsmanagementsystems auf die Verkehrsunfälle. Sie erheben Unfalldaten vor und nach der Einführung des Systems, um etwaige abrupte Veränderungen direkt nach der Einführung zu beobachten.
Jedes dieser quasi-experimentellen Designs bietet einzigartige Vorteile und ist für bestimmte Forschungsfragen und Szenarien am besten geeignet. Die Wahl des richtigen Designs ist entscheidend für die Durchführung robuster und informativer Studien.
Vor- und Nachteile des quasi-experimentellen Designs
Das quasi-experimentelle Design bietet einen wertvollen Forschungsansatz, aber wie jede Methode hat auch diese ihre eigenen Vor- und Nachteile. Lassen Sie uns diese im Detail untersuchen.
Vorteile des quasi-experimentellen Designs
Das quasi-experimentelle Design bietet mehrere Vorteile, die es zu einem wertvollen Instrument in der Forschung machen:
- Anwendbarkeit in der realen Welt: Quasi-experimentelle Studien finden oft in realen Umgebungen statt, wodurch die Ergebnisse besser auf praktische Situationen anwendbar sind. Forscher können die Auswirkungen von Interventionen oder Variablen in dem Kontext untersuchen, in dem sie natürlicherweise auftreten.
- Ethische Erwägungen: In Situationen, in denen es unethisch wäre, die unabhängige Variable in einem kontrollierten Experiment zu manipulieren, bietet das quasi-experimentelle Design eine ethische Alternative. So wäre es beispielsweise unethisch, Teilnehmer für eine Studie über die gesundheitlichen Auswirkungen des Rauchens dem Rauchen zuzuweisen, aber Sie können natürlich vorkommende Gruppen von Rauchern und Nichtrauchern untersuchen.
- Kosteneffizienz: Die Durchführung quasi-experimenteller Forschung ist oft kostengünstiger als die Durchführung kontrollierter Experimente. Der Verzicht auf kontrollierte Umgebungen und umfangreiche Manipulationen kann sowohl Zeit als auch Ressourcen einsparen.
Diese Vorteile machen das quasi-experimentelle Design zu einer attraktiven Wahl für Forscher, die bei ihren Studien mit praktischen oder ethischen Beschränkungen konfrontiert sind.
Nachteile des quasi-experimentellen Designs
Das quasi-experimentelle Design birgt jedoch auch einige Herausforderungen und Nachteile in sich:
- Begrenzte Kontrolle: Im Gegensatz zu kontrollierten Experimenten, bei denen die Forscher die volle Kontrolle über die Variablen haben, fehlt bei quasi-experimentellen Versuchsplänen das gleiche Maß an Kontrolle. Diese eingeschränkte Kontrolle kann zu Störvariablen führen, die es schwierig machen, Kausalität nachzuweisen.
- Gefahren für die interne Validität: Verschiedene Gefahren für die interne Validität, wie z. B. Selektionsverzerrungen, historische Effekte und Reifungseffekte, können die Genauigkeit der kausalen Schlussfolgerungen beeinträchtigen. Die Forscher müssen diese Gefahren sorgfältig berücksichtigen, um die Gültigkeit ihrer Ergebnisse zu gewährleisten.
- Herausforderungen beim Kausalitätsschluss: Der Nachweis der Kausalität kann bei quasi-experimentellen Designs aufgrund des Fehlens von Randomisierung und Kontrolle schwierig sein. Sie können zwar überzeugende Argumente für die Kausalität vorbringen, aber sie sind möglicherweise nicht so schlüssig wie bei kontrollierten Experimenten.
- Potenzielle Störvariablen: Bei einem quasi-experimentellen Design ist es oft schwierig, alle möglichen Störvariablen zu kontrollieren, die die abhängige Variable beeinflussen können. Dies kann dazu führen, dass Veränderungen nicht ausschließlich auf die unabhängige Variable zurückgeführt werden können.
Trotz dieser Nachteile bleibt das quasi-experimentelle Design ein wertvolles Forschungsinstrument, wenn es mit Bedacht und im Bewusstsein seiner Grenzen eingesetzt wird. Forscher sollten ihre Forschungsfragen und die praktischen Einschränkungen, mit denen sie konfrontiert sind, sorgfältig abwägen, bevor sie diesen Ansatz wählen.
Wie führt man eine quasi-experimentelle Studie durch?
Die Durchführung einer quasi-experimentellen Studie erfordert eine sorgfältige Planung und Durchführung, um die Gültigkeit Ihrer Forschung zu gewährleisten. Im Folgenden werden die wesentlichen Schritte erläutert, die Sie bei der Durchführung einer solchen Studie beachten müssen.
1. Definieren Sie Forschungsfragen und Zielsetzungen
Der erste Schritt eines jeden Forschungsvorhabens besteht darin, Ihre Forschungsfragen und -ziele klar zu definieren. Dazu müssen Sie die unabhängige Variable (IV) und die abhängige Variable (DV) bestimmen, die Sie untersuchen wollen. Welche Beziehung wollen Sie erforschen, und was wollen Sie mit Ihrer Forschung erreichen?
- Legen Sie Ihre Forschungsfragen fest: Formulieren Sie zunächst präzise Forschungsfragen, die Ihre Studie beantworten soll. Diese Fragen sollten klar, fokussiert und für Ihr Studiengebiet relevant sein.
- Identifizieren Sie die unabhängige Variable: Definieren Sie die Variable, die Sie in Ihrer Studie manipulieren oder untersuchen wollen. Bestimmen
- Sie die abhängige Variable: Bestimmen Sie die Ergebnis- oder Reaktionsvariable, die durch Änderungen der unabhängigen Variable beeinflusst wird.
- Stellen Sie Hypothesen auf (falls zutreffend): Wenn Sie spezifische Hypothesen über die Beziehung zwischen IV und DV haben, geben Sie diese klar an. Hypothesen bieten einen Rahmen für die Prüfung Ihrer Forschungsfragen.
2. Wählen Sie ein geeignetes quasi-experimentelles Design
Die Wahl des richtigen quasi-experimentellen Designs ist entscheidend für das Erreichen Ihrer Forschungsziele. Wählen Sie ein Design, das auf Ihre Forschungsfragen und die verfügbaren Daten abgestimmt ist. Berücksichtigen Sie Faktoren wie die Durchführbarkeit des Designs und die damit verbundenen ethischen Überlegungen.
- Bewerten Sie Ihre Forschungsziele: Bewerten Sie Ihre Forschungsfragen und -ziele, um zu bestimmen, welche Art von quasi-experimentellem Design am besten geeignet ist. Jedes Design hat seine Stärken und Grenzen, wählen Sie also eines, das Ihren Zielen entspricht.
- Berücksichtigen Sie ethische Einschränkungen: Berücksichtigen Sie alle ethischen Bedenken in Bezug auf Ihre Forschung. Je nach dem Kontext Ihrer Studie können einige Designs ethisch besser vertretbar sein als andere.
- Beurteilen Sie die Datenverfügbarkeit: Stellen Sie sicher, dass Sie Zugang zu den erforderlichen Daten für das von Ihnen gewählte Design haben. Einige Designs erfordern umfangreiche historische Daten, während andere auf Daten angewiesen sind, die während der Studie erhoben werden.
3. Identifizieren und rekrutieren Sie Teilnehmer
Die Auswahl der richtigen Teilnehmer ist ein entscheidender Aspekt der quasi-experimentellen Forschung. Die Teilnehmer sollten die Population repräsentieren, über die Sie Rückschlüsse ziehen wollen, und Sie müssen ethische Erwägungen berücksichtigen, einschließlich der informierten Zustimmung.
- Definieren Sie Ihre Zielpopulation: Bestimmen Sie die Population, auf die Ihre Studie verallgemeinert werden soll. Ihre Stichprobe sollte repräsentativ für diese Population sein.
- Rekrutierungsprozess: Entwickeln Sie einen Plan für die Rekrutierung von Teilnehmern. Je nach Design müssen Sie möglicherweise bestimmte Gruppen oder Institutionen ansprechen.
- Informierte Zustimmung: Stellen Sie sicher, dass Sie die informierte Zustimmung der Teilnehmer einholen. Erläutern Sie klar und deutlich die Art der Studie, mögliche Risiken und ihre Rechte als Teilnehmer.
4. Daten sammeln
Die Datenerhebung ist ein entscheidender Schritt in der quasi-experimentellen Forschung. Sie müssen einen konsistenten und systematischen Prozess einhalten, um relevante Informationen vor und nach der Intervention oder Behandlung zu sammeln.
- Pre-Test-Maßnahmen: Erheben Sie gegebenenfalls Daten, bevor Sie die unabhängige Variable einführen. Stellen Sie sicher, dass die Messungen vor dem Test standardisiert und zuverlässig sind.
- Maßnahmen nach dem Test: Erheben Sie nach der Intervention Daten nach dem Test, indem Sie die gleichen Maßnahmen wie vor dem Test verwenden. So können Sie Veränderungen im Laufe der Zeit beurteilen.
- Aufrechterhaltung der Datenkonsistenz: Stellen Sie sicher, dass die Datenerhebungsverfahren für alle Teilnehmer und Zeitpunkte konsistent sind, um Verzerrungen zu minimieren.
5. Daten auswerten
Sobald Sie Ihre Daten gesammelt haben, ist es an der Zeit, sie mit geeigneten statistischen Verfahren zu analysieren. Die Wahl der Analyse hängt von Ihren Forschungsfragen und der Art der gesammelten Daten ab.
- Statistische Analyse: Verwenden Sie Statistiksoftware, um Ihre Daten zu analysieren. Zu den gebräuchlichen Techniken gehören t-Tests , Varianzanalyse (ANOVA) , Regressionsanalyse und weitere, je nach Design und Variablen.
- Kontrolle von Störvariablen: Achten Sie auf mögliche Störvariablen und beziehen Sie sie als Kovariaten in Ihre Analyse ein, um genaue Ergebnisse zu gewährleisten.
Chi-Quadrat-Rechner :
t-Test-Rechner :
6. Ergebnisse interpretieren
Wenn die Analyse abgeschlossen ist, können Sie die Ergebnisse interpretieren, um aussagekräftige Schlussfolgerungen über die Beziehung zwischen den unabhängigen und abhängigen Variablen zu ziehen.
- Untersuchen Sie die Effektgrößen: Beurteilen Sie die Größenordnung der beobachteten Effekte, um ihre praktische Bedeutung zu bestimmen.
- Berücksichtigen Sie Signifikanzniveaus: Bestimmen Sie, ob die beobachteten Ergebnisse statistisch signifikant sind. Verstehen Sie die p-Werte und ihre Auswirkungen.
- Vergleichen Sie die Ergebnisse mit den Hypothesen: Bewerten Sie, ob Ihre Ergebnisse Ihre Hypothesen und Forschungsfragen unterstützen oder ablehnen.
7. Ziehen Sie Schlussfolgerungen
Ziehen Sie auf der Grundlage Ihrer Analyse und Interpretation der Ergebnisse Schlussfolgerungen zu den Forschungsfragen und -zielen, die Sie sich gesetzt haben.
- Kausale Schlussfolgerungen: Erläutern Sie, inwieweit Ihre Studie kausale Schlussfolgerungen zulässt. Machen Sie die Einschränkungen und möglichen alternativen Erklärungen für Ihre Ergebnisse transparent.
- Implikationen und Anwendungen: Berücksichtigen Sie die praktischen Implikationen Ihrer Forschung. Wie tragen Ihre Ergebnisse zum bestehenden Wissen bei und wie können sie in realen Kontexten angewendet werden?
- Zukünftige Forschung: Nennen Sie Bereiche für zukünftige Forschung und mögliche Verbesserungen im Studiendesign. Weisen Sie auf Einschränkungen oder Zwänge hin, die die Ergebnisse Ihrer Studie beeinflusst haben könnten.
Wenn Sie diese Schritte sorgfältig befolgen, können Sie eine strenge und informative quasi-experimentelle Studie durchführen, die das Wissen in Ihrem Forschungsbereich erweitert.
Beispiele für quasi-experimentelles Design
Quasi-experimentelles Design findet in einer Vielzahl von Forschungsbereichen Anwendung, einschließlich unternehmensbezogener und Marktforschungsszenarien. Im Folgenden finden Sie einige detaillierte Beispiele für die Anwendung dieser Forschungsmethode in der Praxis:
Beispiel 1: Bewertung der Auswirkungen einer neuen Marketingstrategie
Angenommen, ein Unternehmen möchte die Wirksamkeit einer neuen Marketingstrategie zur Steigerung des Absatzes bewerten. Die Durchführung eines kontrollierten Experiments ist aufgrund des bestehenden Kundenstamms des Unternehmens und der Schwierigkeit, die Kunden nach dem Zufallsprinzip verschiedenen Marketingansätzen zuzuordnen, möglicherweise nicht durchführbar. In diesem Fall kann ein quasi-experimentelles Design verwendet werden.
- Unabhängige Variable: Die neue Marketingstrategie.
- Abhängige Variable: Umsatzerlöse.
- Design: Das Unternehmen könnte die neue Strategie für eine Gruppe von Kunden umsetzen, während die bestehende Strategie für eine andere Gruppe beibehalten wird. Beide Gruppen werden auf der Grundlage ähnlicher demografischer Merkmale und Kaufhistorie ausgewählt, um eine Verzerrung der Auswahl zu vermeiden. Daten aus der Zeit vor der Umsetzung (Verkaufszahlen) können als Basis dienen, und nach der Umsetzung können Daten gesammelt werden, um die Auswirkungen der Strategie zu bewerten.
Beispiel 2: Bewertung der Effektivität von Mitarbeiterschulungsprogrammen
Im Zusammenhang mit der Personal- und Mitarbeiterentwicklung versuchen Unternehmen häufig, die Auswirkungen von Schulungsprogrammen zu bewerten. Eine randomisierte kontrollierte Studie (RCT) mit zufälliger Zuweisung ist möglicherweise nicht praktikabel oder ethisch vertretbar, da einige Mitarbeiter eine bestimmte Schulung mehr benötigen als andere. Stattdessen kann ein quasi-experimentelles Design verwendet werden.
- Unabhängige Variable: Schulungsprogramme für Mitarbeiter.
- Abhängige Variable: Leistungskennzahlen der Mitarbeiter, z. B. Produktivität oder Qualität der Arbeit.
- Design: Das Unternehmen kann Schulungsprogramme für Mitarbeiter anbieten, die Interesse bekunden oder einen bestimmten Bedarf aufweisen, und so eine selbst gewählte Behandlungsgruppe bilden. Eine vergleichbare Kontrollgruppe kann aus Mitarbeitern mit ähnlichen Aufgaben und Qualifikationen bestehen, die nicht an der Schulung teilgenommen haben. Die Leistungskennzahlen vor der Schulung können als Basis dienen, und nach der Schulung können Daten gesammelt werden, um die Auswirkungen der Schulungsprogramme zu bewerten.
Beispiel 3: Analyse der Auswirkungen einer Änderung der Steuerpolitik
In den Bereichen Wirtschaft und öffentliche Ordnung untersuchen Forscher häufig die Auswirkungen von Änderungen der Steuerpolitik auf das wirtschaftliche Verhalten. Die Durchführung eines kontrollierten Experiments ist in solchen Fällen praktisch unmöglich. Daher wird in der Regel ein quasi-experimentelles Design verwendet.
- Unabhängige Variable: Steuerpolitische Änderungen (z. B. Steuersatzanpassungen).
- Abhängige Variable: Wirtschaftsindikatoren, wie Verbraucherausgaben oder Unternehmensinvestitionen.
- Design: Die Forscher können Daten aus verschiedenen Regionen oder Gerichtsbarkeiten analysieren, in denen steuerpolitische Änderungen durchgeführt wurden. Eine Region könnte die Behandlungsgruppe (mit steuerpolitischen Änderungen) darstellen, während eine ähnliche Region ohne steuerpolitische Änderungen als Kontrollgruppe dient. Durch den Vergleich der Wirtschaftsdaten vor und nach der Änderung der Politik in beiden Gruppen können die Forscher die Auswirkungen der Änderungen der Steuerpolitik bewerten.
Diese Beispiele veranschaulichen, wie das quasi-experimentelle Design in verschiedenen Forschungskontexten angewandt werden kann und wertvolle Erkenntnisse über die Auswirkungen unabhängiger Variablen in realen Szenarien liefert, in denen kontrollierte Experimente nicht durchführbar oder ethisch vertretbar sind. Durch die sorgfältige Auswahl von Vergleichsgruppen und die Kontrolle potenzieller Verzerrungen können Forscher aussagekräftige Schlussfolgerungen ziehen und Entscheidungsprozesse beeinflussen.
Wie veröffentlicht man quasi-experimentelle Forschung?
Die Veröffentlichung Ihrer quasi-experimentellen Forschungsergebnisse ist ein entscheidender Schritt, um zum Wissen der wissenschaftlichen Gemeinschaft beizutragen. Wir werden die wesentlichen Aspekte einer effektiven Berichterstattung und Veröffentlichung Ihrer quasi-experimentellen Forschung untersuchen.
Strukturierung Ihrer Forschungsarbeit
Bei der Vorbereitung Ihrer Forschungsarbeit ist es wichtig, ein gut strukturiertes Format einzuhalten, um Klarheit und Verständlichkeit zu gewährleisten. Hier sind die wichtigsten Elemente, die Sie berücksichtigen sollten:
Titel und Zusammenfassung
- Titel: Verfassen Sie einen prägnanten und informativen Titel, der das Wesentliche Ihrer Studie widerspiegelt. Er sollte die wichtigste Forschungsfrage oder Hypothese enthalten.
- Zusammenfassung: Fassen Sie Ihre Forschungsarbeit in einer strukturierten Zusammenfassung zusammen, die den Zweck, die Methoden, die Ergebnisse und die Schlussfolgerungen enthält. Achten Sie darauf, dass sie einen klaren Überblick über Ihre Studie gibt.
- Hintergrund und Begründung: Stellen Sie den Kontext Ihrer Studie dar, indem Sie die Forschungslücke oder das Problem, das Ihre Studie behandelt, erläutern. Erläutern Sie, warum Ihre Forschung relevant und wichtig ist.
- Forschungsfragen oder Hypothesen: Geben Sie Ihre Forschungsfragen oder Hypothesen und deren Bedeutung klar an.
Literaturübersicht
- Übersicht über verwandte Arbeiten: Erörtern Sie relevante Literatur, die Ihre Forschung unterstützt. Heben Sie Studien mit ähnlichen Methoden oder Ergebnissen hervor und erklären Sie, wie Ihre Forschung in diesen Kontext passt.
- Teilnehmer: Beschreiben Sie die Teilnehmer Ihrer Studie, einschließlich ihrer Merkmale und wie Sie sie rekrutiert haben.
- Quasi-experimentelles Design: Erläutern Sie das von Ihnen gewählte Design im Detail, einschließlich der unabhängigen und abhängigen Variablen, der Verfahren und der getroffenen Kontrollmaßnahmen.
- Datenerhebung: Erläutern Sie die Methoden der Datenerhebung , die verwendeten Instrumente und alle Maßnahmen vor und nach dem Test.
- Datenanalyse: Beschreiben Sie die verwendeten statistischen Verfahren, einschließlich der Kontrolle von Störvariablen.
- Darstellung der Ergebnisse: Stellen Sie Ihre Ergebnisse übersichtlich dar, gegebenenfalls unter Verwendung von Tabellen, Diagrammen und deskriptiven Statistiken. Geben Sie ggf. p-Werte und Effektgrößen an.
- Interpretation der Ergebnisse: Erläutern Sie die Implikationen Ihrer Ergebnisse und wie sie sich auf Ihre Forschungsfragen oder Hypothesen beziehen.
- Interpretation und Implikationen: Analysieren Sie Ihre Ergebnisse im Kontext der vorhandenen Literatur und Theorien. Erörtern Sie die praktischen Implikationen Ihrer Ergebnisse.
- Beschränkungen: Gehen Sie auf die Beschränkungen Ihrer Studie ein, einschließlich möglicher Verzerrungen oder Gefährdungen der internen Validität.
- Künftige Forschung: Schlagen Sie Bereiche für künftige Forschung vor und erläutern Sie, wie Ihre Studie zum Fachgebiet beiträgt.
Ethische Erwägungen bei der Berichterstattung
Ethische Berichterstattung ist bei quasi-experimenteller Forschung von größter Bedeutung. Stellen Sie sicher, dass Sie die ethischen Standards einhalten, einschließlich:
- Informierte Zustimmung: Geben Sie klar an, dass die informierte Zustimmung aller Teilnehmer eingeholt wurde, und beschreiben Sie den Prozess der informierten Zustimmung.
- Schutz der Teilnehmer: Erläutern Sie, wie Sie die Rechte und das Wohlergehen Ihrer Teilnehmer während der gesamten Studie geschützt haben.
- Vertraulichkeit: Erläutern Sie, wie Sie die Privatsphäre und Anonymität gewahrt haben, insbesondere bei der Präsentation einzelner Daten.
- Offenlegung von Interessenkonflikten: Erklären Sie alle potenziellen Interessenkonflikte, die die Interpretation Ihrer Ergebnisse beeinflussen könnten.
Häufig zu vermeidende Fallstricke
Achten Sie bei der Berichterstattung über Ihre quasi-experimentelle Forschung auf häufige Fallstricke, die die Qualität und Wirkung Ihrer Arbeit beeinträchtigen können:
- Übergeneralisierung: Achten Sie darauf, dass Sie Ihre Ergebnisse nicht übergeneralisieren. Geben Sie klar die Grenzen Ihrer Studie und die Populationen an, auf die Ihre Ergebnisse angewendet werden können.
- Fehlinterpretation der Kausalität: Legen Sie klar die Grenzen des Kausalitätsschlusses in der quasi-experimentellen Forschung dar. Vermeiden Sie starke kausale Behauptungen, wenn sie nicht durch solide Beweise gestützt werden.
- Ignorieren ethischer Bedenken: Ethische Überlegungen sind von größter Bedeutung. Wenn Sie nicht über die informierte Zustimmung, die ethische Aufsicht und den Schutz der Teilnehmer berichten, kann dies die Glaubwürdigkeit Ihrer Studie untergraben.
Richtlinien für eine transparente Berichterstattung
Um die Transparenz und Reproduzierbarkeit Ihrer quasi-experimentellen Forschung zu verbessern, sollten Sie sich an etablierte Berichterstattungsrichtlinien halten, z. B:
- CONSORT-Erklärung: Wenn Ihre Studie Interventionen oder Behandlungen beinhaltet, befolgen Sie die CONSORT-Richtlinien für die transparente Berichterstattung über randomisierte kontrollierte Studien.
- STROBE-Erklärung: Für Beobachtungsstudien bietet die STROBE-Erklärung eine Anleitung für die Berichterstattung über wesentliche Elemente.
- PRISMA-Erklärung: Wenn Ihre Forschung systematische Übersichten oder Meta-Analysen umfasst, halten Sie sich an die PRISMA-Richtlinien.
- Transparent Reporting of Evaluations with Non-Randomized Designs (TREND): Die TREND-Richtlinien bieten spezifische Empfehlungen für die transparente Berichterstattung über nicht-randomisierte Designs, einschließlich quasi-experimenteller Forschung.
Indem Sie diese Berichterstattungsrichtlinien befolgen und die höchsten ethischen Standards einhalten, können Sie zum Wissenszuwachs in Ihrem Bereich beitragen und die Glaubwürdigkeit und Wirkung Ihrer quasi-experimentellen Forschungsergebnisse sicherstellen.
Quasi-Experimentelles Design - Herausforderungen
Die Durchführung einer quasi-experimentellen Studie kann mit Herausforderungen verbunden sein, die sich auf die Gültigkeit und Zuverlässigkeit der Ergebnisse auswirken können. Wir werfen einen Blick auf einige häufige Herausforderungen und stellen Strategien vor, wie Sie diese effektiv angehen können.
Auswahlverzerrung
Herausforderung: Selektionsverzerrungen treten auf, wenn sich nicht-randomisierte Gruppen systematisch in einer Weise unterscheiden, die das Ergebnis der Studie beeinflusst. Diese Verzerrung kann die Validität Ihrer Forschung untergraben, da sie impliziert, dass die Gruppen zu Beginn der Studie nicht gleichwertig sind.
Umgang mit Selektionsverzerrungen:
- Matching: Verwenden Sie Matching-Techniken, um vergleichbare Behandlungs- und Kontrollgruppen zu bilden. Passen Sie die Teilnehmer anhand relevanter Merkmale wie Alter, Geschlecht oder frühere Leistungen an, um ein Gleichgewicht zwischen den Gruppen herzustellen.
- Statistische Kontrollen: Verwenden Sie statistische Kontrollen, um die Unterschiede zwischen den Gruppen zu berücksichtigen. Beziehen Sie Kovariaten in Ihre Analyse ein, um mögliche Verzerrungen auszugleichen.
- Sensitivitätsanalyse: Führen Sie Sensitivitätsanalysen durch, um zu beurteilen, wie anfällig Ihre Ergebnisse für Selektionsverzerrungen sind. Untersuchen Sie verschiedene Szenarien, um die Auswirkungen einer möglichen Verzerrung auf Ihre Schlussfolgerungen zu verstehen.
Historische Effekte
Herausforderung: Historische Effekte beziehen sich auf externe Ereignisse oder Veränderungen im Laufe der Zeit, die die Ergebnisse der Studie beeinflussen. Diese externen Faktoren können Ihre Forschung vereiteln, indem sie Variablen einführen, die Sie nicht berücksichtigt haben.
Umgang mit historischen Effekten:
- Sammeln Sie historische Daten: Sammeln Sie umfangreiche historische Daten, um Trends und Muster zu verstehen, die Ihre Studie beeinflussen könnten. Wenn Sie über einen umfassenden historischen Kontext verfügen, können Sie historische Effekte besser erkennen und berücksichtigen.
- Kontrollgruppen: Beziehen Sie, wann immer möglich, Kontrollgruppen ein. Indem Sie die Ergebnisse der Behandlungsgruppe mit denen einer Kontrollgruppe vergleichen, können Sie externe Einflüsse berücksichtigen, die beide Gruppen gleichermaßen betreffen.
- Zeitreihenanalyse: Verwenden Sie gegebenenfalls eine Zeitreihenanalyse, um zeitliche Trends zu erkennen und zu berücksichtigen. Diese Methode hilft bei der Unterscheidung zwischen den Auswirkungen der unabhängigen Variable und externen Ereignissen.
Reifungseffekte
Herausforderung: Reifungseffekte treten auf, wenn sich die Teilnehmer während der Studie unabhängig von der Intervention natürlich verändern oder entwickeln. Diese Veränderungen können Ihre Ergebnisse verfälschen, so dass es schwierig ist, die beobachteten Effekte ausschließlich der unabhängigen Variable zuzuschreiben.
Umgang mit Reifungseffekten:
- Randomisierung: Verwenden Sie nach Möglichkeit eine Randomisierung, um die Reifungseffekte gleichmäßig auf die Behandlungs- und Kontrollgruppen zu verteilen. Die zufällige Zuweisung minimiert die Auswirkungen der Reifung als Störvariable.
- Abgestimmte Paare: Wenn eine Randomisierung nicht möglich ist, verwenden Sie abgestimmte Paare oder statistische Kontrollen, um sicherzustellen, dass beide Gruppen ähnliche Reifungseffekte erfahren.
- Kürzere Zeiträume: Begrenzen Sie die Dauer Ihrer Studie, um die Wahrscheinlichkeit signifikanter Reifungseffekte zu verringern. Kürzere Studien sind weniger anfällig für langfristige Reifungseffekte.
Regression zum Mittelwert
Herausforderung: Unter Regression zum Mittelwert versteht man die Tendenz, dass sich die Extremwerte einer Variablen bei einer erneuten Prüfung dem Mittelwert annähern. Dies kann den Eindruck erwecken, dass eine Intervention wirksam ist, obwohl es sich in Wirklichkeit um ein natürliches statistisches Phänomen handelt.
Umgang mit der Regression zum Mittelwert:
- Verwenden Sie Kontrollgruppen: Nehmen Sie Kontrollgruppen in Ihre Studie auf, um eine Ausgangsbasis für den Vergleich zu schaffen. Dies hilft, echte Interventionseffekte von einer Regression auf den Mittelwert zu unterscheiden.
- Mehrere Datenpunkte: Sammeln Sie zahlreiche Datenpunkte, um Muster und Trends zu erkennen. Wenn sich extreme Werte bei nachfolgenden Messungen auf den Mittelwert zurückbilden, kann dies eher auf eine Regression auf den Mittelwert als auf einen echten Interventionseffekt hindeuten.
- Statistische Analyse: Wenden Sie bei der Analyse Ihrer Daten statistische Verfahren an, die die Regression zum Mittelwert berücksichtigen. Techniken wie die Analyse der Kovarianz (ANCOVA) können dabei helfen, Unterschiede in der Ausgangslage zu kontrollieren.
Ausfälle und Sterblichkeit
Herausforderung: Unter Abwanderung versteht man den Verlust von Teilnehmern im Verlauf Ihrer Studie, während die Mortalität den dauerhaften Verlust von Teilnehmern bezeichnet. Hohe Schwundraten können zu Verzerrungen führen und die Repräsentativität Ihrer Stichprobe beeinträchtigen.
Umgang mit Abwanderung und Sterblichkeit:
- Sorgfältige Auswahl der Teilnehmer: Wählen Sie Teilnehmer aus, die wahrscheinlich während der gesamten Dauer der Studie engagiert bleiben. Berücksichtigen Sie Faktoren, die zur Abwanderung führen können, wie z. B. die Motivation und das Engagement der Teilnehmer.
- Anreize: Bieten Sie den Teilnehmern Anreize oder Entschädigungen, um sie zur weiteren Teilnahme zu bewegen.
- Follow-up-Strategien: Setzen Sie wirksame Follow-up-Strategien ein, um die Abwanderung zu verringern. Regelmäßige Kommunikation und Erinnerungen können helfen, die Teilnehmer bei der Stange zu halten.
- Sensitivitätsanalyse: Führen Sie Sensitivitätsanalysen durch, um die Auswirkungen von Abbruch und Sterblichkeit auf Ihre Ergebnisse zu bewerten. Vergleichen Sie die Merkmale der Teilnehmer, die die Studie abgebrochen haben, mit denen derjenigen, die die Studie abgeschlossen haben.
Effekte testen
Herausforderung: Testeffekte treten auf, wenn der bloße Akt des Testens oder Beurteilens von Teilnehmern deren spätere Leistung beeinflusst. Dieses Phänomen kann zu Veränderungen in der abhängigen Variable führen, die nicht mit der unabhängigen Variable zusammenhängen.
Umgang mit Testeffekten:
- Ausgewogene Tests: Wenn möglich, sollten Sie die Reihenfolge der Tests oder Beurteilungen zwischen Behandlungs- und Kontrollgruppe ausgleichen. Dies trägt dazu bei, die Testeffekte gleichmäßig auf die Gruppen zu verteilen.
- Kontrollgruppen: Führen Sie Kontrollgruppen ein, die denselben Test- oder Beurteilungsverfahren unterzogen werden wie die Behandlungsgruppe. Durch den Vergleich der beiden Gruppen können Sie feststellen, ob Testeffekte die Ergebnisse beeinflusst haben.
- Minimierung der Testhäufigkeit: Begrenzen Sie die Häufigkeit der Tests oder Beurteilungen, um die Wahrscheinlichkeit von Testeffekten zu verringern. Die Durchführung von weniger Beurteilungen kann die Auswirkungen wiederholter Tests auf die Teilnehmer abschwächen.
Wenn Sie diese allgemeinen Herausforderungen proaktiv angehen, können Sie die Validität und Zuverlässigkeit Ihrer quasi-experimentellen Studie verbessern und Ihre Ergebnisse robuster und vertrauenswürdiger machen.
Fazit zum Quasi-Experimentellen Aufbau
Das quasi-experimentelle Design ist ein leistungsfähiges Instrument, das Forschern hilft, Ursache-Wirkungs-Beziehungen in realen Situationen zu untersuchen , in denen eine strenge Kontrolle nicht immer möglich ist. Wenn Sie die Schlüsselkonzepte und die Arten von Versuchsplänen verstehen und wissen, wie Sie mit den Herausforderungen umgehen, können Sie solide Forschungsarbeiten durchführen und wertvolle Erkenntnisse zu Ihrem Fachgebiet beitragen. Denken Sie daran, dass das quasi-experimentelle Design die Lücke zwischen kontrollierten Experimenten und reinen Beobachtungsstudien schließt, was es zu einem unverzichtbaren Ansatz in verschiedenen Bereichen macht, von der Wirtschafts- und Marktforschung bis hin zur öffentlichen Politik und darüber hinaus. Ganz gleich, ob Sie Forscher, Student oder Entscheidungsträger sind, das Wissen über quasi-experimentelles Design befähigt Sie dazu, fundierte Entscheidungen zu treffen und positive Veränderungen in der Welt voranzutreiben.
Wie kann man quasi-experimentelles Design mit Echtzeit-Insights aufladen?
Wir stellen Ihnen Appinio vor, die Echtzeit-Marktforschungsplattform, die die Welt des quasi-experimentellen Designs verändert. Stellen Sie sich vor, Sie könnten Ihre eigene Marktforschung in wenigen Minuten durchführen und so verwertbare Erkenntnisse gewinnen, die Ihre datengestützten Entscheidungen unterstützen. Appinio kümmert sich um die Forschung und die technische Komplexität, damit Sie sich auf das konzentrieren können, was für Ihr Unternehmen wirklich wichtig ist.
Das sind die Gründe, warum Appinio so einzigartig ist:
- Blitzschnelle Erkenntnisse: Von der Formulierung der Fragen bis zur Aufdeckung der Erkenntnisse liefert Appinio Ergebnisse innerhalb von Minuten und stellt sicher, dass Sie die Antworten erhalten, die Sie brauchen, wenn Sie sie brauchen.
- Kein Forschungsdiplom erforderlich: Unsere intuitive Plattform ist für jedermann geeignet, so dass kein Forschungsdiplom erforderlich ist. Jeder kann sofort einsteigen und die Vorteile von Echtzeit-Konsumentenanalysen nutzen.
- Globale Reichweite, lokales Know-how: Mit Zugang zu über 90 Ländern und der Möglichkeit, präzise Zielgruppen auf der Grundlage von mehr als 1200 Merkmalen zu definieren, können Sie quasi-experimentelle Forschung auf globaler Ebene durchführen und gleichzeitig den lokalen Bezug wahren.
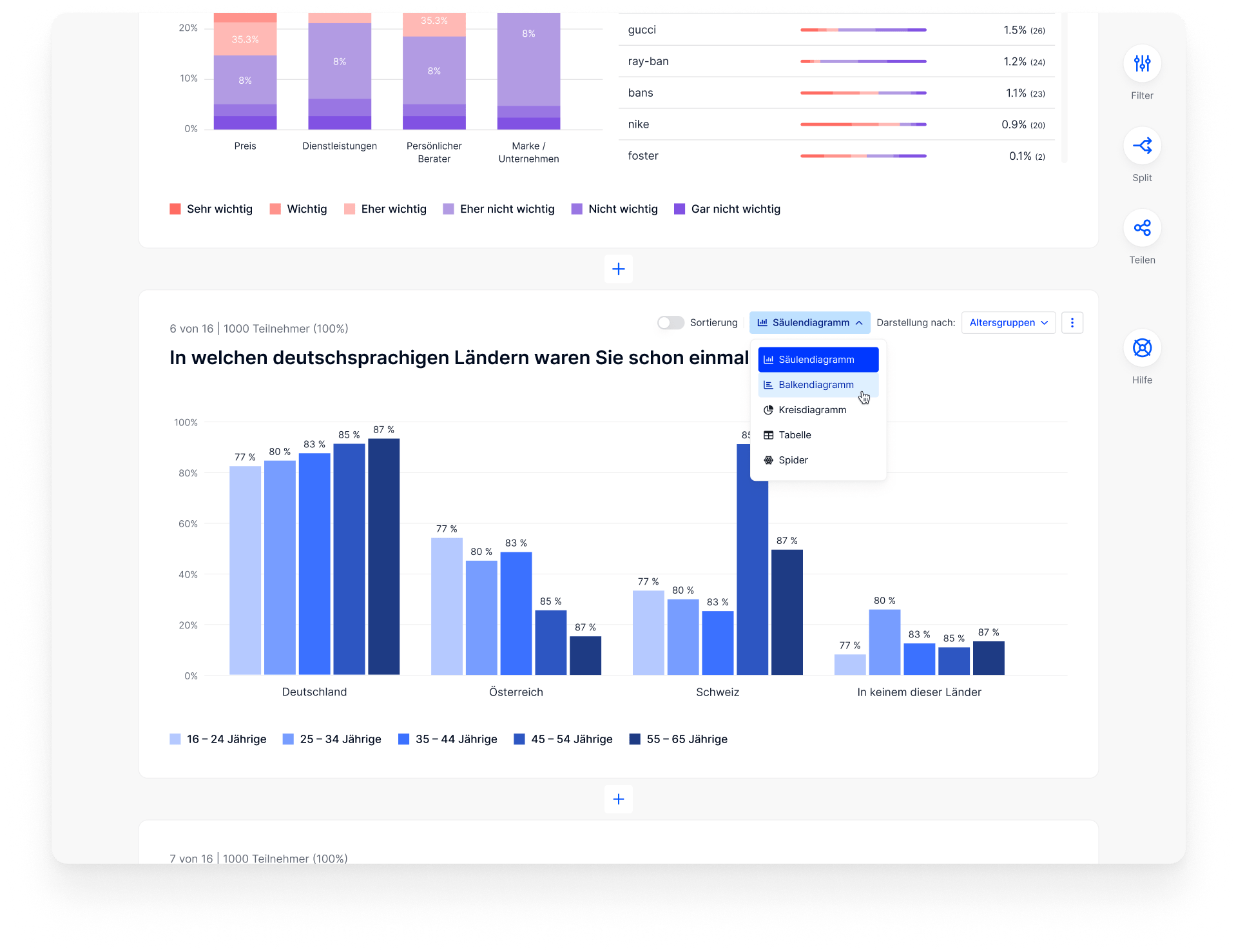
Kostenlosen Zugang zur Appinio Platform erhalten!
Direkt ins Postfach! 📫
Jetzt anmelden und regelmäßig Updates zu den neuesten Reports und/oder Produktneuheiten erhalten.
News und Updates zum Thema Marktforschung - Direkt in's Postfach! 💌
Weitere interessante Artikel
11.09.2024 | 36min Lesezeit
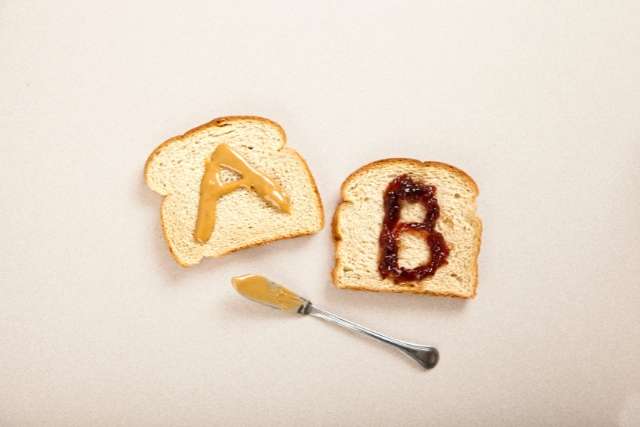
11.09.2024 | 44min Lesezeit
Was ist A/B-Testing? Leitfaden, Tools, Beispiele
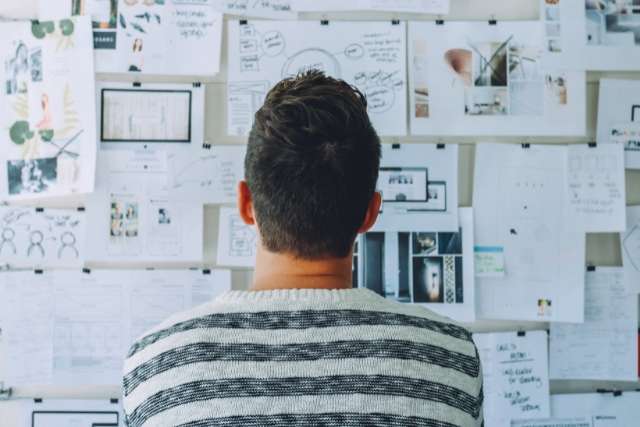
10.09.2024 | 33min Lesezeit
Kausalforschung: Definition, Aufbau, Tipps, Beispiele

IMAGES
VIDEO
COMMENTS
Learn what quasi-experimental design is, how it differs from true experimental design, and what types of quasi-experiments exist. See examples of quasi-experiments in psychology, education, and other fields.
Learn about the types, methods, and steps of quasi-experimental design, a research method that evaluates causal relationships without full control over the independent variable. See examples of quasi-experimental designs in education, health, and policy.
Key Takeaways. Quasi-experimental research involves the manipulation of an independent variable without the random assignment of participants to conditions or orders of conditions. Among the important types are nonequivalent groups designs, pretest-posttest, and interrupted time-series designs.
Quasi-experimental research is a design that closely resembles experimental research but is different. The term "quasi" means "resembling," so you can think of it as a cousin to actual experiments. ... Types of Quasi-Experimental Designs and Examples. Quasi-experimental studies use various methods, depending on the scenario. Natural ...
Quasi-experimental research designs are a type of research design that is similar to experimental designs but doesn't give full control over the independent variable (s) like true experimental designs do. In a quasi-experimental design, the researcher changes or watches an independent variable, but the participants are not put into groups at ...
In traditional experimental designs, randomization is a powerful tool for ensuring that groups are equivalent at the outset of a study. However, quasi-experimental design often involves non-randomization due to the nature of the research. This means that participants are not randomly assigned to treatment and control groups.
A quasi-experimental design is used when it's not logistically feasible or ethical to conduct randomized, controlled trials. As its name suggests, a quasi-experimental design is almost a true experiment. However, researchers don't randomly select elements or participants in this type of research. Researchers prefer to apply quasi-experimental ...
Specifically, we describe four quasi-experimental designs - one-group pretest-posttest designs, non-equivalent group designs, regression discontinuity designs, and interrupted time-series designs - and their statistical analyses in detail. Both simple quasi-experimental designs and embellishments of these simple designs are presented.
7.4 Combination Designs. A type of quasi-experimental design that is generally better than either the nonequivalent groups design or the pretest-posttest design is one that combines elements of both. There is a treatment group that is given a pretest, receives a treatment, and then is given a posttest.
Quasi-Experimental Design: Types, Examples, Pros, and Cons. A quasi-experimental design can be a great option when ethical or practical concerns make true experiments impossible, but the research methodology does have its drawbacks. Learn all the ins and outs of a quasi-experimental design. A quasi-experimental design can be a great option when ...
Quasi-Experimental Designs In this major section, we introduce a common type of research design called the quasi-experimental research design. The quasi-experimental research design, also defined in A quasi-experimental research design is the use of methods and procedures to make observations in a study that is structured similar to an experiment,
Quasi-Experimental Research Designs by Bruce A. Thyer. This pocket guide describes the logic, design, and conduct of the range of quasi-experimental designs, encompassing pre-experiments, quasi-experiments making use of a control or comparison group, and time-series designs. An introductory chapter describes the valuable role these types of ...
A quasi-experiment is an empirical interventional study used to estimate the causal impact of an intervention on target population without random assignment. Quasi-experimental research shares similarities with the traditional experimental design or randomized controlled trial, but it specifically lacks the element of random assignment to ...
A quasi-experimental design is a non-randomized study design used to evaluate the effect of an intervention. The intervention can be a training program, a policy change or a medical treatment. Unlike a true experiment, in a quasi-experimental study the choice of who gets the intervention and who doesn't is not randomized.
Key Takeaways. Quasi-experimental research involves the manipulation of an independent variable without the random assignment of participants to conditions or orders of conditions. Among the important types are nonequivalent groups designs, pretest-posttest, and interrupted time-series designs.
Research. Quasi-Experimental Research: Types, Examples & Application. Let's say you want to study the effects of a new drug on lowering blood pressure. You could randomly assign half of the participants to receive the drug and the other half to receive a placebo. However, this isn't fair to the patients in the placebo group.
Alternative research methods are needed to test interventions for their effectiveness in many real-world settings—and later when evidence-based interventions are known, ... and Stanley JC, "Experimental and Quasi-Experimental Designs for Research on Teaching." In Gage NL (ed.), Handbook of Research on Teaching. Chicago: Rand McNally, 1963.
In medical informatics, the quasi-experimental, sometimes called the pre-post intervention, design often is used to evaluate the benefits of specific interventions. The increasing capacity of health care institutions to collect routine clinical data has led to the growing use of quasi-experimental study designs in the field of medical ...
A quasi-experimental design is common in social research when a true experimental design may not be possible. Overall, the design types are very similar, except that quasi-experimental design does ...
An overview of educational research methodology, including literature review and discussion of approaches to research, experimental design, statistical analysis, ethics, and rhetorical presentation of research findings. Campbell, D. T., & Stanley, J. C. (1963). Experimental and quasi-experimental designs for research. Boston: Houghton Mifflin.
Quasi-experimental designs allow implementation scientists to conduct rigorous studies in these contexts, albeit with certain limitations. We briefly review the characteristics of these designs here; other recent review articles are available for the interested reader (e.g. Handley et al., 2018). 2.1.
In the past few decades, we have seen a rapid proliferation in the use of quasi-experimental research designs in education research. This trend, stemming in part from the "credibility revolution" in the social sciences, particularly economics, is notable along with the increasing use of randomized controlled trials in the strive toward rigorous causal inference.
Abstract. Quasi-experimental research designs are the most widely used research approach employed to evaluate the outcomes of social work programs and policies. This new volume describes the logic, design, and conduct of the range of such designs, encompassing pre-experiments, quasi-experiments making use of a control or comparison group, and ...
Recent high-profile incidents involving the shooting or killing of unarmed Black men have intensified the debate about how police violence affects trust in the criminal justice system, particularly among communities of color. In this article, we propose a quasi-experimental design that leverages the timing of the shooting of Jacob Blake by the Kenosha Police Department relative to when a large ...
Das quasi-experimentelle Design bietet einen wertvollen Forschungsansatz, aber wie jede Methode hat auch diese ihre eigenen Vor- und Nachteile. Lassen Sie uns diese im Detail untersuchen. Vorteile des quasi-experimentellen Designs. Das quasi-experimentelle Design bietet mehrere Vorteile, die es zu einem wertvollen Instrument in der Forschung ...